mirror of
https://github.com/invoke-ai/InvokeAI
synced 2024-08-30 20:32:17 +00:00
Merge branch 'main' into aspect-ratio
This commit is contained in:
commit
3476c58702
189
LICENSE
189
LICENSE
@ -1,21 +1,176 @@
|
||||
MIT License
|
||||
Apache License
|
||||
Version 2.0, January 2004
|
||||
http://www.apache.org/licenses/
|
||||
|
||||
Copyright (c) 2022 InvokeAI Team
|
||||
TERMS AND CONDITIONS FOR USE, REPRODUCTION, AND DISTRIBUTION
|
||||
|
||||
Permission is hereby granted, free of charge, to any person obtaining a copy
|
||||
of this software and associated documentation files (the "Software"), to deal
|
||||
in the Software without restriction, including without limitation the rights
|
||||
to use, copy, modify, merge, publish, distribute, sublicense, and/or sell
|
||||
copies of the Software, and to permit persons to whom the Software is
|
||||
furnished to do so, subject to the following conditions:
|
||||
1. Definitions.
|
||||
|
||||
The above copyright notice and this permission notice shall be included in all
|
||||
copies or substantial portions of the Software.
|
||||
"License" shall mean the terms and conditions for use, reproduction,
|
||||
and distribution as defined by Sections 1 through 9 of this document.
|
||||
|
||||
THE SOFTWARE IS PROVIDED "AS IS", WITHOUT WARRANTY OF ANY KIND, EXPRESS OR
|
||||
IMPLIED, INCLUDING BUT NOT LIMITED TO THE WARRANTIES OF MERCHANTABILITY,
|
||||
FITNESS FOR A PARTICULAR PURPOSE AND NONINFRINGEMENT. IN NO EVENT SHALL THE
|
||||
AUTHORS OR COPYRIGHT HOLDERS BE LIABLE FOR ANY CLAIM, DAMAGES OR OTHER
|
||||
LIABILITY, WHETHER IN AN ACTION OF CONTRACT, TORT OR OTHERWISE, ARISING FROM,
|
||||
OUT OF OR IN CONNECTION WITH THE SOFTWARE OR THE USE OR OTHER DEALINGS IN THE
|
||||
SOFTWARE.
|
||||
"Licensor" shall mean the copyright owner or entity authorized by
|
||||
the copyright owner that is granting the License.
|
||||
|
||||
"Legal Entity" shall mean the union of the acting entity and all
|
||||
other entities that control, are controlled by, or are under common
|
||||
control with that entity. For the purposes of this definition,
|
||||
"control" means (i) the power, direct or indirect, to cause the
|
||||
direction or management of such entity, whether by contract or
|
||||
otherwise, or (ii) ownership of fifty percent (50%) or more of the
|
||||
outstanding shares, or (iii) beneficial ownership of such entity.
|
||||
|
||||
"You" (or "Your") shall mean an individual or Legal Entity
|
||||
exercising permissions granted by this License.
|
||||
|
||||
"Source" form shall mean the preferred form for making modifications,
|
||||
including but not limited to software source code, documentation
|
||||
source, and configuration files.
|
||||
|
||||
"Object" form shall mean any form resulting from mechanical
|
||||
transformation or translation of a Source form, including but
|
||||
not limited to compiled object code, generated documentation,
|
||||
and conversions to other media types.
|
||||
|
||||
"Work" shall mean the work of authorship, whether in Source or
|
||||
Object form, made available under the License, as indicated by a
|
||||
copyright notice that is included in or attached to the work
|
||||
(an example is provided in the Appendix below).
|
||||
|
||||
"Derivative Works" shall mean any work, whether in Source or Object
|
||||
form, that is based on (or derived from) the Work and for which the
|
||||
editorial revisions, annotations, elaborations, or other modifications
|
||||
represent, as a whole, an original work of authorship. For the purposes
|
||||
of this License, Derivative Works shall not include works that remain
|
||||
separable from, or merely link (or bind by name) to the interfaces of,
|
||||
the Work and Derivative Works thereof.
|
||||
|
||||
"Contribution" shall mean any work of authorship, including
|
||||
the original version of the Work and any modifications or additions
|
||||
to that Work or Derivative Works thereof, that is intentionally
|
||||
submitted to Licensor for inclusion in the Work by the copyright owner
|
||||
or by an individual or Legal Entity authorized to submit on behalf of
|
||||
the copyright owner. For the purposes of this definition, "submitted"
|
||||
means any form of electronic, verbal, or written communication sent
|
||||
to the Licensor or its representatives, including but not limited to
|
||||
communication on electronic mailing lists, source code control systems,
|
||||
and issue tracking systems that are managed by, or on behalf of, the
|
||||
Licensor for the purpose of discussing and improving the Work, but
|
||||
excluding communication that is conspicuously marked or otherwise
|
||||
designated in writing by the copyright owner as "Not a Contribution."
|
||||
|
||||
"Contributor" shall mean Licensor and any individual or Legal Entity
|
||||
on behalf of whom a Contribution has been received by Licensor and
|
||||
subsequently incorporated within the Work.
|
||||
|
||||
2. Grant of Copyright License. Subject to the terms and conditions of
|
||||
this License, each Contributor hereby grants to You a perpetual,
|
||||
worldwide, non-exclusive, no-charge, royalty-free, irrevocable
|
||||
copyright license to reproduce, prepare Derivative Works of,
|
||||
publicly display, publicly perform, sublicense, and distribute the
|
||||
Work and such Derivative Works in Source or Object form.
|
||||
|
||||
3. Grant of Patent License. Subject to the terms and conditions of
|
||||
this License, each Contributor hereby grants to You a perpetual,
|
||||
worldwide, non-exclusive, no-charge, royalty-free, irrevocable
|
||||
(except as stated in this section) patent license to make, have made,
|
||||
use, offer to sell, sell, import, and otherwise transfer the Work,
|
||||
where such license applies only to those patent claims licensable
|
||||
by such Contributor that are necessarily infringed by their
|
||||
Contribution(s) alone or by combination of their Contribution(s)
|
||||
with the Work to which such Contribution(s) was submitted. If You
|
||||
institute patent litigation against any entity (including a
|
||||
cross-claim or counterclaim in a lawsuit) alleging that the Work
|
||||
or a Contribution incorporated within the Work constitutes direct
|
||||
or contributory patent infringement, then any patent licenses
|
||||
granted to You under this License for that Work shall terminate
|
||||
as of the date such litigation is filed.
|
||||
|
||||
4. Redistribution. You may reproduce and distribute copies of the
|
||||
Work or Derivative Works thereof in any medium, with or without
|
||||
modifications, and in Source or Object form, provided that You
|
||||
meet the following conditions:
|
||||
|
||||
(a) You must give any other recipients of the Work or
|
||||
Derivative Works a copy of this License; and
|
||||
|
||||
(b) You must cause any modified files to carry prominent notices
|
||||
stating that You changed the files; and
|
||||
|
||||
(c) You must retain, in the Source form of any Derivative Works
|
||||
that You distribute, all copyright, patent, trademark, and
|
||||
attribution notices from the Source form of the Work,
|
||||
excluding those notices that do not pertain to any part of
|
||||
the Derivative Works; and
|
||||
|
||||
(d) If the Work includes a "NOTICE" text file as part of its
|
||||
distribution, then any Derivative Works that You distribute must
|
||||
include a readable copy of the attribution notices contained
|
||||
within such NOTICE file, excluding those notices that do not
|
||||
pertain to any part of the Derivative Works, in at least one
|
||||
of the following places: within a NOTICE text file distributed
|
||||
as part of the Derivative Works; within the Source form or
|
||||
documentation, if provided along with the Derivative Works; or,
|
||||
within a display generated by the Derivative Works, if and
|
||||
wherever such third-party notices normally appear. The contents
|
||||
of the NOTICE file are for informational purposes only and
|
||||
do not modify the License. You may add Your own attribution
|
||||
notices within Derivative Works that You distribute, alongside
|
||||
or as an addendum to the NOTICE text from the Work, provided
|
||||
that such additional attribution notices cannot be construed
|
||||
as modifying the License.
