mirror of
https://github.com/invoke-ai/InvokeAI
synced 2024-08-30 20:32:17 +00:00
feat: Add IP Adapter to InvokeAI (Node & Linear) (#4429)
## What type of PR is this? (check all applicable) - [ ] Refactor - [x] Feature - [ ] Bug Fix - [ ] Optimization - [ ] Documentation Update - [ ] Community Node Submission ## Have you discussed this change with the InvokeAI team? - [x] Yes - [ ] No, because: ## Have you updated all relevant documentation? - [ ] Yes - [ ] No ## Description (edit by @blessedcoolant , @RyanJDick ) This PR adds support for IP-Adapters (a technique for image-based prompts) in Invoke AI. Currently only available in the Node UI. IP-Adapter Paper: [IP-Adapter: Text Compatible Image Prompt Adapter for Text-to-Image Diffusion Models](https://arxiv.org/abs/2308.06721) IP-Adapter reference code: https://github.com/tencent-ailab/IP-Adapter On order to test, install the following models via the InvokeAI UI: Image Encoders: [InvokeAI/ip_adapter_sd_image_encoder](https://huggingface.co/InvokeAI/ip_adapter_sd_image_encoder) [InvokeAI/ip_adapter_sdxl_image_encoder](https://huggingface.co/InvokeAI/ip_adapter_sdxl_image_encoder) IP-Adapters: [InvokeAI/ip_adapter_sd15](https://huggingface.co/InvokeAI/ip_adapter_sd15) [InvokeAI/ip_adapter_plus_sd15](https://huggingface.co/InvokeAI/ip_adapter_plus_sd15) [InvokeAI/ip_adapter_plus_face_sd15](https://huggingface.co/InvokeAI/ip_adapter_plus_face_sd15) [InvokeAI/ip_adapter_sdxl](https://huggingface.co/InvokeAI/ip_adapter_sdxl) Old instructions (for reference only): > In order to test, you need to download and place the following models in your InvokeAI models directory. > > - SD 1.5 - https://huggingface.co/h94/IP-Adapter/tree/main/models --> Download the models and the `image_encoder` folder to `models/core/ip_adapters/sd-1` > - SDXL - https://huggingface.co/h94/IP-Adapter/tree/main/sdxl_models -Download the models and the `image_encoder` folder to `models/core/ip_adapaters/sdxl` > > This is only temporary. This needs to be handled differently. I outlined them here. https://github.com/invoke-ai/InvokeAI/pull/4429#issuecomment-1705776570 ## Examples using this PR ### Image variations, no text prompt Leftmost image in each row is original image used for input to IP-Adapter. The other rows are example outputs with different seeds, other parameters identical. 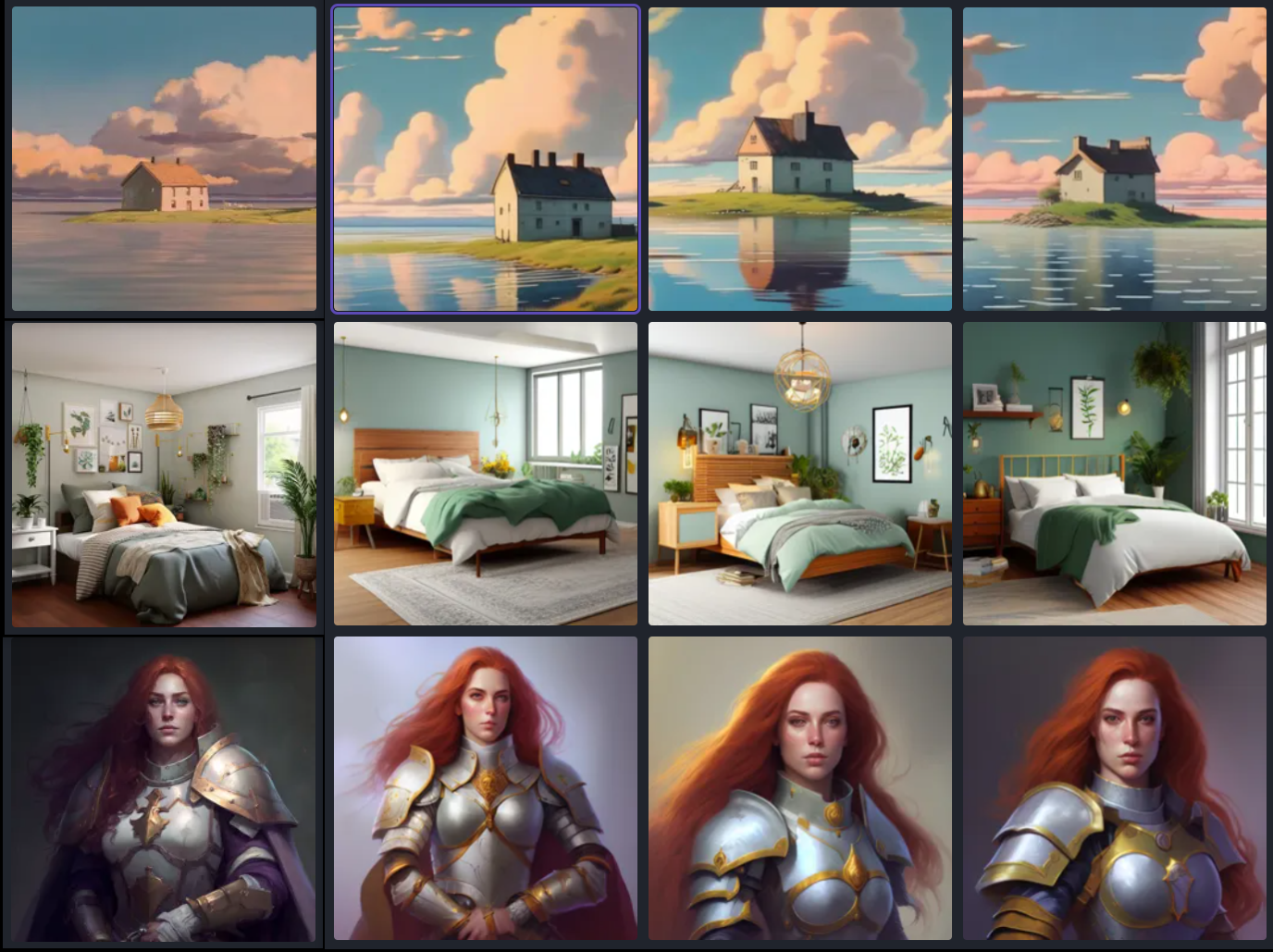 ## Related Tickets & Documents <!-- For pull requests that relate or close an issue, please include them below. For example having the text: "closes #1234" would connect the current pull request to issue 1234. And when we merge the pull request, Github will automatically close the issue. --> - Related Issue # - Closes # ## QA Instructions, Screenshots, Recordings <!-- Please provide steps on how to test changes, any hardware or software specifications as well as any other pertinent information. --> ## Added/updated tests? - [ ] Yes - [ ] No : _please replace this line with details on why tests have not been included_ ## [optional] Are there any post deployment tasks we need to perform?
This commit is contained in:
commit
864f2270c3
@ -67,6 +67,7 @@ class FieldDescriptions:
|
||||
width = "Width of output (px)"
|
||||
height = "Height of output (px)"
|
||||
control = "ControlNet(s) to apply"
|
||||
ip_adapter = "IP-Adapter to apply"
|
||||
denoised_latents = "Denoised latents tensor"
|
||||
latents = "Latents tensor"
|
||||
strength = "Strength of denoising (proportional to steps)"
|
||||
@ -155,6 +156,7 @@ class UIType(str, Enum):
|
||||
VaeModel = "VaeModelField"
|
||||
LoRAModel = "LoRAModelField"
|
||||
ControlNetModel = "ControlNetModelField"
|
||||
IPAdapterModel = "IPAdapterModelField"
|
||||
UNet = "UNetField"
|
||||
Vae = "VaeField"
|
||||
CLIP = "ClipField"
|
||||
|
@ -7,14 +7,14 @@ from compel import Compel, ReturnedEmbeddingsType
|
||||
from compel.prompt_parser import Blend, Conjunction, CrossAttentionControlSubstitute, FlattenedPrompt, Fragment
|
||||
|
||||
from invokeai.app.invocations.primitives import ConditioningField, ConditioningOutput
|
||||
from invokeai.backend.stable_diffusion.diffusion.shared_invokeai_diffusion import (
|
||||
from invokeai.backend.stable_diffusion.diffusion.conditioning_data import (
|
||||
BasicConditioningInfo,
|
||||
ExtraConditioningInfo,
|
||||
SDXLConditioningInfo,
|
||||
)
|
||||
|
||||
from ...backend.model_management.lora import ModelPatcher
|
||||
from ...backend.model_management.models import ModelNotFoundException, ModelType
|
||||
from ...backend.stable_diffusion.diffusion import InvokeAIDiffuserComponent
|
||||
from ...backend.util.devices import torch_dtype
|
||||
from .baseinvocation import (
|
||||
BaseInvocation,
|
||||
@ -99,14 +99,15 @@ class CompelInvocation(BaseInvocation):
|
||||
# print(traceback.format_exc())
|
||||
print(f'Warn: trigger: "{trigger}" not found')
|
||||
|
||||
with ModelPatcher.apply_lora_text_encoder(
|
||||
text_encoder_info.context.model, _lora_loader()
|
||||
), ModelPatcher.apply_ti(tokenizer_info.context.model, text_encoder_info.context.model, ti_list) as (
|
||||
tokenizer,
|
||||
ti_manager,
|
||||
), ModelPatcher.apply_clip_skip(
|
||||
text_encoder_info.context.model, self.clip.skipped_layers
|
||||
), text_encoder_info as text_encoder:
|
||||
with (
|
||||
ModelPatcher.apply_lora_text_encoder(text_encoder_info.context.model, _lora_loader()),
|
||||
ModelPatcher.apply_ti(tokenizer_info.context.model, text_encoder_info.context.model, ti_list) as (
|
||||
tokenizer,
|
||||
ti_manager,
|
||||
),
|
||||
ModelPatcher.apply_clip_skip(text_encoder_info.context.model, self.clip.skipped_layers),
|
||||
text_encoder_info as text_encoder,
|
||||
):
|
||||
compel = Compel(
|
||||
tokenizer=tokenizer,
|
||||
text_encoder=text_encoder,
|
||||
@ -122,7 +123,7 @@ class CompelInvocation(BaseInvocation):
|
||||
|
||||
c, options = compel.build_conditioning_tensor_for_conjunction(conjunction)
|
||||
|
||||
ec = InvokeAIDiffuserComponent.ExtraConditioningInfo(
|
||||
ec = ExtraConditioningInfo(
|
||||
tokens_count_including_eos_bos=get_max_token_count(tokenizer, conjunction),
|
||||
cross_attention_control_args=options.get("cross_attention_control", None),
|
||||
)
|
||||
@ -213,14 +214,15 @@ class SDXLPromptInvocationBase:
|
||||
# print(traceback.format_exc())
|
||||
print(f'Warn: trigger: "{trigger}" not found')
|
||||
|
||||
with ModelPatcher.apply_lora(
|
||||
text_encoder_info.context.model, _lora_loader(), lora_prefix
|
||||
), ModelPatcher.apply_ti(tokenizer_info.context.model, text_encoder_info.context.model, ti_list) as (
|
||||
tokenizer,
|
||||
ti_manager,
|
||||
), ModelPatcher.apply_clip_skip(
|
||||
text_encoder_info.context.model, clip_field.skipped_layers
|
||||
), text_encoder_info as text_encoder:
|
||||
with (
|
||||
ModelPatcher.apply_lora(text_encoder_info.context.model, _lora_loader(), lora_prefix),
|
||||
ModelPatcher.apply_ti(tokenizer_info.context.model, text_encoder_info.context.model, ti_list) as (
|
||||
tokenizer,
|
||||
ti_manager,
|
||||
),
|
||||
ModelPatcher.apply_clip_skip(text_encoder_info.context.model, clip_field.skipped_layers),
|
||||
text_encoder_info as text_encoder,
|
||||
):
|
||||
compel = Compel(
|
||||
tokenizer=tokenizer,
|
||||
text_encoder=text_encoder,
|
||||
@ -244,7 +246,7 @@ class SDXLPromptInvocationBase:
|
||||
else:
|
||||
c_pooled = None
|
||||
|
||||
ec = InvokeAIDiffuserComponent.ExtraConditioningInfo(
|
||||
ec = ExtraConditioningInfo(
|
||||
tokens_count_including_eos_bos=get_max_token_count(tokenizer, conjunction),
|
||||
cross_attention_control_args=options.get("cross_attention_control", None),
|
||||
)
|
||||
@ -436,9 +438,11 @@ def get_tokens_for_prompt_object(tokenizer, parsed_prompt: FlattenedPrompt, trun
|
||||
raise ValueError("Blend is not supported here - you need to get tokens for each of its .children")
|
||||
|
||||
text_fragments = [
|
||||
x.text
|
||||
if type(x) is Fragment
|
||||
else (" ".join([f.text for f in x.original]) if type(x) is CrossAttentionControlSubstitute else str(x))
|
||||
(
|
||||
x.text
|
||||
if type(x) is Fragment
|
||||
else (" ".join([f.text for f in x.original]) if type(x) is CrossAttentionControlSubstitute else str(x))
|
||||
)
|
||||
for x in parsed_prompt.children
|
||||
]
|
||||
text = " ".join(text_fragments)
|
||||
|
105
invokeai/app/invocations/ip_adapter.py
Normal file
105
invokeai/app/invocations/ip_adapter.py
Normal file
@ -0,0 +1,105 @@
|
||||
import os
|
||||
from builtins import float
|
||||
from typing import List, Union
|
||||
|
||||
from pydantic import BaseModel, Field
|
||||
|
||||
from invokeai.app.invocations.baseinvocation import (
|
||||
BaseInvocation,
|
||||
BaseInvocationOutput,
|
||||
FieldDescriptions,
|
||||
Input,
|
||||
InputField,
|
||||
InvocationContext,
|
||||
OutputField,
|
||||
UIType,
|
||||
invocation,
|
||||
invocation_output,
|
||||
)
|
||||
from invokeai.app.invocations.primitives import ImageField
|
||||
from invokeai.backend.model_management.models.base import BaseModelType, ModelType
|
||||
from invokeai.backend.model_management.models.ip_adapter import get_ip_adapter_image_encoder_model_id
|
||||
|
||||
|
||||
class IPAdapterModelField(BaseModel):
|
||||
model_name: str = Field(description="Name of the IP-Adapter model")
|
||||
base_model: BaseModelType = Field(description="Base model")
|
||||
|
||||
|
||||
class CLIPVisionModelField(BaseModel):
|
||||
model_name: str = Field(description="Name of the CLIP Vision image encoder model")
|
||||
base_model: BaseModelType = Field(description="Base model (usually 'Any')")
|
||||
|
||||
|
||||
class IPAdapterField(BaseModel):
|
||||
image: ImageField = Field(description="The IP-Adapter image prompt.")
