mirror of
https://github.com/invoke-ai/InvokeAI
synced 2024-08-30 20:32:17 +00:00
Merge branch 'main' into ryan/model-cache-logging-only
This commit is contained in:
commit
096d195d6e
@ -10,6 +10,20 @@ To use a community workflow, download the the `.json` node graph file and load i
|
||||
|
||||
--------------------------------
|
||||
|
||||
--------------------------------
|
||||
### Make 3D
|
||||
|
||||
**Description:** Create compelling 3D stereo images from 2D originals.
|
||||
|
||||
**Node Link:** [https://gitlab.com/srcrr/shift3d/-/raw/main/make3d.py](https://gitlab.com/srcrr/shift3d)
|
||||
|
||||
**Example Node Graph:** https://gitlab.com/srcrr/shift3d/-/raw/main/example-workflow.json?ref_type=heads&inline=false
|
||||
|
||||
**Output Examples**
|
||||
|
||||
{: style="height:512px;width:512px"}
|
||||
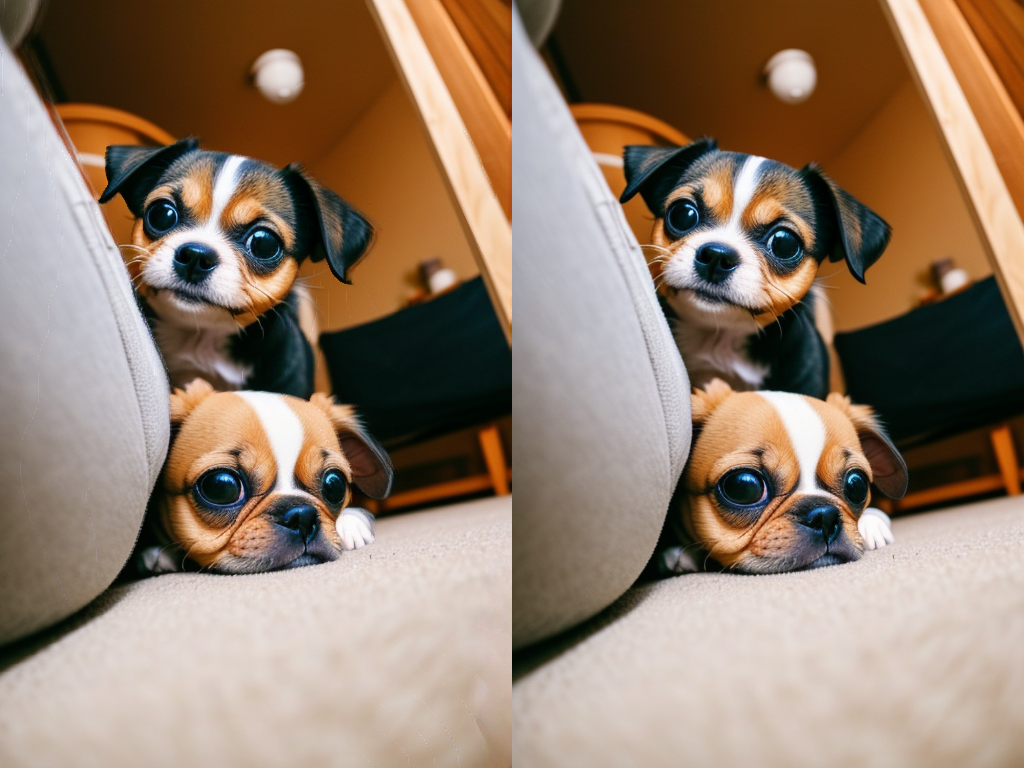{: style="height:512px;width:512px"}
|
||||
|
||||
--------------------------------
|
||||
### Ideal Size
|
||||
|
||||
|
@ -68,6 +68,7 @@ class FieldDescriptions:
|
||||
height = "Height of output (px)"
|
||||
control = "ControlNet(s) to apply"
|
||||
ip_adapter = "IP-Adapter to apply"
|
||||
t2i_adapter = "T2I-Adapter(s) to apply"
|
||||
denoised_latents = "Denoised latents tensor"
|
||||
latents = "Latents tensor"
|
||||
strength = "Strength of denoising (proportional to steps)"
|
||||
|
@ -1,5 +1,4 @@
|
||||
import math
|
||||
import os
|
||||
import re
|
||||
from pathlib import Path
|
||||
from typing import Optional, TypedDict
|
||||
@ -11,6 +10,7 @@ from PIL import Image, ImageDraw, ImageFilter, ImageFont, ImageOps
|
||||
from PIL.Image import Image as ImageType
|
||||
from pydantic import validator
|
||||
|
||||
import invokeai.assets.fonts as font_assets
|
||||
from invokeai.app.invocations.baseinvocation import (
|
||||
BaseInvocation,
|
||||
InputField,
|
||||
@ -138,6 +138,7 @@ def generate_face_box_mask(
|
||||
chunk_x_offset: int = 0,
|
||||
chunk_y_offset: int = 0,
|
||||
draw_mesh: bool = True,
|
||||
check_bounds: bool = True,
|
||||
) -> list[FaceResultData]:
|
||||
result = []
|
||||
mask_pil = None
|
||||
@ -217,7 +218,7 @@ def generate_face_box_mask(
|
||||
im_width, im_height = pil_image.size
|
||||
over_w = im_width * 0.1
|
||||
over_h = im_height * 0.1
|
||||
if (
|
||||
if not check_bounds or (
|
||||
(left_side >= -over_w)
|
||||
and (right_side < im_width + over_w)
|
||||
and (top_side >= -over_h)
|
||||
@ -345,6 +346,7 @@ def get_faces_list(
|
||||
chunk_x_offset=0,
|
||||
chunk_y_offset=0,
|
||||
draw_mesh=draw_mesh,
|
||||
check_bounds=False,
|
||||
)
|
||||
if should_chunk or len(result) == 0:
|
||||
context.services.logger.info("FaceTools --> Chunking image (chunk toggled on, or no face found in full image).")
|
||||
@ -402,7 +404,7 @@ def get_faces_list(
|
||||
return all_faces
|
||||
|
||||
|
||||
@invocation("face_off", title="FaceOff", tags=["image", "faceoff", "face", "mask"], category="image", version="1.0.0")
|
||||
@invocation("face_off", title="FaceOff", tags=["image", "faceoff", "face", "mask"], category="image", version="1.0.1")
|
||||
class FaceOffInvocation(BaseInvocation):
|
||||
"""Bound, extract, and mask a face from an image using MediaPipe detection"""
|
||||
|
||||
@ -496,7 +498,7 @@ class FaceOffInvocation(BaseInvocation):
|
||||
return output
|
||||
|
||||
|
||||
@invocation("face_mask_detection", title="FaceMask", tags=["image", "face", "mask"], category="image", version="1.0.0")
|
||||
@invocation("face_mask_detection", title="FaceMask", tags=["image", "face", "mask"], category="image", version="1.0.1")
|
||||
class FaceMaskInvocation(BaseInvocation):
|
||||
"""Face mask creation using mediapipe face detection"""
|
||||
|
||||
@ -614,7 +616,7 @@ class FaceMaskInvocation(BaseInvocation):
|
||||
|
||||
|
||||
@invocation(
|
||||
"face_identifier", title="FaceIdentifier", tags=["image", "face", "identifier"], category="image", version="1.0.0"
|
||||
"face_identifier", title="FaceIdentifier", tags=["image", "face", "identifier"], category="image", version="1.0.1"
|
||||
)
|
||||
class FaceIdentifierInvocation(BaseInvocation):
|
||||
"""Outputs an image with detected face IDs printed on each face. For use with other FaceTools."""
|
||||
@ -641,9 +643,9 @@ class FaceIdentifierInvocation(BaseInvocation):
|
||||
draw_mesh=False,
|
||||
)
|
||||
|
||||
path = Path(__file__).resolve().parent.parent.parent
|
||||
font_path = os.path.abspath(path / "assets/fonts/inter/Inter-Regular.ttf")
|
||||
font = ImageFont.truetype(font_path, FONT_SIZE)
|
||||
# Note - font may be found either in the repo if running an editable install, or in the venv if running a package install
|
||||
font_path = [x for x in [Path(y, "inter/Inter-Regular.ttf") for y in font_assets.__path__] if x.exists()]
|
||||
font = ImageFont.truetype(font_path[0].as_posix(), FONT_SIZE)
|
||||
|
||||
# Paste face IDs on the output image
|
||||
draw = ImageDraw.Draw(image)
|
||||
|
@ -11,6 +11,7 @@ import torchvision.transforms as T
|
||||
from diffusers import AutoencoderKL, AutoencoderTiny
|
||||
from diffusers.image_processor import VaeImageProcessor
|
||||
from diffusers.models import UNet2DConditionModel
|
||||
from diffusers.models.adapter import FullAdapterXL, T2IAdapter
|
||||
from diffusers.models.attention_processor import (
|
||||
AttnProcessor2_0,
|
||||
LoRAAttnProcessor2_0,
|
||||
@ -33,6 +34,7 @@ from invokeai.app.invocations.primitives import (
|
||||
LatentsOutput,
|
||||
build_latents_output,
|
||||
)
|
||||
from invokeai.app.invocations.t2i_adapter import T2IAdapterField
|
||||
from invokeai.app.util.controlnet_utils import prepare_control_image
|
||||
from invokeai.app.util.step_callback import stable_diffusion_step_callback
|
||||
from invokeai.backend.ip_adapter.ip_adapter import IPAdapter, IPAdapterPlus
|
||||
@ -47,6 +49,7 @@ from ...backend.stable_diffusion.diffusers_pipeline import (
|
||||
ControlNetData,
|
||||
IPAdapterData,
|
||||
StableDiffusionGeneratorPipeline,
|
||||
T2IAdapterData,
|
||||
image_resized_to_grid_as_tensor,
|
||||
)
|
||||
from ...backend.stable_diffusion.diffusion.shared_invokeai_diffusion import PostprocessingSettings
|
||||
@ -196,7 +199,7 @@ def get_scheduler(
|
||||
title="Denoise Latents",
|
||||
tags=["latents", "denoise", "txt2img", "t2i", "t2l", "img2img", "i2i", "l2l"],
|
||||
category="latents",
|
||||
version="1.1.0",
|
||||
version="1.2.0",
|
||||
)
|
||||
class DenoiseLatentsInvocation(BaseInvocation):
|
||||
"""Denoises noisy latents to decodable images"""
|
||||
@ -226,9 +229,12 @@ class DenoiseLatentsInvocation(BaseInvocation):
|
||||
ip_adapter: Optional[IPAdapterField] = InputField(
|
||||
description=FieldDescriptions.ip_adapter, title="IP-Adapter", default=None, input=Input.Connection, ui_order=6
|
||||
)
|
||||
t2i_adapter: Union[T2IAdapterField, list[T2IAdapterField]] = InputField(
|
||||
description=FieldDescriptions.t2i_adapter, title="T2I-Adapter", default=None, input=Input.Connection, ui_order=7
|
||||
)
|
||||
latents: Optional[LatentsField] = InputField(description=FieldDescriptions.latents, input=Input.Connection)
|
||||
denoise_mask: Optional[DenoiseMaskField] = InputField(
|
||||
default=None, description=FieldDescriptions.mask, input=Input.Connection, ui_order=7
|
||||
default=None, description=FieldDescriptions.mask, input=Input.Connection, ui_order=8
|
||||
)
|
||||
|
||||
@validator("cfg_scale")
|
||||
@ -451,6 +457,91 @@ class DenoiseLatentsInvocation(BaseInvocation):
|
||||
end_step_percent=ip_adapter.end_step_percent,
|
||||
)
|
||||
|
||||
def run_t2i_adapters(
|
||||
self,
|
||||
context: InvocationContext,
|
||||
t2i_adapter: Optional[Union[T2IAdapterField, list[T2IAdapterField]]],
|
||||
latents_shape: list[int],
|
||||
do_classifier_free_guidance: bool,
|
||||
) -> Optional[list[T2IAdapterData]]:
|
||||
if t2i_adapter is None:
|
||||
return None
|
||||
|
||||
# Handle the possibility that t2i_adapter could be a list or a single T2IAdapterField.
|
||||
if isinstance(t2i_adapter, T2IAdapterField):
|
||||
t2i_adapter = [t2i_adapter]
|
||||
|
||||
if len(t2i_adapter) == 0:
|
||||
return None
|
||||
|
||||
t2i_adapter_data = []
|
||||
for t2i_adapter_field in t2i_adapter:
|
||||
t2i_adapter_model_info = context.services.model_manager.get_model(
|
||||
model_name=t2i_adapter_field.t2i_adapter_model.model_name,
|
||||
model_type=ModelType.T2IAdapter,
|
||||
base_model=t2i_adapter_field.t2i_adapter_model.base_model,
|
||||
context=context,
|
||||
)
|
||||
image = context.services.images.get_pil_image(t2i_adapter_field.image.image_name)
|
||||
|
||||
# The max_unet_downscale is the maximum amount that the UNet model downscales the latent image internally.
|
||||
if t2i_adapter_field.t2i_adapter_model.base_model == BaseModelType.StableDiffusion1:
|
||||
max_unet_downscale = 8
|
||||
elif t2i_adapter_field.t2i_adapter_model.base_model == BaseModelType.StableDiffusionXL:
|
||||
max_unet_downscale = 4
|
||||
else:
|
||||
raise ValueError(
|
||||
f"Unexpected T2I-Adapter base model type: '{t2i_adapter_field.t2i_adapter_model.base_model}'."
|
||||
)
|
||||
|
||||
t2i_adapter_model: T2IAdapter
|
||||
with t2i_adapter_model_info as t2i_adapter_model:
|
||||
total_downscale_factor = t2i_adapter_model.total_downscale_factor
|
||||
if isinstance(t2i_adapter_model.adapter, FullAdapterXL):
|
||||
# HACK(ryand): Work around a bug in FullAdapterXL. This is being addressed upstream in diffusers by
|
||||
# this PR: https://github.com/huggingface/diffusers/pull/5134.
|
||||
total_downscale_factor = total_downscale_factor // 2
|
||||
|
||||
# Resize the T2I-Adapter input image.
|
||||
# We select the resize dimensions so that after the T2I-Adapter's total_downscale_factor is applied, the
|
||||
# result will match the latent image's dimensions after max_unet_downscale is applied.
|
||||
t2i_input_height = latents_shape[2] // max_unet_downscale * total_downscale_factor
|
||||
t2i_input_width = latents_shape[3] // max_unet_downscale * total_downscale_factor
|
||||
|
||||
# Note: We have hard-coded `do_classifier_free_guidance=False`. This is because we only want to prepare
|
||||
# a single image. If CFG is enabled, we will duplicate the resultant tensor after applying the
|
||||
# T2I-Adapter model.
|
||||
#
|
||||
# Note: We re-use the `prepare_control_image(...)` from ControlNet for T2I-Adapter, because it has many
|
||||
# of the same requirements (e.g. preserving binary masks during resize).
|
||||
t2i_image = prepare_control_image(
|
||||
image=image,
|
||||
do_classifier_free_guidance=False,
|
||||
width=t2i_input_width,
|
||||
height=t2i_input_height,
|
||||
num_channels=t2i_adapter_model.config.in_channels,
|
||||
device=t2i_adapter_model.device,
|
||||
dtype=t2i_adapter_model.dtype,
|
||||
resize_mode=t2i_adapter_field.resize_mode,
|
||||
)
|
||||
|
||||
adapter_state = t2i_adapter_model(t2i_image)
|
||||
|
||||
if do_classifier_free_guidance:
|
||||
for idx, value in enumerate(adapter_state):
|
||||
adapter_state[idx] = torch.cat([value] * 2, dim=0)
|
||||
|
||||
t2i_adapter_data.append(
|
||||
T2IAdapterData(
|
||||
adapter_state=adapter_state,
|
||||
weight=t2i_adapter_field.weight,
|
||||
begin_step_percent=t2i_adapter_field.begin_step_percent,
|
||||
end_step_percent=t2i_adapter_field.end_step_percent,
|
||||
)
|
||||
)
|
||||
|
||||
return t2i_adapter_data
|
||||
|
||||
# original idea by https://github.com/AmericanPresidentJimmyCarter
|
||||
# TODO: research more for second order schedulers timesteps
|
||||
def init_scheduler(self, scheduler, device, steps, denoising_start, denoising_end):
|
||||
@ -522,6 +613,12 @@ class DenoiseLatentsInvocation(BaseInvocation):
|
||||
|
||||
mask, masked_latents = self.prep_inpaint_mask(context, latents)
|
||||
|
||||
# TODO(ryand): I have hard-coded `do_classifier_free_guidance=True` to mirror the behaviour of ControlNets,
|
||||
# below. Investigate whether this is appropriate.
|
||||
t2i_adapter_data = self.run_t2i_adapters(
|
||||
context, self.t2i_adapter, latents.shape, do_classifier_free_guidance=True
|
||||
)
|
||||
|
||||
# Get the source node id (we are invoking the prepared node)
|
||||
graph_execution_state = context.services.graph_execution_manager.get(context.graph_execution_state_id)
|
||||
source_node_id = graph_execution_state.prepared_source_mapping[self.id]
|
||||
@ -602,8 +699,9 @@ class DenoiseLatentsInvocation(BaseInvocation):
|
||||
masked_latents=masked_latents,
|
||||
num_inference_steps=num_inference_steps,
|
||||
conditioning_data=conditioning_data,
|
||||
control_data=controlnet_data, # list[ControlNetData],
|
||||
ip_adapter_data=ip_adapter_data, # IPAdapterData,
|
||||
control_data=controlnet_data,
|
||||
ip_adapter_data=ip_adapter_data,
|
||||
t2i_adapter_data=t2i_adapter_data,
|
||||
callback=step_callback,
|
||||
)
|
||||
|
||||
|
83
invokeai/app/invocations/t2i_adapter.py
Normal file
83
invokeai/app/invocations/t2i_adapter.py
Normal file
@ -0,0 +1,83 @@
|
||||
from typing import Union
|
||||
|
||||
from pydantic import BaseModel, Field
|
||||
|
||||
from invokeai.app.invocations.baseinvocation import (
|
||||
BaseInvocation,
|
||||
BaseInvocationOutput,
|
||||
FieldDescriptions,
|
||||
Input,
|
||||
InputField,
|
||||
InvocationContext,
|
||||
OutputField,
|
||||
UIType,
|
||||
invocation,
|
||||
invocation_output,
|
||||
)
|
||||
from invokeai.app.invocations.controlnet_image_processors import CONTROLNET_RESIZE_VALUES
|
||||
from invokeai.app.invocations.primitives import ImageField
|
||||
from invokeai.backend.model_management.models.base import BaseModelType
|
||||
|
||||
|
||||
class T2IAdapterModelField(BaseModel):
|
||||
model_name: str = Field(description="Name of the T2I-Adapter model")
|
||||
base_model: BaseModelType = Field(description="Base model")
|
||||
|
||||
|
||||
class T2IAdapterField(BaseModel):
|
||||
image: ImageField = Field(description="The T2I-Adapter image prompt.")
|
||||
t2i_adapter_model: T2IAdapterModelField = Field(description="The T2I-Adapter model to use.")
|
||||
weight: Union[float, list[float]] = Field(default=1, description="The weight given to the T2I-Adapter")
|
||||
begin_step_percent: float = Field(
|
||||
default=0, ge=0, le=1, description="When the T2I-Adapter is first applied (% of total steps)"
|
||||
)
|
||||
end_step_percent: float = Field(
|
||||
default=1, ge=0, le=1, description="When the T2I-Adapter is last applied (% of total steps)"
|
||||
)
|
||||
resize_mode: CONTROLNET_RESIZE_VALUES = Field(default="just_resize", description="The resize mode to use")
|
||||
|
||||
|
||||
@invocation_output("t2i_adapter_output")
|
||||
class T2IAdapterOutput(BaseInvocationOutput):
|
||||
t2i_adapter: T2IAdapterField = OutputField(description=FieldDescriptions.t2i_adapter, title="T2I Adapter")
|
||||
|
||||
|
||||
@invocation(
|
||||
"t2i_adapter", title="T2I-Adapter", tags=["t2i_adapter", "control"], category="t2i_adapter", version="1.0.0"
|
||||
)
|
||||
class T2IAdapterInvocation(BaseInvocation):
|
||||
"""Collects T2I-Adapter info to pass to other nodes."""
|
||||
|
||||
# Inputs
|
||||
image: ImageField = InputField(description="The IP-Adapter image prompt.")
|
||||
ip_adapter_model: T2IAdapterModelField = InputField(
|
||||
description="The T2I-Adapter model.",
|
||||
title="T2I-Adapter Model",
|
||||
input=Input.Direct,
|
||||
ui_order=-1,
|
||||
)
|
||||
weight: Union[float, list[float]] = InputField(
|
||||
default=1, ge=0, description="The weight given to the T2I-Adapter", ui_type=UIType.Float, title="Weight"
|
||||
)
|
||||
begin_step_percent: float = InputField(
|
||||
default=0, ge=-1, le=2, description="When the T2I-Adapter is first applied (% of total steps)"
|
||||
)
|
||||
end_step_percent: float = InputField(
|
||||
default=1, ge=0, le=1, description="When the T2I-Adapter is last applied (% of total steps)"
|
||||
)
|
||||
resize_mode: CONTROLNET_RESIZE_VALUES = InputField(
|
||||
default="just_resize",
|
||||
description="The resize mode applied to the T2I-Adapter input image so that it matches the target output size.",
|
||||
)
|
||||
|
||||
def invoke(self, context: InvocationContext) -> T2IAdapterOutput:
|
||||
return T2IAdapterOutput(
|
||||
t2i_adapter=T2IAdapterField(
|
||||
image=self.image,
|
||||
t2i_adapter_model=self.ip_adapter_model,
|
||||
weight=self.weight,
|
||||
begin_step_percent=self.begin_step_percent,
|
||||
end_step_percent=self.end_step_percent,
|
||||
resize_mode=self.resize_mode,
|
||||
)
|
||||
)
|
@ -4,12 +4,14 @@ from typing import Literal
|
||||
|
||||
import cv2 as cv
|
||||
import numpy as np
|
||||
import torch
|
||||
from basicsr.archs.rrdbnet_arch import RRDBNet
|
||||
from PIL import Image
|
||||
from realesrgan import RealESRGANer
|
||||
|
||||
from invokeai.app.invocations.primitives import ImageField, ImageOutput
|
||||
from invokeai.app.models.image import ImageCategory, ResourceOrigin
|
||||
from invokeai.backend.util.devices import choose_torch_device
|
||||
|
||||
from .baseinvocation import BaseInvocation, InputField, InvocationContext, invocation
|
||||
|
||||
@ -22,13 +24,19 @@ ESRGAN_MODELS = Literal[
|
||||
"RealESRGAN_x2plus.pth",
|
||||
]
|
||||
|
||||
if choose_torch_device() == torch.device("mps"):
|
||||
from torch import mps
|
||||
|
||||
@invocation("esrgan", title="Upscale (RealESRGAN)", tags=["esrgan", "upscale"], category="esrgan", version="1.0.0")
|
||||
|
||||
@invocation("esrgan", title="Upscale (RealESRGAN)", tags=["esrgan", "upscale"], category="esrgan", version="1.1.0")
|
||||
class ESRGANInvocation(BaseInvocation):
|
||||
"""Upscales an image using RealESRGAN."""