|
||||
|
||||
You may add Your own copyright statement to Your modifications and
|
||||
may provide additional or different license terms and conditions
|
||||
for use, reproduction, or distribution of Your modifications, or
|
||||
for any such Derivative Works as a whole, provided Your use,
|
||||
reproduction, and distribution of the Work otherwise complies with
|
||||
the conditions stated in this License.
|
||||
|
||||
5. Submission of Contributions. Unless You explicitly state otherwise,
|
||||
any Contribution intentionally submitted for inclusion in the Work
|
||||
by You to the Licensor shall be under the terms and conditions of
|
||||
this License, without any additional terms or conditions.
|
||||
Notwithstanding the above, nothing herein shall supersede or modify
|
||||
the terms of any separate license agreement you may have executed
|
||||
with Licensor regarding such Contributions.
|
||||
|
||||
6. Trademarks. This License does not grant permission to use the trade
|
||||
names, trademarks, service marks, or product names of the Licensor,
|
||||
except as required for reasonable and customary use in describing the
|
||||
origin of the Work and reproducing the content of the NOTICE file.
|
||||
|
||||
7. Disclaimer of Warranty. Unless required by applicable law or
|
||||
agreed to in writing, Licensor provides the Work (and each
|
||||
Contributor provides its Contributions) on an "AS IS" BASIS,
|
||||
WITHOUT WARRANTIES OR CONDITIONS OF ANY KIND, either express or
|
||||
implied, including, without limitation, any warranties or conditions
|
||||
of TITLE, NON-INFRINGEMENT, MERCHANTABILITY, or FITNESS FOR A
|
||||
PARTICULAR PURPOSE. You are solely responsible for determining the
|
||||
appropriateness of using or redistributing the Work and assume any
|
||||
risks associated with Your exercise of permissions under this License.
|
||||
|
||||
8. Limitation of Liability. In no event and under no legal theory,
|
||||
whether in tort (including negligence), contract, or otherwise,
|
||||
unless required by applicable law (such as deliberate and grossly
|
||||
negligent acts) or agreed to in writing, shall any Contributor be
|
||||
liable to You for damages, including any direct, indirect, special,
|
||||
incidental, or consequential damages of any character arising as a
|
||||
result of this License or out of the use or inability to use the
|
||||
Work (including but not limited to damages for loss of goodwill,
|
||||
work stoppage, computer failure or malfunction, or any and all
|
||||
other commercial damages or losses), even if such Contributor
|
||||
has been advised of the possibility of such damages.
|
||||
|
||||
9. Accepting Warranty or Additional Liability. While redistributing
|
||||
the Work or Derivative Works thereof, You may choose to offer,
|
||||
and charge a fee for, acceptance of support, warranty, indemnity,
|
||||
or other liability obligations and/or rights consistent with this
|
||||
License. However, in accepting such obligations, You may act only
|
||||
on Your own behalf and on Your sole responsibility, not on behalf
|
||||
of any other Contributor, and only if You agree to indemnify,
|
||||
defend, and hold each Contributor harmless for any liability
|
||||
incurred by, or claims asserted against, such Contributor by reason
|
||||
of your accepting any such warranty or additional liability.
|
||||
|
||||
|
||||
|
20
README.md
20
README.md
@ -3,8 +3,8 @@
|
||||
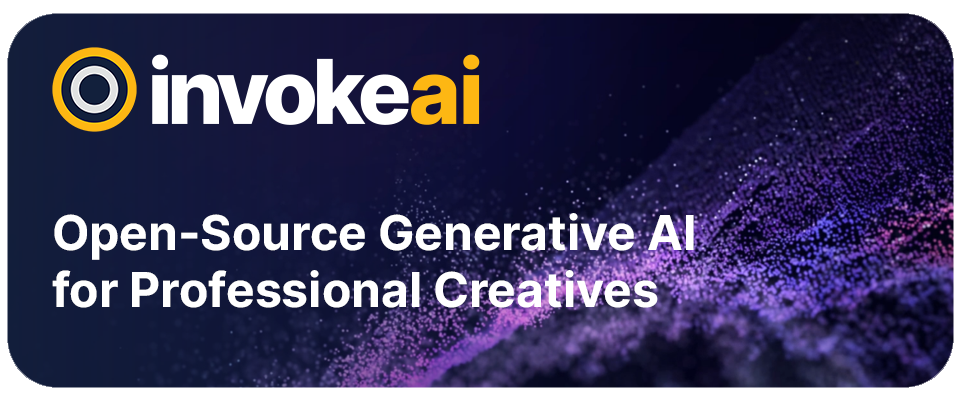
|
||||
|
||||
# Invoke AI - Generative AI for Professional Creatives
|
||||
## Image Generation for Stable Diffusion, Custom-Trained Models, and more.
|
||||
Learn more about us and get started instantly at [invoke.ai](https://invoke.ai)
|
||||
## Professional Creative Tools for Stable Diffusion, Custom-Trained Models, and more.
|
||||
To learn more about Invoke AI, get started instantly, or implement our Business solutions, visit [invoke.ai](https://invoke.ai)
|
||||
|
||||
|
||||
[![discord badge]][discord link]
|
||||
@ -329,24 +329,24 @@ InvokeAI offers a locally hosted Web Server & React Frontend, with an industry l
|
||||
|
||||
The Unified Canvas is a fully integrated canvas implementation with support for all core generation capabilities, in/outpainting, brush tools, and more. This creative tool unlocks the capability for artists to create with AI as a creative collaborator, and can be used to augment AI-generated imagery, sketches, photography, renders, and more.
|
||||
|
||||
### *Advanced Prompt Syntax*
|
||||
### *Node Architecture & Editor (Beta)*
|
||||
|
||||
Invoke AI's advanced prompt syntax allows for token weighting, cross-attention control, and prompt blending, allowing for fine-tuned tweaking of your invocations and exploration of the latent space.
|
||||
Invoke AI's backend is built on a graph-based execution architecture. This allows for customizable generation pipelines to be developed by professional users looking to create specific workflows to support their production use-cases, and will be extended in the future with additional capabilities.
|
||||
|
||||
### *Command Line Interface*
|
||||
### *Board & Gallery Management*
|
||||
|
||||
For users utilizing a terminal-based environment, or who want to take advantage of CLI features, InvokeAI offers an extensive and actively supported command-line interface that provides the full suite of generation functionality available in the tool.
|
||||
Invoke AI provides an organized gallery system for easily storing, accessing, and remixing your content in the Invoke workspace. Images can be dragged/dropped onto any Image-base UI element in the application, and rich metadata within the Image allows for easy recall of key prompts or settings used in your workflow.