|
||||
ip_adapter_model: IPAdapterModelField = Field(description="The IP-Adapter model to use.")
|
||||
image_encoder_model: CLIPVisionModelField = Field(description="The name of the CLIP image encoder model.")
|
||||
weight: Union[float, List[float]] = Field(default=1, description="The weight given to the ControlNet")
|
||||
# weight: float = Field(default=1.0, ge=0, description="The weight of the IP-Adapter.")
|
||||
begin_step_percent: float = Field(
|
||||
default=0, ge=0, le=1, description="When the IP-Adapter is first applied (% of total steps)"
|
||||
)
|
||||
end_step_percent: float = Field(
|
||||
default=1, ge=0, le=1, description="When the IP-Adapter is last applied (% of total steps)"
|
||||
)
|
||||
|
||||
|
||||
@invocation_output("ip_adapter_output")
|
||||
class IPAdapterOutput(BaseInvocationOutput):
|
||||
# Outputs
|
||||
ip_adapter: IPAdapterField = OutputField(description=FieldDescriptions.ip_adapter, title="IP-Adapter")
|
||||
|
||||
|
||||
@invocation("ip_adapter", title="IP-Adapter", tags=["ip_adapter", "control"], category="ip_adapter", version="1.0.0")
|
||||
class IPAdapterInvocation(BaseInvocation):
|
||||
"""Collects IP-Adapter info to pass to other nodes."""
|
||||
|
||||
# Inputs
|
||||
image: ImageField = InputField(description="The IP-Adapter image prompt.")
|
||||
ip_adapter_model: IPAdapterModelField = InputField(
|
||||
description="The IP-Adapter model.",
|
||||
title="IP-Adapter Model",
|
||||
input=Input.Direct,
|
||||
)
|
||||
|
||||
# weight: float = InputField(default=1.0, description="The weight of the IP-Adapter.", ui_type=UIType.Float)
|
||||
weight: Union[float, List[float]] = InputField(
|
||||
default=1, ge=0, description="The weight given to the IP-Adapter", ui_type=UIType.Float, title="Weight"
|
||||
)
|
||||
|
||||
begin_step_percent: float = InputField(
|
||||
default=0, ge=-1, le=2, description="When the IP-Adapter is first applied (% of total steps)"
|
||||
)
|
||||
end_step_percent: float = InputField(
|
||||
default=1, ge=0, le=1, description="When the IP-Adapter is last applied (% of total steps)"
|
||||
)
|
||||
|
||||
def invoke(self, context: InvocationContext) -> IPAdapterOutput:
|
||||
# Lookup the CLIP Vision encoder that is intended to be used with the IP-Adapter model.
|
||||
ip_adapter_info = context.services.model_manager.model_info(
|
||||
self.ip_adapter_model.model_name, self.ip_adapter_model.base_model, ModelType.IPAdapter
|
||||
)
|
||||
# HACK(ryand): This is bad for a couple of reasons: 1) we are bypassing the model manager to read the model
|
||||
# directly, and 2) we are reading from disk every time this invocation is called without caching the result.
|
||||
# A better solution would be to store the image encoder model reference in the IP-Adapter model info, but this
|
||||
# is currently messy due to differences between how the model info is generated when installing a model from
|
||||
# disk vs. downloading the model.
|
||||
image_encoder_model_id = get_ip_adapter_image_encoder_model_id(
|
||||
os.path.join(context.services.configuration.get_config().models_path, ip_adapter_info["path"])
|
||||
)
|
||||
image_encoder_model_name = image_encoder_model_id.split("/")[-1].strip()
|
||||
image_encoder_model = CLIPVisionModelField(
|
||||
model_name=image_encoder_model_name,
|
||||
base_model=BaseModelType.Any,
|
||||
)
|
||||
return IPAdapterOutput(
|
||||
ip_adapter=IPAdapterField(
|
||||
image=self.image,
|
||||
ip_adapter_model=self.ip_adapter_model,
|
||||
image_encoder_model=image_encoder_model,
|
||||
weight=self.weight,
|
||||
begin_step_percent=self.begin_step_percent,
|
||||
end_step_percent=self.end_step_percent,
|
||||
),
|
||||
)
|
@ -8,6 +8,7 @@ import numpy as np
|
||||
import torch
|
||||
import torchvision.transforms as T
|
||||
from diffusers.image_processor import VaeImageProcessor
|
||||
from diffusers.models import UNet2DConditionModel
|
||||
from diffusers.models.attention_processor import (
|
||||
AttnProcessor2_0,
|
||||
LoRAAttnProcessor2_0,
|
||||
@ -19,6 +20,7 @@ from diffusers.schedulers import SchedulerMixin as Scheduler
|
||||
from pydantic import validator
|
||||
from torchvision.transforms.functional import resize as tv_resize
|
||||
|
||||
from invokeai.app.invocations.ip_adapter import IPAdapterField
|
||||
from invokeai.app.invocations.metadata import CoreMetadata
|
||||
from invokeai.app.invocations.primitives import (
|
||||
DenoiseMaskField,
|
||||
@ -31,15 +33,17 @@ from invokeai.app.invocations.primitives import (
|
||||
)
|
||||
from invokeai.app.util.controlnet_utils import prepare_control_image
|
||||
from invokeai.app.util.step_callback import stable_diffusion_step_callback
|
||||
from invokeai.backend.ip_adapter.ip_adapter import IPAdapter, IPAdapterPlus
|
||||
from invokeai.backend.model_management.models import ModelType, SilenceWarnings
|
||||
from invokeai.backend.stable_diffusion.diffusion.conditioning_data import ConditioningData, IPAdapterConditioningInfo
|
||||
|
||||
from ...backend.model_management.lora import ModelPatcher
|
||||
from ...backend.model_management.models import BaseModelType
|
||||
from ...backend.model_management.seamless import set_seamless
|
||||
from ...backend.stable_diffusion import PipelineIntermediateState
|
||||
from ...backend.stable_diffusion.diffusers_pipeline import (
|
||||
ConditioningData,
|
||||
ControlNetData,
|
||||
IPAdapterData,
|
||||
StableDiffusionGeneratorPipeline,
|
||||
image_resized_to_grid_as_tensor,
|
||||
)
|
||||
@ -68,7 +72,6 @@ if choose_torch_device() == torch.device("mps"):
|
||||
|
||||
DEFAULT_PRECISION = choose_precision(choose_torch_device())
|
||||
|
||||
|
||||
SAMPLER_NAME_VALUES = Literal[tuple(list(SCHEDULER_MAP.keys()))]
|
||||
|
||||
|
||||
@ -191,7 +194,7 @@ def get_scheduler(
|
||||
title="Denoise Latents",
|
||||
tags=["latents", "denoise", "txt2img", "t2i", "t2l", "img2img", "i2i", "l2l"],
|
||||
category="latents",
|
||||
version="1.0.0",
|
||||
version="1.1.0",
|
||||
)
|
||||
class DenoiseLatentsInvocation(BaseInvocation):
|
||||
"""Denoises noisy latents to decodable images"""
|
||||
@ -219,9 +222,12 @@ class DenoiseLatentsInvocation(BaseInvocation):
|
||||
input=Input.Connection,
|
||||
ui_order=5,
|
||||
)
|
||||
ip_adapter: Optional[IPAdapterField] = InputField(
|
||||
description=FieldDescriptions.ip_adapter, title="IP-Adapter", default=None, input=Input.Connection, ui_order=6
|
||||
)
|
||||
latents: Optional[LatentsField] = InputField(description=FieldDescriptions.latents, input=Input.Connection)
|
||||
denoise_mask: Optional[DenoiseMaskField] = InputField(
|
||||
default=None, description=FieldDescriptions.mask, input=Input.Connection, ui_order=6
|
||||
default=None, description=FieldDescriptions.mask, input=Input.Connection, ui_order=7
|
||||
)
|
||||
|
||||
@validator("cfg_scale")
|
||||
@ -323,8 +329,6 @@ class DenoiseLatentsInvocation(BaseInvocation):
|
||||
def prep_control_data(
|
||||
self,
|
||||
context: InvocationContext,
|
||||
# really only need model for dtype and device
|
||||
model: StableDiffusionGeneratorPipeline,
|
||||
control_input: Union[ControlField, List[ControlField]],
|
||||
latents_shape: List[int],
|
||||
exit_stack: ExitStack,
|
||||
@ -344,57 +348,107 @@ class DenoiseLatentsInvocation(BaseInvocation):
|
||||
else:
|
||||
control_list = None
|
||||
if control_list is None:
|
||||
control_data = None
|
||||
# from above handling, any control that is not None should now be of type list[ControlField]
|
||||
else:
|
||||
# FIXME: add checks to skip entry if model or image is None
|
||||
# and if weight is None, populate with default 1.0?
|
||||
control_data = []
|
||||
control_models = []
|
||||
for control_info in control_list:
|
||||
control_model = exit_stack.enter_context(
|
||||
context.services.model_manager.get_model(
|
||||
model_name=control_info.control_model.model_name,
|
||||
model_type=ModelType.ControlNet,
|
||||
base_model=control_info.control_model.base_model,
|
||||
context=context,
|
||||
)
|
||||
)
|
||||
return None
|
||||
# After above handling, any control that is not None should now be of type list[ControlField].
|
||||
|
||||
control_models.append(control_model)
|
||||
control_image_field = control_info.image
|
||||
input_image = context.services.images.get_pil_image(control_image_field.image_name)
|
||||
# self.image.image_type, self.image.image_name
|
||||
# FIXME: still need to test with different widths, heights, devices, dtypes
|
||||
# and add in batch_size, num_images_per_prompt?
|
||||
# and do real check for classifier_free_guidance?
|
||||
# prepare_control_image should return torch.Tensor of shape(batch_size, 3, height, width)
|
||||
control_image = prepare_control_image(
|
||||
image=input_image,
|
||||
do_classifier_free_guidance=do_classifier_free_guidance,
|
||||
width=control_width_resize,
|
||||
height=control_height_resize,
|
||||
# batch_size=batch_size * num_images_per_prompt,
|
||||
# num_images_per_prompt=num_images_per_prompt,
|
||||
device=control_model.device,
|
||||
dtype=control_model.dtype,
|
||||
control_mode=control_info.control_mode,
|
||||
resize_mode=control_info.resize_mode,
|
||||
# FIXME: add checks to skip entry if model or image is None
|
||||
# and if weight is None, populate with default 1.0?
|
||||
controlnet_data = []
|
||||
for control_info in control_list:
|
||||
control_model = exit_stack.enter_context(
|
||||
context.services.model_manager.get_model(
|
||||
model_name=control_info.control_model.model_name,
|
||||
model_type=ModelType.ControlNet,
|
||||
base_model=control_info.control_model.base_model,
|
||||
context=context,
|
||||
)
|
||||
control_item = ControlNetData(
|
||||
model=control_model,
|
||||
image_tensor=control_image,
|
||||
weight=control_info.control_weight,
|
||||
begin_step_percent=control_info.begin_step_percent,
|
||||
end_step_percent=control_info.end_step_percent,
|
||||
control_mode=control_info.control_mode,
|
||||
# any resizing needed should currently be happening in prepare_control_image(),
|
||||
# but adding resize_mode to ControlNetData in case needed in the future
|
||||
resize_mode=control_info.resize_mode,
|
||||
)
|
||||
control_data.append(control_item)
|
||||
# MultiControlNetModel has been refactored out, just need list[ControlNetData]
|
||||
return control_data
|
||||
)
|
||||
|
||||
# control_models.append(control_model)
|
||||
control_image_field = control_info.image
|
||||
input_image = context.services.images.get_pil_image(control_image_field.image_name)
|
||||
# self.image.image_type, self.image.image_name
|
||||
# FIXME: still need to test with different widths, heights, devices, dtypes
|
||||
# and add in batch_size, num_images_per_prompt?
|
||||
# and do real check for classifier_free_guidance?
|
||||
# prepare_control_image should return torch.Tensor of shape(batch_size, 3, height, width)
|
||||
control_image = prepare_control_image(
|
||||
image=input_image,
|
||||
do_classifier_free_guidance=do_classifier_free_guidance,
|
||||
width=control_width_resize,
|
||||
height=control_height_resize,
|
||||
# batch_size=batch_size * num_images_per_prompt,
|
||||
# num_images_per_prompt=num_images_per_prompt,
|
||||
device=control_model.device,
|
||||
dtype=control_model.dtype,
|
||||
control_mode=control_info.control_mode,
|
||||
resize_mode=control_info.resize_mode,
|
||||
)
|
||||
control_item = ControlNetData(
|
||||
model=control_model, # model object
|
||||
image_tensor=control_image,
|
||||
weight=control_info.control_weight,
|
||||
begin_step_percent=control_info.begin_step_percent,
|
||||
end_step_percent=control_info.end_step_percent,
|
||||
control_mode=control_info.control_mode,
|
||||
# any resizing needed should currently be happening in prepare_control_image(),
|
||||
# but adding resize_mode to ControlNetData in case needed in the future
|
||||
resize_mode=control_info.resize_mode,
|
||||
)
|
||||
controlnet_data.append(control_item)
|
||||
# MultiControlNetModel has been refactored out, just need list[ControlNetData]
|
||||
|
||||
return controlnet_data
|
||||
|
||||
def prep_ip_adapter_data(
|
||||
self,
|
||||
context: InvocationContext,
|
||||
ip_adapter: Optional[IPAdapterField],
|
||||
conditioning_data: ConditioningData,
|
||||
unet: UNet2DConditionModel,
|
||||
exit_stack: ExitStack,
|
||||
) -> Optional[IPAdapterData]:
|
||||
"""If IP-Adapter is enabled, then this function loads the requisite models, and adds the image prompt embeddings
|
||||
to the `conditioning_data` (in-place).