|
||||
|
||||
image: ImageField = InputField(description="The input image")
|
||||
model_name: ESRGAN_MODELS = InputField(default="RealESRGAN_x4plus.pth", description="The Real-ESRGAN model to use")
|
||||
tile_size: int = InputField(
|
||||
default=400, ge=0, description="Tile size for tiled ESRGAN upscaling (0=tiling disabled)"
|
||||
)
|
||||
|
||||
def invoke(self, context: InvocationContext) -> ImageOutput:
|
||||
image = context.services.images.get_pil_image(self.image.image_name)
|
||||
@ -86,9 +94,11 @@ class ESRGANInvocation(BaseInvocation):
|
||||
model_path=str(models_path / esrgan_model_path),
|
||||
model=rrdbnet_model,
|
||||
half=False,
|
||||
tile=self.tile_size,
|
||||
)
|
||||
|
||||
# prepare image - Real-ESRGAN uses cv2 internally, and cv2 uses BGR vs RGB for PIL
|
||||
# TODO: This strips the alpha... is that okay?
|
||||
cv_image = cv.cvtColor(np.array(image.convert("RGB")), cv.COLOR_RGB2BGR)
|
||||
|
||||
# We can pass an `outscale` value here, but it just resizes the image by that factor after
|
||||
@ -99,6 +109,10 @@ class ESRGANInvocation(BaseInvocation):
|
||||
# back to PIL
|
||||
pil_image = Image.fromarray(cv.cvtColor(upscaled_image, cv.COLOR_BGR2RGB)).convert("RGBA")
|
||||
|
||||
torch.cuda.empty_cache()
|
||||
if choose_torch_device() == torch.device("mps"):
|
||||
mps.empty_cache()
|
||||
|
||||
image_dto = context.services.images.create(
|
||||
image=pil_image,
|
||||
image_origin=ResourceOrigin.INTERNAL,
|
||||
|
@ -255,6 +255,7 @@ class InvokeAIAppConfig(InvokeAISettings):
|
||||
attention_slice_size: Literal["auto", "balanced", "max", 1, 2, 3, 4, 5, 6, 7, 8] = Field(default="auto", description='Slice size, valid when attention_type=="sliced"', category="Generation", )
|
||||
force_tiled_decode : bool = Field(default=False, description="Whether to enable tiled VAE decode (reduces memory consumption with some performance penalty)", category="Generation",)
|
||||
force_tiled_decode: bool = Field(default=False, description="Whether to enable tiled VAE decode (reduces memory consumption with some performance penalty)", category="Generation",)
|
||||
png_compress_level : int = Field(default=6, description="The compress_level setting of PIL.Image.save(), used for PNG encoding. All settings are lossless. 0 = fastest, largest filesize, 9 = slowest, smallest filesize", category="Generation", )
|
||||
|
||||
# QUEUE
|
||||
max_queue_size : int = Field(default=10000, gt=0, description="Maximum number of items in the session queue", category="Queue", )
|
||||
|
@ -2,7 +2,7 @@
|
||||
|
||||
import copy
|
||||
import itertools
|
||||
from typing import Annotated, Any, Optional, Union, cast, get_args, get_origin, get_type_hints
|
||||
from typing import Annotated, Any, Optional, Union, get_args, get_origin, get_type_hints
|
||||
|
||||
import networkx as nx
|
||||
from pydantic import BaseModel, root_validator, validator
|
||||
@ -170,6 +170,18 @@ class NodeIdMismatchError(ValueError):
|
||||
pass
|
||||
|
||||
|
||||
class InvalidSubGraphError(ValueError):
|
||||
pass
|
||||
|
||||
|
||||
class CyclicalGraphError(ValueError):
|
||||
pass
|
||||
|
||||
|
||||
class UnknownGraphValidationError(ValueError):
|
||||
pass
|
||||
|
||||
|
||||
# TODO: Create and use an Empty output?
|
||||
@invocation_output("graph_output")
|
||||
class GraphInvocationOutput(BaseInvocationOutput):
|
||||
@ -254,59 +266,6 @@ class Graph(BaseModel):
|
||||
default_factory=list,
|
||||
)
|
||||
|
||||
@root_validator
|
||||
def validate_nodes_and_edges(cls, values):
|
||||
"""Validates that all edges match nodes in the graph"""
|
||||
nodes = cast(Optional[dict[str, BaseInvocation]], values.get("nodes"))
|
||||
edges = cast(Optional[list[Edge]], values.get("edges"))
|
||||
|
||||
if nodes is not None:
|
||||
# Validate that all node ids are unique
|
||||
node_ids = [n.id for n in nodes.values()]
|
||||
duplicate_node_ids = set([node_id for node_id in node_ids if node_ids.count(node_id) >= 2])
|
||||
if duplicate_node_ids:
|
||||
raise DuplicateNodeIdError(f"Node ids must be unique, found duplicates {duplicate_node_ids}")
|
||||
|
||||
# Validate that all node ids match the keys in the nodes dict
|
||||
for k, v in nodes.items():
|
||||
if k != v.id:
|
||||
raise NodeIdMismatchError(f"Node ids must match, got {k} and {v.id}")
|
||||
|
||||
if edges is not None and nodes is not None:
|
||||
# Validate that all edges match nodes in the graph
|
||||
node_ids = set([e.source.node_id for e in edges] + [e.destination.node_id for e in edges])
|
||||
missing_node_ids = [node_id for node_id in node_ids if node_id not in nodes]
|
||||
if missing_node_ids:
|
||||
raise NodeNotFoundError(
|
||||
f"All edges must reference nodes in the graph, missing nodes: {missing_node_ids}"
|
||||
)
|
||||
|
||||
# Validate that all edge fields match node fields in the graph
|
||||
for edge in edges:
|
||||
source_node = nodes.get(edge.source.node_id, None)
|
||||
if source_node is None:
|
||||
raise NodeFieldNotFoundError(f"Edge source node {edge.source.node_id} does not exist in the graph")
|
||||
|
||||
destination_node = nodes.get(edge.destination.node_id, None)
|
||||
if destination_node is None:
|
||||
raise NodeFieldNotFoundError(
|
||||
f"Edge destination node {edge.destination.node_id} does not exist in the graph"
|
||||
)
|
||||
|
||||
# output fields are not on the node object directly, they are on the output type
|
||||
if edge.source.field not in source_node.get_output_type().__fields__:
|
||||
raise NodeFieldNotFoundError(
|
||||
f"Edge source field {edge.source.field} does not exist in node {edge.source.node_id}"
|
||||
)
|
||||
|
||||
# input fields are on the node
|
||||
if edge.destination.field not in destination_node.__fields__:
|
||||
raise NodeFieldNotFoundError(
|
||||
f"Edge destination field {edge.destination.field} does not exist in node {edge.destination.node_id}"
|
||||
)
|
||||
|
||||
return values
|
||||
|
||||
def add_node(self, node: BaseInvocation) -> None:
|
||||
"""Adds a node to a graph
|
||||
|
||||
@ -377,53 +336,108 @@ class Graph(BaseModel):
|
||||
except KeyError:
|
||||
pass
|
||||
|
||||
def is_valid(self) -> bool:
|
||||
"""Validates the graph."""
|
||||
def validate_self(self) -> None:
|
||||
"""
|
||||
Validates the graph.
|
||||
|
||||
Raises an exception if the graph is invalid:
|
||||
- `DuplicateNodeIdError`
|
||||
- `NodeIdMismatchError`
|
||||
- `InvalidSubGraphError`
|
||||
- `NodeNotFoundError`
|
||||
- `NodeFieldNotFoundError`
|
||||
- `CyclicalGraphError`
|
||||
- `InvalidEdgeError`
|
||||
"""
|
||||
|
||||
# Validate that all node ids are unique
|
||||
node_ids = [n.id for n in self.nodes.values()]
|
||||
duplicate_node_ids = set([node_id for node_id in node_ids if node_ids.count(node_id) >= 2])
|
||||
if duplicate_node_ids:
|
||||
raise DuplicateNodeIdError(f"Node ids must be unique, found duplicates {duplicate_node_ids}")
|
||||
|
||||
# Validate that all node ids match the keys in the nodes dict
|
||||
for k, v in self.nodes.items():
|
||||
if k != v.id:
|
||||
raise NodeIdMismatchError(f"Node ids must match, got {k} and {v.id}")
|
||||
|
||||
# Validate all subgraphs
|
||||
for gn in (n for n in self.nodes.values() if isinstance(n, GraphInvocation)):
|
||||
if not gn.graph.is_valid():
|
||||
return False
|
||||
try:
|
||||
gn.graph.validate_self()
|
||||
except Exception as e:
|
||||
raise InvalidSubGraphError(f"Subgraph {gn.id} is invalid") from e
|
||||
|
||||
# Validate all edges reference nodes in the graph
|
||||
node_ids = set([e.source.node_id for e in self.edges] + [e.destination.node_id for e in self.edges])
|
||||
if not all((self.has_node(node_id) for node_id in node_ids)):
|
||||
return False
|
||||
# Validate that all edges match nodes and fields in the graph
|
||||
for edge in self.edges:
|
||||
source_node = self.nodes.get(edge.source.node_id, None)
|
||||
if source_node is None:
|
||||
raise NodeNotFoundError(f"Edge source node {edge.source.node_id} does not exist in the graph")
|
||||
|
||||
destination_node = self.nodes.get(edge.destination.node_id, None)
|
||||
if destination_node is None:
|
||||
raise NodeNotFoundError(f"Edge destination node {edge.destination.node_id} does not exist in the graph")
|
||||
|
||||
# output fields are not on the node object directly, they are on the output type
|
||||
if edge.source.field not in source_node.get_output_type().__fields__:
|
||||
raise NodeFieldNotFoundError(
|
||||
f"Edge source field {edge.source.field} does not exist in node {edge.source.node_id}"
|
||||
)
|
||||
|
||||
# input fields are on the node
|
||||
if edge.destination.field not in destination_node.__fields__:
|
||||
raise NodeFieldNotFoundError(
|
||||
f"Edge destination field {edge.destination.field} does not exist in node {edge.destination.node_id}"
|
||||
)
|
||||
|
||||
# Validate there are no cycles
|
||||
g = self.nx_graph_flat()
|
||||
if not nx.is_directed_acyclic_graph(g):
|
||||
return False
|
||||
raise CyclicalGraphError("Graph contains cycles")
|
||||
|
||||
# Validate all edge connections are valid
|
||||
if not all(
|
||||
(
|
||||
are_connections_compatible(
|
||||
self.get_node(e.source.node_id),
|
||||
e.source.field,
|
||||
self.get_node(e.destination.node_id),
|
||||
e.destination.field,
|
||||
for e in self.edges:
|
||||
if not are_connections_compatible(
|
||||
self.get_node(e.source.node_id),
|
||||
e.source.field,
|
||||
self.get_node(e.destination.node_id),
|
||||
e.destination.field,
|
||||
):
|
||||
raise InvalidEdgeError(
|
||||
f"Invalid edge from {e.source.node_id}.{e.source.field} to {e.destination.node_id}.{e.destination.field}"
|
||||
)
|
||||
for e in self.edges
|
||||
)
|
||||
|
||||
# Validate all iterators & collectors
|
||||
# TODO: may need to validate all iterators & collectors in subgraphs so edge connections in parent graphs will be available
|
||||
for n in self.nodes.values():
|
||||
if isinstance(n, IterateInvocation) and not self._is_iterator_connection_valid(n.id):
|
||||
raise InvalidEdgeError(f"Invalid iterator node {n.id}")
|
||||
if isinstance(n, CollectInvocation) and not self._is_collector_connection_valid(n.id):
|
||||
raise InvalidEdgeError(f"Invalid collector node {n.id}")
|
||||
|
||||
return None
|
||||
|
||||
def is_valid(self) -> bool:
|
||||
"""
|
||||
Checks if the graph is valid.
|
||||
|
||||
Raises `UnknownGraphValidationError` if there is a problem validating the graph (not a validation error).
|
||||
"""
|
||||
try:
|
||||
self.validate_self()
|
||||
return True
|
||||
except (
|
||||
DuplicateNodeIdError,
|
||||
NodeIdMismatchError,
|
||||
InvalidSubGraphError,
|
||||
NodeNotFoundError,
|
||||
NodeFieldNotFoundError,
|
||||
CyclicalGraphError,
|
||||
InvalidEdgeError,
|
||||
):
|
||||
return False
|
||||
|
||||
# Validate all iterators
|
||||
# TODO: may need to validate all iterators in subgraphs so edge connections in parent graphs will be available
|
||||
if not all(
|
||||
(self._is_iterator_connection_valid(n.id) for n in self.nodes.values() if isinstance(n, IterateInvocation))
|
||||
):
|
||||
return False
|
||||
|
||||
# Validate all collectors
|
||||
# TODO: may need to validate all collectors in subgraphs so edge connections in parent graphs will be available
|
||||
if not all(
|
||||
(self._is_collector_connection_valid(n.id) for n in self.nodes.values() if isinstance(n, CollectInvocation))
|
||||
):
|
||||
return False
|
||||
|
||||
return True
|
||||
except Exception as e:
|
||||
raise UnknownGraphValidationError(f"Problem validating graph {e}") from e
|
||||
|
||||
def _validate_edge(self, edge: Edge):
|
||||
"""Validates that a new edge doesn't create a cycle in the graph"""
|
||||
@ -804,6 +818,12 @@ class GraphExecutionState(BaseModel):
|
||||
default_factory=dict,
|
||||
)
|
||||
|
||||
@validator("graph")
|
||||
def graph_is_valid(cls, v: Graph):
|
||||
"""Validates that the graph is valid"""
|
||||
v.validate_self()
|
||||
return v
|
||||
|
||||
class Config:
|
||||
schema_extra = {
|
||||
"required": [
|
||||
|
@ -9,6 +9,7 @@ from PIL import Image, PngImagePlugin
|
||||
from PIL.Image import Image as PILImageType
|
||||
from send2trash import send2trash
|
||||
|
||||
from invokeai.app.services.config.invokeai_config import InvokeAIAppConfig
|
||||
from invokeai.app.util.thumbnails import get_thumbnail_name, make_thumbnail
|
||||
|
||||
|
||||
@ -79,6 +80,7 @@ class DiskImageFileStorage(ImageFileStorageBase):
|
||||
__cache_ids: Queue # TODO: this is an incredibly naive cache
|
||||
__cache: Dict[Path, PILImageType]
|
||||
__max_cache_size: int
|
||||
__compress_level: int
|
||||
|
||||
def __init__(self, output_folder: Union[str, Path]):
|
||||
self.__cache = dict()
|
||||
@ -87,7 +89,7 @@ class DiskImageFileStorage(ImageFileStorageBase):
|
||||
|
||||
self.__output_folder: Path = output_folder if isinstance(output_folder, Path) else Path(output_folder)
|
||||
self.__thumbnails_folder = self.__output_folder / "thumbnails"
|
||||
|
||||
self.__compress_level = InvokeAIAppConfig.get_config().png_compress_level
|
||||
# Validate required output folders at launch
|
||||
self.__validate_storage_folders()
|
||||
|
||||
@ -134,7 +136,7 @@ class DiskImageFileStorage(ImageFileStorageBase):
|
||||
if original_workflow is not None:
|
||||
pnginfo.add_text("invokeai_workflow", original_workflow)
|
||||
|
||||
image.save(image_path, "PNG", pnginfo=pnginfo)
|
||||
image.save(image_path, "PNG", pnginfo=pnginfo, compress_level=self.__compress_level)
|
||||
|
||||
thumbnail_name = get_thumbnail_name(image_name)
|
||||
thumbnail_path = self.get_path(thumbnail_name, thumbnail=True)
|
||||
|
@ -584,7 +584,7 @@ class SqliteImageRecordStorage(ImageRecordStorageBase):
|
||||
FROM images
|
||||
JOIN board_images ON images.image_name = board_images.image_name
|
||||
WHERE board_images.board_id = ?
|
||||
ORDER BY images.created_at DESC
|
||||
ORDER BY images.starred DESC, images.created_at DESC
|
||||
LIMIT 1;
|
||||
""",
|
||||
(board_id,),
|
||||
|
@ -1,3 +1,4 @@
|
||||
import traceback
|
||||
from threading import BoundedSemaphore
|
||||
from threading import Event as ThreadEvent
|
||||
from threading import Thread
|
||||
@ -123,6 +124,10 @@ class DefaultSessionProcessor(SessionProcessorBase):
|
||||
continue
|
||||
except Exception as e:
|
||||
self.__invoker.services.logger.error(f"Error in session processor: {e}")
|
||||
if queue_item is not None:
|
||||
self.__invoker.services.session_queue.cancel_queue_item(
|
||||
queue_item.item_id, error=traceback.format_exc()
|
||||
)
|
||||
poll_now_event.wait(POLLING_INTERVAL)
|
||||
continue
|
||||
except Exception as e:
|
||||
|
@ -80,7 +80,7 @@ class SessionQueueBase(ABC):
|
||||
pass
|
||||
|
||||
@abstractmethod
|
||||
def cancel_queue_item(self, item_id: int) -> SessionQueueItem:
|
||||
def cancel_queue_item(self, item_id: int, error: Optional[str] = None) -> SessionQueueItem:
|
||||
"""Cancels a session queue item"""
|
||||
pass
|
||||
|
||||
|
@ -123,6 +123,11 @@ class Batch(BaseModel):
|
||||
raise NodeNotFoundError(f"Field {batch_data.field_name} not found in node {batch_data.node_path}")
|
||||
return values
|
||||
|
||||
@validator("graph")
|
||||
def validate_graph(cls, v: Graph):
|
||||
v.validate_self()
|
||||
return v
|
||||
|
||||
class Config:
|
||||
schema_extra = {
|
||||
"required": [
|
||||
|
@ -555,10 +555,11 @@ class SqliteSessionQueue(SessionQueueBase):
|
||||
self.__lock.release()
|
||||
return PruneResult(deleted=count)
|
||||
|
||||
def cancel_queue_item(self, item_id: int) -> SessionQueueItem:
|
||||
def cancel_queue_item(self, item_id: int, error: Optional[str] = None) -> SessionQueueItem:
|
||||
queue_item = self.get_queue_item(item_id)
|
||||
if queue_item.status not in ["canceled", "failed", "completed"]:
|
||||
queue_item = self._set_queue_item_status(item_id=item_id, status="canceled")
|
||||
status = "failed" if error is not None else "canceled"
|
||||
queue_item = self._set_queue_item_status(item_id=item_id, status=status, error=error)
|
||||
self.__invoker.services.queue.cancel(queue_item.session_id)
|
||||
self.__invoker.services.events.emit_session_canceled(
|
||||
queue_item_id=queue_item.item_id,
|
||||
|
@ -265,22 +265,41 @@ def np_img_resize(np_img: np.ndarray, resize_mode: str, h: int, w: int, device:
|
||||
|
||||
|
||||
def prepare_control_image(
|
||||
# image used to be Union[PIL.Image.Image, List[PIL.Image.Image], torch.Tensor, List[torch.Tensor]]
|
||||
# but now should be able to assume that image is a single PIL.Image, which simplifies things
|
||||
image: Image,
|
||||
# FIXME: need to fix hardwiring of width and height, change to basing on latents dimensions?
|
||||
# latents_to_match_resolution, # TorchTensor of shape (batch_size, 3, height, width)
|
||||
width=512, # should be 8 * latent.shape[3]
|
||||
height=512, # should be 8 * latent height[2]
|
||||
# batch_size=1, # currently no batching
|
||||
# num_images_per_prompt=1, # currently only single image
|
||||
width: int,
|
||||
height: int,
|
||||
num_channels: int = 3,
|
||||
device="cuda",
|
||||
dtype=torch.float16,
|
||||
do_classifier_free_guidance=True,
|
||||
control_mode="balanced",
|
||||
resize_mode="just_resize_simple",
|
||||
):
|
||||
# FIXME: implement "crop_resize_simple" and "fill_resize_simple", or pull them out
|
||||
"""Pre-process images for ControlNets or T2I-Adapters.
|
||||
|
||||
Args:
|
||||
image (Image): The PIL image to pre-process.
|
||||
width (int): The target width in pixels.
|
||||
height (int): The target height in pixels.
|
||||
num_channels (int, optional): The target number of image channels. This is achieved by converting the input
|
||||
image to RGB, then naively taking the first `num_channels` channels. The primary use case is converting a
|
||||
RGB image to a single-channel grayscale image. Raises if `num_channels` cannot be achieved. Defaults to 3.
|
||||
device (str, optional): The target device for the output image. Defaults to "cuda".
|
||||
dtype (_type_, optional): The dtype for the output image. Defaults to torch.float16.
|
||||
do_classifier_free_guidance (bool, optional): If True, repeat the output image along the batch dimension.
|
||||
Defaults to True.
|
||||
control_mode (str, optional): Defaults to "balanced".
|
||||
resize_mode (str, optional): Defaults to "just_resize_simple".