|
||||
|
||||
### Other features
|
||||
|
||||
- *Support for both ckpt and diffusers models*
|
||||
- *SD 2.0, 2.1 support*
|
||||
- *Upscaling & Face Restoration Tools*
|
||||
- *Upscaling Tools*
|
||||
- *Embedding Manager & Support*
|
||||
- *Model Manager & Support*
|
||||
- *Node-Based Architecture*
|
||||
- *Node-Based Plug-&-Play UI (Beta)*
|
||||
- *Boards & Gallery Management
|
||||
- *SDXL Support* (Coming soon)
|
||||
|
||||
### Latest Changes
|
||||
|
||||
@ -359,7 +359,7 @@ Notes](https://github.com/invoke-ai/InvokeAI/releases) and the
|
||||
Please check out our **[Q&A](https://invoke-ai.github.io/InvokeAI/help/TROUBLESHOOT/#faq)** to get solutions for common installation
|
||||
problems and other issues.
|
||||
|
||||
## 🤝 Contributing
|
||||
## Contributing
|
||||
|
||||
Anyone who wishes to contribute to this project, whether documentation, features, bug fixes, code
|
||||
cleanup, testing, or code reviews, is very much encouraged to do so.
|
||||
@ -378,7 +378,7 @@ to become part of our community.
|
||||
|
||||
Welcome to InvokeAI!
|
||||
|
||||
### 👥 Contributors
|
||||
### Contributors
|
||||
|
||||
This fork is a combined effort of various people from across the world.
|
||||
[Check out the list of all these amazing people](https://invoke-ai.github.io/InvokeAI/other/CONTRIBUTORS/). We thank them for
|
||||
|
BIN
docs/assets/contributing/resize_invocation.png
Normal file
BIN
docs/assets/contributing/resize_invocation.png
Normal file
Binary file not shown.
After Width: | Height: | Size: 7.1 KiB |
BIN
docs/assets/contributing/resize_node_editor.png
Normal file
BIN
docs/assets/contributing/resize_node_editor.png
Normal file
Binary file not shown.
After Width: | Height: | Size: 17 KiB |
@ -1,8 +1,521 @@
|
||||
# Invocations
|
||||
|
||||
Invocations represent a single operation, its inputs, and its outputs. These
|
||||
operations and their outputs can be chained together to generate and modify
|
||||
images.
|
||||
Features in InvokeAI are added in the form of modular node-like systems called
|
||||
**Invocations**.
|
||||
|
||||
An Invocation is simply a single operation that takes in some inputs and gives
|
||||
out some outputs. We can then chain multiple Invocations together to create more
|
||||
complex functionality.
|
||||
|
||||
## Invocations Directory
|
||||
|
||||
InvokeAI Invocations can be found in the `invokeai/app/invocations` directory.
|
||||
|
||||
You can add your new functionality to one of the existing Invocations in this
|
||||
directory or create a new file in this directory as per your needs.
|
||||
|
||||
**Note:** _All Invocations must be inside this directory for InvokeAI to
|
||||
recognize them as valid Invocations._
|
||||
|
||||
## Creating A New Invocation
|
||||
|
||||
In order to understand the process of creating a new Invocation, let us actually
|
||||
create one.
|
||||
|
||||
In our example, let us create an Invocation that will take in an image, resize
|
||||
it and output the resized image.
|
||||
|
||||
The first set of things we need to do when creating a new Invocation are -
|
||||
|
||||
- Create a new class that derives from a predefined parent class called
|
||||
`BaseInvocation`.
|
||||
- The name of every Invocation must end with the word `Invocation` in order for
|
||||
it to be recognized as an Invocation.
|
||||
- Every Invocation must have a `docstring` that describes what this Invocation
|
||||
does.
|
||||
- Every Invocation must have a unique `type` field defined which becomes its
|
||||
indentifier.
|
||||
- Invocations are strictly typed. We make use of the native
|
||||
[typing](https://docs.python.org/3/library/typing.html) library and the
|
||||
installed [pydantic](https://pydantic-docs.helpmanual.io/) library for
|
||||
validation.
|
||||
|
||||
So let us do that.
|
||||
|
||||
```python
|
||||
from typing import Literal
|
||||
from .baseinvocation import BaseInvocation
|
||||
|
||||
class ResizeInvocation(BaseInvocation):
|
||||
'''Resizes an image'''
|
||||
type: Literal['resize'] = 'resize'
|
||||
```
|
||||
|
||||
That's great.
|
||||
|
||||
Now we have setup the base of our new Invocation. Let us think about what inputs
|
||||
our Invocation takes.
|
||||
|
||||
- We need an `image` that we are going to resize.
|
||||
- We will need new `width` and `height` values to which we need to resize the
|
||||
image to.
|
||||
|
||||
### **Inputs**
|
||||
|
||||
Every Invocation input is a pydantic `Field` and like everything else should be
|
||||
strictly typed and defined.
|
||||
|
||||
So let us create these inputs for our Invocation. First up, the `image` input we
|
||||
need. Generally, we can use standard variable types in Python but InvokeAI
|
||||
already has a custom `ImageField` type that handles all the stuff that is needed
|
||||
for image inputs.
|
||||
|
||||
But what is this `ImageField` ..? It is a special class type specifically
|
||||
written to handle how images are dealt with in InvokeAI. We will cover how to
|
||||
create your own custom field types later in this guide. For now, let's go ahead
|
||||
and use it.
|
||||
|
||||
```python
|
||||
from typing import Literal, Union
|
||||
from pydantic import Field
|
||||
|
||||
from .baseinvocation import BaseInvocation
|
||||
from ..models.image import ImageField
|
||||
|
||||
class ResizeInvocation(BaseInvocation):
|
||||
'''Resizes an image'''
|
||||
type: Literal['resize'] = 'resize'
|
||||
|
||||
# Inputs
|
||||
image: Union[ImageField, None] = Field(description="The input image", default=None)
|
||||
```
|
||||
|
||||
Let us break down our input code.
|
||||
|
||||
```python
|
||||
image: Union[ImageField, None] = Field(description="The input image", default=None)
|
||||
```
|
||||
|
||||
| Part | Value | Description |
|
||||
| --------- | ---------------------------------------------------- | -------------------------------------------------------------------------------------------------- |
|
||||
| Name | `image` | The variable that will hold our image |
|
||||
| Type Hint | `Union[ImageField, None]` | The types for our field. Indicates that the image can either be an `ImageField` type or `None` |
|
||||
| Field | `Field(description="The input image", default=None)` | The image variable is a field which needs a description and a default value that we set to `None`. |
|
||||
|
||||
Great. Now let us create our other inputs for `width` and `height`
|
||||
|
||||
```python
|
||||
from typing import Literal, Union
|
||||
from pydantic import Field
|
||||
|
||||
from .baseinvocation import BaseInvocation
|
||||
from ..models.image import ImageField
|
||||
|
||||
class ResizeInvocation(BaseInvocation):
|
||||
'''Resizes an image'''
|
||||
type: Literal['resize'] = 'resize'
|
||||
|
||||
# Inputs
|
||||
image: Union[ImageField, None] = Field(description="The input image", default=None)
|
||||
width: int = Field(default=512, ge=64, le=2048, description="Width of the new image")
|
||||
height: int = Field(default=512, ge=64, le=2048, description="Height of the new image")
|
||||
```
|
||||
|
||||
As you might have noticed, we added two new parameters to the field type for
|
||||
`width` and `height` called `gt` and `le`. These basically stand for _greater
|
||||
than or equal to_ and _less than or equal to_. There are various other param
|
||||
types for field that you can find on the **pydantic** documentation.
|
||||
|
||||
**Note:** _Any time it is possible to define constraints for our field, we
|
||||
should do it so the frontend has more information on how to parse this field._
|
||||
|
||||
Perfect. We now have our inputs. Let us do something with these.
|
||||
|
||||
### **Invoke Function**
|
||||
|
||||
The `invoke` function is where all the magic happens. This function provides you
|
||||
the `context` parameter that is of the type `InvocationContext` which will give
|
||||
you access to the current context of the generation and all the other services
|
||||
that are provided by it by InvokeAI.