|
||||
"""
|
||||
if ip_adapter is None:
|
||||
return None
|
||||
|
||||
image_encoder_model_info = context.services.model_manager.get_model(
|
||||
model_name=ip_adapter.image_encoder_model.model_name,
|
||||
model_type=ModelType.CLIPVision,
|
||||
base_model=ip_adapter.image_encoder_model.base_model,
|
||||
context=context,
|
||||
)
|
||||
|
||||
ip_adapter_model: Union[IPAdapter, IPAdapterPlus] = exit_stack.enter_context(
|
||||
context.services.model_manager.get_model(
|
||||
model_name=ip_adapter.ip_adapter_model.model_name,
|
||||
model_type=ModelType.IPAdapter,
|
||||
base_model=ip_adapter.ip_adapter_model.base_model,
|
||||
context=context,
|
||||
)
|
||||
)
|
||||
|
||||
input_image = context.services.images.get_pil_image(ip_adapter.image.image_name)
|
||||
|
||||
# TODO(ryand): With some effort, the step of running the CLIP Vision encoder could be done before any other
|
||||
# models are needed in memory. This would help to reduce peak memory utilization in low-memory environments.
|
||||
with image_encoder_model_info as image_encoder_model:
|
||||
# Get image embeddings from CLIP and ImageProjModel.
|
||||
image_prompt_embeds, uncond_image_prompt_embeds = ip_adapter_model.get_image_embeds(
|
||||
input_image, image_encoder_model
|
||||
)
|
||||
conditioning_data.ip_adapter_conditioning = IPAdapterConditioningInfo(
|
||||
image_prompt_embeds, uncond_image_prompt_embeds
|
||||
)
|
||||
|
||||
return IPAdapterData(
|
||||
ip_adapter_model=ip_adapter_model,
|
||||
weight=ip_adapter.weight,
|
||||
begin_step_percent=ip_adapter.begin_step_percent,
|
||||
end_step_percent=ip_adapter.end_step_percent,
|
||||
)
|
||||
|
||||
# original idea by https://github.com/AmericanPresidentJimmyCarter
|
||||
# TODO: research more for second order schedulers timesteps
|
||||
@ -488,9 +542,12 @@ class DenoiseLatentsInvocation(BaseInvocation):
|
||||
**self.unet.unet.dict(),
|
||||
context=context,
|
||||
)
|
||||
with ExitStack() as exit_stack, ModelPatcher.apply_lora_unet(
|
||||
unet_info.context.model, _lora_loader()
|
||||
), set_seamless(unet_info.context.model, self.unet.seamless_axes), unet_info as unet:
|
||||
with (
|
||||
ExitStack() as exit_stack,
|
||||
ModelPatcher.apply_lora_unet(unet_info.context.model, _lora_loader()),
|
||||
set_seamless(unet_info.context.model, self.unet.seamless_axes),
|
||||
unet_info as unet,
|
||||
):
|
||||
latents = latents.to(device=unet.device, dtype=unet.dtype)
|
||||
if noise is not None:
|
||||
noise = noise.to(device=unet.device, dtype=unet.dtype)
|
||||
@ -509,8 +566,7 @@ class DenoiseLatentsInvocation(BaseInvocation):
|
||||
pipeline = self.create_pipeline(unet, scheduler)
|
||||
conditioning_data = self.get_conditioning_data(context, scheduler, unet, seed)
|
||||
|
||||
control_data = self.prep_control_data(
|
||||
model=pipeline,
|
||||
controlnet_data = self.prep_control_data(
|
||||
context=context,
|
||||
control_input=self.control,
|
||||
latents_shape=latents.shape,
|
||||
@ -519,6 +575,14 @@ class DenoiseLatentsInvocation(BaseInvocation):
|
||||
exit_stack=exit_stack,
|
||||
)
|
||||
|
||||
ip_adapter_data = self.prep_ip_adapter_data(
|
||||
context=context,
|
||||
ip_adapter=self.ip_adapter,
|
||||
conditioning_data=conditioning_data,
|
||||
unet=unet,
|
||||
exit_stack=exit_stack,
|
||||
)
|
||||
|
||||
num_inference_steps, timesteps, init_timestep = self.init_scheduler(
|
||||
scheduler,
|
||||
device=unet.device,
|
||||
@ -537,7 +601,8 @@ class DenoiseLatentsInvocation(BaseInvocation):
|
||||
masked_latents=masked_latents,
|
||||
num_inference_steps=num_inference_steps,
|
||||
conditioning_data=conditioning_data,
|
||||
control_data=control_data, # list[ControlNetData]
|
||||
control_data=controlnet_data, # list[ControlNetData],
|
||||
ip_adapter_data=ip_adapter_data, # IPAdapterData,
|
||||
callback=step_callback,
|
||||
)
|
||||
|
||||
|
@ -95,9 +95,10 @@ class ONNXPromptInvocation(BaseInvocation):
|
||||
print(f'Warn: trigger: "{trigger}" not found')
|
||||
if loras or ti_list:
|
||||
text_encoder.release_session()
|
||||
with ONNXModelPatcher.apply_lora_text_encoder(text_encoder, loras), ONNXModelPatcher.apply_ti(
|
||||
orig_tokenizer, text_encoder, ti_list
|
||||
) as (tokenizer, ti_manager):
|
||||
with (
|
||||
ONNXModelPatcher.apply_lora_text_encoder(text_encoder, loras),
|
||||
ONNXModelPatcher.apply_ti(orig_tokenizer, text_encoder, ti_list) as (tokenizer, ti_manager),
|
||||
):
|
||||
text_encoder.create_session()
|
||||
|
||||
# copy from
|
||||
|
@ -326,6 +326,16 @@ class ModelInstall(object):
|
||||
elif f"learned_embeds.{suffix}" in files:
|
||||
location = self._download_hf_model(repo_id, [f"learned_embeds.{suffix}"], staging)
|
||||
break
|
||||
elif "image_encoder.txt" in files and f"ip_adapter.{suffix}" in files: # IP-Adapter
|
||||
files = ["image_encoder.txt", f"ip_adapter.{suffix}"]
|
||||
location = self._download_hf_model(repo_id, files, staging)
|
||||
break
|
||||
elif f"model.{suffix}" in files and "config.json" in files:
|
||||
# This elif-condition is pretty fragile, but it is intended to handle CLIP Vision models hosted
|
||||
# by InvokeAI for use with IP-Adapters.
|
||||
files = ["config.json", f"model.{suffix}"]
|
||||
location = self._download_hf_model(repo_id, files, staging)
|
||||
break
|
||||
if not location:
|
||||
logger.warning(f"Could not determine type of repo {repo_id}. Skipping install.")
|
||||
return {}
|
||||
@ -534,14 +544,17 @@ def hf_download_with_resume(
|
||||
logger.info(f"{model_name}: Downloading...")
|
||||
|
||||
try:
|
||||
with open(model_dest, open_mode) as file, tqdm(
|
||||
desc=model_name,
|
||||
initial=exist_size,
|
||||
total=total + exist_size,
|
||||
unit="iB",
|
||||
unit_scale=True,
|
||||
unit_divisor=1000,
|
||||
) as bar:
|
||||
with (
|
||||
open(model_dest, open_mode) as file,
|
||||
tqdm(
|
||||
desc=model_name,
|
||||
initial=exist_size,
|
||||
total=total + exist_size,
|
||||
unit="iB",
|
||||
unit_scale=True,
|
||||
unit_divisor=1000,
|
||||
) as bar,
|
||||
):
|
||||
for data in resp.iter_content(chunk_size=1024):
|
||||
size = file.write(data)
|
||||
bar.update(size)
|
||||
|
45
invokeai/backend/ip_adapter/README.md
Normal file
45
invokeai/backend/ip_adapter/README.md
Normal file
@ -0,0 +1,45 @@
|
||||
# IP-Adapter Model Formats
|
||||
|
||||
The official IP-Adapter models are released here: [h94/IP-Adapter](https://huggingface.co/h94/IP-Adapter)
|
||||
|
||||
This official model repo does not integrate well with InvokeAI's current approach to model management, so we have defined a new file structure for IP-Adapter models. The InvokeAI format is described below.
|
||||
|
||||
## CLIP Vision Models
|
||||
|
||||
CLIP Vision models are organized in `diffusers`` format. The expected directory structure is:
|
||||
|
||||
```bash
|
||||
ip_adapter_sd_image_encoder/
|
||||
├── config.json
|
||||
└── model.safetensors
|
||||
```
|
||||
|
||||
## IP-Adapter Models
|
||||
|
||||
IP-Adapter models are stored in a directory containing two files
|
||||
- `image_encoder.txt`: A text file containing the model identifier for the CLIP Vision encoder that is intended to be used with this IP-Adapter model.
|
||||
- `ip_adapter.bin`: The IP-Adapter weights.
|
||||
|
||||
Sample directory structure:
|
||||
```bash
|
||||
ip_adapter_sd15/
|
||||
├── image_encoder.txt
|
||||
└── ip_adapter.bin
|
||||
```
|
||||
|
||||
### Why save the weights in a .safetensors file?
|
||||
|
||||
The weights in `ip_adapter.bin` are stored in a nested dict, which is not supported by `safetensors`. This could be solved by splitting `ip_adapter.bin` into multiple files, but for now we have decided to maintain consistency with the checkpoint structure used in the official [h94/IP-Adapter](https://huggingface.co/h94/IP-Adapter) repo.
|
||||
|
||||
## InvokeAI Hosted IP-Adapters
|
||||
|
||||
Image Encoders:
|
||||
- [InvokeAI/ip_adapter_sd_image_encoder](https://huggingface.co/InvokeAI/ip_adapter_sd_image_encoder)
|
||||
- [InvokeAI/ip_adapter_sdxl_image_encoder](https://huggingface.co/InvokeAI/ip_adapter_sdxl_image_encoder)
|
||||
|
||||
IP-Adapters:
|
||||
- [InvokeAI/ip_adapter_sd15](https://huggingface.co/InvokeAI/ip_adapter_sd15)
|
||||
- [InvokeAI/ip_adapter_plus_sd15](https://huggingface.co/InvokeAI/ip_adapter_plus_sd15)
|
||||
- [InvokeAI/ip_adapter_plus_face_sd15](https://huggingface.co/InvokeAI/ip_adapter_plus_face_sd15)
|
||||
- [InvokeAI/ip_adapter_sdxl](https://huggingface.co/InvokeAI/ip_adapter_sdxl)
|
||||
- Not yet supported: [InvokeAI/ip_adapter_sdxl_vit_h](https://huggingface.co/InvokeAI/ip_adapter_sdxl_vit_h)
|
0
invokeai/backend/ip_adapter/__init__.py
Normal file
0
invokeai/backend/ip_adapter/__init__.py
Normal file
162
invokeai/backend/ip_adapter/attention_processor.py
Normal file
162
invokeai/backend/ip_adapter/attention_processor.py
Normal file
@ -0,0 +1,162 @@
|
||||
# copied from https://github.com/tencent-ailab/IP-Adapter (Apache License 2.0)
|
||||
# and modified as needed
|
||||
|
||||
# tencent-ailab comment:
|
||||
# modified from https://github.com/huggingface/diffusers/blob/main/src/diffusers/models/attention_processor.py
|
||||
import torch
|
||||
import torch.nn as nn
|
||||
import torch.nn.functional as F
|
||||
from diffusers.models.attention_processor import AttnProcessor2_0 as DiffusersAttnProcessor2_0
|
||||
|
||||
|
||||
# Create a version of AttnProcessor2_0 that is a sub-class of nn.Module. This is required for IP-Adapter state_dict
|
||||
# loading.
|
||||
class AttnProcessor2_0(DiffusersAttnProcessor2_0, nn.Module):
|
||||
def __init__(self):
|
||||
DiffusersAttnProcessor2_0.__init__(self)
|
||||
nn.Module.__init__(self)
|
||||
|
||||
def __call__(
|
||||
self,
|
||||
attn,
|
||||
hidden_states,
|
||||
encoder_hidden_states=None,
|
||||
attention_mask=None,
|
||||
temb=None,
|
||||
ip_adapter_image_prompt_embeds=None,
|
||||
):
|
||||
"""Re-definition of DiffusersAttnProcessor2_0.__call__(...) that accepts and ignores the
|
||||
ip_adapter_image_prompt_embeds parameter.
|
||||
"""
|
||||
return DiffusersAttnProcessor2_0.__call__(
|
||||
self, attn, hidden_states, encoder_hidden_states, attention_mask, temb
|
||||
)
|
||||
|
||||
|
||||
class IPAttnProcessor2_0(torch.nn.Module):
|
||||
r"""
|
||||
Attention processor for IP-Adapater for PyTorch 2.0.
|
||||
Args:
|
||||
hidden_size (`int`):
|
||||
The hidden size of the attention layer.
|
||||
cross_attention_dim (`int`):
|
||||
The number of channels in the `encoder_hidden_states`.
|
||||
scale (`float`, defaults to 1.0):
|
||||
the weight scale of image prompt.
|
||||
"""
|
||||
|
||||
def __init__(self, hidden_size, cross_attention_dim=None, scale=1.0):
|
||||
super().__init__()
|
||||
|
||||
if not hasattr(F, "scaled_dot_product_attention"):
|
||||
raise ImportError("AttnProcessor2_0 requires PyTorch 2.0, to use it, please upgrade PyTorch to 2.0.")