|
||||
|
||||
Raises:
|
||||
NotImplementedError: If resize_mode == "crop_resize_simple".
|
||||
NotImplementedError: If resize_mode == "fill_resize_simple".
|
||||
ValueError: If `resize_mode` is not recognized.
|
||||
ValueError: If `num_channels` is out of range.
|
||||
|
||||
Returns:
|
||||
torch.Tensor: The pre-processed input tensor.
|
||||
"""
|
||||
if (
|
||||
resize_mode == "just_resize_simple"
|
||||
or resize_mode == "crop_resize_simple"
|
||||
@ -289,10 +308,10 @@ def prepare_control_image(
|
||||
image = image.convert("RGB")
|
||||
if resize_mode == "just_resize_simple":
|
||||
image = image.resize((width, height), resample=PIL_INTERPOLATION["lanczos"])
|
||||
elif resize_mode == "crop_resize_simple": # not yet implemented
|
||||
pass
|
||||
elif resize_mode == "fill_resize_simple": # not yet implemented
|
||||
pass
|
||||
elif resize_mode == "crop_resize_simple":
|
||||
raise NotImplementedError(f"prepare_control_image is not implemented for resize_mode='{resize_mode}'.")
|
||||
elif resize_mode == "fill_resize_simple":
|
||||
raise NotImplementedError(f"prepare_control_image is not implemented for resize_mode='{resize_mode}'.")
|
||||
nimage = np.array(image)
|
||||
nimage = nimage[None, :]
|
||||
nimage = np.concatenate([nimage], axis=0)
|
||||
@ -313,9 +332,11 @@ def prepare_control_image(
|
||||
device=device,
|
||||
)
|
||||
else:
|
||||
pass
|
||||
print("ERROR: invalid resize_mode ==> ", resize_mode)
|
||||
exit(1)
|
||||
raise ValueError(f"Unsupported resize_mode: '{resize_mode}'.")
|
||||
|
||||
if timage.shape[1] < num_channels or num_channels <= 0:
|
||||
raise ValueError(f"Cannot achieve the target of num_channels={num_channels}.")
|
||||
timage = timage[:, :num_channels, :, :]
|
||||
|
||||
timage = timage.to(device=device, dtype=dtype)
|
||||
cfg_injection = control_mode == "more_control" or control_mode == "unbalanced"
|
||||
|
@ -218,6 +218,20 @@ class IPAdapterPlus(IPAdapter):
|
||||
return image_prompt_embeds, uncond_image_prompt_embeds
|
||||
|
||||
|
||||
class IPAdapterPlusXL(IPAdapterPlus):
|
||||
"""IP-Adapter Plus for SDXL."""
|
||||
|
||||
def _init_image_proj_model(self, state_dict):
|
||||
return Resampler.from_state_dict(
|
||||
state_dict=state_dict,
|
||||
depth=4,
|
||||
dim_head=64,
|
||||
heads=20,
|
||||
num_queries=self._num_tokens,
|
||||
ff_mult=4,
|
||||
).to(self.device, dtype=self.dtype)
|
||||
|
||||
|
||||
def build_ip_adapter(
|
||||
ip_adapter_ckpt_path: str, device: torch.device, dtype: torch.dtype = torch.float16
|
||||
) -> Union[IPAdapter, IPAdapterPlus]:
|
||||
@ -228,6 +242,14 @@ def build_ip_adapter(
|
||||
is_plus = "proj.weight" not in state_dict["image_proj"]
|
||||
|
||||
if is_plus:
|
||||
return IPAdapterPlus(state_dict, device=device, dtype=dtype)
|
||||
cross_attention_dim = state_dict["ip_adapter"]["1.to_k_ip.weight"].shape[-1]
|
||||
if cross_attention_dim == 768:
|
||||
# SD1 IP-Adapter Plus
|
||||
return IPAdapterPlus(state_dict, device=device, dtype=dtype)
|
||||
elif cross_attention_dim == 2048:
|
||||
# SDXL IP-Adapter Plus
|
||||
return IPAdapterPlusXL(state_dict, device=device, dtype=dtype)
|
||||
else:
|
||||
raise Exception(f"Unsupported IP-Adapter Plus cross-attention dimension: {cross_attention_dim}.")
|
||||
else:
|
||||
return IPAdapter(state_dict, device=device, dtype=dtype)
|
||||
|
@ -57,6 +57,7 @@ class ModelProbe(object):
|
||||
"AutoencoderTiny": ModelType.Vae,
|
||||
"ControlNetModel": ModelType.ControlNet,
|
||||
"CLIPVisionModelWithProjection": ModelType.CLIPVision,
|
||||
"T2IAdapter": ModelType.T2IAdapter,
|
||||
}
|
||||
|
||||
@classmethod
|
||||
@ -408,6 +409,11 @@ class CLIPVisionCheckpointProbe(CheckpointProbeBase):
|
||||
raise NotImplementedError()
|
||||
|
||||
|
||||
class T2IAdapterCheckpointProbe(CheckpointProbeBase):
|
||||
def get_base_type(self) -> BaseModelType:
|
||||
raise NotImplementedError()
|
||||
|
||||
|
||||
########################################################
|
||||
# classes for probing folders
|
||||
#######################################################
|
||||
@ -595,6 +601,26 @@ class CLIPVisionFolderProbe(FolderProbeBase):
|
||||
return BaseModelType.Any
|
||||
|
||||
|
||||
class T2IAdapterFolderProbe(FolderProbeBase):
|
||||
def get_base_type(self) -> BaseModelType:
|
||||
config_file = self.folder_path / "config.json"
|
||||
if not config_file.exists():
|
||||
raise InvalidModelException(f"Cannot determine base type for {self.folder_path}")
|
||||
with open(config_file, "r") as file:
|
||||
config = json.load(file)
|
||||
|
||||
adapter_type = config.get("adapter_type", None)
|
||||
if adapter_type == "full_adapter_xl":
|
||||
return BaseModelType.StableDiffusionXL
|
||||
elif adapter_type == "full_adapter" or "light_adapter":
|
||||
# I haven't seen any T2I adapter models for SD2, so assume that this is an SD1 adapter.
|
||||
return BaseModelType.StableDiffusion1
|
||||
else:
|
||||
raise InvalidModelException(
|
||||
f"Unable to determine base model for '{self.folder_path}' (adapter_type = {adapter_type})."
|
||||
)
|
||||
|
||||
|
||||
############## register probe classes ######
|
||||
ModelProbe.register_probe("diffusers", ModelType.Main, PipelineFolderProbe)
|
||||
ModelProbe.register_probe("diffusers", ModelType.Vae, VaeFolderProbe)
|
||||
@ -603,6 +629,7 @@ ModelProbe.register_probe("diffusers", ModelType.TextualInversion, TextualInvers
|
||||
ModelProbe.register_probe("diffusers", ModelType.ControlNet, ControlNetFolderProbe)
|
||||
ModelProbe.register_probe("diffusers", ModelType.IPAdapter, IPAdapterFolderProbe)
|
||||
ModelProbe.register_probe("diffusers", ModelType.CLIPVision, CLIPVisionFolderProbe)
|
||||
ModelProbe.register_probe("diffusers", ModelType.T2IAdapter, T2IAdapterFolderProbe)
|
||||
|
||||
ModelProbe.register_probe("checkpoint", ModelType.Main, PipelineCheckpointProbe)
|
||||
ModelProbe.register_probe("checkpoint", ModelType.Vae, VaeCheckpointProbe)
|
||||
@ -611,5 +638,6 @@ ModelProbe.register_probe("checkpoint", ModelType.TextualInversion, TextualInver
|
||||
ModelProbe.register_probe("checkpoint", ModelType.ControlNet, ControlNetCheckpointProbe)
|
||||
ModelProbe.register_probe("checkpoint", ModelType.IPAdapter, IPAdapterCheckpointProbe)
|
||||
ModelProbe.register_probe("checkpoint", ModelType.CLIPVision, CLIPVisionCheckpointProbe)
|
||||
ModelProbe.register_probe("checkpoint", ModelType.T2IAdapter, T2IAdapterCheckpointProbe)
|
||||
|
||||
ModelProbe.register_probe("onnx", ModelType.ONNX, ONNXFolderProbe)
|
||||
|
@ -25,6 +25,7 @@ from .lora import LoRAModel
|
||||
from .sdxl import StableDiffusionXLModel
|
||||
from .stable_diffusion import StableDiffusion1Model, StableDiffusion2Model
|
||||
from .stable_diffusion_onnx import ONNXStableDiffusion1Model, ONNXStableDiffusion2Model
|
||||
from .t2i_adapter import T2IAdapterModel
|
||||
from .textual_inversion import TextualInversionModel
|
||||
from .vae import VaeModel
|
||||
|
||||
@ -38,6 +39,7 @@ MODEL_CLASSES = {
|
||||
ModelType.TextualInversion: TextualInversionModel,
|
||||
ModelType.IPAdapter: IPAdapterModel,
|
||||
ModelType.CLIPVision: CLIPVisionModel,
|
||||
ModelType.T2IAdapter: T2IAdapterModel,
|
||||
},
|
||||
BaseModelType.StableDiffusion2: {
|
||||
ModelType.ONNX: ONNXStableDiffusion2Model,
|
||||
@ -48,6 +50,7 @@ MODEL_CLASSES = {
|
||||
ModelType.TextualInversion: TextualInversionModel,
|
||||
ModelType.IPAdapter: IPAdapterModel,
|
||||
ModelType.CLIPVision: CLIPVisionModel,
|
||||
ModelType.T2IAdapter: T2IAdapterModel,
|
||||
},
|
||||
BaseModelType.StableDiffusionXL: {
|
||||
ModelType.Main: StableDiffusionXLModel,
|
||||
@ -59,6 +62,7 @@ MODEL_CLASSES = {
|
||||
ModelType.ONNX: ONNXStableDiffusion2Model,
|
||||
ModelType.IPAdapter: IPAdapterModel,
|
||||
ModelType.CLIPVision: CLIPVisionModel,
|
||||
ModelType.T2IAdapter: T2IAdapterModel,
|
||||
},
|
||||
BaseModelType.StableDiffusionXLRefiner: {
|
||||
ModelType.Main: StableDiffusionXLModel,
|
||||
@ -70,6 +74,7 @@ MODEL_CLASSES = {
|
||||
ModelType.ONNX: ONNXStableDiffusion2Model,
|
||||
ModelType.IPAdapter: IPAdapterModel,
|
||||
ModelType.CLIPVision: CLIPVisionModel,
|
||||
ModelType.T2IAdapter: T2IAdapterModel,
|
||||
},
|
||||
BaseModelType.Any: {
|
||||
ModelType.CLIPVision: CLIPVisionModel,
|
||||
@ -81,6 +86,7 @@ MODEL_CLASSES = {
|
||||
ModelType.ControlNet: ControlNetModel,
|
||||
ModelType.TextualInversion: TextualInversionModel,
|
||||
ModelType.IPAdapter: IPAdapterModel,
|
||||
ModelType.T2IAdapter: T2IAdapterModel,
|
||||
},
|
||||
# BaseModelType.Kandinsky2_1: {
|
||||
# ModelType.Main: Kandinsky2_1Model,
|
||||
|
@ -53,6 +53,7 @@ class ModelType(str, Enum):
|
||||
TextualInversion = "embedding"
|
||||
IPAdapter = "ip_adapter"
|
||||
CLIPVision = "clip_vision"
|
||||
T2IAdapter = "t2i_adapter"
|
||||
|
||||
|
||||
class SubModelType(str, Enum):
|
||||
|
102
invokeai/backend/model_management/models/t2i_adapter.py
Normal file
102
invokeai/backend/model_management/models/t2i_adapter.py
Normal file
@ -0,0 +1,102 @@
|
||||
import os
|
||||
from enum import Enum
|
||||
from typing import Literal, Optional
|
||||
|
||||
import torch
|
||||
from diffusers import T2IAdapter
|
||||
|
||||
from invokeai.backend.model_management.models.base import (
|
||||
BaseModelType,
|
||||
EmptyConfigLoader,
|
||||
InvalidModelException,
|
||||
ModelBase,
|
||||
ModelConfigBase,
|
||||
ModelNotFoundException,
|
||||
ModelType,
|
||||
SubModelType,
|
||||
calc_model_size_by_data,
|
||||
calc_model_size_by_fs,
|
||||
classproperty,
|
||||
)
|
||||
|
||||
|
||||
class T2IAdapterModelFormat(str, Enum):
|
||||
Diffusers = "diffusers"
|
||||
|
||||
|
||||
class T2IAdapterModel(ModelBase):
|
||||
class DiffusersConfig(ModelConfigBase):
|
||||
model_format: Literal[T2IAdapterModelFormat.Diffusers]
|
||||
|
||||
def __init__(self, model_path: str, base_model: BaseModelType, model_type: ModelType):
|
||||
assert model_type == ModelType.T2IAdapter
|
||||
super().__init__(model_path, base_model, model_type)
|
||||
|
||||
config = EmptyConfigLoader.load_config(self.model_path, config_name="config.json")
|
||||
|
||||
model_class_name = config.get("_class_name", None)
|
||||
if model_class_name not in {"T2IAdapter"}:
|
||||
raise InvalidModelException(f"Invalid T2I-Adapter model. Unknown _class_name: '{model_class_name}'.")
|
||||
|
||||
self.model_class = self._hf_definition_to_type(["diffusers", model_class_name])
|
||||
self.model_size = calc_model_size_by_fs(self.model_path)
|
||||
|
||||
def get_size(self, child_type: Optional[SubModelType] = None):
|
||||
if child_type is not None:
|
||||
raise ValueError(f"T2I-Adapters do not have child models. Invalid child type: '{child_type}'.")
|
||||
return self.model_size
|
||||
|
||||
def get_model(
|
||||
self,
|
||||
torch_dtype: Optional[torch.dtype],
|
||||
child_type: Optional[SubModelType] = None,
|
||||
) -> T2IAdapter:
|
||||
if child_type is not None:
|
||||
raise ValueError(f"T2I-Adapters do not have child models. Invalid child type: '{child_type}'.")
|
||||
|
||||
model = None
|
||||
for variant in ["fp16", None]:
|
||||
try:
|
||||
model = self.model_class.from_pretrained(
|
||||
self.model_path,
|
||||
torch_dtype=torch_dtype,
|
||||
variant=variant,
|
||||
)
|
||||
break
|
||||
except Exception:
|
||||
pass
|
||||
if not model:
|
||||
raise ModelNotFoundException()
|
||||
|
||||
# Calculate a more accurate size after loading the model into memory.
|
||||
self.model_size = calc_model_size_by_data(model)
|
||||
return model
|
||||
|
||||
@classproperty
|
||||
def save_to_config(cls) -> bool:
|
||||
return False
|
||||
|
||||
@classmethod
|
||||
def detect_format(cls, path: str):
|
||||
if not os.path.exists(path):
|
||||
raise ModelNotFoundException(f"Model not found at '{path}'.")
|
||||
|
||||
if os.path.isdir(path):
|
||||
if os.path.exists(os.path.join(path, "config.json")):
|
||||
return T2IAdapterModelFormat.Diffusers
|
||||
|
||||
raise InvalidModelException(f"Unsupported T2I-Adapter format: '{path}'.")
|
||||
|
||||
@classmethod
|
||||
def convert_if_required(
|
||||
cls,
|
||||
model_path: str,
|
||||
output_path: str,
|
||||
config: ModelConfigBase,
|
||||
base_model: BaseModelType,
|
||||
) -> str:
|
||||
format = cls.detect_format(model_path)
|
||||
if format == T2IAdapterModelFormat.Diffusers:
|
||||
return model_path
|
||||
else:
|
||||
raise ValueError(f"Unsupported format: '{format}'.")
|
@ -173,6 +173,16 @@ class IPAdapterData:
|
||||
end_step_percent: float = Field(default=1.0)
|
||||
|
||||
|
||||
@dataclass
|
||||
class T2IAdapterData:
|
||||
"""A structure containing the information required to apply conditioning from a single T2I-Adapter model."""
|
||||
|
||||
adapter_state: dict[torch.Tensor] = Field()
|
||||
weight: Union[float, list[float]] = Field(default=1.0)
|
||||
begin_step_percent: float = Field(default=0.0)
|
||||
end_step_percent: float = Field(default=1.0)
|
||||
|
||||
|
||||
@dataclass
|
||||
class InvokeAIStableDiffusionPipelineOutput(StableDiffusionPipelineOutput):
|
||||
r"""
|
||||
@ -327,6 +337,7 @@ class StableDiffusionGeneratorPipeline(StableDiffusionPipeline):
|
||||
callback: Callable[[PipelineIntermediateState], None] = None,
|
||||
control_data: List[ControlNetData] = None,
|
||||
ip_adapter_data: Optional[IPAdapterData] = None,
|
||||
t2i_adapter_data: Optional[list[T2IAdapterData]] = None,
|
||||
mask: Optional[torch.Tensor] = None,
|
||||
masked_latents: Optional[torch.Tensor] = None,
|
||||
seed: Optional[int] = None,
|
||||
@ -379,6 +390,7 @@ class StableDiffusionGeneratorPipeline(StableDiffusionPipeline):
|
||||
additional_guidance=additional_guidance,
|
||||
control_data=control_data,
|
||||
ip_adapter_data=ip_adapter_data,
|
||||
t2i_adapter_data=t2i_adapter_data,
|
||||
callback=callback,
|
||||
)
|
||||
finally:
|
||||
@ -399,6 +411,7 @@ class StableDiffusionGeneratorPipeline(StableDiffusionPipeline):
|
||||
additional_guidance: List[Callable] = None,
|
||||
control_data: List[ControlNetData] = None,
|
||||
ip_adapter_data: Optional[IPAdapterData] = None,
|
||||
t2i_adapter_data: Optional[list[T2IAdapterData]] = None,
|
||||
callback: Callable[[PipelineIntermediateState], None] = None,
|
||||
):
|
||||
self._adjust_memory_efficient_attention(latents)
|
||||
@ -454,6 +467,7 @@ class StableDiffusionGeneratorPipeline(StableDiffusionPipeline):
|
||||
additional_guidance=additional_guidance,
|
||||
control_data=control_data,
|
||||
ip_adapter_data=ip_adapter_data,
|
||||
t2i_adapter_data=t2i_adapter_data,
|
||||
)
|
||||
latents = step_output.prev_sample
|
||||
|
||||
@ -500,6 +514,7 @@ class StableDiffusionGeneratorPipeline(StableDiffusionPipeline):
|
||||
additional_guidance: List[Callable] = None,
|
||||
control_data: List[ControlNetData] = None,
|
||||
ip_adapter_data: Optional[IPAdapterData] = None,
|
||||
t2i_adapter_data: Optional[list[T2IAdapterData]] = None,
|
||||
):
|
||||
# invokeai_diffuser has batched timesteps, but diffusers schedulers expect a single value
|
||||
timestep = t[0]
|
||||
@ -527,11 +542,15 @@ class StableDiffusionGeneratorPipeline(StableDiffusionPipeline):
|
||||
# otherwise, set IP-Adapter scale to 0, so it has no effect
|
||||
ip_adapter_data.ip_adapter_model.set_scale(0.0)
|
||||
|
||||
# handle ControlNet(s)
|
||||
# default is no controlnet, so set controlnet processing output to None
|
||||
controlnet_down_block_samples, controlnet_mid_block_sample = None, None
|
||||
if control_data is not None:
|
||||
controlnet_down_block_samples, controlnet_mid_block_sample = self.invokeai_diffuser.do_controlnet_step(
|
||||
# Handle ControlNet(s) and T2I-Adapter(s)
|
||||
down_block_additional_residuals = None
|
||||
mid_block_additional_residual = None
|
||||
if control_data is not None and t2i_adapter_data is not None:
|
||||
# TODO(ryand): This is a limitation of the UNet2DConditionModel API, not a fundamental incompatibility
|
||||
# between ControlNets and T2I-Adapters. We will try to fix this upstream in diffusers.
|
||||
raise Exception("ControlNet(s) and T2I-Adapter(s) cannot be used simultaneously (yet).")