|
||||
|
||||
Let us create this function first.
|
||||
|
||||
```python
|
||||
from typing import Literal, Union
|
||||
from pydantic import Field
|
||||
|
||||
from .baseinvocation import BaseInvocation, InvocationContext
|
||||
from ..models.image import ImageField
|
||||
|
||||
class ResizeInvocation(BaseInvocation):
|
||||
'''Resizes an image'''
|
||||
type: Literal['resize'] = 'resize'
|
||||
|
||||
# Inputs
|
||||
image: Union[ImageField, None] = Field(description="The input image", default=None)
|
||||
width: int = Field(default=512, ge=64, le=2048, description="Width of the new image")
|
||||
height: int = Field(default=512, ge=64, le=2048, description="Height of the new image")
|
||||
|
||||
def invoke(self, context: InvocationContext):
|
||||
pass
|
||||
```
|
||||
|
||||
### **Outputs**
|
||||
|
||||
The output of our Invocation will be whatever is returned by this `invoke`
|
||||
function. Like with our inputs, we need to strongly type and define our outputs
|
||||
too.
|
||||
|
||||
What is our output going to be? Another image. Normally you'd have to create a
|
||||
type for this but InvokeAI already offers you an `ImageOutput` type that handles
|
||||
all the necessary info related to image outputs. So let us use that.
|
||||
|
||||
We will cover how to create your own output types later in this guide.
|
||||
|
||||
```python
|
||||
from typing import Literal, Union
|
||||
from pydantic import Field
|
||||
|
||||
from .baseinvocation import BaseInvocation, InvocationContext
|
||||
from ..models.image import ImageField
|
||||
from .image import ImageOutput
|
||||
|
||||
class ResizeInvocation(BaseInvocation):
|
||||
'''Resizes an image'''
|
||||
type: Literal['resize'] = 'resize'
|
||||
|
||||
# Inputs
|
||||
image: Union[ImageField, None] = Field(description="The input image", default=None)
|
||||
width: int = Field(default=512, ge=64, le=2048, description="Width of the new image")
|
||||
height: int = Field(default=512, ge=64, le=2048, description="Height of the new image")
|
||||
|
||||
def invoke(self, context: InvocationContext) -> ImageOutput:
|
||||
pass
|
||||
```
|
||||
|
||||
Perfect. Now that we have our Invocation setup, let us do what we want to do.
|
||||
|
||||
- We will first load the image. Generally we do this using the `PIL` library but
|
||||
we can use one of the services provided by InvokeAI to load the image.
|
||||
- We will resize the image using `PIL` to our input data.
|
||||
- We will output this image in the format we set above.
|
||||
|
||||
So let's do that.
|
||||
|
||||
```python
|
||||
from typing import Literal, Union
|
||||
from pydantic import Field
|
||||
|
||||
from .baseinvocation import BaseInvocation, InvocationContext
|
||||
from ..models.image import ImageField, ResourceOrigin, ImageCategory
|
||||
from .image import ImageOutput
|
||||
|
||||
class ResizeInvocation(BaseInvocation):
|
||||
'''Resizes an image'''
|
||||
type: Literal['resize'] = 'resize'
|
||||
|
||||
# Inputs
|
||||
image: Union[ImageField, None] = Field(description="The input image", default=None)
|
||||
width: int = Field(default=512, ge=64, le=2048, description="Width of the new image")
|
||||
height: int = Field(default=512, ge=64, le=2048, description="Height of the new image")
|
||||
|
||||
def invoke(self, context: InvocationContext) -> ImageOutput:
|
||||
# Load the image using InvokeAI's predefined Image Service.
|
||||
image = context.services.images.get_pil_image(self.image.image_origin, self.image.image_name)
|
||||
|
||||
# Resizing the image
|
||||
# Because we used the above service, we already have a PIL image. So we can simply resize.
|
||||
resized_image = image.resize((self.width, self.height))
|
||||
|
||||
# Preparing the image for output using InvokeAI's predefined Image Service.
|
||||
output_image = context.services.images.create(
|
||||
image=resized_image,
|
||||
image_origin=ResourceOrigin.INTERNAL,
|
||||
image_category=ImageCategory.GENERAL,
|
||||
node_id=self.id,
|
||||
session_id=context.graph_execution_state_id,
|
||||
is_intermediate=self.is_intermediate,
|
||||
)
|
||||
|
||||
# Returning the Image
|
||||
return ImageOutput(
|
||||
image=ImageField(
|
||||
image_name=output_image.image_name,
|
||||
image_origin=output_image.image_origin,
|
||||
),
|
||||
width=output_image.width,
|
||||
height=output_image.height,
|
||||
)
|
||||
```
|
||||
|
||||
**Note:** Do not be overwhelmed by the `ImageOutput` process. InvokeAI has a
|
||||
certain way that the images need to be dispatched in order to be stored and read
|
||||
correctly. In 99% of the cases when dealing with an image output, you can simply
|
||||
copy-paste the template above.
|
||||
|
||||
That's it. You made your own **Resize Invocation**.
|
||||
|
||||
## Result
|
||||
|
||||
Once you make your Invocation correctly, the rest of the process is fully
|
||||
automated for you.
|
||||
|
||||
When you launch InvokeAI, you can go to `http://localhost:9090/docs` and see
|
||||
your new Invocation show up there with all the relevant info.
|
||||
|
||||

|
||||
|
||||
When you launch the frontend UI, you can go to the Node Editor tab and find your
|
||||
new Invocation ready to be used.
|
||||
|
||||

|
||||
|
||||
# Advanced
|
||||
|
||||
## Custom Input Fields
|
||||
|
||||
Now that you know how to create your own Invocations, let us dive into slightly
|
||||
more advanced topics.
|
||||
|
||||
While creating your own Invocations, you might run into a scenario where the
|
||||
existing input types in InvokeAI do not meet your requirements. In such cases,
|
||||
you can create your own input types.
|
||||
|
||||
Let us create one as an example. Let us say we want to create a color input
|
||||
field that represents a color code. But before we start on that here are some
|
||||
general good practices to keep in mind.
|
||||
|
||||
**Good Practices**
|
||||
|
||||
- There is no naming convention for input fields but we highly recommend that
|
||||
you name it something appropriate like `ColorField`.
|
||||
- It is not mandatory but it is heavily recommended to add a relevant
|
||||
`docstring` to describe your input field.
|
||||
- Keep your field in the same file as the Invocation that it is made for or in
|
||||
another file where it is relevant.
|
||||
|
||||
All input types a class that derive from the `BaseModel` type from `pydantic`.
|
||||
So let's create one.
|
||||
|
||||
```python
|
||||
from pydantic import BaseModel
|
||||
|
||||
class ColorField(BaseModel):
|
||||
'''A field that holds the rgba values of a color'''
|
||||
pass
|
||||
```
|
||||
|
||||
Perfect. Now let us create our custom inputs for our field. This is exactly
|
||||
similar how you created input fields for your Invocation. All the same rules
|
||||
apply. Let us create four fields representing the _red(r)_, _blue(b)_,
|
||||
_green(g)_ and _alpha(a)_ channel of the color.
|
||||
|
||||
```python
|
||||
class ColorField(BaseModel):
|
||||
'''A field that holds the rgba values of a color'''
|
||||
r: int = Field(ge=0, le=255, description="The red channel")
|
||||
g: int = Field(ge=0, le=255, description="The green channel")
|
||||
b: int = Field(ge=0, le=255, description="The blue channel")
|
||||
a: int = Field(ge=0, le=255, description="The alpha channel")
|
||||
```
|
||||
|
||||
That's it. We now have a new input field type that we can use in our Invocations
|
||||
like this.