|
||||
|
||||
self.hidden_size = hidden_size
|
||||
self.cross_attention_dim = cross_attention_dim
|
||||
self.scale = scale
|
||||
|
||||
self.to_k_ip = nn.Linear(cross_attention_dim or hidden_size, hidden_size, bias=False)
|
||||
self.to_v_ip = nn.Linear(cross_attention_dim or hidden_size, hidden_size, bias=False)
|
||||
|
||||
def __call__(
|
||||
self,
|
||||
attn,
|
||||
hidden_states,
|
||||
encoder_hidden_states=None,
|
||||
attention_mask=None,
|
||||
temb=None,
|
||||
ip_adapter_image_prompt_embeds=None,
|
||||
):
|
||||
if encoder_hidden_states is not None:
|
||||
# If encoder_hidden_states is not None, then we are doing cross-attention, not self-attention. In this case,
|
||||
# we will apply IP-Adapter conditioning. We validate the inputs for IP-Adapter conditioning here.
|
||||
assert ip_adapter_image_prompt_embeds is not None
|
||||
# The batch dimensions should match.
|
||||
assert ip_adapter_image_prompt_embeds.shape[0] == encoder_hidden_states.shape[0]
|
||||
# The channel dimensions should match.
|
||||
assert ip_adapter_image_prompt_embeds.shape[2] == encoder_hidden_states.shape[2]
|
||||
ip_hidden_states = ip_adapter_image_prompt_embeds
|
||||
|
||||
residual = hidden_states
|
||||
|
||||
if attn.spatial_norm is not None:
|
||||
hidden_states = attn.spatial_norm(hidden_states, temb)
|
||||
|
||||
input_ndim = hidden_states.ndim
|
||||
|
||||
if input_ndim == 4:
|
||||
batch_size, channel, height, width = hidden_states.shape
|
||||
hidden_states = hidden_states.view(batch_size, channel, height * width).transpose(1, 2)
|
||||
|
||||
batch_size, sequence_length, _ = (
|
||||
hidden_states.shape if encoder_hidden_states is None else encoder_hidden_states.shape
|
||||
)
|
||||
|
||||
if attention_mask is not None:
|
||||
attention_mask = attn.prepare_attention_mask(attention_mask, sequence_length, batch_size)
|
||||
# scaled_dot_product_attention expects attention_mask shape to be
|
||||
# (batch, heads, source_length, target_length)
|
||||
attention_mask = attention_mask.view(batch_size, attn.heads, -1, attention_mask.shape[-1])
|
||||
|
||||
if attn.group_norm is not None:
|
||||
hidden_states = attn.group_norm(hidden_states.transpose(1, 2)).transpose(1, 2)
|
||||
|
||||
query = attn.to_q(hidden_states)
|
||||
|
||||
if encoder_hidden_states is None:
|
||||
encoder_hidden_states = hidden_states
|
||||
elif attn.norm_cross:
|
||||
encoder_hidden_states = attn.norm_encoder_hidden_states(encoder_hidden_states)
|
||||
|
||||
key = attn.to_k(encoder_hidden_states)
|
||||
value = attn.to_v(encoder_hidden_states)
|
||||
|
||||
inner_dim = key.shape[-1]
|
||||
head_dim = inner_dim // attn.heads
|
||||
|
||||
query = query.view(batch_size, -1, attn.heads, head_dim).transpose(1, 2)
|
||||
|
||||
key = key.view(batch_size, -1, attn.heads, head_dim).transpose(1, 2)
|
||||
value = value.view(batch_size, -1, attn.heads, head_dim).transpose(1, 2)
|
||||
|
||||
# the output of sdp = (batch, num_heads, seq_len, head_dim)
|
||||
# TODO: add support for attn.scale when we move to Torch 2.1
|
||||
hidden_states = F.scaled_dot_product_attention(
|
||||
query, key, value, attn_mask=attention_mask, dropout_p=0.0, is_causal=False
|
||||
)
|
||||
|
||||
hidden_states = hidden_states.transpose(1, 2).reshape(batch_size, -1, attn.heads * head_dim)
|
||||
hidden_states = hidden_states.to(query.dtype)
|
||||
|
||||
if ip_hidden_states is not None:
|
||||
ip_key = self.to_k_ip(ip_hidden_states)
|
||||
ip_value = self.to_v_ip(ip_hidden_states)
|
||||
|
||||
ip_key = ip_key.view(batch_size, -1, attn.heads, head_dim).transpose(1, 2)
|
||||
ip_value = ip_value.view(batch_size, -1, attn.heads, head_dim).transpose(1, 2)
|
||||
|
||||
# the output of sdp = (batch, num_heads, seq_len, head_dim)
|
||||
# TODO: add support for attn.scale when we move to Torch 2.1
|
||||
ip_hidden_states = F.scaled_dot_product_attention(
|
||||
query, ip_key, ip_value, attn_mask=None, dropout_p=0.0, is_causal=False
|
||||
)
|
||||
|
||||
ip_hidden_states = ip_hidden_states.transpose(1, 2).reshape(batch_size, -1, attn.heads * head_dim)
|
||||
ip_hidden_states = ip_hidden_states.to(query.dtype)
|
||||
|
||||
hidden_states = hidden_states + self.scale * ip_hidden_states
|
||||
|
||||
# linear proj
|
||||
hidden_states = attn.to_out[0](hidden_states)
|
||||
# dropout
|
||||
hidden_states = attn.to_out[1](hidden_states)
|
||||
|
||||
if input_ndim == 4:
|
||||
hidden_states = hidden_states.transpose(-1, -2).reshape(batch_size, channel, height, width)
|
||||
|
||||
if attn.residual_connection:
|
||||
hidden_states = hidden_states + residual
|
||||
|
||||
hidden_states = hidden_states / attn.rescale_output_factor
|
||||
|
||||
return hidden_states
|
217
invokeai/backend/ip_adapter/ip_adapter.py
Normal file
217
invokeai/backend/ip_adapter/ip_adapter.py
Normal file
@ -0,0 +1,217 @@
|
||||
# copied from https://github.com/tencent-ailab/IP-Adapter (Apache License 2.0)
|
||||
# and modified as needed
|
||||
|
||||
from contextlib import contextmanager
|
||||
from typing import Optional, Union
|
||||
|
||||
import torch
|
||||
from diffusers.models import UNet2DConditionModel
|
||||
from PIL import Image
|
||||
from transformers import CLIPImageProcessor, CLIPVisionModelWithProjection
|
||||
|
||||
from .attention_processor import AttnProcessor2_0, IPAttnProcessor2_0
|
||||
from .resampler import Resampler
|
||||
|
||||
|
||||
class ImageProjModel(torch.nn.Module):
|
||||
"""Image Projection Model"""
|
||||
|
||||
def __init__(self, cross_attention_dim=1024, clip_embeddings_dim=1024, clip_extra_context_tokens=4):
|
||||
super().__init__()
|
||||
|
||||
self.cross_attention_dim = cross_attention_dim
|
||||
self.clip_extra_context_tokens = clip_extra_context_tokens
|
||||
self.proj = torch.nn.Linear(clip_embeddings_dim, self.clip_extra_context_tokens * cross_attention_dim)
|
||||
self.norm = torch.nn.LayerNorm(cross_attention_dim)
|
||||
|
||||
@classmethod
|
||||
def from_state_dict(cls, state_dict: dict[torch.Tensor], clip_extra_context_tokens=4):
|
||||
"""Initialize an ImageProjModel from a state_dict.
|
||||
|
||||
The cross_attention_dim and clip_embeddings_dim are inferred from the shape of the tensors in the state_dict.
|
||||
|
||||
Args:
|
||||
state_dict (dict[torch.Tensor]): The state_dict of model weights.
|
||||
clip_extra_context_tokens (int, optional): Defaults to 4.
|
||||
|
||||
Returns:
|
||||
ImageProjModel
|
||||
"""
|
||||
cross_attention_dim = state_dict["norm.weight"].shape[0]
|
||||
clip_embeddings_dim = state_dict["proj.weight"].shape[-1]
|
||||
|
||||
model = cls(cross_attention_dim, clip_embeddings_dim, clip_extra_context_tokens)
|
||||
|
||||
model.load_state_dict(state_dict)
|
||||
return model
|
||||
|
||||
def forward(self, image_embeds):
|
||||
embeds = image_embeds
|
||||
clip_extra_context_tokens = self.proj(embeds).reshape(
|
||||
-1, self.clip_extra_context_tokens, self.cross_attention_dim
|
||||
)
|
||||
clip_extra_context_tokens = self.norm(clip_extra_context_tokens)
|
||||
return clip_extra_context_tokens
|
||||
|
||||
|
||||
class IPAdapter:
|
||||
"""IP-Adapter: https://arxiv.org/pdf/2308.06721.pdf"""
|
||||
|
||||
def __init__(
|
||||
self,
|
||||
state_dict: dict[torch.Tensor],
|
||||
device: torch.device,
|
||||
dtype: torch.dtype = torch.float16,
|
||||
num_tokens: int = 4,
|
||||
):
|
||||
self.device = device
|
||||
self.dtype = dtype
|
||||
|
||||
self._num_tokens = num_tokens
|
||||
|
||||
self._clip_image_processor = CLIPImageProcessor()
|
||||
|
||||
self._state_dict = state_dict
|
||||
|
||||
self._image_proj_model = self._init_image_proj_model(self._state_dict["image_proj"])
|
||||
|
||||
# The _attn_processors will be initialized later when we have access to the UNet.
|
||||
self._attn_processors = None
|
||||
|
||||
def to(self, device: torch.device, dtype: Optional[torch.dtype] = None):
|
||||
self.device = device
|
||||
if dtype is not None:
|
||||
self.dtype = dtype
|
||||
|
||||
self._image_proj_model.to(device=self.device, dtype=self.dtype)
|
||||
if self._attn_processors is not None:
|
||||
torch.nn.ModuleList(self._attn_processors.values()).to(device=self.device, dtype=self.dtype)
|
||||
|
||||
def _init_image_proj_model(self, state_dict):
|
||||
return ImageProjModel.from_state_dict(state_dict, self._num_tokens).to(self.device, dtype=self.dtype)
|
||||
|
||||
def _prepare_attention_processors(self, unet: UNet2DConditionModel):
|
||||
"""Prepare a dict of attention processors that can later be injected into a unet, and load the IP-Adapter
|
||||
attention weights into them.
|
||||
|
||||
Note that the `unet` param is only used to determine attention block dimensions and naming.
|
||||
TODO(ryand): As a future improvement, this could all be inferred from the state_dict when the IPAdapter is
|
||||
intialized.
|
||||
"""
|
||||
attn_procs = {}
|
||||
for name in unet.attn_processors.keys():
|
||||
cross_attention_dim = None if name.endswith("attn1.processor") else unet.config.cross_attention_dim
|
||||
if name.startswith("mid_block"):
|
||||
hidden_size = unet.config.block_out_channels[-1]
|
||||
elif name.startswith("up_blocks"):
|
||||
block_id = int(name[len("up_blocks.")])
|
||||
hidden_size = list(reversed(unet.config.block_out_channels))[block_id]
|
||||
elif name.startswith("down_blocks"):
|
||||
block_id = int(name[len("down_blocks.")])
|
||||
hidden_size = unet.config.block_out_channels[block_id]
|
||||
if cross_attention_dim is None:
|
||||
attn_procs[name] = AttnProcessor2_0()
|
||||
else:
|
||||
attn_procs[name] = IPAttnProcessor2_0(
|
||||
hidden_size=hidden_size,
|
||||
cross_attention_dim=cross_attention_dim,
|
||||
scale=1.0,
|
||||
).to(self.device, dtype=self.dtype)
|
||||
|
||||
ip_layers = torch.nn.ModuleList(attn_procs.values())
|
||||
ip_layers.load_state_dict(self._state_dict["ip_adapter"])
|
||||
self._attn_processors = attn_procs
|
||||
self._state_dict = None
|
||||
|
||||
# @genomancer: pushed scaling back out into its own method (like original Tencent implementation)
|
||||
# which makes implementing begin_step_percent and end_step_percent easier
|
||||
# but based on self._attn_processors (ala @Ryan) instead of original Tencent unet.attn_processors,
|
||||
# which should make it easier to implement multiple IPAdapters
|
||||
def set_scale(self, scale):
|
||||
if self._attn_processors is not None:
|
||||
for attn_processor in self._attn_processors.values():
|
||||
if isinstance(attn_processor, IPAttnProcessor2_0):
|
||||
attn_processor.scale = scale
|
||||
|
||||
@contextmanager
|
||||
def apply_ip_adapter_attention(self, unet: UNet2DConditionModel, scale: float):
|
||||
"""A context manager that patches `unet` with this IP-Adapter's attention processors while it is active.
|
||||
|
||||
Yields:
|
||||
None
|
||||
"""
|
||||
if self._attn_processors is None:
|
||||
# We only have to call _prepare_attention_processors(...) once, and then the result is cached and can be
|
||||
# used on any UNet model (with the same dimensions).
|
||||
self._prepare_attention_processors(unet)
|
||||
|
||||
# Set scale
|
||||
self.set_scale(scale)
|
||||
# for attn_processor in self._attn_processors.values():
|
||||
# if isinstance(attn_processor, IPAttnProcessor2_0):
|
||||
# attn_processor.scale = scale
|
||||
|
||||
orig_attn_processors = unet.attn_processors
|
||||
|
||||
# Make a (moderately-) shallow copy of the self._attn_processors dict, because unet.set_attn_processor(...)
|
||||
# actually pops elements from the passed dict.
|
||||
ip_adapter_attn_processors = {k: v for k, v in self._attn_processors.items()}
|
||||
|
||||
try:
|
||||
unet.set_attn_processor(ip_adapter_attn_processors)
|
||||
yield None
|
||||
finally:
|
||||
unet.set_attn_processor(orig_attn_processors)
|
||||
|
||||
@torch.inference_mode()
|
||||
def get_image_embeds(self, pil_image, image_encoder: CLIPVisionModelWithProjection):
|
||||
if isinstance(pil_image, Image.Image):
|
||||
pil_image = [pil_image]
|
||||
clip_image = self._clip_image_processor(images=pil_image, return_tensors="pt").pixel_values
|
||||
clip_image_embeds = image_encoder(clip_image.to(self.device, dtype=self.dtype)).image_embeds
|
||||
image_prompt_embeds = self._image_proj_model(clip_image_embeds)
|
||||
uncond_image_prompt_embeds = self._image_proj_model(torch.zeros_like(clip_image_embeds))
|
||||
return image_prompt_embeds, uncond_image_prompt_embeds
|
||||
|
||||
|
||||
class IPAdapterPlus(IPAdapter):
|
||||
"""IP-Adapter with fine-grained features"""
|
||||
|
||||
def _init_image_proj_model(self, state_dict):
|
||||
return Resampler.from_state_dict(
|
||||
state_dict=state_dict,
|
||||
depth=4,
|
||||
dim_head=64,
|
||||
heads=12,
|
||||
num_queries=self._num_tokens,
|
||||
ff_mult=4,
|
||||
).to(self.device, dtype=self.dtype)
|
||||
|
||||
@torch.inference_mode()
|
||||
def get_image_embeds(self, pil_image, image_encoder: CLIPVisionModelWithProjection):
|
||||
if isinstance(pil_image, Image.Image):
|
||||
pil_image = [pil_image]
|
||||
clip_image = self._clip_image_processor(images=pil_image, return_tensors="pt").pixel_values
|
||||
clip_image = clip_image.to(self.device, dtype=self.dtype)
|
||||
clip_image_embeds = image_encoder(clip_image, output_hidden_states=True).hidden_states[-2]
|
||||
image_prompt_embeds = self._image_proj_model(clip_image_embeds)
|
||||
uncond_clip_image_embeds = image_encoder(torch.zeros_like(clip_image), output_hidden_states=True).hidden_states[
|
||||
-2
|
||||
]
|
||||
uncond_image_prompt_embeds = self._image_proj_model(uncond_clip_image_embeds)
|
||||
return image_prompt_embeds, uncond_image_prompt_embeds
|
||||
|
||||
|
||||
def build_ip_adapter(
|
||||
ip_adapter_ckpt_path: str, device: torch.device, dtype: torch.dtype = torch.float16
|
||||
) -> Union[IPAdapter, IPAdapterPlus]:
|
||||
state_dict = torch.load(ip_adapter_ckpt_path, map_location="cpu")