|
||||
elif control_data is not None:
|
||||
down_block_additional_residuals, mid_block_additional_residual = self.invokeai_diffuser.do_controlnet_step(
|
||||
control_data=control_data,
|
||||
sample=latent_model_input,
|
||||
timestep=timestep,
|
||||
@ -539,6 +558,32 @@ class StableDiffusionGeneratorPipeline(StableDiffusionPipeline):
|
||||
total_step_count=total_step_count,
|
||||
conditioning_data=conditioning_data,
|
||||
)
|
||||
elif t2i_adapter_data is not None:
|
||||
accum_adapter_state = None
|
||||
for single_t2i_adapter_data in t2i_adapter_data:
|
||||
# Determine the T2I-Adapter weights for the current denoising step.
|
||||
first_t2i_adapter_step = math.floor(single_t2i_adapter_data.begin_step_percent * total_step_count)
|
||||
last_t2i_adapter_step = math.ceil(single_t2i_adapter_data.end_step_percent * total_step_count)
|
||||
t2i_adapter_weight = (
|
||||
single_t2i_adapter_data.weight[step_index]
|
||||
if isinstance(single_t2i_adapter_data.weight, list)
|
||||
else single_t2i_adapter_data.weight
|
||||
)
|
||||
if step_index < first_t2i_adapter_step or step_index > last_t2i_adapter_step:
|
||||
# If the current step is outside of the T2I-Adapter's begin/end step range, then set its weight to 0
|
||||
# so it has no effect.
|
||||
t2i_adapter_weight = 0.0
|
||||
|
||||
# Apply the t2i_adapter_weight, and accumulate.
|
||||
if accum_adapter_state is None:
|
||||
# Handle the first T2I-Adapter.
|
||||
accum_adapter_state = [val * t2i_adapter_weight for val in single_t2i_adapter_data.adapter_state]
|
||||
else:
|
||||
# Add to the previous adapter states.
|
||||
for idx, value in enumerate(single_t2i_adapter_data.adapter_state):
|
||||
accum_adapter_state[idx] += value * t2i_adapter_weight
|
||||
|
||||
down_block_additional_residuals = accum_adapter_state
|
||||
|
||||
uc_noise_pred, c_noise_pred = self.invokeai_diffuser.do_unet_step(
|
||||
sample=latent_model_input,
|
||||
@ -547,8 +592,8 @@ class StableDiffusionGeneratorPipeline(StableDiffusionPipeline):
|
||||
total_step_count=total_step_count,
|
||||
conditioning_data=conditioning_data,
|
||||
# extra:
|
||||
down_block_additional_residuals=controlnet_down_block_samples, # from controlnet(s)
|
||||
mid_block_additional_residual=controlnet_mid_block_sample, # from controlnet(s)
|
||||
down_block_additional_residuals=down_block_additional_residuals,
|
||||
mid_block_additional_residual=mid_block_additional_residual,
|
||||
)
|
||||
|
||||
guidance_scale = conditioning_data.guidance_scale
|
||||
|
File diff suppressed because one or more lines are too long
File diff suppressed because one or more lines are too long
@ -1,4 +1,4 @@
|
||||
import{w as s,hY as T,v as l,a2 as I,hZ as R,ae as V,h_ as z,h$ as j,i0 as D,i1 as F,i2 as G,i3 as W,i4 as K,aG as Y,i5 as Z,i6 as H}from"./index-94062f76.js";import{M as U}from"./MantineProvider-a057bfc9.js";var P=String.raw,E=P`
|
||||
import{w as s,h$ as T,v as l,a2 as I,i0 as R,ae as V,i1 as z,i2 as j,i3 as D,i4 as F,i5 as G,i6 as W,i7 as K,aG as H,i8 as U,i9 as Y}from"./index-6f7e7659.js";import{M as Z}from"./MantineProvider-2072a471.js";var P=String.raw,E=P`
|
||||
:root,
|
||||
:host {
|
||||
--chakra-vh: 100vh;
|
||||
@ -277,4 +277,4 @@ import{w as s,hY as T,v as l,a2 as I,hZ as R,ae as V,h_ as z,h$ as j,i0 as D,i1
|
||||
}
|
||||
|
||||
${E}
|
||||
`}),g={light:"chakra-ui-light",dark:"chakra-ui-dark"};function Q(e={}){const{preventTransition:o=!0}=e,n={setDataset:r=>{const t=o?n.preventTransition():void 0;document.documentElement.dataset.theme=r,document.documentElement.style.colorScheme=r,t==null||t()},setClassName(r){document.body.classList.add(r?g.dark:g.light),document.body.classList.remove(r?g.light:g.dark)},query(){return window.matchMedia("(prefers-color-scheme: dark)")},getSystemTheme(r){var t;return((t=n.query().matches)!=null?t:r==="dark")?"dark":"light"},addListener(r){const t=n.query(),i=a=>{r(a.matches?"dark":"light")};return typeof t.addListener=="function"?t.addListener(i):t.addEventListener("change",i),()=>{typeof t.removeListener=="function"?t.removeListener(i):t.removeEventListener("change",i)}},preventTransition(){const r=document.createElement("style");return r.appendChild(document.createTextNode("*{-webkit-transition:none!important;-moz-transition:none!important;-o-transition:none!important;-ms-transition:none!important;transition:none!important}")),document.head.appendChild(r),()=>{window.getComputedStyle(document.body),requestAnimationFrame(()=>{requestAnimationFrame(()=>{document.head.removeChild(r)})})}}};return n}var X="chakra-ui-color-mode";function L(e){return{ssr:!1,type:"localStorage",get(o){if(!(globalThis!=null&&globalThis.document))return o;let n;try{n=localStorage.getItem(e)||o}catch{}return n||o},set(o){try{localStorage.setItem(e,o)}catch{}}}}var ee=L(X),M=()=>{};function S(e,o){return e.type==="cookie"&&e.ssr?e.get(o):o}function O(e){const{value:o,children:n,options:{useSystemColorMode:r,initialColorMode:t,disableTransitionOnChange:i}={},colorModeManager:a=ee}=e,d=t==="dark"?"dark":"light",[u,p]=l.useState(()=>S(a,d)),[y,b]=l.useState(()=>S(a)),{getSystemTheme:w,setClassName:k,setDataset:x,addListener:$}=l.useMemo(()=>Q({preventTransition:i}),[i]),v=t==="system"&&!u?y:u,c=l.useCallback(h=>{const f=h==="system"?w():h;p(f),k(f==="dark"),x(f),a.set(f)},[a,w,k,x]);I(()=>{t==="system"&&b(w())},[]),l.useEffect(()=>{const h=a.get();if(h){c(h);return}if(t==="system"){c("system");return}c(d)},[a,d,t,c]);const C=l.useCallback(()=>{c(v==="dark"?"light":"dark")},[v,c]);l.useEffect(()=>{if(r)return $(c)},[r,$,c]);const A=l.useMemo(()=>({colorMode:o??v,toggleColorMode:o?M:C,setColorMode:o?M:c,forced:o!==void 0}),[v,C,c,o]);return s.jsx(R.Provider,{value:A,children:n})}O.displayName="ColorModeProvider";var te=["borders","breakpoints","colors","components","config","direction","fonts","fontSizes","fontWeights","letterSpacings","lineHeights","radii","shadows","sizes","space","styles","transition","zIndices"];function re(e){return V(e)?te.every(o=>Object.prototype.hasOwnProperty.call(e,o)):!1}function m(e){return typeof e=="function"}function oe(...e){return o=>e.reduce((n,r)=>r(n),o)}var ne=e=>function(...n){let r=[...n],t=n[n.length-1];return re(t)&&r.length>1?r=r.slice(0,r.length-1):t=e,oe(...r.map(i=>a=>m(i)?i(a):ae(a,i)))(t)},ie=ne(j);function ae(...e){return z({},...e,_)}function _(e,o,n,r){if((m(e)||m(o))&&Object.prototype.hasOwnProperty.call(r,n))return(...t)=>{const i=m(e)?e(...t):e,a=m(o)?o(...t):o;return z({},i,a,_)}}var q=l.createContext({getDocument(){return document},getWindow(){return window}});q.displayName="EnvironmentContext";function N(e){const{children:o,environment:n,disabled:r}=e,t=l.useRef(null),i=l.useMemo(()=>n||{getDocument:()=>{var d,u;return(u=(d=t.current)==null?void 0:d.ownerDocument)!=null?u:document},getWindow:()=>{var d,u;return(u=(d=t.current)==null?void 0:d.ownerDocument.defaultView)!=null?u:window}},[n]),a=!r||!n;return s.jsxs(q.Provider,{value:i,children:[o,a&&s.jsx("span",{id:"__chakra_env",hidden:!0,ref:t})]})}N.displayName="EnvironmentProvider";var se=e=>{const{children:o,colorModeManager:n,portalZIndex:r,resetScope:t,resetCSS:i=!0,theme:a={},environment:d,cssVarsRoot:u,disableEnvironment:p,disableGlobalStyle:y}=e,b=s.jsx(N,{environment:d,disabled:p,children:o});return s.jsx(D,{theme:a,cssVarsRoot:u,children:s.jsxs(O,{colorModeManager:n,options:a.config,children:[i?s.jsx(J,{scope:t}):s.jsx(B,{}),!y&&s.jsx(F,{}),r?s.jsx(G,{zIndex:r,children:b}):b]})})},le=e=>function({children:n,theme:r=e,toastOptions:t,...i}){return s.jsxs(se,{theme:r,...i,children:[s.jsx(W,{value:t==null?void 0:t.defaultOptions,children:n}),s.jsx(K,{...t})]})},de=le(j);const ue=()=>l.useMemo(()=>({colorScheme:"dark",fontFamily:"'Inter Variable', sans-serif",components:{ScrollArea:{defaultProps:{scrollbarSize:10},styles:{scrollbar:{"&:hover":{backgroundColor:"var(--invokeai-colors-baseAlpha-300)"}},thumb:{backgroundColor:"var(--invokeai-colors-baseAlpha-300)"}}}}}),[]),ce=L("@@invokeai-color-mode");function he({children:e}){const{i18n:o}=Y(),n=o.dir(),r=l.useMemo(()=>ie({...Z,direction:n}),[n]);l.useEffect(()=>{document.body.dir=n},[n]);const t=ue();return s.jsx(U,{theme:t,children:s.jsx(de,{theme:r,colorModeManager:ce,toastOptions:H,children:e})})}const ve=l.memo(he);export{ve as default};
|
||||
`}),g={light:"chakra-ui-light",dark:"chakra-ui-dark"};function Q(e={}){const{preventTransition:o=!0}=e,n={setDataset:r=>{const t=o?n.preventTransition():void 0;document.documentElement.dataset.theme=r,document.documentElement.style.colorScheme=r,t==null||t()},setClassName(r){document.body.classList.add(r?g.dark:g.light),document.body.classList.remove(r?g.light:g.dark)},query(){return window.matchMedia("(prefers-color-scheme: dark)")},getSystemTheme(r){var t;return((t=n.query().matches)!=null?t:r==="dark")?"dark":"light"},addListener(r){const t=n.query(),i=a=>{r(a.matches?"dark":"light")};return typeof t.addListener=="function"?t.addListener(i):t.addEventListener("change",i),()=>{typeof t.removeListener=="function"?t.removeListener(i):t.removeEventListener("change",i)}},preventTransition(){const r=document.createElement("style");return r.appendChild(document.createTextNode("*{-webkit-transition:none!important;-moz-transition:none!important;-o-transition:none!important;-ms-transition:none!important;transition:none!important}")),document.head.appendChild(r),()=>{window.getComputedStyle(document.body),requestAnimationFrame(()=>{requestAnimationFrame(()=>{document.head.removeChild(r)})})}}};return n}var X="chakra-ui-color-mode";function L(e){return{ssr:!1,type:"localStorage",get(o){if(!(globalThis!=null&&globalThis.document))return o;let n;try{n=localStorage.getItem(e)||o}catch{}return n||o},set(o){try{localStorage.setItem(e,o)}catch{}}}}var ee=L(X),M=()=>{};function S(e,o){return e.type==="cookie"&&e.ssr?e.get(o):o}function O(e){const{value:o,children:n,options:{useSystemColorMode:r,initialColorMode:t,disableTransitionOnChange:i}={},colorModeManager:a=ee}=e,d=t==="dark"?"dark":"light",[u,p]=l.useState(()=>S(a,d)),[y,b]=l.useState(()=>S(a)),{getSystemTheme:w,setClassName:k,setDataset:x,addListener:$}=l.useMemo(()=>Q({preventTransition:i}),[i]),v=t==="system"&&!u?y:u,c=l.useCallback(h=>{const f=h==="system"?w():h;p(f),k(f==="dark"),x(f),a.set(f)},[a,w,k,x]);I(()=>{t==="system"&&b(w())},[]),l.useEffect(()=>{const h=a.get();if(h){c(h);return}if(t==="system"){c("system");return}c(d)},[a,d,t,c]);const C=l.useCallback(()=>{c(v==="dark"?"light":"dark")},[v,c]);l.useEffect(()=>{if(r)return $(c)},[r,$,c]);const A=l.useMemo(()=>({colorMode:o??v,toggleColorMode:o?M:C,setColorMode:o?M:c,forced:o!==void 0}),[v,C,c,o]);return s.jsx(R.Provider,{value:A,children:n})}O.displayName="ColorModeProvider";var te=["borders","breakpoints","colors","components","config","direction","fonts","fontSizes","fontWeights","letterSpacings","lineHeights","radii","shadows","sizes","space","styles","transition","zIndices"];function re(e){return V(e)?te.every(o=>Object.prototype.hasOwnProperty.call(e,o)):!1}function m(e){return typeof e=="function"}function oe(...e){return o=>e.reduce((n,r)=>r(n),o)}var ne=e=>function(...n){let r=[...n],t=n[n.length-1];return re(t)&&r.length>1?r=r.slice(0,r.length-1):t=e,oe(...r.map(i=>a=>m(i)?i(a):ae(a,i)))(t)},ie=ne(j);function ae(...e){return z({},...e,_)}function _(e,o,n,r){if((m(e)||m(o))&&Object.prototype.hasOwnProperty.call(r,n))return(...t)=>{const i=m(e)?e(...t):e,a=m(o)?o(...t):o;return z({},i,a,_)}}var q=l.createContext({getDocument(){return document},getWindow(){return window}});q.displayName="EnvironmentContext";function N(e){const{children:o,environment:n,disabled:r}=e,t=l.useRef(null),i=l.useMemo(()=>n||{getDocument:()=>{var d,u;return(u=(d=t.current)==null?void 0:d.ownerDocument)!=null?u:document},getWindow:()=>{var d,u;return(u=(d=t.current)==null?void 0:d.ownerDocument.defaultView)!=null?u:window}},[n]),a=!r||!n;return s.jsxs(q.Provider,{value:i,children:[o,a&&s.jsx("span",{id:"__chakra_env",hidden:!0,ref:t})]})}N.displayName="EnvironmentProvider";var se=e=>{const{children:o,colorModeManager:n,portalZIndex:r,resetScope:t,resetCSS:i=!0,theme:a={},environment:d,cssVarsRoot:u,disableEnvironment:p,disableGlobalStyle:y}=e,b=s.jsx(N,{environment:d,disabled:p,children:o});return s.jsx(D,{theme:a,cssVarsRoot:u,children:s.jsxs(O,{colorModeManager:n,options:a.config,children:[i?s.jsx(J,{scope:t}):s.jsx(B,{}),!y&&s.jsx(F,{}),r?s.jsx(G,{zIndex:r,children:b}):b]})})},le=e=>function({children:n,theme:r=e,toastOptions:t,...i}){return s.jsxs(se,{theme:r,...i,children:[s.jsx(W,{value:t==null?void 0:t.defaultOptions,children:n}),s.jsx(K,{...t})]})},de=le(j);const ue=()=>l.useMemo(()=>({colorScheme:"dark",fontFamily:"'Inter Variable', sans-serif",components:{ScrollArea:{defaultProps:{scrollbarSize:10},styles:{scrollbar:{"&:hover":{backgroundColor:"var(--invokeai-colors-baseAlpha-300)"}},thumb:{backgroundColor:"var(--invokeai-colors-baseAlpha-300)"}}}}}),[]),ce=L("@@invokeai-color-mode");function he({children:e}){const{i18n:o}=H(),n=o.dir(),r=l.useMemo(()=>ie({...U,direction:n}),[n]);l.useEffect(()=>{document.body.dir=n},[n]);const t=ue();return s.jsx(Z,{theme:t,children:s.jsx(de,{theme:r,colorModeManager:ce,toastOptions:Y,children:e})})}const ve=l.memo(he);export{ve as default};
|
158
invokeai/frontend/web/dist/assets/index-6f7e7659.js
vendored
Normal file
158
invokeai/frontend/web/dist/assets/index-6f7e7659.js
vendored
Normal file
File diff suppressed because one or more lines are too long
158
invokeai/frontend/web/dist/assets/index-94062f76.js
vendored
158
invokeai/frontend/web/dist/assets/index-94062f76.js
vendored
File diff suppressed because one or more lines are too long
5
invokeai/frontend/web/dist/index.html
vendored
5
invokeai/frontend/web/dist/index.html
vendored
@ -3,6 +3,9 @@
|
||||
<head>
|
||||
<meta charset="UTF-8" />
|
||||
<meta name="viewport" content="width=device-width, initial-scale=1.0" />
|
||||
<meta http-equiv="Cache-Control" content="no-cache, no-store, must-revalidate">
|
||||
<meta http-equiv="Pragma" content="no-cache">
|
||||
<meta http-equiv="Expires" content="0">
|
||||
<title>InvokeAI - A Stable Diffusion Toolkit</title>
|
||||
<link rel="shortcut icon" type="icon" href="./assets/favicon-0d253ced.ico" />
|
||||
<style>
|
||||
@ -12,7 +15,7 @@
|
||||
margin: 0;
|
||||
}
|
||||
</style>
|
||||
<script type="module" crossorigin src="./assets/index-94062f76.js"></script>
|
||||
<script type="module" crossorigin src="./assets/index-6f7e7659.js"></script>
|
||||
</head>
|
||||
|
||||
<body dir="ltr">
|
||||
|
2
invokeai/frontend/web/dist/locales/en.json
vendored
2
invokeai/frontend/web/dist/locales/en.json
vendored
@ -697,7 +697,7 @@
|
||||
"noLoRAsAvailable": "No LoRAs available",
|
||||
"noMatchingLoRAs": "No matching LoRAs",
|
||||
"noMatchingModels": "No matching Models",
|
||||
"noModelsAvailable": "No Modelss available",
|
||||
"noModelsAvailable": "No models available",
|
||||
"selectLoRA": "Select a LoRA",
|
||||
"selectModel": "Select a Model"
|
||||
},
|
||||
|
@ -3,6 +3,9 @@
|
||||
<head>
|
||||
<meta charset="UTF-8" />
|
||||
<meta name="viewport" content="width=device-width, initial-scale=1.0" />
|
||||
<meta http-equiv="Cache-Control" content="no-cache, no-store, must-revalidate">
|
||||
<meta http-equiv="Pragma" content="no-cache">
|
||||
<meta http-equiv="Expires" content="0">
|
||||
<title>InvokeAI - A Stable Diffusion Toolkit</title>
|
||||
<link rel="shortcut icon" type="icon" href="favicon.ico" />
|
||||
<style>
|
||||
|
@ -697,7 +697,7 @@
|
||||
"noLoRAsAvailable": "No LoRAs available",
|
||||
"noMatchingLoRAs": "No matching LoRAs",
|
||||
"noMatchingModels": "No matching Models",
|
||||
"noModelsAvailable": "No Modelss available",
|
||||
"noModelsAvailable": "No models available",
|
||||
"selectLoRA": "Select a LoRA",
|
||||
"selectModel": "Select a Model"
|
||||
},
|
||||
|
@ -1,5 +1,8 @@
|
||||
import { logger } from 'app/logging/logger';
|
||||
import { controlNetRemoved } from 'features/controlNet/store/controlNetSlice';
|
||||
import {
|
||||
controlNetRemoved,
|
||||
ipAdapterModelChanged,
|
||||
} from 'features/controlNet/store/controlNetSlice';
|
||||
import { loraRemoved } from 'features/lora/store/loraSlice';
|
||||
import {
|
||||
modelChanged,
|
||||
@ -16,12 +19,14 @@ import {
|
||||
} from 'features/sdxl/store/sdxlSlice';
|
||||
import { forEach, some } from 'lodash-es';
|
||||
import {
|
||||
ipAdapterModelsAdapter,
|
||||
mainModelsAdapter,
|
||||
modelsApi,
|
||||
vaeModelsAdapter,
|
||||
} from 'services/api/endpoints/models';
|
||||
import { TypeGuardFor } from 'services/api/types';
|
||||
import { startAppListening } from '..';
|
||||
import { zIPAdapterModel } from 'features/nodes/types/types';
|
||||
|
||||
export const addModelsLoadedListener = () => {
|
||||
startAppListening({
|
||||
@ -234,6 +239,50 @@ export const addModelsLoadedListener = () => {
|
||||
});
|
||||
},
|
||||
});
|
||||
startAppListening({
|
||||
matcher: modelsApi.endpoints.getIPAdapterModels.matchFulfilled,
|
||||
effect: async (action, { getState, dispatch }) => {
|
||||
// ControlNet models loaded - need to remove missing ControlNets from state
|
||||
const log = logger('models');
|
||||
log.info(
|
||||
{ models: action.payload.entities },
|
||||
`IP Adapter models loaded (${action.payload.ids.length})`
|
||||
);
|
||||
|
||||
const { model } = getState().controlNet.ipAdapterInfo;
|
||||
|
||||
const isModelAvailable = some(
|
||||
action.payload.entities,
|
||||
(m) =>
|
||||
m?.model_name === model?.model_name &&
|
||||
m?.base_model === model?.base_model
|
||||
);
|
||||
|
||||
if (isModelAvailable) {
|
||||
return;
|
||||
}
|
||||
|
||||
const firstModel = ipAdapterModelsAdapter
|
||||
.getSelectors()
|
||||
.selectAll(action.payload)[0];
|
||||
|
||||
if (!firstModel) {
|
||||
dispatch(ipAdapterModelChanged(null));
|
||||
}
|
||||
|
||||
const result = zIPAdapterModel.safeParse(firstModel);
|
||||
|
||||
if (!result.success) {
|
||||
log.error(
|
||||
{ error: result.error.format() },
|
||||
'Failed to parse IP Adapter model'
|
||||
);
|
||||
return;
|
||||
}
|
||||
|
||||
dispatch(ipAdapterModelChanged(result.data));