|
||||
|
||||
```python
|
||||
color: ColorField = Field(default=ColorField(r=0, g=0, b=0, a=0), description='Background color of an image')
|
||||
```
|
||||
|
||||
**Extra Config**
|
||||
|
||||
All input fields also take an additional `Config` class that you can use to do
|
||||
various advanced things like setting required parameters and etc.
|
||||
|
||||
Let us do that for our _ColorField_ and enforce all the values because we did
|
||||
not define any defaults for our fields.
|
||||
|
||||
```python
|
||||
class ColorField(BaseModel):
|
||||
'''A field that holds the rgba values of a color'''
|
||||
r: int = Field(ge=0, le=255, description="The red channel")
|
||||
g: int = Field(ge=0, le=255, description="The green channel")
|
||||
b: int = Field(ge=0, le=255, description="The blue channel")
|
||||
a: int = Field(ge=0, le=255, description="The alpha channel")
|
||||
|
||||
class Config:
|
||||
schema_extra = {"required": ["r", "g", "b", "a"]}
|
||||
```
|
||||
|
||||
Now it becomes mandatory for the user to supply all the values required by our
|
||||
input field.
|
||||
|
||||
We will discuss the `Config` class in extra detail later in this guide and how
|
||||
you can use it to make your Invocations more robust.
|
||||
|
||||
## Custom Output Types
|
||||
|
||||
Like with custom inputs, sometimes you might find yourself needing custom
|
||||
outputs that InvokeAI does not provide. We can easily set one up.
|
||||
|
||||
Now that you are familiar with Invocations and Inputs, let us use that knowledge
|
||||
to put together a custom output type for an Invocation that returns _width_,
|
||||
_height_ and _background_color_ that we need to create a blank image.
|
||||
|
||||
- A custom output type is a class that derives from the parent class of
|
||||
`BaseInvocationOutput`.
|
||||
- It is not mandatory but we recommend using names ending with `Output` for
|
||||
output types. So we'll call our class `BlankImageOutput`
|
||||
- It is not mandatory but we highly recommend adding a `docstring` to describe
|
||||
what your output type is for.
|
||||
- Like Invocations, each output type should have a `type` variable that is
|
||||
**unique**
|
||||
|
||||
Now that we know the basic rules for creating a new output type, let us go ahead
|
||||
and make it.
|
||||
|
||||
```python
|
||||
from typing import Literal
|
||||
from pydantic import Field
|
||||
|
||||
from .baseinvocation import BaseInvocationOutput
|
||||
|
||||
class BlankImageOutput(BaseInvocationOutput):
|
||||
'''Base output type for creating a blank image'''
|
||||
type: Literal['blank_image_output'] = 'blank_image_output'
|
||||
|
||||
# Inputs
|
||||
width: int = Field(description='Width of blank image')
|
||||
height: int = Field(description='Height of blank image')
|
||||
bg_color: ColorField = Field(description='Background color of blank image')
|
||||
|
||||
class Config:
|
||||
schema_extra = {"required": ["type", "width", "height", "bg_color"]}
|
||||
```
|
||||
|
||||
All set. We now have an output type that requires what we need to create a
|
||||
blank_image. And if you noticed it, we even used the `Config` class to ensure
|
||||
the fields are required.
|
||||
|
||||
## Custom Configuration
|
||||
|
||||
As you might have noticed when making inputs and outputs, we used a class called
|
||||
`Config` from _pydantic_ to further customize them. Because our inputs and
|
||||
outputs essentially inherit from _pydantic_'s `BaseModel` class, all
|
||||
[configuration options](https://docs.pydantic.dev/latest/usage/schema/#schema-customization)
|
||||
that are valid for _pydantic_ classes are also valid for our inputs and outputs.
|
||||
You can do the same for your Invocations too but InvokeAI makes our life a
|
||||
little bit easier on that end.
|
||||
|
||||
InvokeAI provides a custom configuration class called `InvocationConfig`
|
||||
particularly for configuring Invocations. This is exactly the same as the raw
|
||||
`Config` class from _pydantic_ with some extra stuff on top to help faciliate
|
||||
parsing of the scheme in the frontend UI.
|
||||
|
||||
At the current moment, tihs `InvocationConfig` class is further improved with
|
||||
the following features related the `ui`.
|
||||
|
||||
| Config Option | Field Type | Example |
|
||||
| ------------- | ------------------------------------------------------------------------------------------------------------- | --------------------------------------------------------------------------------------------------------------------- |
|
||||
| type_hints | `Dict[str, Literal["integer", "float", "boolean", "string", "enum", "image", "latents", "model", "control"]]` | `type_hint: "model"` provides type hints related to the model like displaying a list of available models |
|
||||
| tags | `List[str]` | `tags: ['resize', 'image']` will classify your invocation under the tags of resize and image. |
|
||||
| title | `str` | `title: 'Resize Image` will rename your to this custom title rather than infer from the name of the Invocation class. |
|
||||
|
||||
So let us update your `ResizeInvocation` with some extra configuration and see
|
||||
how that works.
|
||||
|
||||
```python
|
||||
from typing import Literal, Union
|
||||
from pydantic import Field
|
||||
|
||||
from .baseinvocation import BaseInvocation, InvocationContext, InvocationConfig
|
||||
from ..models.image import ImageField, ResourceOrigin, ImageCategory
|
||||
from .image import ImageOutput
|
||||
|
||||
class ResizeInvocation(BaseInvocation):
|
||||
'''Resizes an image'''
|
||||
type: Literal['resize'] = 'resize'
|
||||
|
||||
# Inputs
|
||||
image: Union[ImageField, None] = Field(description="The input image", default=None)
|
||||
width: int = Field(default=512, ge=64, le=2048, description="Width of the new image")
|
||||
height: int = Field(default=512, ge=64, le=2048, description="Height of the new image")
|
||||
|
||||
class Config(InvocationConfig):
|
||||
schema_extra: {
|
||||
ui: {
|
||||
tags: ['resize', 'image'],
|
||||
title: ['My Custom Resize']
|
||||
}
|
||||
}
|
||||
|
||||
def invoke(self, context: InvocationContext) -> ImageOutput:
|
||||
# Load the image using InvokeAI's predefined Image Service.
|
||||
image = context.services.images.get_pil_image(self.image.image_origin, self.image.image_name)
|
||||
|
||||
# Resizing the image
|
||||
# Because we used the above service, we already have a PIL image. So we can simply resize.
|
||||
resized_image = image.resize((self.width, self.height))
|
||||
|
||||
# Preparing the image for output using InvokeAI's predefined Image Service.
|
||||
output_image = context.services.images.create(
|
||||
image=resized_image,
|
||||
image_origin=ResourceOrigin.INTERNAL,
|
||||
image_category=ImageCategory.GENERAL,
|
||||
node_id=self.id,
|
||||
session_id=context.graph_execution_state_id,
|
||||
is_intermediate=self.is_intermediate,
|
||||
)
|
||||
|
||||
# Returning the Image
|
||||
return ImageOutput(
|
||||
image=ImageField(
|
||||
image_name=output_image.image_name,
|
||||
image_origin=output_image.image_origin,
|
||||
),
|
||||
width=output_image.width,
|
||||
height=output_image.height,
|
||||
)
|
||||
```
|
||||
|
||||
We now customized our code to let the frontend know that our Invocation falls
|
||||
under `resize` and `image` categories. So when the user searches for these
|
||||
particular words, our Invocation will show up too.