|
||||
|
||||
# Determine if the state_dict is from an IPAdapter or IPAdapterPlus based on the image_proj weights that it
|
||||
# contains.
|
||||
is_plus = "proj.weight" not in state_dict["image_proj"]
|
||||
|
||||
if is_plus:
|
||||
return IPAdapterPlus(state_dict, device=device, dtype=dtype)
|
||||
else:
|
||||
return IPAdapter(state_dict, device=device, dtype=dtype)
|
158
invokeai/backend/ip_adapter/resampler.py
Normal file
158
invokeai/backend/ip_adapter/resampler.py
Normal file
@ -0,0 +1,158 @@
|
||||
# copied from https://github.com/tencent-ailab/IP-Adapter (Apache License 2.0)
|
||||
|
||||
# tencent ailab comment: modified from
|
||||
# https://github.com/mlfoundations/open_flamingo/blob/main/open_flamingo/src/helpers.py
|
||||
import math
|
||||
|
||||
import torch
|
||||
import torch.nn as nn
|
||||
|
||||
|
||||
# FFN
|
||||
def FeedForward(dim, mult=4):
|
||||
inner_dim = int(dim * mult)
|
||||
return nn.Sequential(
|
||||
nn.LayerNorm(dim),
|
||||
nn.Linear(dim, inner_dim, bias=False),
|
||||
nn.GELU(),
|
||||
nn.Linear(inner_dim, dim, bias=False),
|
||||
)
|
||||
|
||||
|
||||
def reshape_tensor(x, heads):
|
||||
bs, length, width = x.shape
|
||||
# (bs, length, width) --> (bs, length, n_heads, dim_per_head)
|
||||
x = x.view(bs, length, heads, -1)
|
||||
# (bs, length, n_heads, dim_per_head) --> (bs, n_heads, length, dim_per_head)
|
||||
x = x.transpose(1, 2)
|
||||
# (bs, n_heads, length, dim_per_head) --> (bs*n_heads, length, dim_per_head)
|
||||
x = x.reshape(bs, heads, length, -1)
|
||||
return x
|
||||
|
||||
|
||||
class PerceiverAttention(nn.Module):
|
||||
def __init__(self, *, dim, dim_head=64, heads=8):
|
||||
super().__init__()
|
||||
self.scale = dim_head**-0.5
|
||||
self.dim_head = dim_head
|
||||
self.heads = heads
|
||||
inner_dim = dim_head * heads
|
||||
|
||||
self.norm1 = nn.LayerNorm(dim)
|
||||
self.norm2 = nn.LayerNorm(dim)
|
||||
|
||||
self.to_q = nn.Linear(dim, inner_dim, bias=False)
|
||||
self.to_kv = nn.Linear(dim, inner_dim * 2, bias=False)
|
||||
self.to_out = nn.Linear(inner_dim, dim, bias=False)
|
||||
|
||||
def forward(self, x, latents):
|
||||
"""
|
||||
Args:
|
||||
x (torch.Tensor): image features
|
||||
shape (b, n1, D)
|
||||
latent (torch.Tensor): latent features
|
||||
shape (b, n2, D)
|
||||
"""
|
||||
x = self.norm1(x)
|
||||
latents = self.norm2(latents)
|
||||
|
||||
b, l, _ = latents.shape
|
||||
|
||||
q = self.to_q(latents)
|
||||
kv_input = torch.cat((x, latents), dim=-2)
|
||||
k, v = self.to_kv(kv_input).chunk(2, dim=-1)
|
||||
|
||||
q = reshape_tensor(q, self.heads)
|
||||
k = reshape_tensor(k, self.heads)
|
||||
v = reshape_tensor(v, self.heads)
|
||||
|
||||
# attention
|
||||
scale = 1 / math.sqrt(math.sqrt(self.dim_head))
|
||||
weight = (q * scale) @ (k * scale).transpose(-2, -1) # More stable with f16 than dividing afterwards
|
||||
weight = torch.softmax(weight.float(), dim=-1).type(weight.dtype)
|
||||
out = weight @ v
|
||||
|
||||
out = out.permute(0, 2, 1, 3).reshape(b, l, -1)
|
||||
|
||||
return self.to_out(out)
|
||||
|
||||
|
||||
class Resampler(nn.Module):
|
||||
def __init__(
|
||||
self,
|
||||
dim=1024,
|
||||
depth=8,
|
||||
dim_head=64,
|
||||
heads=16,
|
||||
num_queries=8,
|
||||
embedding_dim=768,
|
||||
output_dim=1024,
|
||||
ff_mult=4,
|
||||
):
|
||||
super().__init__()
|
||||
|
||||
self.latents = nn.Parameter(torch.randn(1, num_queries, dim) / dim**0.5)
|
||||
|
||||
self.proj_in = nn.Linear(embedding_dim, dim)
|
||||
|
||||
self.proj_out = nn.Linear(dim, output_dim)
|
||||
self.norm_out = nn.LayerNorm(output_dim)
|
||||
|
||||
self.layers = nn.ModuleList([])
|
||||
for _ in range(depth):
|
||||
self.layers.append(
|
||||
nn.ModuleList(
|
||||
[
|
||||
PerceiverAttention(dim=dim, dim_head=dim_head, heads=heads),
|
||||
FeedForward(dim=dim, mult=ff_mult),
|
||||
]
|
||||
)
|
||||
)
|
||||
|
||||
@classmethod
|
||||
def from_state_dict(cls, state_dict: dict[torch.Tensor], depth=8, dim_head=64, heads=16, num_queries=8, ff_mult=4):
|
||||
"""A convenience function that initializes a Resampler from a state_dict.
|
||||
|
||||
Some of the shape parameters are inferred from the state_dict (e.g. dim, embedding_dim, etc.). At the time of
|
||||
writing, we did not have a need for inferring ALL of the shape parameters from the state_dict, but this would be
|
||||
possible if needed in the future.
|
||||
|
||||
Args:
|
||||
state_dict (dict[torch.Tensor]): The state_dict to load.
|
||||
depth (int, optional):
|
||||
dim_head (int, optional):
|
||||
heads (int, optional):
|
||||
ff_mult (int, optional):
|
||||
|
||||
Returns:
|
||||
Resampler
|
||||
"""
|
||||
dim = state_dict["latents"].shape[2]
|
||||
num_queries = state_dict["latents"].shape[1]
|
||||
embedding_dim = state_dict["proj_in.weight"].shape[-1]
|
||||
output_dim = state_dict["norm_out.weight"].shape[0]
|
||||
|
||||
model = cls(
|
||||
dim=dim,
|
||||
depth=depth,
|
||||
dim_head=dim_head,
|
||||
heads=heads,
|
||||
num_queries=num_queries,
|
||||
embedding_dim=embedding_dim,
|
||||
output_dim=output_dim,
|
||||
ff_mult=ff_mult,
|
||||
)
|
||||
model.load_state_dict(state_dict)
|
||||
return model
|
||||
|
||||
def forward(self, x):
|
||||
latents = self.latents.repeat(x.size(0), 1, 1)
|
||||
|
||||
x = self.proj_in(x)
|
||||
|
||||
for attn, ff in self.layers:
|
||||
latents = attn(x, latents) + latents
|
||||
latents = ff(latents) + latents
|
||||
|
||||
latents = self.proj_out(latents)
|
||||
return self.norm_out(latents)
|
@ -25,6 +25,7 @@ Models are described using four attributes:
|
||||
ModelType.Lora -- a LoRA or LyCORIS fine-tune
|
||||
ModelType.TextualInversion -- a textual inversion embedding
|
||||
ModelType.ControlNet -- a ControlNet model
|
||||
ModelType.IPAdapter -- an IPAdapter model
|
||||
|
||||
3) BaseModelType -- an enum indicating the stable diffusion base model, one of:
|
||||
BaseModelType.StableDiffusion1
|
||||
@ -1000,8 +1001,8 @@ class ModelManager(object):
|
||||
new_models_found = True
|
||||
except DuplicateModelException as e:
|
||||
self.logger.warning(e)
|
||||
except InvalidModelException:
|
||||
self.logger.warning(f"Not a valid model: {model_path}")
|
||||
except InvalidModelException as e:
|
||||
self.logger.warning(f"Not a valid model: {model_path}. {e}")
|
||||
except NotImplementedError as e:
|
||||
self.logger.warning(e)
|
||||
|
||||
|
@ -8,6 +8,8 @@ import torch
|
||||
from diffusers import ConfigMixin, ModelMixin
|
||||
from picklescan.scanner import scan_file_path
|
||||
|
||||
from invokeai.backend.model_management.models.ip_adapter import IPAdapterModelFormat
|
||||
|
||||
from .models import (
|
||||
BaseModelType,
|
||||
InvalidModelException,
|
||||
@ -52,6 +54,7 @@ class ModelProbe(object):
|
||||
"StableDiffusionXLInpaintPipeline": ModelType.Main,
|
||||
"AutoencoderKL": ModelType.Vae,
|
||||
"ControlNetModel": ModelType.ControlNet,
|
||||
"CLIPVisionModelWithProjection": ModelType.CLIPVision,
|
||||
}
|
||||
|
||||
@classmethod
|
||||
@ -118,14 +121,18 @@ class ModelProbe(object):
|
||||
and prediction_type == SchedulerPredictionType.VPrediction
|
||||
),
|
||||
format=format,
|
||||
image_size=1024
|
||||
if (base_type in {BaseModelType.StableDiffusionXL, BaseModelType.StableDiffusionXLRefiner})
|
||||
else 768
|
||||
if (
|
||||
base_type == BaseModelType.StableDiffusion2
|
||||
and prediction_type == SchedulerPredictionType.VPrediction
|
||||
)
|
||||
else 512,
|
||||
image_size=(
|
||||
1024
|
||||
if (base_type in {BaseModelType.StableDiffusionXL, BaseModelType.StableDiffusionXLRefiner})
|
||||
else (
|
||||
768
|
||||
if (
|
||||
base_type == BaseModelType.StableDiffusion2
|
||||
and prediction_type == SchedulerPredictionType.VPrediction
|
||||
)
|
||||
else 512
|
||||
)
|
||||
),
|
||||
)
|
||||
except Exception:
|
||||
raise
|
||||
@ -177,9 +184,10 @@ class ModelProbe(object):
|
||||
return ModelType.ONNX
|
||||
if (folder_path / "learned_embeds.bin").exists():
|
||||
return ModelType.TextualInversion
|
||||
|
||||
if (folder_path / "pytorch_lora_weights.bin").exists():
|
||||
return ModelType.Lora
|
||||
if (folder_path / "image_encoder.txt").exists():
|
||||
return ModelType.IPAdapter
|
||||
|
||||
i = folder_path / "model_index.json"
|
||||
c = folder_path / "config.json"
|
||||
@ -188,7 +196,12 @@ class ModelProbe(object):
|
||||
if config_path:
|
||||
with open(config_path, "r") as file:
|
||||
conf = json.load(file)
|
||||
class_name = conf["_class_name"]
|
||||
if "_class_name" in conf:
|
||||
class_name = conf["_class_name"]
|
||||
elif "architectures" in conf:
|
||||
class_name = conf["architectures"][0]
|
||||
else:
|
||||
class_name = None
|
||||
|
||||
if class_name and (type := cls.CLASS2TYPE.get(class_name)):
|
||||
return type
|
||||
@ -366,6 +379,16 @@ class ControlNetCheckpointProbe(CheckpointProbeBase):
|
||||
raise InvalidModelException("Unable to determine base type for {self.checkpoint_path}")
|
||||
|
||||
|
||||
class IPAdapterCheckpointProbe(CheckpointProbeBase):
|
||||
def get_base_type(self) -> BaseModelType:
|
||||
raise NotImplementedError()
|
||||
|
||||
|
||||
class CLIPVisionCheckpointProbe(CheckpointProbeBase):
|
||||
def get_base_type(self) -> BaseModelType:
|
||||
raise NotImplementedError()
|
||||
|
||||
|
||||
########################################################
|
||||
# classes for probing folders
|
||||
#######################################################
|
||||
@ -485,11 +508,13 @@ class ControlNetFolderProbe(FolderProbeBase):
|
||||
base_model = (
|
||||
BaseModelType.StableDiffusion1
|
||||
if dimension == 768
|
||||
else BaseModelType.StableDiffusion2
|
||||
if dimension == 1024
|
||||
else BaseModelType.StableDiffusionXL
|
||||
if dimension == 2048
|
||||
else None
|
||||
else (
|
||||
BaseModelType.StableDiffusion2
|
||||
if dimension == 1024
|
||||
else BaseModelType.StableDiffusionXL
|
||||
if dimension == 2048
|
||||
else None
|
||||
)
|
||||
)
|
||||
if not base_model:
|
||||
raise InvalidModelException(f"Unable to determine model base for {self.folder_path}")
|
||||
@ -509,15 +534,47 @@ class LoRAFolderProbe(FolderProbeBase):
|
||||
return LoRACheckpointProbe(model_file, None).get_base_type()
|
||||
|
||||
|
||||
class IPAdapterFolderProbe(FolderProbeBase):
|
||||
def get_format(self) -> str:
|
||||
return IPAdapterModelFormat.InvokeAI.value
|
||||
|
||||
def get_base_type(self) -> BaseModelType:
|
||||
model_file = self.folder_path / "ip_adapter.bin"
|
||||
if not model_file.exists():
|
||||
raise InvalidModelException("Unknown IP-Adapter model format.")
|
||||
|
||||
state_dict = torch.load(model_file, map_location="cpu")
|
||||
cross_attention_dim = state_dict["ip_adapter"]["1.to_k_ip.weight"].shape[-1]
|
||||
if cross_attention_dim == 768:
|
||||
return BaseModelType.StableDiffusion1
|
||||
elif cross_attention_dim == 1024:
|
||||
return BaseModelType.StableDiffusion2
|
||||
elif cross_attention_dim == 2048:
|
||||
return BaseModelType.StableDiffusionXL
|
||||
else:
|
||||
raise InvalidModelException(f"IP-Adapter had unexpected cross-attention dimension: {cross_attention_dim}.")