|
||||
},
|
||||
});
|
||||
startAppListening({
|
||||
matcher: modelsApi.endpoints.getTextualInversionModels.matchFulfilled,
|
||||
effect: async (action) => {
|
||||
|
@ -8,6 +8,7 @@ import {
|
||||
} from 'features/gallery/store/gallerySlice';
|
||||
import { IMAGE_CATEGORIES } from 'features/gallery/store/types';
|
||||
import { CANVAS_OUTPUT } from 'features/nodes/util/graphBuilders/constants';
|
||||
import { boardsApi } from 'services/api/endpoints/boards';
|
||||
import { imagesApi } from 'services/api/endpoints/images';
|
||||
import { isImageOutput } from 'services/api/guards';
|
||||
import { imagesAdapter } from 'services/api/util';
|
||||
@ -70,11 +71,21 @@ export const addInvocationCompleteEventListener = () => {
|
||||
)
|
||||
);
|
||||
|
||||
// update the total images for the board
|
||||
dispatch(
|
||||
boardsApi.util.updateQueryData(
|
||||
'getBoardImagesTotal',
|
||||
imageDTO.board_id ?? 'none',
|
||||
(draft) => {
|
||||
// eslint-disable-next-line @typescript-eslint/no-unused-vars
|
||||
draft.total += 1;
|
||||
}
|
||||
)
|
||||
);
|
||||
|
||||
dispatch(
|
||||
imagesApi.util.invalidateTags([
|
||||
{ type: 'BoardImagesTotal', id: imageDTO.board_id },
|
||||
{ type: 'BoardAssetsTotal', id: imageDTO.board_id },
|
||||
{ type: 'Board', id: imageDTO.board_id },
|
||||
{ type: 'Board', id: imageDTO.board_id ?? 'none' },
|
||||
])
|
||||
);
|
||||
|
||||
|
@ -5,8 +5,23 @@ import ParamIPAdapterFeatureToggle from './ParamIPAdapterFeatureToggle';
|
||||
import ParamIPAdapterImage from './ParamIPAdapterImage';
|
||||
import ParamIPAdapterModelSelect from './ParamIPAdapterModelSelect';
|
||||
import ParamIPAdapterWeight from './ParamIPAdapterWeight';
|
||||
import { createSelector } from '@reduxjs/toolkit';
|
||||
import { stateSelector } from '../../../../app/store/store';
|
||||
import { defaultSelectorOptions } from '../../../../app/store/util/defaultMemoizeOptions';
|
||||
import { useAppSelector } from '../../../../app/store/storeHooks';
|
||||
|
||||
const selector = createSelector(
|
||||
stateSelector,
|
||||
(state) => {
|
||||
const { isIPAdapterEnabled } = state.controlNet;
|
||||
|
||||
return { isIPAdapterEnabled };
|
||||
},
|
||||
defaultSelectorOptions
|
||||
);
|
||||
|
||||
const IPAdapterPanel = () => {
|
||||
const { isIPAdapterEnabled } = useAppSelector(selector);
|
||||
return (
|
||||
<Flex
|
||||
sx={{
|
||||
@ -14,7 +29,6 @@ const IPAdapterPanel = () => {
|
||||
gap: 3,
|
||||
paddingInline: 3,
|
||||
paddingBlock: 2,
|
||||
paddingBottom: 5,
|
||||
borderRadius: 'base',
|
||||
position: 'relative',
|
||||
bg: 'base.250',
|
||||
@ -24,10 +38,26 @@ const IPAdapterPanel = () => {
|
||||
}}
|
||||
>
|
||||
<ParamIPAdapterFeatureToggle />
|
||||
<ParamIPAdapterImage />
|
||||
<ParamIPAdapterModelSelect />
|
||||
<ParamIPAdapterWeight />
|
||||
<ParamIPAdapterBeginEnd />
|
||||
{isIPAdapterEnabled && (
|
||||
<>
|
||||
<ParamIPAdapterModelSelect />
|
||||
<Flex gap="3">
|
||||
<Flex
|
||||
flexDirection="column"
|
||||
sx={{
|
||||
h: 28,
|
||||
w: 'full',
|
||||
gap: 4,
|
||||
mb: 4,
|
||||
}}
|
||||
>
|
||||
<ParamIPAdapterWeight />
|
||||
<ParamIPAdapterBeginEnd />
|
||||
</Flex>
|
||||
<ParamIPAdapterImage />
|
||||
</Flex>
|
||||
</>
|
||||
)}
|
||||
</Flex>
|
||||
);
|
||||
};
|
||||
|
@ -66,7 +66,8 @@ const ParamIPAdapterImage = () => {
|
||||
layerStyle="second"
|
||||
sx={{
|
||||
position: 'relative',
|
||||
w: 'full',
|
||||
h: 28,
|
||||
w: 28,
|
||||
alignItems: 'center',
|
||||
justifyContent: 'center',
|
||||
aspectRatio: '1/1',
|
||||
|
@ -88,12 +88,16 @@ const ParamIPAdapterModelSelect = () => {
|
||||
className="nowheel nodrag"
|
||||
tooltip={selectedModel?.description}
|
||||
value={selectedModel?.id ?? null}
|
||||
placeholder="Pick one"
|
||||
error={!selectedModel}
|
||||
placeholder={
|
||||
data.length > 0
|
||||
? t('models.selectModel')
|
||||
: t('models.noModelsAvailable')
|
||||
}
|
||||
error={!selectedModel && data.length > 0}
|
||||
data={data}
|
||||
onChange={handleValueChanged}
|
||||
sx={{ width: '100%' }}
|
||||
disabled={!isEnabled}
|
||||
disabled={!isEnabled || data.length === 0}
|
||||
/>
|
||||
);
|
||||
};
|
||||
|
@ -53,8 +53,12 @@ export const isValidDrop = (
|
||||
}
|
||||
|
||||
if (payloadType === 'IMAGE_DTOS') {
|
||||
// TODO (multi-select)
|
||||
return true;
|
||||
// Assume all images are on the same board - this is true for the moment
|
||||
const { imageDTOs } = active.data.current.payload;
|
||||
const currentBoard = imageDTOs[0]?.board_id ?? 'none';
|
||||
const destinationBoard = overData.context.boardId;
|
||||
|
||||
return currentBoard !== destinationBoard;
|
||||
}
|
||||
|
||||
return false;
|
||||
@ -71,14 +75,17 @@ export const isValidDrop = (
|
||||
// Check if the image's board is the board we are dragging onto
|
||||
if (payloadType === 'IMAGE_DTO') {
|
||||
const { imageDTO } = active.data.current.payload;
|
||||
const currentBoard = imageDTO.board_id;
|
||||
const currentBoard = imageDTO.board_id ?? 'none';
|
||||
|
||||
return currentBoard !== 'none';
|
||||
}
|
||||
|
||||
if (payloadType === 'IMAGE_DTOS') {
|
||||
// TODO (multi-select)
|
||||
return true;
|
||||
// Assume all images are on the same board - this is true for the moment
|
||||
const { imageDTOs } = active.data.current.payload;
|
||||
const currentBoard = imageDTOs[0]?.board_id ?? 'none';
|
||||
|
||||
return currentBoard !== 'none';
|
||||
}
|
||||
|
||||
return false;
|
||||
|
@ -77,12 +77,12 @@ const GalleryBoard = ({
|
||||
const { data: imagesTotal } = useGetBoardImagesTotalQuery(board.board_id);
|
||||
const { data: assetsTotal } = useGetBoardAssetsTotalQuery(board.board_id);
|
||||
const tooltip = useMemo(() => {
|
||||
if (!imagesTotal || !assetsTotal) {
|
||||
if (imagesTotal?.total === undefined || assetsTotal?.total === undefined) {
|
||||
return undefined;
|
||||
}
|
||||
return `${imagesTotal} image${
|
||||
imagesTotal > 1 ? 's' : ''
|
||||
}, ${assetsTotal} asset${assetsTotal > 1 ? 's' : ''}`;
|
||||
return `${imagesTotal.total} image${imagesTotal.total === 1 ? '' : 's'}, ${
|
||||
assetsTotal.total
|
||||
} asset${assetsTotal.total === 1 ? '' : 's'}`;
|
||||
}, [assetsTotal, imagesTotal]);
|
||||
|
||||
const { currentData: coverImage } = useGetImageDTOQuery(
|
||||
|
@ -1,4 +1,4 @@
|
||||
import { Box, Flex, Image, Text } from '@chakra-ui/react';
|
||||
import { Box, Flex, Image, Text, Tooltip } from '@chakra-ui/react';
|
||||
import { createSelector } from '@reduxjs/toolkit';
|
||||
import { stateSelector } from 'app/store/store';
|
||||
import { useAppDispatch, useAppSelector } from 'app/store/storeHooks';
|
||||
@ -15,6 +15,10 @@ import { memo, useCallback, useMemo, useState } from 'react';
|
||||
import { useBoardName } from 'services/api/hooks/useBoardName';
|
||||
import AutoAddIcon from '../AutoAddIcon';
|
||||
import BoardContextMenu from '../BoardContextMenu';
|
||||
import {
|
||||
useGetBoardAssetsTotalQuery,
|
||||
useGetBoardImagesTotalQuery,
|
||||
} from 'services/api/endpoints/boards';
|
||||
|
||||
interface Props {
|
||||
isSelected: boolean;
|
||||
@ -41,6 +45,17 @@ const NoBoardBoard = memo(({ isSelected }: Props) => {
|
||||
}, [dispatch, autoAssignBoardOnClick]);
|
||||
const [isHovered, setIsHovered] = useState(false);
|
||||
|
||||
const { data: imagesTotal } = useGetBoardImagesTotalQuery('none');
|
||||
const { data: assetsTotal } = useGetBoardAssetsTotalQuery('none');
|
||||
const tooltip = useMemo(() => {
|
||||
if (imagesTotal?.total === undefined || assetsTotal?.total === undefined) {
|
||||
return undefined;
|
||||
}
|
||||
return `${imagesTotal.total} image${imagesTotal.total === 1 ? '' : 's'}, ${
|
||||
assetsTotal.total
|
||||
} asset${assetsTotal.total === 1 ? '' : 's'}`;
|
||||
}, [assetsTotal, imagesTotal]);
|
||||
|
||||
const handleMouseOver = useCallback(() => {
|
||||
setIsHovered(true);
|
||||
}, []);
|
||||
@ -74,77 +89,82 @@ const NoBoardBoard = memo(({ isSelected }: Props) => {
|
||||
>
|
||||
<BoardContextMenu board_id="none">
|
||||
{(ref) => (
|
||||
<Flex
|
||||
ref={ref}
|
||||
onClick={handleSelectBoard}
|
||||
sx={{
|
||||
w: 'full',
|
||||
h: 'full',
|
||||
position: 'relative',
|
||||
justifyContent: 'center',
|
||||
alignItems: 'center',
|
||||
borderRadius: 'base',
|
||||
cursor: 'pointer',
|
||||
bg: 'base.200',
|
||||
_dark: {
|
||||
bg: 'base.800',
|
||||
},
|
||||
}}
|
||||
>
|
||||
<Tooltip label={tooltip} openDelay={1000} hasArrow>
|
||||
<Flex
|
||||
ref={ref}
|
||||
onClick={handleSelectBoard}
|
||||
sx={{
|
||||
w: 'full',
|
||||
h: 'full',
|
||||
position: 'relative',
|
||||
justifyContent: 'center',
|
||||
alignItems: 'center',
|
||||
borderRadius: 'base',
|
||||
cursor: 'pointer',
|
||||
bg: 'base.200',
|
||||
_dark: {
|
||||
bg: 'base.800',
|
||||
},
|
||||
}}
|
||||
>
|
||||
<Image
|
||||
src={InvokeAILogoImage}
|
||||
alt="invoke-ai-logo"
|
||||
<Flex
|
||||
sx={{
|
||||
opacity: 0.4,
|
||||
filter: 'grayscale(1)',
|
||||
mt: -6,
|
||||
w: 16,
|
||||
h: 16,
|
||||
minW: 16,
|
||||
minH: 16,
|
||||
userSelect: 'none',
|
||||
w: 'full',
|
||||
h: 'full',
|
||||
justifyContent: 'center',
|
||||
alignItems: 'center',
|
||||
}}
|
||||
>
|
||||
<Image
|
||||
src={InvokeAILogoImage}
|
||||
alt="invoke-ai-logo"
|
||||
sx={{
|
||||
opacity: 0.4,
|
||||
filter: 'grayscale(1)',
|
||||
mt: -6,
|
||||
w: 16,
|
||||
h: 16,
|
||||
minW: 16,
|
||||
minH: 16,
|
||||
userSelect: 'none',
|
||||
}}
|
||||
/>
|
||||
</Flex>
|
||||
{autoAddBoardId === 'none' && <AutoAddIcon />}
|
||||
<Flex
|
||||
sx={{
|
||||
position: 'absolute',
|
||||
bottom: 0,
|
||||
left: 0,
|
||||
p: 1,
|
||||
justifyContent: 'center',
|
||||
alignItems: 'center',
|
||||
w: 'full',
|
||||
maxW: 'full',
|
||||
borderBottomRadius: 'base',
|
||||
bg: isSelected ? 'accent.400' : 'base.500',
|
||||
color: isSelected ? 'base.50' : 'base.100',
|
||||
_dark: {
|
||||
bg: isSelected ? 'accent.500' : 'base.600',
|
||||
color: isSelected ? 'base.50' : 'base.100',
|
||||
},
|
||||
lineHeight: 'short',
|
||||
fontSize: 'xs',
|
||||
fontWeight: isSelected ? 700 : 500,
|
||||
}}
|
||||
>
|
||||
{boardName}
|
||||
</Flex>
|
||||
<SelectionOverlay
|
||||
isSelected={isSelected}
|
||||
isHovered={isHovered}
|
||||
/>
|
||||
<IAIDroppable
|
||||
data={droppableData}
|
||||
dropLabel={<Text fontSize="md">Move</Text>}
|
||||
/>
|
||||
</Flex>
|
||||
{autoAddBoardId === 'none' && <AutoAddIcon />}
|
||||
<Flex
|
||||
sx={{
|
||||
position: 'absolute',
|
||||
bottom: 0,
|
||||
left: 0,
|
||||
p: 1,
|
||||
justifyContent: 'center',
|
||||
alignItems: 'center',
|
||||
w: 'full',
|
||||
maxW: 'full',
|
||||
borderBottomRadius: 'base',
|
||||
bg: isSelected ? 'accent.400' : 'base.500',
|
||||
color: isSelected ? 'base.50' : 'base.100',
|
||||
_dark: {
|
||||
bg: isSelected ? 'accent.500' : 'base.600',
|
||||
color: isSelected ? 'base.50' : 'base.100',
|
||||
},
|
||||
lineHeight: 'short',
|
||||
fontSize: 'xs',
|
||||
fontWeight: isSelected ? 700 : 500,
|
||||
}}
|
||||
>
|
||||
{boardName}
|
||||
</Flex>
|
||||
<SelectionOverlay isSelected={isSelected} isHovered={isHovered} />
|
||||
<IAIDroppable
|
||||
data={droppableData}
|
||||
dropLabel={<Text fontSize="md">Move</Text>}
|
||||
/>
|
||||
</Flex>
|
||||
</Tooltip>
|
||||
)}
|
||||
</BoardContextMenu>
|
||||
</Flex>
|
||||
|
@ -3,13 +3,12 @@ import { stateSelector } from 'app/store/store';
|
||||
import { useAppDispatch, useAppSelector } from 'app/store/storeHooks';
|
||||
import { defaultSelectorOptions } from 'app/store/util/defaultMemoizeOptions';
|
||||
import { selectListImagesBaseQueryArgs } from 'features/gallery/store/gallerySelectors';
|
||||
import { uniq } from 'lodash-es';
|
||||
import { MouseEvent, useCallback, useMemo } from 'react';
|
||||
import { useListImagesQuery } from 'services/api/endpoints/images';
|
||||
import { ImageDTO } from 'services/api/types';
|
||||
import { selectionChanged } from '../store/gallerySlice';
|
||||
import { imagesSelectors } from 'services/api/util';
|
||||
import { useFeatureStatus } from '../../system/hooks/useFeatureStatus';
|
||||
import { selectionChanged } from '../store/gallerySlice';
|
||||
|
||||
const selector = createSelector(
|
||||
[stateSelector, selectListImagesBaseQueryArgs],
|
||||
@ -60,7 +59,7 @@ export const useMultiselect = (imageDTO?: ImageDTO) => {
|
||||
const start = Math.min(lastClickedIndex, currentClickedIndex);
|
||||
const end = Math.max(lastClickedIndex, currentClickedIndex);
|
||||
const imagesToSelect = imageDTOs.slice(start, end + 1);
|
||||
dispatch(selectionChanged(uniq(selection.concat(imagesToSelect))));
|
||||
dispatch(selectionChanged(selection.concat(imagesToSelect)));
|
||||
}
|
||||
} else if (e.ctrlKey || e.metaKey) {
|
||||
if (
|
||||
@ -73,7 +72,7 @@ export const useMultiselect = (imageDTO?: ImageDTO) => {
|
||||
)
|
||||
);
|
||||
} else {
|
||||
dispatch(selectionChanged(uniq(selection.concat(imageDTO))));
|
||||
dispatch(selectionChanged(selection.concat(imageDTO)));
|
||||
}
|
||||
} else {
|
||||
dispatch(selectionChanged([imageDTO]));
|
||||
|
@ -20,7 +20,7 @@ export const nextPrevImageButtonsSelector = createSelector(
|
||||
const { data, status } =
|
||||
imagesApi.endpoints.listImages.select(baseQueryArgs)(state);
|
||||
|
||||
const { data: total } =
|
||||
const { data: totalsData } =
|
||||
state.gallery.galleryView === 'images'
|
||||
? boardsApi.endpoints.getBoardImagesTotal.select(
|
||||
baseQueryArgs.board_id ?? 'none'
|
||||
@ -34,7 +34,7 @@ export const nextPrevImageButtonsSelector = createSelector(
|
||||
|
||||
const isFetching = status === 'pending';
|
||||
|
||||
if (!data || !lastSelectedImage || total === 0) {
|
||||
if (!data || !lastSelectedImage || totalsData?.total === 0) {
|
||||
return {
|
||||
isFetching,
|
||||
queryArgs: baseQueryArgs,
|
||||
@ -74,7 +74,7 @@ export const nextPrevImageButtonsSelector = createSelector(
|
||||
return {
|
||||
loadedImagesCount: images.length,
|
||||
currentImageIndex,
|
||||
areMoreImagesAvailable: (total ?? 0) > imagesLength,
|
||||
areMoreImagesAvailable: (totalsData?.total ?? 0) > imagesLength,
|
||||
isFetching: status === 'pending',
|
||||
nextImage,
|
||||
prevImage,
|
||||
|
@ -4,6 +4,7 @@ import { boardsApi } from 'services/api/endpoints/boards';
|
||||
import { imagesApi } from 'services/api/endpoints/images';
|
||||
import { ImageDTO } from 'services/api/types';
|
||||
import { BoardId, GalleryState, GalleryView } from './types';
|
||||
import { uniqBy } from 'lodash-es';
|
||||
|
||||
export const initialGalleryState: GalleryState = {
|
||||
selection: [],
|
||||
@ -24,7 +25,7 @@ export const gallerySlice = createSlice({
|
||||
state.selection = action.payload ? [action.payload] : [];
|
||||
},
|
||||
selectionChanged: (state, action: PayloadAction<ImageDTO[]>) => {
|
||||
state.selection = action.payload;
|
||||
state.selection = uniqBy(action.payload, (i) => i.image_name);
|
||||
},
|
||||
shouldAutoSwitchChanged: (state, action: PayloadAction<boolean>) => {
|
||||
state.shouldAutoSwitch = action.payload;
|
||||
|
@ -16,6 +16,7 @@ import SchedulerInputField from './inputs/SchedulerInputField';
|
||||
import StringInputField from './inputs/StringInputField';
|
||||
import VaeModelInputField from './inputs/VaeModelInputField';
|
||||
import IPAdapterModelInputField from './inputs/IPAdapterModelInputField';
|
||||
import T2IAdapterModelInputField from './inputs/T2IAdapterModelInputField';
|
||||
import BoardInputField from './inputs/BoardInputField';
|
||||
|
||||
type InputFieldProps = {
|
||||
@ -188,6 +189,18 @@ const InputFieldRenderer = ({ nodeId, fieldName }: InputFieldProps) => {
|
||||
);
|
||||
}
|
||||
|
||||
if (
|
||||
field?.type === 'T2IAdapterModelField' &&
|
||||
fieldTemplate?.type === 'T2IAdapterModelField'
|
||||
) {
|
||||
return (
|
||||
<T2IAdapterModelInputField
|
||||
nodeId={nodeId}
|
||||
field={field}
|
||||
fieldTemplate={fieldTemplate}
|
||||
/>
|
||||
);
|
||||
}
|
||||
if (field?.type === 'ColorField' && fieldTemplate?.type === 'ColorField') {
|
||||
return (
|
||||
<ColorInputField
|
||||
|
@ -0,0 +1,19 @@
|
||||
import {
|
||||
T2IAdapterInputFieldTemplate,
|
||||
T2IAdapterInputFieldValue,
|
||||
T2IAdapterPolymorphicInputFieldTemplate,
|
||||
T2IAdapterPolymorphicInputFieldValue,
|
||||
FieldComponentProps,
|
||||
} from 'features/nodes/types/types';
|
||||
import { memo } from 'react';
|
||||
|
||||
const T2IAdapterInputFieldComponent = (
|
||||
_props: FieldComponentProps<
|
||||
T2IAdapterInputFieldValue | T2IAdapterPolymorphicInputFieldValue,
|
||||
T2IAdapterInputFieldTemplate | T2IAdapterPolymorphicInputFieldTemplate
|
||||
>
|
||||
) => {
|
||||
return null;
|
||||
};
|
||||
|
||||
export default memo(T2IAdapterInputFieldComponent);
|
@ -0,0 +1,100 @@
|
||||
import { SelectItem } from '@mantine/core';
|
||||
import { useAppDispatch } from 'app/store/storeHooks';
|
||||
import IAIMantineSelect from 'common/components/IAIMantineSelect';
|
||||
import { fieldT2IAdapterModelValueChanged } from 'features/nodes/store/nodesSlice';
|
||||
import {
|
||||
T2IAdapterModelInputFieldTemplate,
|
||||
T2IAdapterModelInputFieldValue,
|
||||
FieldComponentProps,
|
||||
} from 'features/nodes/types/types';
|
||||
import { MODEL_TYPE_MAP } from 'features/parameters/types/constants';
|
||||
import { modelIdToT2IAdapterModelParam } from 'features/parameters/util/modelIdToT2IAdapterModelParam';
|
||||
import { forEach } from 'lodash-es';
|
||||
import { memo, useCallback, useMemo } from 'react';
|
||||
import { useGetT2IAdapterModelsQuery } from 'services/api/endpoints/models';
|
||||
|
||||
const T2IAdapterModelInputFieldComponent = (
|
||||
props: FieldComponentProps<
|
||||
T2IAdapterModelInputFieldValue,
|
||||
T2IAdapterModelInputFieldTemplate
|
||||
>
|
||||
) => {
|
||||
const { nodeId, field } = props;
|
||||
const t2iAdapterModel = field.value;
|
||||
const dispatch = useAppDispatch();
|
||||
|
||||
const { data: t2iAdapterModels } = useGetT2IAdapterModelsQuery();
|
||||
|
||||
// grab the full model entity from the RTK Query cache
|
||||
const selectedModel = useMemo(
|
||||
() =>
|
||||
t2iAdapterModels?.entities[
|
||||