|
||||
|
||||
We also set a custom title for our Invocation. So instead of being called
|
||||
`Resize`, it will be called `My Custom Resize`.
|
||||
|
||||
As simple as that.
|
||||
|
||||
As time goes by, InvokeAI will further improve and add more customizability for
|
||||
Invocation configuration. We will have more documentation regarding this at a
|
||||
later time.
|
||||
|
||||
# **[TODO]**
|
||||
|
||||
## Custom Components For Frontend
|
||||
|
||||
Every backend input type should have a corresponding frontend component so the
|
||||
UI knows what to render when you use a particular field type.
|
||||
|
||||
If you are using existing field types, we already have components for those. So
|
||||
you don't have to worry about creating anything new. But this might not always
|
||||
be the case. Sometimes you might want to create new field types and have the
|
||||
frontend UI deal with it in a different way.
|
||||
|
||||
This is where we venture into the world of React and Javascript and create our
|
||||
own new components for our Invocations. Do not fear the world of JS. It's
|
||||
actually pretty straightforward.
|
||||
|
||||
Let us create a new component for our custom color field we created above. When
|
||||
we use a color field, let us say we want the UI to display a color picker for
|
||||
the user to pick from rather than entering values. That is what we will build
|
||||
now.
|
||||
|
||||
---
|
||||
|
||||
# OLD -- TO BE DELETED OR MOVED LATER
|
||||
|
||||
---
|
||||
|
||||
## Creating a new invocation
|
||||
|
||||
|
@ -1,9 +1,12 @@
|
||||
---
|
||||
title: Concepts Library
|
||||
title: Concepts
|
||||
---
|
||||
|
||||
# :material-library-shelves: The Hugging Face Concepts Library and Importing Textual Inversion files
|
||||
|
||||
With the advances in research, many new capabilities are available to customize the knowledge and understanding of novel concepts not originally contained in the base model.
|
||||
|
||||
|
||||
## Using Textual Inversion Files
|
||||
|
||||
Textual inversion (TI) files are small models that customize the output of
|
||||
@ -12,18 +15,16 @@ and artistic styles. They are also known as "embeds" in the machine learning
|
||||
world.
|
||||
|
||||
Each TI file introduces one or more vocabulary terms to the SD model. These are
|
||||
known in InvokeAI as "triggers." Triggers are often, but not always, denoted
|
||||
using angle brackets as in "<trigger-phrase>". The two most common type of
|
||||
known in InvokeAI as "triggers." Triggers are denoted using angle brackets
|
||||
as in "<trigger-phrase>". The two most common type of
|
||||
TI files that you'll encounter are `.pt` and `.bin` files, which are produced by
|
||||
different TI training packages. InvokeAI supports both formats, but its
|
||||
[built-in TI training system](TEXTUAL_INVERSION.md) produces `.pt`.
|
||||
[built-in TI training system](TRAINING.md) produces `.pt`.
|
||||
|
||||
The [Hugging Face company](https://huggingface.co/sd-concepts-library) has
|
||||
amassed a large ligrary of >800 community-contributed TI files covering a
|
||||
broad range of subjects and styles. InvokeAI has built-in support for this
|
||||
library which downloads and merges TI files automatically upon request. You can
|
||||
also install your own or others' TI files by placing them in a designated
|
||||
directory.
|
||||
broad range of subjects and styles. You can also install your own or others' TI files
|
||||
by placing them in the designated directory for the compatible model type
|
||||
|
||||
### An Example
|
||||
|
||||
@ -41,66 +42,43 @@ You can also combine styles and concepts:
|
||||
| :--------------------------------------------------------: |
|
||||
|  |
|
||||
</figure>
|
||||
## Using a Hugging Face Concept
|
||||
|
||||
!!! warning "Authenticating to HuggingFace"
|
||||
|
||||
Some concepts require valid authentication to HuggingFace. Without it, they will not be downloaded
|
||||
and will be silently ignored.
|
||||
|
||||
If you used an installer to install InvokeAI, you may have already set a HuggingFace token.
|
||||
If you skipped this step, you can:
|
||||
|
||||
- run the InvokeAI configuration script again (if you used a manual installer): `invokeai-configure`
|
||||
- set one of the `HUGGINGFACE_TOKEN` or `HUGGING_FACE_HUB_TOKEN` environment variables to contain your token
|
||||
|
||||
Finally, if you already used any HuggingFace library on your computer, you might already have a token
|
||||
in your local cache. Check for a hidden `.huggingface` directory in your home folder. If it
|
||||
contains a `token` file, then you are all set.
|
||||
|
||||
|
||||
Hugging Face TI concepts are downloaded and installed automatically as you
|
||||
require them. This requires your machine to be connected to the Internet. To
|
||||
find out what each concept is for, you can browse the
|
||||
[Hugging Face concepts library](https://huggingface.co/sd-concepts-library) and
|
||||
look at examples of what each concept produces.
|
||||
|
||||
To load concepts, you will need to open the Web UI's configuration
|
||||
dialogue and activate "Show Textual Inversions from HF Concepts
|
||||
Library". This will then add a list of HF Concepts to the dropdown
|
||||
"Add Textual Inversion" menu. Select the concept(s) of your choice and
|
||||
they will be incorporated into the positive prompt. A few concepts are
|
||||
designed for the negative prompt, in which case you can add them to
|
||||
the negative prompt box by select the down arrow icon next to the
|
||||
textual inversion menu.
|
||||
|
||||
There are nearly 1000 HF concepts, more than will fit into a menu. For
|
||||
this reason we only show the most popular concepts (those which have
|
||||
received 5 or more likes). If you wish to use a concept that is not on
|
||||
the list, you may simply type its name surrounded by brackets. For
|
||||
example, to load the concept named "xidiversity", add `<xidiversity>`
|
||||
to the positive or negative prompt text.
|
||||
|
||||
## Installing your Own TI Files
|
||||
|
||||
You may install any number of `.pt` and `.bin` files simply by copying them into
|
||||
the `embeddings` directory of the InvokeAI runtime directory (usually `invokeai`
|
||||
in your home directory). You may create subdirectories in order to organize the
|
||||
files in any way you wish. Be careful not to overwrite one file with another.
|
||||
the `embedding` directory of the corresponding InvokeAI models directory (usually `invokeai`
|
||||
in your home directory). For example, you can simply move a Stable Diffusion 1.5 embedding file to
|
||||
the `sd-1/embedding` folder. Be careful not to overwrite one file with another.
|
||||
For example, TI files generated by the Hugging Face toolkit share the named
|
||||
`learned_embedding.bin`. You can use subdirectories to keep them distinct.
|
||||
`learned_embedding.bin`. You can rename these, or use subdirectories to keep them distinct.
|
||||
|
||||
At startup time, InvokeAI will scan the `embeddings` directory and load any TI
|
||||
files it finds there. At startup you will see a message similar to this one:
|
||||
At startup time, InvokeAI will scan the various `embedding` directories and load any TI
|
||||
files it finds there for compatible models. At startup you will see a message similar to this one:
|
||||
|
||||
```bash
|
||||
>> Current embedding manager terms: <HOI4-Leader>, <princess-knight>
|
||||
```
|
||||
To use these when generating, simply type the `<` key in your prompt to open the Textual Inversion WebUI and
|
||||
select the embedding you'd like to use. This UI has type-ahead support, so you can easily find supported embeddings.
|
||||
|
||||
The terms you can use will appear in the "Add Textual Inversion"
|
||||
dropdown menu above the HF Concepts.
|
||||
## Using LoRAs
|
||||
|
||||
## Further Reading
|
||||
LoRA files are models that customize the output of Stable Diffusion image generation.