|
||||
|
||||
|
||||
class CLIPVisionFolderProbe(FolderProbeBase):
|
||||
def get_base_type(self) -> BaseModelType:
|
||||
return BaseModelType.Any
|
||||
|
||||
|
||||
############## register probe classes ######
|
||||
ModelProbe.register_probe("diffusers", ModelType.Main, PipelineFolderProbe)
|
||||
ModelProbe.register_probe("diffusers", ModelType.Vae, VaeFolderProbe)
|
||||
ModelProbe.register_probe("diffusers", ModelType.Lora, LoRAFolderProbe)
|
||||
ModelProbe.register_probe("diffusers", ModelType.TextualInversion, TextualInversionFolderProbe)
|
||||
ModelProbe.register_probe("diffusers", ModelType.ControlNet, ControlNetFolderProbe)
|
||||
ModelProbe.register_probe("diffusers", ModelType.IPAdapter, IPAdapterFolderProbe)
|
||||
ModelProbe.register_probe("diffusers", ModelType.CLIPVision, CLIPVisionFolderProbe)
|
||||
|
||||
ModelProbe.register_probe("checkpoint", ModelType.Main, PipelineCheckpointProbe)
|
||||
ModelProbe.register_probe("checkpoint", ModelType.Vae, VaeCheckpointProbe)
|
||||
ModelProbe.register_probe("checkpoint", ModelType.Lora, LoRACheckpointProbe)
|
||||
ModelProbe.register_probe("checkpoint", ModelType.TextualInversion, TextualInversionCheckpointProbe)
|
||||
ModelProbe.register_probe("checkpoint", ModelType.ControlNet, ControlNetCheckpointProbe)
|
||||
ModelProbe.register_probe("checkpoint", ModelType.IPAdapter, IPAdapterCheckpointProbe)
|
||||
ModelProbe.register_probe("checkpoint", ModelType.CLIPVision, CLIPVisionCheckpointProbe)
|
||||
|
||||
ModelProbe.register_probe("onnx", ModelType.ONNX, ONNXFolderProbe)
|
||||
|
@ -79,7 +79,7 @@ class ModelSearch(ABC):
|
||||
self._models_found += 1
|
||||
self._scanned_dirs.add(path)
|
||||
except Exception as e:
|
||||
self.logger.warning(str(e))
|
||||
self.logger.warning(f"Failed to process '{path}': {e}")
|
||||
|
||||
for f in files:
|
||||
path = Path(root) / f
|
||||
@ -90,7 +90,7 @@ class ModelSearch(ABC):
|
||||
self.on_model_found(path)
|
||||
self._models_found += 1
|
||||
except Exception as e:
|
||||
self.logger.warning(str(e))
|
||||
self.logger.warning(f"Failed to process '{path}': {e}")
|
||||
|
||||
|
||||
class FindModels(ModelSearch):
|
||||
|
@ -18,7 +18,9 @@ from .base import ( # noqa: F401
|
||||
SilenceWarnings,
|
||||
SubModelType,
|
||||
)
|
||||
from .clip_vision import CLIPVisionModel
|
||||
from .controlnet import ControlNetModel # TODO:
|
||||
from .ip_adapter import IPAdapterModel
|
||||
from .lora import LoRAModel
|
||||
from .sdxl import StableDiffusionXLModel
|
||||
from .stable_diffusion import StableDiffusion1Model, StableDiffusion2Model
|
||||
@ -34,6 +36,8 @@ MODEL_CLASSES = {
|
||||
ModelType.Lora: LoRAModel,
|
||||
ModelType.ControlNet: ControlNetModel,
|
||||
ModelType.TextualInversion: TextualInversionModel,
|
||||
ModelType.IPAdapter: IPAdapterModel,
|
||||
ModelType.CLIPVision: CLIPVisionModel,
|
||||
},
|
||||
BaseModelType.StableDiffusion2: {
|
||||
ModelType.ONNX: ONNXStableDiffusion2Model,
|
||||
@ -42,6 +46,8 @@ MODEL_CLASSES = {
|
||||
ModelType.Lora: LoRAModel,
|
||||
ModelType.ControlNet: ControlNetModel,
|
||||
ModelType.TextualInversion: TextualInversionModel,
|
||||
ModelType.IPAdapter: IPAdapterModel,
|
||||
ModelType.CLIPVision: CLIPVisionModel,
|
||||
},
|
||||
BaseModelType.StableDiffusionXL: {
|
||||
ModelType.Main: StableDiffusionXLModel,
|
||||
@ -51,6 +57,8 @@ MODEL_CLASSES = {
|
||||
ModelType.ControlNet: ControlNetModel,
|
||||
ModelType.TextualInversion: TextualInversionModel,
|
||||
ModelType.ONNX: ONNXStableDiffusion2Model,
|
||||
ModelType.IPAdapter: IPAdapterModel,
|
||||
ModelType.CLIPVision: CLIPVisionModel,
|
||||
},
|
||||
BaseModelType.StableDiffusionXLRefiner: {
|
||||
ModelType.Main: StableDiffusionXLModel,
|
||||
@ -60,6 +68,19 @@ MODEL_CLASSES = {
|
||||
ModelType.ControlNet: ControlNetModel,
|
||||
ModelType.TextualInversion: TextualInversionModel,
|
||||
ModelType.ONNX: ONNXStableDiffusion2Model,
|
||||
ModelType.IPAdapter: IPAdapterModel,
|
||||
ModelType.CLIPVision: CLIPVisionModel,
|
||||
},
|
||||
BaseModelType.Any: {
|
||||
ModelType.CLIPVision: CLIPVisionModel,
|
||||
# The following model types are not expected to be used with BaseModelType.Any.
|
||||
ModelType.ONNX: ONNXStableDiffusion2Model,
|
||||
ModelType.Main: StableDiffusion2Model,
|
||||
ModelType.Vae: VaeModel,
|
||||
ModelType.Lora: LoRAModel,
|
||||
ModelType.ControlNet: ControlNetModel,
|
||||
ModelType.TextualInversion: TextualInversionModel,
|
||||
ModelType.IPAdapter: IPAdapterModel,
|
||||
},
|
||||
# BaseModelType.Kandinsky2_1: {
|
||||
# ModelType.Main: Kandinsky2_1Model,
|
||||
|
@ -36,6 +36,7 @@ class ModelNotFoundException(Exception):
|
||||
|
||||
|
||||
class BaseModelType(str, Enum):
|
||||
Any = "any" # For models that are not associated with any particular base model.
|
||||
StableDiffusion1 = "sd-1"
|
||||
StableDiffusion2 = "sd-2"
|
||||
StableDiffusionXL = "sdxl"
|
||||
@ -50,6 +51,8 @@ class ModelType(str, Enum):
|
||||
Lora = "lora"
|
||||
ControlNet = "controlnet" # used by model_probe
|
||||
TextualInversion = "embedding"
|
||||
IPAdapter = "ip_adapter"
|
||||
CLIPVision = "clip_vision"
|
||||
|
||||
|
||||
class SubModelType(str, Enum):
|
||||
|
82
invokeai/backend/model_management/models/clip_vision.py
Normal file
82
invokeai/backend/model_management/models/clip_vision.py
Normal file
@ -0,0 +1,82 @@
|
||||
import os
|
||||
from enum import Enum
|
||||
from typing import Literal, Optional
|
||||
|
||||
import torch
|
||||
from transformers import CLIPVisionModelWithProjection
|
||||
|
||||
from invokeai.backend.model_management.models.base import (
|
||||
BaseModelType,
|
||||
InvalidModelException,
|
||||
ModelBase,
|
||||
ModelConfigBase,
|
||||
ModelType,
|
||||
SubModelType,
|
||||
calc_model_size_by_data,
|
||||
calc_model_size_by_fs,
|
||||
classproperty,
|
||||
)
|
||||
|
||||
|
||||
class CLIPVisionModelFormat(str, Enum):
|
||||
Diffusers = "diffusers"
|
||||
|
||||
|
||||
class CLIPVisionModel(ModelBase):
|
||||
class DiffusersConfig(ModelConfigBase):
|
||||
model_format: Literal[CLIPVisionModelFormat.Diffusers]
|
||||
|
||||
def __init__(self, model_path: str, base_model: BaseModelType, model_type: ModelType):
|
||||
assert model_type == ModelType.CLIPVision
|
||||
super().__init__(model_path, base_model, model_type)
|
||||
|
||||
self.model_size = calc_model_size_by_fs(self.model_path)
|
||||
|
||||
@classmethod
|
||||
def detect_format(cls, path: str) -> str:
|
||||
if not os.path.exists(path):
|
||||
raise ModuleNotFoundError(f"No CLIP Vision model at path '{path}'.")
|
||||
|
||||
if os.path.isdir(path) and os.path.exists(os.path.join(path, "config.json")):
|
||||
return CLIPVisionModelFormat.Diffusers
|
||||
|
||||
raise InvalidModelException(f"Unexpected CLIP Vision model format: {path}")
|
||||
|
||||
@classproperty
|
||||
def save_to_config(cls) -> bool:
|
||||
return True
|
||||
|
||||
def get_size(self, child_type: Optional[SubModelType] = None) -> int:
|
||||
if child_type is not None:
|
||||
raise ValueError("There are no child models in a CLIP Vision model.")
|
||||
|
||||
return self.model_size
|
||||
|
||||
def get_model(
|
||||
self,
|
||||
torch_dtype: Optional[torch.dtype],
|
||||
child_type: Optional[SubModelType] = None,
|
||||
) -> CLIPVisionModelWithProjection:
|
||||
if child_type is not None:
|
||||
raise ValueError("There are no child models in a CLIP Vision model.")
|
||||
|
||||
model = CLIPVisionModelWithProjection.from_pretrained(self.model_path, torch_dtype=torch_dtype)
|
||||
|
||||
# Calculate a more accurate model size.
|
||||
self.model_size = calc_model_size_by_data(model)
|
||||
|
||||
return model
|
||||
|
||||
@classmethod
|
||||
def convert_if_required(
|
||||
cls,
|
||||
model_path: str,
|
||||
output_path: str,
|
||||
config: ModelConfigBase,
|
||||
base_model: BaseModelType,
|
||||
) -> str:
|
||||
format = cls.detect_format(model_path)
|
||||
if format == CLIPVisionModelFormat.Diffusers:
|
||||
return model_path
|
||||
else:
|
||||
raise ValueError(f"Unsupported format: '{format}'.")
|
92
invokeai/backend/model_management/models/ip_adapter.py
Normal file
92
invokeai/backend/model_management/models/ip_adapter.py
Normal file
@ -0,0 +1,92 @@
|
||||
import os
|
||||
import typing
|
||||
from enum import Enum
|
||||
from typing import Literal, Optional
|
||||
|
||||
import torch
|
||||
|
||||
from invokeai.backend.ip_adapter.ip_adapter import IPAdapter, IPAdapterPlus, build_ip_adapter
|
||||
from invokeai.backend.model_management.models.base import (
|
||||
BaseModelType,
|
||||
InvalidModelException,
|
||||
ModelBase,
|
||||
ModelConfigBase,
|
||||
ModelType,
|
||||
SubModelType,
|
||||
classproperty,
|
||||
)
|
||||
|
||||
|
||||
class IPAdapterModelFormat(str, Enum):
|
||||
# The custom IP-Adapter model format defined by InvokeAI.
|
||||
InvokeAI = "invokeai"
|
||||
|
||||
|
||||
class IPAdapterModel(ModelBase):
|
||||
class InvokeAIConfig(ModelConfigBase):
|
||||
model_format: Literal[IPAdapterModelFormat.InvokeAI]
|
||||
|
||||
def __init__(self, model_path: str, base_model: BaseModelType, model_type: ModelType):
|
||||
assert model_type == ModelType.IPAdapter
|
||||
super().__init__(model_path, base_model, model_type)
|
||||
|
||||
self.model_size = os.path.getsize(self.model_path)
|
||||
|
||||
@classmethod
|
||||
def detect_format(cls, path: str) -> str:
|
||||
if not os.path.exists(path):
|
||||
raise ModuleNotFoundError(f"No IP-Adapter model at path '{path}'.")
|
||||
|
||||
if os.path.isdir(path):
|
||||
model_file = os.path.join(path, "ip_adapter.bin")
|
||||
image_encoder_config_file = os.path.join(path, "image_encoder.txt")
|
||||
if os.path.exists(model_file) and os.path.exists(image_encoder_config_file):
|
||||
return IPAdapterModelFormat.InvokeAI
|
||||
|
||||
raise InvalidModelException(f"Unexpected IP-Adapter model format: {path}")
|
||||
|
||||
@classproperty
|
||||
def save_to_config(cls) -> bool:
|
||||
return True
|
||||
|
||||
def get_size(self, child_type: Optional[SubModelType] = None) -> int:
|
||||
if child_type is not None:
|
||||
raise ValueError("There are no child models in an IP-Adapter model.")
|
||||
|
||||
return self.model_size
|
||||
|
||||
def get_model(
|
||||
self,
|
||||
torch_dtype: Optional[torch.dtype],
|
||||
child_type: Optional[SubModelType] = None,
|
||||
) -> typing.Union[IPAdapter, IPAdapterPlus]:
|
||||
if child_type is not None:
|
||||
raise ValueError("There are no child models in an IP-Adapter model.")
|
||||
|
||||
return build_ip_adapter(
|
||||
ip_adapter_ckpt_path=os.path.join(self.model_path, "ip_adapter.bin"), device="cpu", dtype=torch_dtype
|
||||
)
|
||||
|
||||
@classmethod
|
||||
def convert_if_required(
|
||||
cls,
|
||||
model_path: str,
|
||||
output_path: str,
|
||||
config: ModelConfigBase,
|
||||
base_model: BaseModelType,
|
||||
) -> str:
|
||||
format = cls.detect_format(model_path)
|
||||
if format == IPAdapterModelFormat.InvokeAI:
|
||||
return model_path
|
||||
else:
|
||||
raise ValueError(f"Unsupported format: '{format}'.")
|
||||
|
||||
|
||||
def get_ip_adapter_image_encoder_model_id(model_path: str):
|
||||
"""Read the ID of the image encoder associated with the IP-Adapter at `model_path`."""