`${t2iAdapterModel?.base_model}/t2i_adapter/${t2iAdapterModel?.model_name}`
|
||||
] ?? null,
|
||||
[
|
||||
t2iAdapterModel?.base_model,
|
||||
t2iAdapterModel?.model_name,
|
||||
t2iAdapterModels?.entities,
|
||||
]
|
||||
);
|
||||
|
||||
const data = useMemo(() => {
|
||||
if (!t2iAdapterModels) {
|
||||
return [];
|
||||
}
|
||||
|
||||
const data: SelectItem[] = [];
|
||||
|
||||
forEach(t2iAdapterModels.entities, (model, id) => {
|
||||
if (!model) {
|
||||
return;
|
||||
}
|
||||
|
||||
data.push({
|
||||
value: id,
|
||||
label: model.model_name,
|
||||
group: MODEL_TYPE_MAP[model.base_model],
|
||||
});
|
||||
});
|
||||
|
||||
return data;
|
||||
}, [t2iAdapterModels]);
|
||||
|
||||
const handleValueChanged = useCallback(
|
||||
(v: string | null) => {
|
||||
if (!v) {
|
||||
return;
|
||||
}
|
||||
|
||||
const newT2IAdapterModel = modelIdToT2IAdapterModelParam(v);
|
||||
|
||||
if (!newT2IAdapterModel) {
|
||||
return;
|
||||
}
|
||||
|
||||
dispatch(
|
||||
fieldT2IAdapterModelValueChanged({
|
||||
nodeId,
|
||||
fieldName: field.name,
|
||||
value: newT2IAdapterModel,
|
||||
})
|
||||
);
|
||||
},
|
||||
[dispatch, field.name, nodeId]
|
||||
);
|
||||
|
||||
return (
|
||||
<IAIMantineSelect
|
||||
className="nowheel nodrag"
|
||||
tooltip={selectedModel?.description}
|
||||
value={selectedModel?.id ?? null}
|
||||
placeholder="Pick one"
|
||||
error={!selectedModel}
|
||||
data={data}
|
||||
onChange={handleValueChanged}
|
||||
sx={{ width: '100%' }}
|
||||
/>
|
||||
);
|
||||
};
|
||||
|
||||
export default memo(T2IAdapterModelInputFieldComponent);
|
@ -55,6 +55,7 @@ import {
|
||||
SchedulerInputFieldValue,
|
||||
SDXLRefinerModelInputFieldValue,
|
||||
StringInputFieldValue,
|
||||
T2IAdapterModelInputFieldValue,
|
||||
VaeModelInputFieldValue,
|
||||
Workflow,
|
||||
} from '../types/types';
|
||||
@ -645,6 +646,12 @@ const nodesSlice = createSlice({
|
||||
) => {
|
||||
fieldValueReducer(state, action);
|
||||
},
|
||||
fieldT2IAdapterModelValueChanged: (
|
||||
state,
|
||||
action: FieldValueAction<T2IAdapterModelInputFieldValue>
|
||||
) => {
|
||||
fieldValueReducer(state, action);
|
||||
},
|
||||
fieldEnumModelValueChanged: (
|
||||
state,
|
||||
action: FieldValueAction<EnumInputFieldValue>
|
||||
@ -1009,6 +1016,7 @@ export const {
|
||||
fieldEnumModelValueChanged,
|
||||
fieldImageValueChanged,
|
||||
fieldIPAdapterModelValueChanged,
|
||||
fieldT2IAdapterModelValueChanged,
|
||||
fieldLabelChanged,
|
||||
fieldLoRAModelValueChanged,
|
||||
fieldMainModelValueChanged,
|
||||
|
@ -31,6 +31,7 @@ export const COLLECTION_TYPES: FieldType[] = [
|
||||
'ConditioningCollection',
|
||||
'ControlCollection',
|
||||
'ColorCollection',
|
||||
'T2IAdapterCollection',
|
||||
];
|
||||
|
||||
export const POLYMORPHIC_TYPES: FieldType[] = [
|
||||
@ -43,6 +44,7 @@ export const POLYMORPHIC_TYPES: FieldType[] = [
|
||||
'ConditioningPolymorphic',
|
||||
'ControlPolymorphic',
|
||||
'ColorPolymorphic',
|
||||
'T2IAdapterPolymorphic',
|
||||
];
|
||||
|
||||
export const MODEL_TYPES: FieldType[] = [
|
||||
@ -57,6 +59,7 @@ export const MODEL_TYPES: FieldType[] = [
|
||||
'UNetField',
|
||||
'VaeField',
|
||||
'ClipField',
|
||||
'T2IAdapterModelField',
|
||||
];
|
||||
|
||||
export const COLLECTION_MAP: FieldTypeMapWithNumber = {
|
||||
@ -70,6 +73,7 @@ export const COLLECTION_MAP: FieldTypeMapWithNumber = {
|
||||
ConditioningField: 'ConditioningCollection',
|
||||
ControlField: 'ControlCollection',
|
||||
ColorField: 'ColorCollection',
|
||||
T2IAdapterField: 'T2IAdapterCollection',
|
||||
};
|
||||
export const isCollectionItemType = (
|
||||
itemType: string | undefined
|
||||
@ -87,6 +91,7 @@ export const SINGLE_TO_POLYMORPHIC_MAP: FieldTypeMapWithNumber = {
|
||||
ConditioningField: 'ConditioningPolymorphic',
|
||||
ControlField: 'ControlPolymorphic',
|
||||
ColorField: 'ColorPolymorphic',
|
||||
T2IAdapterField: 'T2IAdapterPolymorphic',
|
||||
};
|
||||
|
||||
export const POLYMORPHIC_TO_SINGLE_MAP: FieldTypeMap = {
|
||||
@ -99,6 +104,7 @@ export const POLYMORPHIC_TO_SINGLE_MAP: FieldTypeMap = {
|
||||
ConditioningPolymorphic: 'ConditioningField',
|
||||
ControlPolymorphic: 'ControlField',
|
||||
ColorPolymorphic: 'ColorField',
|
||||
T2IAdapterPolymorphic: 'T2IAdapterField',
|
||||
};
|
||||
|
||||
export const TYPES_WITH_INPUT_COMPONENTS: FieldType[] = [
|
||||
@ -123,6 +129,7 @@ export const TYPES_WITH_INPUT_COMPONENTS: FieldType[] = [
|
||||
'Scheduler',
|
||||
'IPAdapterModelField',
|
||||
'BoardField',
|
||||
'T2IAdapterModelField',
|
||||
];
|
||||
|
||||
export const isPolymorphicItemType = (
|
||||
@ -272,7 +279,7 @@ export const FIELDS: Record<FieldType, FieldUIConfig> = {
|
||||
title: t('nodes.integerPolymorphic'),
|
||||
},
|
||||
IPAdapterField: {
|
||||
color: 'green.300',
|
||||
color: 'teal.500',
|
||||
description: 'IP-Adapter info passed between nodes.',
|
||||
title: 'IP-Adapter',
|
||||
},
|
||||
@ -341,6 +348,26 @@ export const FIELDS: Record<FieldType, FieldUIConfig> = {
|
||||
description: t('nodes.stringPolymorphicDescription'),
|
||||
title: t('nodes.stringPolymorphic'),
|
||||
},
|
||||
T2IAdapterCollection: {
|
||||
color: 'teal.500',
|
||||
description: t('nodes.t2iAdapterCollectionDescription'),
|
||||
title: t('nodes.t2iAdapterCollection'),
|
||||
},
|
||||
T2IAdapterField: {
|
||||
color: 'teal.500',
|
||||
description: t('nodes.t2iAdapterFieldDescription'),
|
||||
title: t('nodes.t2iAdapterField'),
|
||||
},
|
||||
T2IAdapterModelField: {
|
||||
color: 'teal.500',
|
||||
description: 'TODO',
|
||||
title: 'T2I-Adapter',
|
||||
},
|
||||
T2IAdapterPolymorphic: {
|
||||
color: 'teal.500',
|
||||
description: 'T2I-Adapter info passed between nodes.',
|
||||
title: 'T2I-Adapter Polymorphic',
|
||||
},
|
||||
UNetField: {
|
||||
color: 'red.500',
|
||||
description: t('nodes.uNetFieldDescription'),
|
||||
|
@ -114,6 +114,10 @@ export const zFieldType = z.enum([
|
||||
'string',
|
||||
'StringCollection',
|
||||
'StringPolymorphic',
|
||||
'T2IAdapterCollection',
|
||||
'T2IAdapterField',
|
||||
'T2IAdapterModelField',
|
||||
'T2IAdapterPolymorphic',
|
||||
'UNetField',
|
||||
'VaeField',
|
||||
'VaeModelField',
|
||||
@ -426,6 +430,48 @@ export type IPAdapterInputFieldValue = z.infer<
|
||||
typeof zIPAdapterInputFieldValue
|
||||
>;
|
||||
|
||||
export const zT2IAdapterModel = zModelIdentifier;
|
||||
export type T2IAdapterModel = z.infer<typeof zT2IAdapterModel>;
|
||||
|
||||
export const zT2IAdapterField = z.object({
|
||||
image: zImageField,
|
||||
t2i_adapter_model: zT2IAdapterModel,
|
||||
weight: z.union([z.number(), z.array(z.number())]).optional(),
|
||||
begin_step_percent: z.number().optional(),
|
||||
end_step_percent: z.number().optional(),
|
||||
resize_mode: z
|
||||
.enum(['just_resize', 'crop_resize', 'fill_resize', 'just_resize_simple'])
|
||||
.optional(),
|
||||
});
|
||||
export type T2IAdapterField = z.infer<typeof zT2IAdapterField>;
|
||||
|
||||
export const zT2IAdapterInputFieldValue = zInputFieldValueBase.extend({
|
||||
type: z.literal('T2IAdapterField'),
|
||||
value: zT2IAdapterField.optional(),
|
||||
});
|
||||
export type T2IAdapterInputFieldValue = z.infer<
|
||||
typeof zT2IAdapterInputFieldValue
|
||||
>;
|
||||
|
||||
export const zT2IAdapterPolymorphicInputFieldValue =
|
||||
zInputFieldValueBase.extend({
|
||||
type: z.literal('T2IAdapterPolymorphic'),
|
||||
value: z.union([zT2IAdapterField, z.array(zT2IAdapterField)]).optional(),
|
||||
});
|
||||
export type T2IAdapterPolymorphicInputFieldValue = z.infer<
|
||||
typeof zT2IAdapterPolymorphicInputFieldValue
|
||||
>;
|
||||
|
||||
export const zT2IAdapterCollectionInputFieldValue = zInputFieldValueBase.extend(
|
||||
{
|
||||
type: z.literal('T2IAdapterCollection'),
|
||||
value: z.array(zT2IAdapterField).optional(),
|
||||
}
|
||||
);
|
||||
export type T2IAdapterCollectionInputFieldValue = z.infer<
|
||||
typeof zT2IAdapterCollectionInputFieldValue
|
||||
>;
|
||||
|
||||
export const zModelType = z.enum([
|
||||
'onnx',
|
||||
'main',
|
||||
@ -592,6 +638,17 @@ export type IPAdapterModelInputFieldValue = z.infer<
|
||||
typeof zIPAdapterModelInputFieldValue
|
||||
>;
|
||||
|
||||
export const zT2IAdapterModelField = zModelIdentifier;
|
||||
export type T2IAdapterModelField = z.infer<typeof zT2IAdapterModelField>;
|
||||
|
||||
export const zT2IAdapterModelInputFieldValue = zInputFieldValueBase.extend({
|
||||
type: z.literal('T2IAdapterModelField'),
|
||||
value: zT2IAdapterModelField.optional(),
|
||||
});
|
||||
export type T2IAdapterModelInputFieldValue = z.infer<
|
||||
typeof zT2IAdapterModelInputFieldValue
|
||||
>;
|
||||
|
||||
export const zCollectionInputFieldValue = zInputFieldValueBase.extend({
|
||||
type: z.literal('Collection'),
|
||||
value: z.array(z.any()).optional(), // TODO: should this field ever have a value?
|
||||
@ -688,6 +745,10 @@ export const zInputFieldValue = z.discriminatedUnion('type', [
|
||||
zStringCollectionInputFieldValue,
|
||||
zStringPolymorphicInputFieldValue,
|
||||
zStringInputFieldValue,
|
||||
zT2IAdapterInputFieldValue,
|
||||
zT2IAdapterModelInputFieldValue,
|
||||
zT2IAdapterCollectionInputFieldValue,
|
||||
zT2IAdapterPolymorphicInputFieldValue,
|
||||
zUNetInputFieldValue,
|
||||
zVaeInputFieldValue,
|
||||
zVaeModelInputFieldValue,
|
||||
@ -889,6 +950,24 @@ export type IPAdapterInputFieldTemplate = InputFieldTemplateBase & {
|
||||
type: 'IPAdapterField';
|
||||
};
|
||||
|
||||
export type T2IAdapterInputFieldTemplate = InputFieldTemplateBase & {
|
||||
default: undefined;
|
||||
type: 'T2IAdapterField';
|
||||
};
|
||||
|
||||
export type T2IAdapterCollectionInputFieldTemplate = InputFieldTemplateBase & {
|
||||
default: undefined;
|
||||
type: 'T2IAdapterCollection';
|
||||
item_default?: T2IAdapterField;
|
||||
};
|
||||
|
||||
export type T2IAdapterPolymorphicInputFieldTemplate = Omit<
|
||||
T2IAdapterInputFieldTemplate,
|
||||
'type'
|
||||
> & {
|
||||
type: 'T2IAdapterPolymorphic';
|
||||
};
|
||||
|
||||
export type EnumInputFieldTemplate = InputFieldTemplateBase & {
|
||||
default: string;
|
||||
type: 'enum';
|
||||
@ -931,6 +1010,11 @@ export type IPAdapterModelInputFieldTemplate = InputFieldTemplateBase & {
|
||||
type: 'IPAdapterModelField';
|
||||
};
|
||||
|
||||
export type T2IAdapterModelInputFieldTemplate = InputFieldTemplateBase & {
|
||||
default: string;
|
||||
type: 'T2IAdapterModelField';
|
||||
};
|
||||
|
||||
export type CollectionInputFieldTemplate = InputFieldTemplateBase & {
|
||||
default: [];
|
||||
type: 'Collection';
|
||||
@ -1016,6 +1100,10 @@ export type InputFieldTemplate =
|
||||
| StringCollectionInputFieldTemplate
|
||||
| StringPolymorphicInputFieldTemplate
|
||||
| StringInputFieldTemplate
|
||||
| T2IAdapterInputFieldTemplate
|
||||
| T2IAdapterCollectionInputFieldTemplate
|
||||
| T2IAdapterModelInputFieldTemplate
|
||||
| T2IAdapterPolymorphicInputFieldTemplate
|
||||
| UNetInputFieldTemplate
|
||||
| VaeInputFieldTemplate
|
||||
| VaeModelInputFieldTemplate;
|
||||
|
@ -62,6 +62,11 @@ import {
|
||||
ConditioningField,
|
||||
IPAdapterInputFieldTemplate,
|
||||
IPAdapterModelInputFieldTemplate,
|
||||
T2IAdapterField,
|
||||
T2IAdapterInputFieldTemplate,
|
||||
T2IAdapterModelInputFieldTemplate,
|
||||
T2IAdapterPolymorphicInputFieldTemplate,
|
||||
T2IAdapterCollectionInputFieldTemplate,
|
||||
BoardInputFieldTemplate,
|
||||
InputFieldTemplate,
|
||||
} from '../types/types';
|
||||
@ -452,6 +457,19 @@ const buildIPAdapterModelInputFieldTemplate = ({
|
||||
return template;
|
||||
};
|
||||
|
||||
const buildT2IAdapterModelInputFieldTemplate = ({
|
||||
schemaObject,
|
||||
baseField,
|
||||
}: BuildInputFieldArg): T2IAdapterModelInputFieldTemplate => {
|
||||
const template: T2IAdapterModelInputFieldTemplate = {
|
||||
...baseField,
|
||||
type: 'T2IAdapterModelField',
|
||||
default: schemaObject.default ?? undefined,
|
||||
};
|
||||
|
||||
return template;
|
||||
};
|
||||
|
||||
const buildBoardInputFieldTemplate = ({
|
||||
schemaObject,
|
||||
baseField,
|
||||
@ -691,6 +709,46 @@ const buildIPAdapterInputFieldTemplate = ({
|
||||
return template;
|
||||
};
|
||||
|
||||
const buildT2IAdapterInputFieldTemplate = ({
|
||||
schemaObject,
|
||||
baseField,
|
||||
}: BuildInputFieldArg): T2IAdapterInputFieldTemplate => {
|
||||
const template: T2IAdapterInputFieldTemplate = {
|
||||
...baseField,
|
||||
type: 'T2IAdapterField',
|
||||
default: schemaObject.default ?? undefined,
|
||||
};
|
||||
|
||||
return template;
|
||||
};
|
||||
|
||||
const buildT2IAdapterPolymorphicInputFieldTemplate = ({
|
||||
schemaObject,
|
||||
baseField,
|
||||
}: BuildInputFieldArg): T2IAdapterPolymorphicInputFieldTemplate => {
|
||||
const template: T2IAdapterPolymorphicInputFieldTemplate = {
|
||||
...baseField,
|
||||
type: 'T2IAdapterPolymorphic',
|
||||
default: schemaObject.default ?? undefined,
|
||||
};
|
||||
|
||||
return template;
|
||||
};
|
||||
|
||||
const buildT2IAdapterCollectionInputFieldTemplate = ({
|
||||
schemaObject,
|
||||
baseField,
|
||||
}: BuildInputFieldArg): T2IAdapterCollectionInputFieldTemplate => {
|
||||
const template: T2IAdapterCollectionInputFieldTemplate = {
|
||||
...baseField,
|
||||
type: 'T2IAdapterCollection',
|
||||
default: schemaObject.default ?? [],
|
||||
item_default: (schemaObject.item_default as T2IAdapterField) ?? undefined,
|
||||
};
|
||||
|
||||
return template;
|
||||
};
|
||||
|
||||
const buildEnumInputFieldTemplate = ({
|
||||
schemaObject,
|
||||
baseField,
|
||||
@ -910,6 +968,10 @@ const TEMPLATE_BUILDER_MAP: {
|
||||
string: buildStringInputFieldTemplate,
|
||||
StringCollection: buildStringCollectionInputFieldTemplate,
|
||||
StringPolymorphic: buildStringPolymorphicInputFieldTemplate,
|
||||
T2IAdapterCollection: buildT2IAdapterCollectionInputFieldTemplate,
|
||||
T2IAdapterField: buildT2IAdapterInputFieldTemplate,
|
||||
T2IAdapterModelField: buildT2IAdapterModelInputFieldTemplate,
|
||||
T2IAdapterPolymorphic: buildT2IAdapterPolymorphicInputFieldTemplate,
|
||||
UNetField: buildUNetInputFieldTemplate,
|
||||
VaeField: buildVaeInputFieldTemplate,
|
||||
VaeModelField: buildVaeModelInputFieldTemplate,
|
||||
|
@ -45,6 +45,10 @@ const FIELD_VALUE_FALLBACK_MAP: {
|
||||
string: '',
|
||||
StringCollection: [],
|
||||
StringPolymorphic: '',
|
||||
T2IAdapterCollection: [],
|
||||
T2IAdapterField: undefined,
|
||||
T2IAdapterModelField: undefined,
|
||||
T2IAdapterPolymorphic: undefined,
|
||||
UNetField: undefined,
|
||||
VaeField: undefined,
|
||||
VaeModelField: undefined,
|
||||
|
@ -340,6 +340,17 @@ export const zIPAdapterModel = z.object({
|
||||
* Type alias for model parameter, inferred from its zod schema
|
||||
*/
|
||||
export type IPAdapterModelParam = z.infer<typeof zIPAdapterModel>;
|
||||
/**
|
||||
* Zod schema for T2I-Adapter models
|
||||
*/
|
||||
export const zT2IAdapterModel = z.object({
|
||||
model_name: z.string().min(1),
|
||||
base_model: zBaseModel,
|
||||
});
|
||||
/**
|
||||
* Type alias for model parameter, inferred from its zod schema
|
||||
*/
|
||||
export type T2IAdapterModelParam = z.infer<typeof zT2IAdapterModel>;
|
||||
/**
|
||||
* Zod schema for l2l strength parameter
|
||||
*/
|
||||
|
@ -0,0 +1,29 @@
|
||||
import { logger } from 'app/logging/logger';
|
||||
import { zT2IAdapterModel } from 'features/parameters/types/parameterSchemas';
|
||||
import { T2IAdapterModelField } from 'services/api/types';
|
||||
|
||||
export const modelIdToT2IAdapterModelParam = (
|
||||
t2iAdapterModelId: string
|
||||
): T2IAdapterModelField | undefined => {
|
||||
const log = logger('models');
|
||||
const [base_model, _model_type, model_name] = t2iAdapterModelId.split('/');
|
||||
|
||||
const result = zT2IAdapterModel.safeParse({
|
||||
base_model,
|
||||
model_name,
|
||||
});
|
||||
|
||||
if (!result.success) {
|
||||
log.error(
|
||||
{
|
||||
t2iAdapterModelId,
|
||||
errors: result.error.format(),
|
||||
},
|
||||
'Failed to parse T2I-Adapter model id'
|
||||
);
|
||||
|
||||
return;
|
||||
}
|
||||
|
||||
return result.data;
|
||||
};
|
@ -25,6 +25,9 @@ export const useCopyImageToClipboard = () => {
|
||||
try {
|
||||
const getImageBlob = async () => {
|
||||
const response = await fetch(image_url);
|
||||
if (!response.ok) {
|
||||
throw new Error(`Problem retrieving image data`);
|
||||
}
|
||||
return await response.blob();
|
||||
};
|
||||
|
||||
|
@ -70,7 +70,7 @@ export const boardsApi = api.injectEndpoints({
|
||||
keepUnusedDataFor: 0,
|
||||
}),
|
||||
|
||||
getBoardImagesTotal: build.query<number, string | undefined>({
|
||||
getBoardImagesTotal: build.query<{ total: number }, string | undefined>({
|
||||
query: (board_id) => ({
|
||||
url: getListImagesUrl({
|
||||
board_id: board_id ?? 'none',
|
||||
@ -85,11 +85,11 @@ export const boardsApi = api.injectEndpoints({
|
||||
{ type: 'BoardImagesTotal', id: arg ?? 'none' },
|
||||
],
|
||||
transformResponse: (response: OffsetPaginatedResults_ImageDTO_) => {
|
||||
return response.total;
|
||||
return { total: response.total };
|
||||
},
|
||||
}),
|
||||
|
||||
getBoardAssetsTotal: build.query<number, string | undefined>({
|
||||
getBoardAssetsTotal: build.query<{ total: number }, string | undefined>({
|
||||
query: (board_id) => ({
|
||||
url: getListImagesUrl({
|
||||
board_id: board_id ?? 'none',
|
||||
@ -104,7 +104,7 @@ export const boardsApi = api.injectEndpoints({
|
||||
{ type: 'BoardAssetsTotal', id: arg ?? 'none' },
|
||||
],
|
||||
transformResponse: (response: OffsetPaginatedResults_ImageDTO_) => {
|
||||
return response.total;
|
||||
return { total: response.total };
|
||||
},
|
||||
}),
|
||||
|
||||
|
@ -103,6 +103,9 @@ export const imagesApi = api.injectEndpoints({
|
||||
query: () => ({ url: getListImagesUrl({ is_intermediate: true }) }),
|
||||
providesTags: ['IntermediatesCount'],
|
||||
transformResponse: (response: OffsetPaginatedResults_ImageDTO_) => {
|
||||
// TODO: This is storing a primitive value in the cache. `immer` cannot track state changes, so
|
||||
// attempts to use manual cache updates on this value will fail. This should be changed into an
|
||||
// object.