|
||||
Larger than embeddings, but much smaller than full models, they augment SD with improved
|
||||
understanding of subjects and artistic styles.
|
||||
|
||||
Unlike TI files, LoRAs do not introduce novel vocabulary into the model's known tokens. Instead,
|
||||
LoRAs augment the model's weights that are applied to generate imagery. LoRAs may be supplied
|
||||
with a "trigger" word that they have been explicitly trained on, or may simply apply their
|
||||
effect without being triggered.
|
||||
|
||||
LoRAs are typically stored in .safetensors files, which are the most secure way to store and transmit
|
||||
these types of weights. You may install any number of `.safetensors` LoRA files simply by copying them into
|
||||
the `lora` directory of the corresponding InvokeAI models directory (usually `invokeai`
|
||||
in your home directory). For example, you can simply move a Stable Diffusion 1.5 LoRA file to
|
||||
the `sd-1/lora` folder.
|
||||
|
||||
To use these when generating, open the LoRA menu item in the options panel, select the LoRAs you want to apply
|
||||
and ensure that they have the appropriate weight recommended by the model provider. Typically, most LoRAs perform best at a weight of .75-1.
|
||||
|
||||
Please see [the repository](https://github.com/rinongal/textual_inversion) and
|
||||
associated paper for details and limitations.
|
||||
|
@ -301,5 +301,48 @@ summoning up the concept of some sort of scifi creature? Let's find out.
|
||||
Indeed, removing the word "hybrid" produces an image that is more like what we'd
|
||||
expect.
|
||||
|
||||
In conclusion, prompt blending is great for exploring creative space,
|
||||
but takes some trial and error to achieve the desired effect.
|
||||
## Dynamic Prompts
|
||||
|
||||
Dynamic Prompts are a powerful feature designed to produce a variety of prompts based on user-defined options. Using a special syntax, you can construct a prompt with multiple possibilities, and the system will automatically generate a series of permutations based on your settings. This is extremely beneficial for ideation, exploring various scenarios, or testing different concepts swiftly and efficiently.
|
||||
|
||||
### Structure of a Dynamic Prompt
|
||||
|
||||
A Dynamic Prompt comprises of regular text, supplemented with alternatives enclosed within curly braces {} and separated by a vertical bar |. For example: {option1|option2|option3}. The system will then select one of the options to include in the final prompt. This flexible system allows for options to be placed throughout the text as needed.
|
||||
|
||||
Furthermore, Dynamic Prompts can designate multiple selections from a single group of options. This feature is triggered by prefixing the options with a numerical value followed by $$. For example, in {2$$option1|option2|option3}, the system will select two distinct options from the set.
|
||||
### Creating Dynamic Prompts
|
||||
|
||||
To create a Dynamic Prompt, follow these steps:
|
||||
|
||||
Draft your sentence or phrase, identifying words or phrases with multiple possible options.
|
||||
Encapsulate the different options within curly braces {}.
|
||||
Within the braces, separate each option using a vertical bar |.
|
||||
If you want to include multiple options from a single group, prefix with the desired number and $$.
|
||||
|
||||
For instance: A {house|apartment|lodge|cottage} in {summer|winter|autumn|spring} designed in {2$$style1|style2|style3}.
|
||||
### How Dynamic Prompts Work
|
||||
|
||||
Once a Dynamic Prompt is configured, the system generates an array of combinations using the options provided. Each group of options in curly braces is treated independently, with the system selecting one option from each group. For a prefixed set (e.g., 2$$), the system will select two distinct options.
|
||||
|
||||
For example, the following prompts could be generated from the above Dynamic Prompt:
|
||||
|
||||
A house in summer designed in style1, style2
|
||||
A lodge in autumn designed in style3, style1
|
||||
A cottage in winter designed in style2, style3
|
||||
And many more!
|
||||
|
||||
When the `Combinatorial` setting is on, Invoke will disable the "Images" selection, and generate every combination up until the setting for Max Prompts is reached.
|
||||
When the `Combinatorial` setting is off, Invoke will randomly generate combinations up until the setting for Images has been reached.
|
||||
|
||||
|
||||
|
||||
### Tips and Tricks for Using Dynamic Prompts
|
||||
|
||||
Below are some useful strategies for creating Dynamic Prompts:
|
||||
|
||||
Utilize Dynamic Prompts to generate a wide spectrum of prompts, perfect for brainstorming and exploring diverse ideas.
|
||||
Ensure that the options within a group are contextually relevant to the part of the sentence where they are used. For instance, group building types together, and seasons together.
|
||||
Apply the 2$$ prefix when you want to incorporate more than one option from a single group. This becomes quite handy when mixing and matching different elements.
|
||||
Experiment with different quantities for the prefix. For example, 3$$ will select three distinct options.
|
||||
Be aware of coherence in your prompts. Although the system can generate all possible combinations, not all may semantically make sense. Therefore, carefully choose the options for each group.
|
||||
Always review and fine-tune the generated prompts as needed. While Dynamic Prompts can help you generate a multitude of combinations, the final polishing and refining remain in your hands.
|
||||
|
@ -1,9 +1,10 @@
|
||||
---
|
||||
title: Textual-Inversion
|
||||
title: Training
|
||||
---
|
||||
|
||||
# :material-file-document: Textual Inversion
|
||||
# :material-file-document: Training
|
||||
|
||||
# Textual Inversion Training
|
||||
## **Personalizing Text-to-Image Generation**
|
||||
|
||||
You may personalize the generated images to provide your own styles or objects
|
||||
@ -258,16 +259,6 @@ invokeai-ti \
|
||||
--only_save_embeds
|
||||
```
|
||||
|
||||
## Using Embeddings
|
||||
|
||||
After training completes, the resultant embeddings will be saved into your `$INVOKEAI_ROOT/embeddings/<trigger word>/learned_embeds.bin`.
|
||||
|
||||
These will be automatically loaded when you start InvokeAI.
|
||||
|
||||
Add the trigger word, surrounded by angle brackets, to use that embedding. For example, if your trigger word was `terence`, use `<terence>` in prompts. This is the same syntax used by the HuggingFace concepts library.