|
||||
image_encoder_config_file = os.path.join(model_path, "image_encoder.txt")
|
||||
|
||||
with open(image_encoder_config_file, "r") as f:
|
||||
image_encoder_model = f.readline().strip()
|
||||
|
||||
return image_encoder_model
|
@ -1,15 +1,6 @@
|
||||
"""
|
||||
Initialization file for the invokeai.backend.stable_diffusion package
|
||||
"""
|
||||
from .diffusers_pipeline import ( # noqa: F401
|
||||
ConditioningData,
|
||||
PipelineIntermediateState,
|
||||
StableDiffusionGeneratorPipeline,
|
||||
)
|
||||
from .diffusers_pipeline import PipelineIntermediateState, StableDiffusionGeneratorPipeline # noqa: F401
|
||||
from .diffusion import InvokeAIDiffuserComponent # noqa: F401
|
||||
from .diffusion.cross_attention_map_saving import AttentionMapSaver # noqa: F401
|
||||
from .diffusion.shared_invokeai_diffusion import ( # noqa: F401
|
||||
BasicConditioningInfo,
|
||||
PostprocessingSettings,
|
||||
SDXLConditioningInfo,
|
||||
)
|
||||
|
@ -1,8 +1,8 @@
|
||||
from __future__ import annotations
|
||||
|
||||
import dataclasses
|
||||
import inspect
|
||||
from dataclasses import dataclass, field
|
||||
import math
|
||||
from contextlib import nullcontext
|
||||
from dataclasses import dataclass
|
||||
from typing import Any, Callable, List, Optional, Union
|
||||
|
||||
import einops
|
||||
@ -23,9 +23,11 @@ from pydantic import Field
|
||||
from transformers import CLIPFeatureExtractor, CLIPTextModel, CLIPTokenizer
|
||||
|
||||
from invokeai.app.services.config import InvokeAIAppConfig
|
||||
from invokeai.backend.ip_adapter.ip_adapter import IPAdapter
|
||||
from invokeai.backend.stable_diffusion.diffusion.conditioning_data import ConditioningData
|
||||
|
||||
from ..util import auto_detect_slice_size, normalize_device
|
||||
from .diffusion import AttentionMapSaver, BasicConditioningInfo, InvokeAIDiffuserComponent, PostprocessingSettings
|
||||
from .diffusion import AttentionMapSaver, InvokeAIDiffuserComponent
|
||||
|
||||
|
||||
@dataclass
|
||||
@ -95,7 +97,7 @@ class AddsMaskGuidance:
|
||||
# Mask anything that has the same shape as prev_sample, return others as-is.
|
||||
return output_class(
|
||||
{
|
||||
k: (self.apply_mask(v, self._t_for_field(k, t)) if are_like_tensors(prev_sample, v) else v)
|
||||
k: self.apply_mask(v, self._t_for_field(k, t)) if are_like_tensors(prev_sample, v) else v
|
||||
for k, v in step_output.items()
|
||||
}
|
||||
)
|
||||
@ -162,39 +164,13 @@ class ControlNetData:
|
||||
|
||||
|
||||
@dataclass
|
||||
class ConditioningData:
|
||||
unconditioned_embeddings: BasicConditioningInfo
|
||||
text_embeddings: BasicConditioningInfo
|
||||
guidance_scale: Union[float, List[float]]
|
||||
"""
|
||||
Guidance scale as defined in [Classifier-Free Diffusion Guidance](https://arxiv.org/abs/2207.12598).
|
||||
`guidance_scale` is defined as `w` of equation 2. of [Imagen Paper](https://arxiv.org/pdf/2205.11487.pdf).
|
||||
Guidance scale is enabled by setting `guidance_scale > 1`. Higher guidance scale encourages to generate
|
||||
images that are closely linked to the text `prompt`, usually at the expense of lower image quality.
|
||||
"""
|
||||
extra: Optional[InvokeAIDiffuserComponent.ExtraConditioningInfo] = None
|
||||
scheduler_args: dict[str, Any] = field(default_factory=dict)
|
||||
"""
|
||||
Additional arguments to pass to invokeai_diffuser.do_latent_postprocessing().
|
||||
"""
|
||||
postprocessing_settings: Optional[PostprocessingSettings] = None
|
||||
|
||||
@property
|
||||
def dtype(self):
|
||||
return self.text_embeddings.dtype
|
||||
|
||||
def add_scheduler_args_if_applicable(self, scheduler, **kwargs):
|
||||
scheduler_args = dict(self.scheduler_args)
|
||||
step_method = inspect.signature(scheduler.step)
|
||||
for name, value in kwargs.items():
|
||||
try:
|
||||
step_method.bind_partial(**{name: value})
|
||||
except TypeError:
|
||||
# FIXME: don't silently discard arguments
|
||||
pass # debug("%s does not accept argument named %r", scheduler, name)
|
||||
else:
|
||||
scheduler_args[name] = value
|
||||
return dataclasses.replace(self, scheduler_args=scheduler_args)
|
||||
class IPAdapterData:
|
||||
ip_adapter_model: IPAdapter = Field(default=None)
|
||||
# TODO: change to polymorphic so can do different weights per step (once implemented...)
|
||||
weight: Union[float, List[float]] = Field(default=1.0)
|
||||
# weight: float = Field(default=1.0)
|
||||
begin_step_percent: float = Field(default=0.0)
|
||||
end_step_percent: float = Field(default=1.0)
|
||||
|
||||
|
||||
@dataclass
|
||||
@ -277,6 +253,7 @@ class StableDiffusionGeneratorPipeline(StableDiffusionPipeline):
|
||||
)
|
||||
self.invokeai_diffuser = InvokeAIDiffuserComponent(self.unet, self._unet_forward)
|
||||
self.control_model = control_model
|
||||
self.use_ip_adapter = False
|
||||
|
||||
def _adjust_memory_efficient_attention(self, latents: torch.Tensor):
|
||||
"""
|
||||
@ -349,6 +326,7 @@ class StableDiffusionGeneratorPipeline(StableDiffusionPipeline):
|
||||
additional_guidance: List[Callable] = None,
|
||||
callback: Callable[[PipelineIntermediateState], None] = None,
|
||||
control_data: List[ControlNetData] = None,
|
||||
ip_adapter_data: Optional[IPAdapterData] = None,
|
||||
mask: Optional[torch.Tensor] = None,
|
||||
masked_latents: Optional[torch.Tensor] = None,
|
||||
seed: Optional[int] = None,
|
||||
@ -400,6 +378,7 @@ class StableDiffusionGeneratorPipeline(StableDiffusionPipeline):
|
||||
conditioning_data,
|
||||
additional_guidance=additional_guidance,
|
||||
control_data=control_data,
|
||||
ip_adapter_data=ip_adapter_data,
|
||||
callback=callback,
|
||||
)
|
||||
finally:
|
||||
@ -419,6 +398,7 @@ class StableDiffusionGeneratorPipeline(StableDiffusionPipeline):
|
||||
*,
|
||||
additional_guidance: List[Callable] = None,
|
||||
control_data: List[ControlNetData] = None,
|
||||
ip_adapter_data: Optional[IPAdapterData] = None,
|
||||
callback: Callable[[PipelineIntermediateState], None] = None,
|
||||
):
|
||||
self._adjust_memory_efficient_attention(latents)
|
||||
@ -431,12 +411,26 @@ class StableDiffusionGeneratorPipeline(StableDiffusionPipeline):
|
||||
if timesteps.shape[0] == 0:
|
||||
return latents, attention_map_saver
|
||||
|
||||
extra_conditioning_info = conditioning_data.extra
|
||||
with self.invokeai_diffuser.custom_attention_context(
|
||||
self.invokeai_diffuser.model,
|
||||
extra_conditioning_info=extra_conditioning_info,
|
||||
step_count=len(self.scheduler.timesteps),
|
||||
):
|
||||
if conditioning_data.extra is not None and conditioning_data.extra.wants_cross_attention_control:
|
||||
attn_ctx = self.invokeai_diffuser.custom_attention_context(
|
||||
self.invokeai_diffuser.model,
|
||||
extra_conditioning_info=conditioning_data.extra,
|
||||
step_count=len(self.scheduler.timesteps),
|
||||
)
|
||||
self.use_ip_adapter = False
|
||||
elif ip_adapter_data is not None:
|
||||
# TODO(ryand): Should we raise an exception if both custom attention and IP-Adapter attention are active?
|
||||
# As it is now, the IP-Adapter will silently be skipped.
|
||||
weight = ip_adapter_data.weight[0] if isinstance(ip_adapter_data.weight, List) else ip_adapter_data.weight
|
||||
attn_ctx = ip_adapter_data.ip_adapter_model.apply_ip_adapter_attention(
|
||||
unet=self.invokeai_diffuser.model,
|
||||
scale=weight,
|
||||
)
|
||||
self.use_ip_adapter = True
|
||||
else:
|
||||
attn_ctx = nullcontext()
|
||||
|
||||
with attn_ctx:
|
||||
if callback is not None:
|
||||
callback(
|
||||
PipelineIntermediateState(
|
||||
@ -459,6 +453,7 @@ class StableDiffusionGeneratorPipeline(StableDiffusionPipeline):
|
||||
total_step_count=len(timesteps),
|
||||
additional_guidance=additional_guidance,
|
||||
control_data=control_data,
|
||||
ip_adapter_data=ip_adapter_data,
|
||||
)
|
||||
latents = step_output.prev_sample
|
||||
|
||||
@ -504,6 +499,7 @@ class StableDiffusionGeneratorPipeline(StableDiffusionPipeline):
|
||||
total_step_count: int,
|
||||
additional_guidance: List[Callable] = None,
|
||||
control_data: List[ControlNetData] = None,
|
||||
ip_adapter_data: Optional[IPAdapterData] = None,
|
||||
):
|
||||
# invokeai_diffuser has batched timesteps, but diffusers schedulers expect a single value
|
||||
timestep = t[0]
|
||||
@ -514,6 +510,24 @@ class StableDiffusionGeneratorPipeline(StableDiffusionPipeline):
|
||||
# i.e. before or after passing it to InvokeAIDiffuserComponent
|
||||
latent_model_input = self.scheduler.scale_model_input(latents, timestep)
|
||||
|
||||
# handle IP-Adapter
|
||||
if self.use_ip_adapter and ip_adapter_data is not None: # somewhat redundant but logic is clearer
|
||||
first_adapter_step = math.floor(ip_adapter_data.begin_step_percent * total_step_count)
|
||||
last_adapter_step = math.ceil(ip_adapter_data.end_step_percent * total_step_count)
|
||||
weight = (
|
||||
ip_adapter_data.weight[step_index]
|
||||
if isinstance(ip_adapter_data.weight, List)
|
||||
else ip_adapter_data.weight
|
||||
)
|
||||
if step_index >= first_adapter_step and step_index <= last_adapter_step:
|
||||
# only apply IP-Adapter if current step is within the IP-Adapter's begin/end step range
|
||||
# ip_adapter_data.ip_adapter_model.set_scale(ip_adapter_data.weight)
|
||||
ip_adapter_data.ip_adapter_model.set_scale(weight)
|
||||
else:
|
||||
# otherwise, set IP-Adapter scale to 0, so it has no effect
|
||||
ip_adapter_data.ip_adapter_model.set_scale(0.0)
|
||||
|
||||
# handle ControlNet(s)
|
||||
# default is no controlnet, so set controlnet processing output to None
|
||||
controlnet_down_block_samples, controlnet_mid_block_sample = None, None
|
||||
if control_data is not None:
|
||||
|
@ -3,9 +3,4 @@ Initialization file for invokeai.models.diffusion
|
||||
"""
|
||||
from .cross_attention_control import InvokeAICrossAttentionMixin # noqa: F401
|
||||
from .cross_attention_map_saving import AttentionMapSaver # noqa: F401
|
||||
from .shared_invokeai_diffusion import ( # noqa: F401
|
||||
BasicConditioningInfo,
|
||||
InvokeAIDiffuserComponent,
|
||||
PostprocessingSettings,
|
||||
SDXLConditioningInfo,
|
||||
)
|
||||
from .shared_invokeai_diffusion import InvokeAIDiffuserComponent # noqa: F401
|
||||
|
101
invokeai/backend/stable_diffusion/diffusion/conditioning_data.py
Normal file
101
invokeai/backend/stable_diffusion/diffusion/conditioning_data.py
Normal file
@ -0,0 +1,101 @@
|
||||
import dataclasses
|
||||
import inspect
|
||||
from dataclasses import dataclass, field
|
||||
from typing import Any, List, Optional, Union
|
||||
|
||||
import torch
|
||||
|
||||
from .cross_attention_control import Arguments
|
||||
|
||||
|
||||
@dataclass
|
||||
class ExtraConditioningInfo:
|
||||
tokens_count_including_eos_bos: int
|
||||
cross_attention_control_args: Optional[Arguments] = None
|
||||
|
||||
@property
|
||||
def wants_cross_attention_control(self):
|
||||
return self.cross_attention_control_args is not None
|
||||
|
||||
|
||||
@dataclass
|
||||
class BasicConditioningInfo:
|
||||
embeds: torch.Tensor
|
||||
# TODO(ryand): Right now we awkwardly copy the extra conditioning info from here up to `ConditioningData`. This
|
||||
# should only be stored in one place.
|
||||
extra_conditioning: Optional[ExtraConditioningInfo]
|
||||
# weight: float
|
||||
# mode: ConditioningAlgo
|
||||
|
||||
def to(self, device, dtype=None):
|
||||
self.embeds = self.embeds.to(device=device, dtype=dtype)
|
||||
return self
|
||||
|
||||
|
||||
@dataclass
|
||||
class SDXLConditioningInfo(BasicConditioningInfo):
|
||||
pooled_embeds: torch.Tensor
|
||||
add_time_ids: torch.Tensor
|
||||
|
||||
def to(self, device, dtype=None):
|
||||
self.pooled_embeds = self.pooled_embeds.to(device=device, dtype=dtype)
|
||||
self.add_time_ids = self.add_time_ids.to(device=device, dtype=dtype)
|
||||
return super().to(device=device, dtype=dtype)
|
||||
|
||||
|
||||
@dataclass(frozen=True)
|
||||
class PostprocessingSettings:
|
||||
threshold: float
|
||||
warmup: float
|
||||
h_symmetry_time_pct: Optional[float]
|
||||
v_symmetry_time_pct: Optional[float]
|
||||
|
||||
|
||||
@dataclass
|
||||
class IPAdapterConditioningInfo:
|
||||
cond_image_prompt_embeds: torch.Tensor
|
||||
"""IP-Adapter image encoder conditioning embeddings.
|
||||
Shape: (batch_size, num_tokens, encoding_dim).
|
||||
"""
|
||||
uncond_image_prompt_embeds: torch.Tensor
|
||||
"""IP-Adapter image encoding embeddings to use for unconditional generation.
|
||||
Shape: (batch_size, num_tokens, encoding_dim).
|
||||
"""
|
||||
|
||||
|
||||
@dataclass
|
||||
class ConditioningData:
|
||||
unconditioned_embeddings: BasicConditioningInfo
|
||||
text_embeddings: BasicConditioningInfo
|
||||
guidance_scale: Union[float, List[float]]
|
||||
"""
|
||||
Guidance scale as defined in [Classifier-Free Diffusion Guidance](https://arxiv.org/abs/2207.12598).
|
||||
`guidance_scale` is defined as `w` of equation 2. of [Imagen Paper](https://arxiv.org/pdf/2205.11487.pdf).
|
||||
Guidance scale is enabled by setting `guidance_scale > 1`. Higher guidance scale encourages to generate
|
||||
images that are closely linked to the text `prompt`, usually at the expense of lower image quality.
|
||||
"""
|
||||
extra: Optional[ExtraConditioningInfo] = None
|
||||
scheduler_args: dict[str, Any] = field(default_factory=dict)
|
||||
"""
|
||||
Additional arguments to pass to invokeai_diffuser.do_latent_postprocessing().