|
||||
return response.total;
|
||||
},
|
||||
}),
|
||||
@ -191,35 +194,51 @@ export const imagesApi = api.injectEndpoints({
|
||||
url: `images/i/${image_name}`,
|
||||
method: 'DELETE',
|
||||
}),
|
||||
invalidatesTags: (result, error, { board_id }) => [
|
||||
{ type: 'BoardImagesTotal', id: board_id ?? 'none' },
|
||||
{ type: 'BoardAssetsTotal', id: board_id ?? 'none' },
|
||||
],
|
||||
async onQueryStarted(imageDTO, { dispatch, queryFulfilled }) {
|
||||
/**
|
||||
* Cache changes for `deleteImage`:
|
||||
* - NOT POSSIBLE: *remove* from getImageDTO
|
||||
* - $cache = [board_id|no_board]/[images|assets]
|
||||
* - *remove* from $cache
|
||||
* - decrement the image's board's total
|
||||
*/
|
||||
|
||||
const { image_name, board_id } = imageDTO;
|
||||
const isAsset = ASSETS_CATEGORIES.includes(imageDTO.image_category);
|
||||
|
||||
const queryArg = {
|
||||
board_id: board_id ?? 'none',
|
||||
categories: getCategories(imageDTO),
|
||||
};
|
||||
|
||||
const patch = dispatch(
|
||||
imagesApi.util.updateQueryData('listImages', queryArg, (draft) => {
|
||||
imagesAdapter.removeOne(draft, image_name);
|
||||
})
|
||||
const patches: PatchCollection[] = [];
|
||||
|
||||
patches.push(
|
||||
dispatch(
|
||||
imagesApi.util.updateQueryData('listImages', queryArg, (draft) => {
|
||||
imagesAdapter.removeOne(draft, image_name);
|
||||
})
|
||||
)
|
||||
);
|
||||
|
||||
patches.push(
|
||||
dispatch(
|
||||
boardsApi.util.updateQueryData(
|
||||
isAsset ? 'getBoardAssetsTotal' : 'getBoardImagesTotal',
|
||||
imageDTO.board_id ?? 'none',
|
||||
(draft) => {
|
||||
draft.total = Math.max(draft.total - 1, 0);
|
||||
}
|
||||
)
|
||||
)
|
||||
); // decrement the image board's total
|
||||
|
||||
try {
|
||||
await queryFulfilled;
|
||||
} catch {
|
||||
patch.undo();
|
||||
patches.forEach((patch) => {
|
||||
patch.undo();
|
||||
});
|
||||
}
|
||||
},
|
||||
}),
|
||||
@ -237,18 +256,11 @@ export const imagesApi = api.injectEndpoints({
|
||||
},
|
||||
};
|
||||
},
|
||||
invalidatesTags: (result, error, { imageDTOs }) => {
|
||||
// for now, assume bulk delete is all on one board
|
||||
const boardId = imageDTOs[0]?.board_id;
|
||||
return [
|
||||
{ type: 'BoardImagesTotal', id: boardId ?? 'none' },
|
||||
{ type: 'BoardAssetsTotal', id: boardId ?? 'none' },
|
||||
];
|
||||
},
|
||||
async onQueryStarted({ imageDTOs }, { dispatch, queryFulfilled }) {
|
||||
/**
|
||||
* Cache changes for `deleteImages`:
|
||||
* - *remove* the deleted images from their boards
|
||||
* - decrement the images' board's totals
|
||||
*
|
||||
* Unfortunately we cannot do an optimistic update here due to how immer handles patching
|
||||
* arrays. You have to undo *all* patches, else the entity adapter's `ids` array is borked.
|
||||
@ -279,6 +291,21 @@ export const imagesApi = api.injectEndpoints({
|
||||
}
|
||||
)
|
||||
);
|
||||
|
||||
const isAsset = ASSETS_CATEGORIES.includes(
|
||||
imageDTO.image_category
|
||||
);
|
||||
|
||||
// decrement the image board's total
|
||||
dispatch(
|
||||
boardsApi.util.updateQueryData(
|
||||
isAsset ? 'getBoardAssetsTotal' : 'getBoardImagesTotal',
|
||||
imageDTO.board_id ?? 'none',
|
||||
(draft) => {
|
||||
draft.total = Math.max(draft.total - 1, 0);
|
||||
}
|
||||
)
|
||||
);
|
||||
}
|
||||
});
|
||||
} catch {
|
||||
@ -298,10 +325,6 @@ export const imagesApi = api.injectEndpoints({
|
||||
method: 'PATCH',
|
||||
body: { is_intermediate },
|
||||
}),
|
||||
invalidatesTags: (result, error, { imageDTO }) => [
|
||||
{ type: 'BoardImagesTotal', id: imageDTO.board_id ?? 'none' },
|
||||
{ type: 'BoardAssetsTotal', id: imageDTO.board_id ?? 'none' },
|
||||
],
|
||||
async onQueryStarted(
|
||||
{ imageDTO, is_intermediate },
|
||||
{ dispatch, queryFulfilled, getState }
|
||||
@ -312,9 +335,11 @@ export const imagesApi = api.injectEndpoints({
|
||||
* - $cache = [board_id|no_board]/[images|assets]
|
||||
* - IF it is being changed to an intermediate:
|
||||
* - remove from $cache
|
||||
* - decrement the image's board's total
|
||||
* - ELSE (it is being changed to a non-intermediate):
|
||||
* - IF it eligible for insertion into existing $cache:
|
||||
* - *upsert* to $cache
|
||||
* - increment the image's board's total
|
||||
*/
|
||||
|
||||
// Store patches so we can undo if the query fails
|
||||
@ -335,6 +360,7 @@ export const imagesApi = api.injectEndpoints({
|
||||
|
||||
// $cache = [board_id|no_board]/[images|assets]
|
||||
const categories = getCategories(imageDTO);
|
||||
const isAsset = ASSETS_CATEGORIES.includes(imageDTO.image_category);
|
||||
|
||||
if (is_intermediate) {
|
||||
// IF it is being changed to an intermediate:
|
||||
@ -350,8 +376,35 @@ export const imagesApi = api.injectEndpoints({
|
||||
)
|
||||
)
|
||||
);
|
||||
|
||||
// decrement the image board's total
|
||||
patches.push(
|
||||
dispatch(
|
||||
boardsApi.util.updateQueryData(
|
||||
isAsset ? 'getBoardAssetsTotal' : 'getBoardImagesTotal',
|
||||
imageDTO.board_id ?? 'none',
|
||||
(draft) => {
|
||||
draft.total = Math.max(draft.total - 1, 0);
|
||||
}
|
||||
)
|
||||
)
|
||||
);
|
||||
} else {
|
||||
// ELSE (it is being changed to a non-intermediate):
|
||||
|
||||
// increment the image board's total
|
||||
patches.push(
|
||||
dispatch(
|
||||
boardsApi.util.updateQueryData(
|
||||
isAsset ? 'getBoardAssetsTotal' : 'getBoardImagesTotal',
|
||||
imageDTO.board_id ?? 'none',
|
||||
(draft) => {
|
||||
draft.total += 1;
|
||||
}
|
||||
)
|
||||
)
|
||||
);
|
||||
|
||||
const queryArgs = {
|
||||
board_id: imageDTO.board_id ?? 'none',
|
||||
categories,
|
||||
@ -361,9 +414,7 @@ export const imagesApi = api.injectEndpoints({
|
||||
getState()
|
||||
);
|
||||
|
||||
const { data: total } = IMAGE_CATEGORIES.includes(
|
||||
imageDTO.image_category
|
||||
)
|
||||
const { data } = IMAGE_CATEGORIES.includes(imageDTO.image_category)
|
||||
? boardsApi.endpoints.getBoardImagesTotal.select(
|
||||
imageDTO.board_id ?? 'none'
|
||||
)(getState())
|
||||
@ -378,7 +429,8 @@ export const imagesApi = api.injectEndpoints({
|
||||
// - The image's `created_at` is within the range of the cached images
|
||||
|
||||
const isCacheFullyPopulated =
|
||||
currentCache.data && currentCache.data.ids.length >= (total ?? 0);
|
||||
currentCache.data &&
|
||||
currentCache.data.ids.length >= (data?.total ?? 0);
|
||||
|
||||
const isInDateRange = getIsImageInDateRange(
|
||||
currentCache.data,
|
||||
@ -420,10 +472,6 @@ export const imagesApi = api.injectEndpoints({
|
||||
method: 'PATCH',
|
||||
body: { session_id },
|
||||
}),
|
||||
invalidatesTags: (result, error, { imageDTO }) => [
|
||||
{ type: 'BoardImagesTotal', id: imageDTO.board_id ?? 'none' },
|
||||
{ type: 'BoardAssetsTotal', id: imageDTO.board_id ?? 'none' },
|
||||
],
|
||||
async onQueryStarted(
|
||||
{ imageDTO, session_id },
|
||||
{ dispatch, queryFulfilled }
|
||||
@ -473,6 +521,7 @@ export const imagesApi = api.injectEndpoints({
|
||||
if (images[0]) {
|
||||
const categories = getCategories(images[0]);
|
||||
const boardId = images[0].board_id;
|
||||
|
||||
return [
|
||||
{
|
||||
type: 'ImageList',
|
||||
@ -481,6 +530,10 @@ export const imagesApi = api.injectEndpoints({
|
||||
categories,
|
||||
}),
|
||||
},
|
||||
{
|
||||
type: 'Board',
|
||||
id: boardId,
|
||||
},
|
||||
];
|
||||
}
|
||||
return [];
|
||||
@ -530,9 +583,7 @@ export const imagesApi = api.injectEndpoints({
|
||||
queryArgs
|
||||
)(getState());
|
||||
|
||||
const { data: previousTotal } = IMAGE_CATEGORIES.includes(
|
||||
imageDTO.image_category
|
||||
)
|
||||
const { data } = IMAGE_CATEGORIES.includes(imageDTO.image_category)
|
||||
? boardsApi.endpoints.getBoardImagesTotal.select(
|
||||
boardId ?? 'none'
|
||||
)(getState())
|
||||
@ -542,10 +593,10 @@ export const imagesApi = api.injectEndpoints({
|
||||
|
||||
const isCacheFullyPopulated =
|
||||
currentCache.data &&
|
||||
currentCache.data.ids.length >= (previousTotal ?? 0);
|
||||
currentCache.data.ids.length >= (data?.total ?? 0);
|
||||
|
||||
const isInDateRange =
|
||||
(previousTotal || 0) >= IMAGE_LIMIT
|
||||
(data?.total ?? 0) >= IMAGE_LIMIT
|
||||
? getIsImageInDateRange(currentCache.data, imageDTO)
|
||||
: true;
|
||||
|
||||
@ -595,6 +646,10 @@ export const imagesApi = api.injectEndpoints({
|
||||
categories,
|
||||
}),
|
||||
},
|
||||
{
|
||||
type: 'Board',
|
||||
id: boardId,
|
||||
},
|
||||
];
|
||||
}
|
||||
return [];
|
||||
@ -643,9 +698,7 @@ export const imagesApi = api.injectEndpoints({
|
||||
queryArgs
|
||||
)(getState());
|
||||
|
||||
const { data: previousTotal } = IMAGE_CATEGORIES.includes(
|
||||
imageDTO.image_category
|
||||
)
|
||||
const { data } = IMAGE_CATEGORIES.includes(imageDTO.image_category)
|
||||
? boardsApi.endpoints.getBoardImagesTotal.select(
|
||||
boardId ?? 'none'
|
||||
)(getState())
|
||||
@ -655,10 +708,10 @@ export const imagesApi = api.injectEndpoints({
|
||||
|
||||
const isCacheFullyPopulated =
|
||||
currentCache.data &&
|
||||
currentCache.data.ids.length >= (previousTotal ?? 0);
|
||||
currentCache.data.ids.length >= (data?.total ?? 0);
|
||||
|
||||
const isInDateRange =
|
||||
(previousTotal || 0) >= IMAGE_LIMIT
|
||||
(data?.total ?? 0) >= IMAGE_LIMIT
|
||||
? getIsImageInDateRange(currentCache.data, imageDTO)
|
||||
: true;
|
||||
|
||||
@ -727,6 +780,7 @@ export const imagesApi = api.injectEndpoints({
|
||||
* - BAIL OUT
|
||||
* - *add* to `getImageDTO`
|
||||
* - *add* to no_board/assets
|
||||
* - update the image's board's assets total
|
||||
*/
|
||||
|
||||
const { data: imageDTO } = await queryFulfilled;
|
||||
@ -761,11 +815,15 @@ export const imagesApi = api.injectEndpoints({
|
||||
)
|
||||
);
|
||||
|
||||
// increment new board's total
|
||||
dispatch(
|
||||
imagesApi.util.invalidateTags([
|
||||
{ type: 'BoardImagesTotal', id: imageDTO.board_id ?? 'none' },
|
||||
{ type: 'BoardAssetsTotal', id: imageDTO.board_id ?? 'none' },
|
||||
])
|
||||
boardsApi.util.updateQueryData(
|
||||
'getBoardAssetsTotal',
|
||||
imageDTO.board_id ?? 'none',
|
||||
(draft) => {
|
||||
draft.total += 1;
|
||||
}
|
||||
)
|
||||
);
|
||||
} catch {
|
||||
// query failed, no action needed
|
||||
@ -792,8 +850,6 @@ export const imagesApi = api.injectEndpoints({
|
||||
categories: ASSETS_CATEGORIES,
|
||||
}),
|
||||
},
|
||||
{ type: 'BoardImagesTotal', id: 'none' },
|
||||
{ type: 'BoardAssetsTotal', id: 'none' },
|
||||
],
|
||||
async onQueryStarted(board_id, { dispatch, queryFulfilled }) {
|
||||
/**
|
||||
@ -806,6 +862,7 @@ export const imagesApi = api.injectEndpoints({
|
||||
* have access to the deleted images DTOs - only the names, and a network request
|
||||
* for all of a board's DTOs could be very large. Instead, we invalidate the 'No Board'
|
||||
* cache.
|
||||
* - set the board's totals to zero
|
||||
*/
|
||||
|
||||
try {
|
||||
@ -825,6 +882,28 @@ export const imagesApi = api.injectEndpoints({
|
||||
);
|
||||
});
|
||||
|
||||
// set the board's asset total to 0 (feels unnecessary since we are deleting it?)
|
||||
dispatch(
|
||||
boardsApi.util.updateQueryData(
|
||||
'getBoardAssetsTotal',
|
||||
board_id,
|
||||
(draft) => {
|
||||
draft.total = 0;
|
||||
}
|
||||
)
|
||||
);
|
||||
|
||||
// set the board's images total to 0 (feels unnecessary since we are deleting it?)
|
||||
dispatch(
|
||||
boardsApi.util.updateQueryData(
|
||||
'getBoardImagesTotal',
|
||||
board_id,
|
||||
(draft) => {
|
||||
draft.total = 0;
|
||||
}
|
||||
)
|
||||
);
|
||||
|
||||
// update 'All Images' & 'All Assets' caches
|
||||
const queryArgsToUpdate = [
|
||||
{
|
||||
@ -881,8 +960,6 @@ export const imagesApi = api.injectEndpoints({
|
||||
categories: ASSETS_CATEGORIES,
|
||||
}),
|
||||
},
|
||||
{ type: 'BoardImagesTotal', id: 'none' },
|
||||
{ type: 'BoardAssetsTotal', id: 'none' },
|
||||
],
|
||||
async onQueryStarted(board_id, { dispatch, queryFulfilled }) {
|
||||
/**
|
||||
@ -892,6 +969,7 @@ export const imagesApi = api.injectEndpoints({
|
||||
* Instead, we rely on the UI to remove all components that use the deleted images.
|
||||
* - Remove every image in the 'All Images' cache that has the board_id
|
||||
* - Remove every image in the 'All Assets' cache that has the board_id
|
||||
* - set the board's totals to zero
|
||||
*/
|
||||
|
||||
try {
|
||||
@ -919,6 +997,28 @@ export const imagesApi = api.injectEndpoints({
|
||||
)
|
||||
);
|
||||
});
|
||||
|
||||
// set the board's asset total to 0 (feels unnecessary since we are deleting it?)
|
||||
dispatch(
|
||||
boardsApi.util.updateQueryData(
|
||||
'getBoardAssetsTotal',
|
||||
board_id,
|
||||
(draft) => {
|
||||
draft.total = 0;
|
||||
}
|
||||
)
|
||||
);
|
||||
|
||||
// set the board's images total to 0 (feels unnecessary since we are deleting it?)