|
||||
|
||||
**Note:** `.pt` embeddings do not require the angle brackets.
|
||||
|
||||
## Troubleshooting
|
||||
|
||||
### `Cannot load embedding for <trigger>. It was trained on a model with token dimension 1024, but the current model has token dimension 768`
|
@ -1,22 +1,16 @@
|
||||
import sqlite3
|
||||
import threading
|
||||
from abc import ABC, abstractmethod
|
||||
from datetime import datetime
|
||||
from typing import Generic, Optional, TypeVar, cast
|
||||
import sqlite3
|
||||
import threading
|
||||
|
||||
from pydantic import BaseModel, Field
|
||||
from pydantic.generics import GenericModel
|
||||
|
||||
from invokeai.app.models.image import ImageCategory, ResourceOrigin
|
||||
from invokeai.app.models.metadata import ImageMetadata
|
||||
from invokeai.app.models.image import (
|
||||
ImageCategory,
|
||||
ResourceOrigin,
|
||||
)
|
||||
from invokeai.app.services.models.image_record import (
|
||||
ImageRecord,
|
||||
ImageRecordChanges,
|
||||
deserialize_image_record,
|
||||
)
|
||||
ImageRecord, ImageRecordChanges, deserialize_image_record)
|
||||
|
||||
T = TypeVar("T", bound=BaseModel)
|
||||
|
||||
@ -162,7 +156,6 @@ class SqliteImageRecordStorage(ImageRecordStorageBase):
|
||||
node_id TEXT,
|
||||
metadata TEXT,
|
||||
is_intermediate BOOLEAN DEFAULT FALSE,
|
||||
board_id TEXT,
|
||||
created_at DATETIME NOT NULL DEFAULT(STRFTIME('%Y-%m-%d %H:%M:%f', 'NOW')),
|
||||
-- Updated via trigger
|
||||
updated_at DATETIME NOT NULL DEFAULT(STRFTIME('%Y-%m-%d %H:%M:%f', 'NOW')),
|
||||
|
@ -1,49 +1,32 @@
|
||||
import { MenuItem, MenuList } from '@chakra-ui/react';
|
||||
import { useAppDispatch, useAppSelector } from 'app/store/storeHooks';
|
||||
import { memo, useCallback, useContext } from 'react';
|
||||
import {
|
||||
FaExpand,
|
||||
FaFolder,
|
||||
FaFolderPlus,
|
||||
FaShare,
|
||||
FaTrash,
|
||||
} from 'react-icons/fa';
|
||||
import { ContextMenu, ContextMenuProps } from 'chakra-ui-contextmenu';
|
||||
import {
|
||||
resizeAndScaleCanvas,
|
||||
setInitialCanvasImage,
|
||||
} from 'features/canvas/store/canvasSlice';
|
||||
import { setActiveTab } from 'features/ui/store/uiSlice';
|
||||
import { useTranslation } from 'react-i18next';
|
||||
import { ExternalLinkIcon } from '@chakra-ui/icons';
|
||||
import { IoArrowUndoCircleOutline } from 'react-icons/io5';
|
||||
import { MenuItem, MenuList } from '@chakra-ui/react';
|
||||
import { createSelector } from '@reduxjs/toolkit';
|
||||
import { useFeatureStatus } from 'features/system/hooks/useFeatureStatus';
|
||||
import { useRecallParameters } from 'features/parameters/hooks/useRecallParameters';
|
||||
import { initialImageSelected } from 'features/parameters/store/actions';
|
||||
import { sentImageToCanvas, sentImageToImg2Img } from '../store/actions';
|
||||
import { useAppToaster } from 'app/components/Toaster';
|
||||
import { AddImageToBoardContext } from '../../../app/contexts/AddImageToBoardContext';
|
||||
import { useRemoveImageFromBoardMutation } from 'services/api/endpoints/boardImages';
|
||||
import { ImageDTO } from 'services/api/types';
|
||||
import { RootState, stateSelector } from 'app/store/store';
|
||||
import { stateSelector } from 'app/store/store';
|
||||
import { useAppDispatch, useAppSelector } from 'app/store/storeHooks';
|
||||
import { defaultSelectorOptions } from 'app/store/util/defaultMemoizeOptions';
|
||||
import { ContextMenu, ContextMenuProps } from 'chakra-ui-contextmenu';
|
||||
import {
|
||||
imagesAddedToBatch,
|
||||
selectionAddedToBatch,
|
||||
} from 'features/batch/store/batchSlice';
|
||||
import { defaultSelectorOptions } from 'app/store/util/defaultMemoizeOptions';
|
||||
import {
|
||||
resizeAndScaleCanvas,
|
||||
setInitialCanvasImage,
|
||||
} from 'features/canvas/store/canvasSlice';
|
||||
import { imageToDeleteSelected } from 'features/imageDeletion/store/imageDeletionSlice';
|
||||
|
||||
const selector = createSelector(
|
||||
[stateSelector, (state: RootState, imageDTO: ImageDTO) => imageDTO],
|
||||
({ gallery, batch }, imageDTO) => {
|
||||
const selectionCount = gallery.selection.length;
|
||||
const isInBatch = batch.imageNames.includes(imageDTO.image_name);
|
||||
|
||||
return { selectionCount, isInBatch };
|
||||
},
|
||||
defaultSelectorOptions
|
||||
);
|
||||
import { useRecallParameters } from 'features/parameters/hooks/useRecallParameters';
|
||||
import { initialImageSelected } from 'features/parameters/store/actions';
|
||||
import { useFeatureStatus } from 'features/system/hooks/useFeatureStatus';
|
||||
import { setActiveTab } from 'features/ui/store/uiSlice';
|
||||
import { memo, useCallback, useContext, useMemo } from 'react';
|
||||
import { useTranslation } from 'react-i18next';
|
||||
import { FaExpand, FaFolder, FaShare, FaTrash } from 'react-icons/fa';
|
||||
import { IoArrowUndoCircleOutline } from 'react-icons/io5';
|
||||
import { useRemoveImageFromBoardMutation } from 'services/api/endpoints/boardImages';
|
||||
import { ImageDTO } from 'services/api/types';
|
||||
import { AddImageToBoardContext } from '../../../app/contexts/AddImageToBoardContext';
|
||||
import { sentImageToCanvas, sentImageToImg2Img } from '../store/actions';
|
||||
|
||||
type Props = {
|
||||
image: ImageDTO;
|
||||
@ -51,9 +34,21 @@ type Props = {
|
||||
};
|
||||
|
||||
const ImageContextMenu = ({ image, children }: Props) => {
|
||||
const { selectionCount, isInBatch } = useAppSelector((state) =>
|
||||
selector(state, image)
|
||||
const selector = useMemo(
|
||||
() =>
|
||||
createSelector(
|
||||
[stateSelector],
|
||||
({ gallery, batch }) => {
|
||||
const selectionCount = gallery.selection.length;
|
||||
const isInBatch = batch.imageNames.includes(image.image_name);
|
||||
|
||||
return { selectionCount, isInBatch };
|
||||
},
|
||||
defaultSelectorOptions
|
||||
),
|
||||
[image.image_name]
|
||||
);
|
||||
const { selectionCount, isInBatch } = useAppSelector(selector);
|
||||
const dispatch = useAppDispatch();
|
||||
const { t } = useTranslation();
|
||||
|
||||
|
@ -8,7 +8,7 @@ import {
|
||||
selectImagesById,
|
||||
} from 'features/gallery/store/gallerySlice';
|
||||
import { clamp, isEqual } from 'lodash-es';
|
||||
import { useCallback, useState } from 'react';
|
||||
import { memo, useCallback, useState } from 'react';
|
||||
import { useHotkeys } from 'react-hotkeys-hook';
|
||||
import { useTranslation } from 'react-i18next';
|
||||
import { FaAngleDoubleRight, FaAngleLeft, FaAngleRight } from 'react-icons/fa';
|
||||
@ -227,4 +227,4 @@ const NextPrevImageButtons = () => {
|
||||
);
|
||||
};
|
||||
|
||||
export default NextPrevImageButtons;
|
||||
export default memo(NextPrevImageButtons);
|
||||
|
@ -78,7 +78,7 @@ export default function InvokeButton(props: InvokeButton) {
|
||||
aria-label={t('parameters.invoke')}
|
||||
type="submit"
|
||||
icon={<FaPlay />}
|
||||
isDisabled={!isReady}
|
||||
isDisabled={!isReady || isProcessing}
|
||||
onClick={handleInvoke}
|
||||
tooltip={t('parameters.invoke')}
|
||||
tooltipProps={{ placement: 'top' }}
|
||||
@ -95,7 +95,7 @@ export default function InvokeButton(props: InvokeButton) {
|
||||
<IAIButton
|
||||
aria-label={t('parameters.invoke')}
|
||||
type="submit"
|
||||
isDisabled={!isReady}
|
||||
isDisabled={!isReady || isProcessing}
|
||||
onClick={handleInvoke}
|
||||
colorScheme="accent"
|
||||
id="invoke-button"
|
||||
|
Loading…
x
Reference in New Issue
Block a user