|
||||
dispatch(
|
||||
boardsApi.util.updateQueryData(
|
||||
'getBoardImagesTotal',
|
||||
board_id,
|
||||
(draft) => {
|
||||
draft.total = 0;
|
||||
}
|
||||
)
|
||||
);
|
||||
} catch {
|
||||
//no-op
|
||||
}
|
||||
@ -936,15 +1036,9 @@ export const imagesApi = api.injectEndpoints({
|
||||
body: { board_id, image_name },
|
||||
};
|
||||
},
|
||||
invalidatesTags: (result, error, { board_id, imageDTO }) => [
|
||||
invalidatesTags: (result, error, { board_id }) => [
|
||||
// refresh the board itself
|
||||
{ type: 'Board', id: board_id },
|
||||
// update old board totals
|
||||
{ type: 'BoardImagesTotal', id: board_id },
|
||||
{ type: 'BoardAssetsTotal', id: board_id },
|
||||
// update new board totals
|
||||
{ type: 'BoardImagesTotal', id: imageDTO.board_id ?? 'none' },
|
||||
{ type: 'BoardAssetsTotal', id: imageDTO.board_id ?? 'none' },
|
||||
],
|
||||
async onQueryStarted(
|
||||
{ board_id, imageDTO },
|
||||
@ -961,11 +1055,13 @@ export const imagesApi = api.injectEndpoints({
|
||||
* - $cache = board_id/[images|assets]
|
||||
* - IF it eligible for insertion into existing $cache:
|
||||
* - THEN *add* to $cache
|
||||
* - decrement both old board's total
|
||||
* - increment the new board's total
|
||||
*/
|
||||
|
||||
const patches: PatchCollection[] = [];
|
||||
const categories = getCategories(imageDTO);
|
||||
|
||||
const isAsset = ASSETS_CATEGORIES.includes(imageDTO.image_category);
|
||||
// *update* getImageDTO
|
||||
patches.push(
|
||||
dispatch(
|
||||
@ -996,6 +1092,32 @@ export const imagesApi = api.injectEndpoints({
|
||||
)
|
||||
);
|
||||
|
||||
// decrement old board's total
|
||||
patches.push(
|
||||
dispatch(
|
||||
boardsApi.util.updateQueryData(
|
||||
isAsset ? 'getBoardAssetsTotal' : 'getBoardImagesTotal',
|
||||
imageDTO.board_id ?? 'none',
|
||||
(draft) => {
|
||||
draft.total = Math.max(draft.total - 1, 0);
|
||||
}
|
||||
)
|
||||
)
|
||||
);
|
||||
|
||||
// increment new board's total
|
||||
patches.push(
|
||||
dispatch(
|
||||
boardsApi.util.updateQueryData(
|
||||
isAsset ? 'getBoardAssetsTotal' : 'getBoardImagesTotal',
|
||||
board_id ?? 'none',
|
||||
(draft) => {
|
||||
draft.total += 1;
|
||||
}
|
||||
)
|
||||
)
|
||||
);
|
||||
|
||||
// $cache = board_id/[images|assets]
|
||||
const queryArgs = { board_id: board_id ?? 'none', categories };
|
||||
const currentCache = imagesApi.endpoints.listImages.select(queryArgs)(
|
||||
@ -1008,9 +1130,7 @@ export const imagesApi = api.injectEndpoints({
|
||||
// OR
|
||||
// - The image's `created_at` is within the range of the cached images
|
||||
|
||||
const { data: total } = IMAGE_CATEGORIES.includes(
|
||||
imageDTO.image_category
|
||||
)
|
||||
const { data } = IMAGE_CATEGORIES.includes(imageDTO.image_category)
|
||||
? boardsApi.endpoints.getBoardImagesTotal.select(
|
||||
imageDTO.board_id ?? 'none'
|
||||
)(getState())
|
||||
@ -1019,7 +1139,8 @@ export const imagesApi = api.injectEndpoints({
|
||||
)(getState());
|
||||
|
||||
const isCacheFullyPopulated =
|
||||
currentCache.data && currentCache.data.ids.length >= (total ?? 0);
|
||||
currentCache.data &&
|
||||
currentCache.data.ids.length >= (data?.total ?? 0);
|
||||
|
||||
const isInDateRange = getIsImageInDateRange(
|
||||
currentCache.data,
|
||||
@ -1063,12 +1184,6 @@ export const imagesApi = api.injectEndpoints({
|
||||
return [
|
||||
// invalidate the image's old board
|
||||
{ type: 'Board', id: board_id ?? 'none' },
|
||||
// update old board totals
|
||||
{ type: 'BoardImagesTotal', id: board_id ?? 'none' },
|
||||
{ type: 'BoardAssetsTotal', id: board_id ?? 'none' },
|
||||
// update the no_board totals
|
||||
{ type: 'BoardImagesTotal', id: 'none' },
|
||||
{ type: 'BoardAssetsTotal', id: 'none' },
|
||||
];
|
||||
},
|
||||
async onQueryStarted(
|
||||
@ -1082,10 +1197,13 @@ export const imagesApi = api.injectEndpoints({
|
||||
* - $cache = no_board/[images|assets]
|
||||
* - IF it eligible for insertion into existing $cache:
|
||||
* - THEN *upsert* to $cache
|
||||
* - decrement old board's total
|
||||
* - increment the new board's total (no board)
|
||||
*/
|
||||
|
||||
const categories = getCategories(imageDTO);
|
||||
const patches: PatchCollection[] = [];
|
||||
const isAsset = ASSETS_CATEGORIES.includes(imageDTO.image_category);
|
||||
|
||||
// *update* getImageDTO
|
||||
patches.push(
|
||||
@ -1116,6 +1234,32 @@ export const imagesApi = api.injectEndpoints({
|
||||
)
|
||||
);
|
||||
|
||||
// decrement old board's total
|
||||
patches.push(
|
||||
dispatch(
|
||||
boardsApi.util.updateQueryData(
|
||||
isAsset ? 'getBoardAssetsTotal' : 'getBoardImagesTotal',
|
||||
imageDTO.board_id ?? 'none',
|
||||
(draft) => {
|
||||
draft.total = Math.max(draft.total - 1, 0);
|
||||
}
|
||||
)
|
||||
)
|
||||
);
|
||||
|
||||
// increment new board's total (no board)
|
||||
patches.push(
|
||||
dispatch(
|
||||
boardsApi.util.updateQueryData(
|
||||
isAsset ? 'getBoardAssetsTotal' : 'getBoardImagesTotal',
|
||||
'none',
|
||||
(draft) => {
|
||||
draft.total += 1;
|
||||
}
|
||||
)
|
||||
)
|
||||
);
|
||||
|
||||
// $cache = no_board/[images|assets]
|
||||
const queryArgs = { board_id: 'none', categories };
|
||||
const currentCache = imagesApi.endpoints.listImages.select(queryArgs)(
|
||||
@ -1128,9 +1272,7 @@ export const imagesApi = api.injectEndpoints({
|
||||
// OR
|
||||
// - The image's `created_at` is within the range of the cached images
|
||||
|
||||
const { data: total } = IMAGE_CATEGORIES.includes(
|
||||
imageDTO.image_category
|
||||
)
|
||||
const { data } = IMAGE_CATEGORIES.includes(imageDTO.image_category)
|
||||
? boardsApi.endpoints.getBoardImagesTotal.select(
|
||||
imageDTO.board_id ?? 'none'
|
||||
)(getState())
|
||||
@ -1139,7 +1281,8 @@ export const imagesApi = api.injectEndpoints({
|
||||
)(getState());
|
||||
|
||||
const isCacheFullyPopulated =
|
||||
currentCache.data && currentCache.data.ids.length >= (total ?? 0);
|
||||
currentCache.data &&
|
||||
currentCache.data.ids.length >= (data?.total ?? 0);
|
||||
|
||||
const isInDateRange = getIsImageInDateRange(
|
||||
currentCache.data,
|
||||
@ -1183,21 +1326,10 @@ export const imagesApi = api.injectEndpoints({
|
||||
board_id,
|
||||
},
|
||||
}),
|
||||
invalidatesTags: (result, error, { imageDTOs, board_id }) => {
|
||||
//assume all images are being moved from one board for now
|
||||
const oldBoardId = imageDTOs[0]?.board_id;
|
||||
invalidatesTags: (result, error, { board_id }) => {
|
||||
return [
|
||||
// update the destination board
|
||||
{ type: 'Board', id: board_id ?? 'none' },
|
||||
// update new board totals
|
||||
{ type: 'BoardImagesTotal', id: board_id ?? 'none' },
|
||||
{ type: 'BoardAssetsTotal', id: board_id ?? 'none' },
|
||||
// update old board totals
|
||||
{ type: 'BoardImagesTotal', id: oldBoardId ?? 'none' },
|
||||
{ type: 'BoardAssetsTotal', id: oldBoardId ?? 'none' },
|
||||
// update the no_board totals
|
||||
{ type: 'BoardImagesTotal', id: 'none' },
|
||||
{ type: 'BoardAssetsTotal', id: 'none' },
|
||||
];
|
||||
},
|
||||
async onQueryStarted(
|
||||
@ -1213,6 +1345,8 @@ export const imagesApi = api.injectEndpoints({
|
||||
* - *update* getImageDTO for each image
|
||||
* - *add* to board_id/[images|assets]
|
||||
* - *remove* from [old_board_id|no_board]/[images|assets]
|
||||
* - decrement old board's totals for each image
|
||||
* - increment new board's totals for each image
|
||||
*/
|
||||
|
||||
added_image_names.forEach((image_name) => {
|
||||
@ -1221,7 +1355,8 @@ export const imagesApi = api.injectEndpoints({
|
||||
'getImageDTO',
|
||||
image_name,
|
||||
(draft) => {
|
||||
draft.board_id = new_board_id;
|
||||
draft.board_id =
|
||||
new_board_id === 'none' ? undefined : new_board_id;
|
||||
}
|
||||
)
|
||||
);
|
||||
@ -1234,6 +1369,7 @@ export const imagesApi = api.injectEndpoints({
|
||||
|
||||
const categories = getCategories(imageDTO);
|
||||
const old_board_id = imageDTO.board_id;
|
||||
const isAsset = ASSETS_CATEGORIES.includes(imageDTO.image_category);
|
||||
|
||||
// remove from the old board
|
||||
dispatch(
|
||||
@ -1246,6 +1382,28 @@ export const imagesApi = api.injectEndpoints({
|
||||
)
|
||||
);
|
||||
|
||||
// decrement old board's total
|
||||
dispatch(
|
||||
boardsApi.util.updateQueryData(
|
||||
isAsset ? 'getBoardAssetsTotal' : 'getBoardImagesTotal',
|
||||
old_board_id ?? 'none',
|
||||
(draft) => {
|
||||
draft.total = Math.max(draft.total - 1, 0);
|
||||
}
|
||||
)
|
||||
);
|
||||
|
||||
// increment new board's total
|
||||
dispatch(
|
||||
boardsApi.util.updateQueryData(
|
||||
isAsset ? 'getBoardAssetsTotal' : 'getBoardImagesTotal',
|
||||
new_board_id ?? 'none',
|
||||
(draft) => {
|
||||
draft.total += 1;
|
||||
}
|
||||
)
|
||||
);
|
||||
|
||||
const queryArgs = {
|
||||
board_id: new_board_id,
|
||||
categories,
|
||||
@ -1255,9 +1413,7 @@ export const imagesApi = api.injectEndpoints({
|
||||
queryArgs
|
||||
)(getState());
|
||||
|
||||
const { data: previousTotal } = IMAGE_CATEGORIES.includes(
|
||||
imageDTO.image_category
|
||||
)
|
||||
const { data } = IMAGE_CATEGORIES.includes(imageDTO.image_category)
|
||||
? boardsApi.endpoints.getBoardImagesTotal.select(
|
||||
new_board_id ?? 'none'
|
||||
)(getState())
|
||||
@ -1267,10 +1423,10 @@ export const imagesApi = api.injectEndpoints({
|
||||
|
||||
const isCacheFullyPopulated =
|
||||
currentCache.data &&
|
||||
currentCache.data.ids.length >= (previousTotal ?? 0);
|
||||
currentCache.data.ids.length >= (data?.total ?? 0);
|
||||
|
||||
const isInDateRange =
|
||||
(previousTotal || 0) >= IMAGE_LIMIT
|
||||
(data?.total ?? 0) >= IMAGE_LIMIT
|
||||
? getIsImageInDateRange(currentCache.data, imageDTO)
|
||||
: true;
|
||||
|
||||
@ -1310,10 +1466,7 @@ export const imagesApi = api.injectEndpoints({
|
||||
}),
|
||||
invalidatesTags: (result, error, { imageDTOs }) => {
|
||||
const touchedBoardIds: string[] = [];
|
||||
const tags: ApiTagDescription[] = [
|
||||
{ type: 'BoardImagesTotal', id: 'none' },
|
||||
{ type: 'BoardAssetsTotal', id: 'none' },
|
||||
];
|
||||
const tags: ApiTagDescription[] = [];
|
||||
|
||||
result?.removed_image_names.forEach((image_name) => {
|
||||
const board_id = imageDTOs.find((i) => i.image_name === image_name)
|
||||
@ -1324,8 +1477,6 @@ export const imagesApi = api.injectEndpoints({
|
||||
}
|
||||
|
||||
tags.push({ type: 'Board', id: board_id });
|
||||
tags.push({ type: 'BoardImagesTotal', id: board_id });
|
||||
tags.push({ type: 'BoardAssetsTotal', id: board_id });
|
||||
});
|
||||
|
||||
return tags;
|
||||
@ -1343,6 +1494,8 @@ export const imagesApi = api.injectEndpoints({
|
||||
* - *update* getImageDTO for each image
|
||||
* - *remove* from old_board_id/[images|assets]
|
||||
* - *add* to no_board/[images|assets]
|
||||
* - decrement old board's totals for each image
|
||||
* - increment new board's (no board) totals for each image
|
||||
*/
|
||||
|
||||
removed_image_names.forEach((image_name) => {
|
||||
@ -1363,6 +1516,7 @@ export const imagesApi = api.injectEndpoints({
|
||||
}
|
||||
|
||||
const categories = getCategories(imageDTO);
|
||||
const isAsset = ASSETS_CATEGORIES.includes(imageDTO.image_category);
|
||||
|
||||
// remove from the old board
|
||||
dispatch(
|
||||
@ -1375,6 +1529,28 @@ export const imagesApi = api.injectEndpoints({
|
||||
)
|
||||
);
|
||||
|
||||
// decrement old board's total
|
||||
dispatch(
|
||||
boardsApi.util.updateQueryData(
|
||||
isAsset ? 'getBoardAssetsTotal' : 'getBoardImagesTotal',
|
||||
imageDTO.board_id ?? 'none',
|
||||
(draft) => {
|
||||
draft.total = Math.max(draft.total - 1, 0);
|
||||
}
|
||||
)
|
||||
);
|
||||
|
||||
// increment new board's total (no board)
|
||||
dispatch(
|
||||
boardsApi.util.updateQueryData(
|
||||
isAsset ? 'getBoardAssetsTotal' : 'getBoardImagesTotal',
|
||||
'none',
|
||||
(draft) => {
|
||||
draft.total += 1;
|
||||
}
|
||||
)
|
||||
);
|
||||
|
||||
// add to `no_board`
|
||||
const queryArgs = {
|
||||
board_id: 'none',
|
||||
@ -1385,9 +1561,7 @@ export const imagesApi = api.injectEndpoints({
|
||||
queryArgs
|
||||
)(getState());
|
||||
|
||||
const { data: total } = IMAGE_CATEGORIES.includes(
|
||||
imageDTO.image_category
|
||||
)
|
||||
const { data } = IMAGE_CATEGORIES.includes(imageDTO.image_category)
|
||||
? boardsApi.endpoints.getBoardImagesTotal.select(
|
||||
imageDTO.board_id ?? 'none'
|
||||
)(getState())
|
||||
@ -1396,10 +1570,11 @@ export const imagesApi = api.injectEndpoints({
|
||||
)(getState());
|
||||
|
||||
const isCacheFullyPopulated =
|
||||
currentCache.data && currentCache.data.ids.length >= (total ?? 0);
|
||||
currentCache.data &&
|
||||
currentCache.data.ids.length >= (data?.total ?? 0);
|
||||
|
||||
const isInDateRange =
|
||||
(total || 0) >= IMAGE_LIMIT
|
||||
(data?.total ?? 0) >= IMAGE_LIMIT
|
||||
? getIsImageInDateRange(currentCache.data, imageDTO)
|
||||
: true;
|
||||
|
||||
|
@ -6,6 +6,7 @@ import {
|
||||
CheckpointModelConfig,
|
||||
ControlNetModelConfig,
|
||||
IPAdapterModelConfig,
|
||||
T2IAdapterModelConfig,
|
||||
DiffusersModelConfig,
|
||||
ImportModelConfig,
|
||||
LoRAModelConfig,
|
||||
@ -41,6 +42,10 @@ export type IPAdapterModelConfigEntity = IPAdapterModelConfig & {
|
||||
id: string;
|
||||
};
|
||||
|
||||
export type T2IAdapterModelConfigEntity = T2IAdapterModelConfig & {
|
||||
id: string;
|
||||
};
|
||||
|
||||
export type TextualInversionModelConfigEntity = TextualInversionModelConfig & {
|
||||
id: string;
|
||||
};
|
||||
@ -53,6 +58,7 @@ type AnyModelConfigEntity =
|
||||
| LoRAModelConfigEntity
|
||||
| ControlNetModelConfigEntity
|
||||
| IPAdapterModelConfigEntity
|
||||
| T2IAdapterModelConfigEntity
|
||||
| TextualInversionModelConfigEntity
|
||||
| VaeModelConfigEntity;
|
||||
|
||||
@ -145,6 +151,10 @@ export const ipAdapterModelsAdapter =
|
||||
createEntityAdapter<IPAdapterModelConfigEntity>({
|
||||
sortComparer: (a, b) => a.model_name.localeCompare(b.model_name),
|
||||
});
|
||||
export const t2iAdapterModelsAdapter =
|
||||
createEntityAdapter<T2IAdapterModelConfigEntity>({
|
||||
sortComparer: (a, b) => a.model_name.localeCompare(b.model_name),
|
||||
});
|
||||
export const textualInversionModelsAdapter =
|
||||
createEntityAdapter<TextualInversionModelConfigEntity>({
|
||||
sortComparer: (a, b) => a.model_name.localeCompare(b.model_name),
|
||||
@ -470,6 +480,37 @@ export const modelsApi = api.injectEndpoints({
|
||||
);
|
||||
},
|
||||
}),
|
||||
getT2IAdapterModels: build.query<
|
||||
EntityState<T2IAdapterModelConfigEntity>,
|
||||
void
|
||||
>({
|
||||
query: () => ({ url: 'models/', params: { model_type: 't2i_adapter' } }),
|
||||
providesTags: (result) => {
|
||||
const tags: ApiTagDescription[] = [
|
||||
{ type: 'T2IAdapterModel', id: LIST_TAG },
|
||||
];
|
||||
|
||||
if (result) {
|
||||
tags.push(
|
||||
...result.ids.map((id) => ({
|
||||
type: 'T2IAdapterModel' as const,
|
||||
id,
|
||||
}))
|
||||
);
|
||||
}
|
||||
|
||||
return tags;
|
||||
},
|
||||
transformResponse: (response: { models: T2IAdapterModelConfig[] }) => {
|
||||
const entities = createModelEntities<T2IAdapterModelConfigEntity>(
|
||||
response.models
|
||||
);
|
||||
return t2iAdapterModelsAdapter.setAll(
|
||||
t2iAdapterModelsAdapter.getInitialState(),
|
||||
entities
|
||||
);
|
||||
},
|
||||
}),
|
||||
getVaeModels: build.query<EntityState<VaeModelConfigEntity>, void>({
|
||||
query: () => ({ url: 'models/', params: { model_type: 'vae' } }),
|
||||
providesTags: (result) => {
|
||||
@ -567,6 +608,7 @@ export const {
|
||||
useGetOnnxModelsQuery,
|
||||
useGetControlNetModelsQuery,
|
||||
useGetIPAdapterModelsQuery,
|
||||
useGetT2IAdapterModelsQuery,
|
||||
useGetLoRAModelsQuery,
|
||||
useGetTextualInversionModelsQuery,
|
||||
useGetVaeModelsQuery,
|
||||
|
@ -13,7 +13,7 @@ export const useBoardTotal = (board_id: BoardId) => {
|
||||
const { data: totalAssets } = useGetBoardAssetsTotalQuery(board_id);
|
||||
|
||||
const currentViewTotal = useMemo(
|
||||
() => (galleryView === 'images' ? totalImages : totalAssets),
|
||||
() => (galleryView === 'images' ? totalImages?.total : totalAssets?.total),
|
||||
[galleryView, totalAssets, totalImages]
|
||||
);
|
||||
|
||||
|
594
invokeai/frontend/web/src/services/api/schema.d.ts
vendored
594
invokeai/frontend/web/src/services/api/schema.d.ts
vendored
File diff suppressed because one or more lines are too long
@ -67,6 +67,7 @@ export type VAEModelField = s['VAEModelField'];
|
||||
export type LoRAModelField = s['LoRAModelField'];
|
||||
export type ControlNetModelField = s['ControlNetModelField'];
|
||||
export type IPAdapterModelField = s['IPAdapterModelField'];
|
||||
export type T2IAdapterModelField = s['T2IAdapterModelField'];
|
||||
export type ModelsList = s['ModelsList'];
|
||||
export type ControlField = s['ControlField'];
|
||||
export type IPAdapterField = s['IPAdapterField'];
|
||||
@ -83,6 +84,9 @@ export type ControlNetModelConfig =
|
||||
| ControlNetModelDiffusersConfig;
|
||||
export type IPAdapterModelInvokeAIConfig = s['IPAdapterModelInvokeAIConfig'];
|
||||
export type IPAdapterModelConfig = IPAdapterModelInvokeAIConfig;
|
||||
export type T2IAdapterModelDiffusersConfig =
|
||||
s['T2IAdapterModelDiffusersConfig'];
|
||||
export type T2IAdapterModelConfig = T2IAdapterModelDiffusersConfig;
|
||||
export type TextualInversionModelConfig = s['TextualInversionModelConfig'];
|
||||
export type DiffusersModelConfig =
|
||||
| s['StableDiffusion1ModelDiffusersConfig']
|
||||
@ -99,6 +103,7 @@ export type AnyModelConfig =
|
||||
| VaeModelConfig
|
||||
| ControlNetModelConfig
|
||||
| IPAdapterModelConfig
|
||||
| T2IAdapterModelConfig
|
||||
| TextualInversionModelConfig
|
||||
| MainModelConfig
|
||||
| OnnxModelConfig;
|
||||
|
@ -161,16 +161,16 @@ version = { attr = "invokeai.version.__version__" }
|
||||
[tool.setuptools.packages.find]
|
||||
"where" = ["."]
|
||||
"include" = [
|
||||
"invokeai.assets.web*","invokeai.version*",
|
||||
"invokeai.assets.fonts*","invokeai.version*",
|
||||
"invokeai.generator*","invokeai.backend*",
|
||||
"invokeai.frontend*", "invokeai.frontend.web.dist*",
|
||||
"invokeai.frontend.web.static*",
|
||||
"invokeai.configs*",
|
||||
"invokeai.app*","ldm*",
|
||||
"invokeai.app*",
|
||||
]
|
||||
|
||||
[tool.setuptools.package-data]
|
||||
"invokeai.assets.web" = ["**.png","**.js","**.woff2","**.css"]
|
||||
"invokeai.assets.fonts" = ["**/*.ttf"]
|
||||
"invokeai.backend" = ["**.png"]
|
||||
"invokeai.configs" = ["*.example", "**/*.yaml", "*.txt"]
|
||||
"invokeai.frontend.web.dist" = ["**"]
|
||||
|
0
tests/app/__init__.py
Normal file
0
tests/app/__init__.py
Normal file
0
tests/app/util/__init__.py
Normal file
0
tests/app/util/__init__.py
Normal file
42
tests/app/util/test_controlnet_utils.py
Normal file
42
tests/app/util/test_controlnet_utils.py
Normal file
@ -0,0 +1,42 @@
|
||||
import numpy as np
|
||||
import pytest
|
||||
from PIL import Image
|
||||
|
||||
from invokeai.app.util.controlnet_utils import prepare_control_image
|
||||
|
||||
|
||||
@pytest.mark.parametrize("num_channels", [1, 2, 3])
|
||||
def test_prepare_control_image_num_channels(num_channels):
|
||||
"""Test that the `num_channels` parameter is applied correctly in prepare_control_image(...)."""
|
||||
np_image = np.zeros((256, 256, 3), dtype=np.uint8)
|
||||
pil_image = Image.fromarray(np_image)
|
||||
|
||||
torch_image = prepare_control_image(
|
||||
image=pil_image,
|
||||
width=256,
|
||||
height=256,
|
||||
num_channels=num_channels,
|
||||
device="cpu",
|
||||
do_classifier_free_guidance=False,
|
||||
)
|
||||
|
||||
assert torch_image.shape == (1, num_channels, 256, 256)
|
||||
|
||||
|
||||
@pytest.mark.parametrize("num_channels", [0, 4])
|
||||
def test_prepare_control_image_num_channels_too_large(num_channels):
|
||||
"""Test that an exception is raised in prepare_control_image(...) if the `num_channels` parameter is out of the
|
||||
supported range.
|
||||
"""
|
||||
np_image = np.zeros((256, 256, 3), dtype=np.uint8)
|
||||
pil_image = Image.fromarray(np_image)
|
||||
|
||||
with pytest.raises(ValueError):
|
||||
_ = prepare_control_image(
|
||||
image=pil_image,
|
||||
width=256,
|
||||
height=256,
|
||||
num_channels=num_channels,
|
||||
device="cpu",
|
||||
do_classifier_free_guidance=False,
|
||||
)
|
Loading…
x
Reference in New Issue
Block a user