|
||||
"""
|
||||
postprocessing_settings: Optional[PostprocessingSettings] = None
|
||||
|
||||
ip_adapter_conditioning: Optional[IPAdapterConditioningInfo] = None
|
||||
|
||||
@property
|
||||
def dtype(self):
|
||||
return self.text_embeddings.dtype
|
||||
|
||||
def add_scheduler_args_if_applicable(self, scheduler, **kwargs):
|
||||
scheduler_args = dict(self.scheduler_args)
|
||||
step_method = inspect.signature(scheduler.step)
|
||||
for name, value in kwargs.items():
|
||||
try:
|
||||
step_method.bind_partial(**{name: value})
|
||||
except TypeError:
|
||||
# FIXME: don't silently discard arguments
|
||||
pass # debug("%s does not accept argument named %r", scheduler, name)
|
||||
else:
|
||||
scheduler_args[name] = value
|
||||
return dataclasses.replace(self, scheduler_args=scheduler_args)
|
@ -376,11 +376,11 @@ def get_cross_attention_modules(model, which: CrossAttentionType) -> list[tuple[
|
||||
# non-fatal error but .swap() won't work.
|
||||
logger.error(
|
||||
f"Error! CrossAttentionControl found an unexpected number of {cross_attention_class} modules in the model "
|
||||
+ f"(expected {expected_count}, found {cross_attention_modules_in_model_count}). Either monkey-patching failed "
|
||||
+ "or some assumption has changed about the structure of the model itself. Please fix the monkey-patching, "
|
||||
+ f"and/or update the {expected_count} above to an appropriate number, and/or find and inform someone who knows "
|
||||
+ "what it means. This error is non-fatal, but it is likely that .swap() and attention map display will not "
|
||||
+ "work properly until it is fixed."
|
||||
f"(expected {expected_count}, found {cross_attention_modules_in_model_count}). Either monkey-patching "
|
||||
"failed or some assumption has changed about the structure of the model itself. Please fix the "
|
||||
f"monkey-patching, and/or update the {expected_count} above to an appropriate number, and/or find and "
|
||||
"inform someone who knows what it means. This error is non-fatal, but it is likely that .swap() and "
|
||||
"attention map display will not work properly until it is fixed."
|
||||
)
|
||||
return attention_module_tuples
|
||||
|
||||
@ -577,6 +577,7 @@ class SlicedSwapCrossAttnProcesser(SlicedAttnProcessor):
|
||||
attention_mask=None,
|
||||
# kwargs
|
||||
swap_cross_attn_context: SwapCrossAttnContext = None,
|
||||
**kwargs,
|
||||
):
|
||||
attention_type = CrossAttentionType.SELF if encoder_hidden_states is None else CrossAttentionType.TOKENS
|
||||
|
||||
|
@ -2,7 +2,6 @@ from __future__ import annotations
|
||||
|
||||
import math
|
||||
from contextlib import contextmanager
|
||||
from dataclasses import dataclass
|
||||
from typing import Any, Callable, Optional, Union
|
||||
|
||||
import torch
|
||||
@ -10,9 +9,14 @@ from diffusers import UNet2DConditionModel
|
||||
from typing_extensions import TypeAlias
|
||||
|
||||
from invokeai.app.services.config import InvokeAIAppConfig
|
||||
from invokeai.backend.stable_diffusion.diffusion.conditioning_data import (
|
||||
ConditioningData,
|
||||
ExtraConditioningInfo,
|
||||
PostprocessingSettings,
|
||||
SDXLConditioningInfo,
|
||||
)
|
||||
|
||||
from .cross_attention_control import (
|
||||
Arguments,
|
||||
Context,
|
||||
CrossAttentionType,
|
||||
SwapCrossAttnContext,
|
||||
@ -31,37 +35,6 @@ ModelForwardCallback: TypeAlias = Union[
|
||||
]
|
||||
|
||||
|
||||
@dataclass
|
||||
class BasicConditioningInfo:
|
||||
embeds: torch.Tensor
|
||||
extra_conditioning: Optional[InvokeAIDiffuserComponent.ExtraConditioningInfo]
|
||||
# weight: float
|
||||
# mode: ConditioningAlgo
|
||||
|
||||
def to(self, device, dtype=None):
|
||||
self.embeds = self.embeds.to(device=device, dtype=dtype)
|
||||
return self
|
||||
|
||||
|
||||
@dataclass
|
||||
class SDXLConditioningInfo(BasicConditioningInfo):
|
||||
pooled_embeds: torch.Tensor
|
||||
add_time_ids: torch.Tensor
|
||||
|
||||
def to(self, device, dtype=None):
|
||||
self.pooled_embeds = self.pooled_embeds.to(device=device, dtype=dtype)
|
||||
self.add_time_ids = self.add_time_ids.to(device=device, dtype=dtype)
|
||||
return super().to(device=device, dtype=dtype)
|
||||
|
||||
|
||||
@dataclass(frozen=True)
|
||||
class PostprocessingSettings:
|
||||
threshold: float
|
||||
warmup: float
|
||||
h_symmetry_time_pct: Optional[float]
|
||||
v_symmetry_time_pct: Optional[float]
|
||||
|
||||
|
||||
class InvokeAIDiffuserComponent:
|
||||
"""
|
||||
The aim of this component is to provide a single place for code that can be applied identically to
|
||||
@ -75,15 +48,6 @@ class InvokeAIDiffuserComponent:
|
||||
debug_thresholding = False
|
||||
sequential_guidance = False
|
||||
|
||||
@dataclass
|
||||
class ExtraConditioningInfo:
|
||||
tokens_count_including_eos_bos: int
|
||||
cross_attention_control_args: Optional[Arguments] = None
|
||||
|
||||
@property
|
||||
def wants_cross_attention_control(self):
|
||||
return self.cross_attention_control_args is not None
|
||||
|
||||
def __init__(
|
||||
self,
|
||||
model,
|
||||
@ -103,30 +67,26 @@ class InvokeAIDiffuserComponent:
|
||||
@contextmanager
|
||||
def custom_attention_context(
|
||||
self,
|
||||
unet: UNet2DConditionModel, # note: also may futz with the text encoder depending on requested LoRAs
|
||||
unet: UNet2DConditionModel,
|
||||
extra_conditioning_info: Optional[ExtraConditioningInfo],
|
||||
step_count: int,
|
||||
):
|
||||
old_attn_processors = None
|
||||
if extra_conditioning_info and (extra_conditioning_info.wants_cross_attention_control):
|
||||
old_attn_processors = unet.attn_processors
|
||||
# Load lora conditions into the model
|
||||
if extra_conditioning_info.wants_cross_attention_control:
|
||||
self.cross_attention_control_context = Context(
|
||||
arguments=extra_conditioning_info.cross_attention_control_args,
|
||||
step_count=step_count,
|
||||
)
|
||||
setup_cross_attention_control_attention_processors(
|
||||
unet,
|
||||
self.cross_attention_control_context,
|
||||
)
|
||||
old_attn_processors = unet.attn_processors
|
||||
|
||||
try:
|
||||
self.cross_attention_control_context = Context(
|
||||
arguments=extra_conditioning_info.cross_attention_control_args,
|
||||
step_count=step_count,
|
||||
)
|
||||
setup_cross_attention_control_attention_processors(
|
||||
unet,
|
||||
self.cross_attention_control_context,
|
||||
)
|
||||
|
||||
yield None
|
||||
finally:
|
||||
self.cross_attention_control_context = None
|
||||
if old_attn_processors is not None:
|
||||
unet.set_attn_processor(old_attn_processors)
|
||||
unet.set_attn_processor(old_attn_processors)
|
||||
# TODO resuscitate attention map saving
|
||||
# self.remove_attention_map_saving()
|
||||
|
||||
@ -376,11 +336,24 @@ class InvokeAIDiffuserComponent:
|
||||
|
||||
# methods below are called from do_diffusion_step and should be considered private to this class.
|
||||
|
||||
def _apply_standard_conditioning(self, x, sigma, conditioning_data, **kwargs):
|
||||
# fast batched path
|
||||
def _apply_standard_conditioning(self, x, sigma, conditioning_data: ConditioningData, **kwargs):
|
||||
"""Runs the conditioned and unconditioned UNet forward passes in a single batch for faster inference speed at
|
||||
the cost of higher memory usage.
|
||||
"""
|
||||
x_twice = torch.cat([x] * 2)
|
||||
sigma_twice = torch.cat([sigma] * 2)
|
||||
|
||||
cross_attention_kwargs = None
|
||||
if conditioning_data.ip_adapter_conditioning is not None:
|
||||
cross_attention_kwargs = {
|
||||
"ip_adapter_image_prompt_embeds": torch.cat(
|
||||
[
|
||||
conditioning_data.ip_adapter_conditioning.uncond_image_prompt_embeds,
|
||||
conditioning_data.ip_adapter_conditioning.cond_image_prompt_embeds,
|
||||
]
|
||||
)
|
||||
}
|
||||
|
||||
added_cond_kwargs = None
|
||||
if type(conditioning_data.text_embeddings) is SDXLConditioningInfo:
|
||||
added_cond_kwargs = {
|
||||
@ -408,6 +381,7 @@ class InvokeAIDiffuserComponent:
|
||||
x_twice,
|
||||
sigma_twice,
|
||||
both_conditionings,
|
||||
cross_attention_kwargs=cross_attention_kwargs,
|
||||
encoder_attention_mask=encoder_attention_mask,
|
||||
added_cond_kwargs=added_cond_kwargs,
|
||||
**kwargs,
|
||||
@ -419,9 +393,12 @@ class InvokeAIDiffuserComponent:
|
||||
self,
|
||||
x: torch.Tensor,
|
||||
sigma,
|
||||
conditioning_data,
|
||||
conditioning_data: ConditioningData,
|
||||
**kwargs,
|
||||
):
|
||||
"""Runs the conditioned and unconditioned UNet forward passes sequentially for lower memory usage at the cost of
|
||||
slower execution speed.
|
||||
"""
|
||||
# low-memory sequential path
|
||||
uncond_down_block, cond_down_block = None, None
|
||||
down_block_additional_residuals = kwargs.pop("down_block_additional_residuals", None)
|
||||
@ -437,6 +414,13 @@ class InvokeAIDiffuserComponent:
|
||||
if mid_block_additional_residual is not None:
|
||||
uncond_mid_block, cond_mid_block = mid_block_additional_residual.chunk(2)
|
||||
|
||||
# Run unconditional UNet denoising.
|
||||
cross_attention_kwargs = None
|
||||
if conditioning_data.ip_adapter_conditioning is not None:
|
||||
cross_attention_kwargs = {
|
||||
"ip_adapter_image_prompt_embeds": conditioning_data.ip_adapter_conditioning.uncond_image_prompt_embeds
|
||||
}
|
||||
|
||||
added_cond_kwargs = None
|
||||
is_sdxl = type(conditioning_data.text_embeddings) is SDXLConditioningInfo
|
||||
if is_sdxl:
|
||||
@ -449,12 +433,21 @@ class InvokeAIDiffuserComponent:
|
||||
x,
|
||||
sigma,
|
||||
conditioning_data.unconditioned_embeddings.embeds,
|
||||
cross_attention_kwargs=cross_attention_kwargs,
|
||||
down_block_additional_residuals=uncond_down_block,
|
||||
mid_block_additional_residual=uncond_mid_block,
|
||||
added_cond_kwargs=added_cond_kwargs,
|
||||
**kwargs,
|
||||
)
|
||||
|
||||
# Run conditional UNet denoising.
|
||||
cross_attention_kwargs = None
|
||||
if conditioning_data.ip_adapter_conditioning is not None:
|
||||
cross_attention_kwargs = {
|
||||
"ip_adapter_image_prompt_embeds": conditioning_data.ip_adapter_conditioning.cond_image_prompt_embeds
|
||||
}
|
||||
|
||||
added_cond_kwargs = None
|
||||
if is_sdxl:
|
||||
added_cond_kwargs = {
|
||||
"text_embeds": conditioning_data.text_embeddings.pooled_embeds,
|
||||
@ -465,6 +458,7 @@ class InvokeAIDiffuserComponent:
|
||||
x,
|
||||
sigma,
|
||||
conditioning_data.text_embeddings.embeds,
|
||||
cross_attention_kwargs=cross_attention_kwargs,
|
||||
down_block_additional_residuals=cond_down_block,
|
||||
mid_block_additional_residual=cond_mid_block,
|
||||
added_cond_kwargs=added_cond_kwargs,
|
||||
|
169
invokeai/frontend/web/dist/assets/App-d1567775.js
vendored
169
invokeai/frontend/web/dist/assets/App-d1567775.js
vendored
File diff suppressed because one or more lines are too long
169
invokeai/frontend/web/dist/assets/App-dbf8f111.js
vendored
Normal file
169
invokeai/frontend/web/dist/assets/App-dbf8f111.js
vendored
Normal file
File diff suppressed because one or more lines are too long
@ -1,4 +1,4 @@
|
||||
import{v as m,h5 as Je,u as y,Y as Xa,h6 as Ja,a7 as ua,ab as d,h7 as b,h8 as o,h9 as Qa,ha as h,hb as fa,hc as Za,hd as eo,aE as ro,he as ao,a4 as oo,hf as to}from"./index-f83c2c5c.js";import{s as ha,n as t,t as io,o as ma,p as no,q as ga,v as ya,w as pa,x as lo,y as Sa,z as xa,A as xr,B as so,D as co,E as bo,F as $a,G as ka,H as _a,J as vo,K as wa,L as uo,M as fo,N as ho,O as mo,Q as za,R as go,S as yo,T as po,U as So,V as xo,W as $o,e as ko,X as _o}from"./menu-31376327.js";var Ca=String.raw,Aa=Ca`
|
||||
import{v as m,hj as Je,u as y,Y as Xa,hk as Ja,a7 as ua,ab as d,hl as b,hm as o,hn as Qa,ho as h,hp as fa,hq as Za,hr as eo,aE as ro,hs as ao,a4 as oo,ht as to}from"./index-f6c3f475.js";import{s as ha,n as t,t as io,o as ma,p as no,q as ga,v as ya,w as pa,x as lo,y as Sa,z as xa,A as xr,B as so,D as co,E as bo,F as $a,G as ka,H as _a,J as vo,K as wa,L as uo,M as fo,N as ho,O as mo,Q as za,R as go,S as yo,T as po,U as So,V as xo,W as $o,e as ko,X as _o}from"./menu-c9cc8c3d.js";var Ca=String.raw,Aa=Ca`
|
||||
:root,
|
||||
:host {
|
||||
--chakra-vh: 100vh;
|
128
invokeai/frontend/web/dist/assets/index-f6c3f475.js
vendored
Normal file
128
invokeai/frontend/web/dist/assets/index-f6c3f475.js
vendored
Normal file
File diff suppressed because one or more lines are too long
128
invokeai/frontend/web/dist/assets/index-f83c2c5c.js
vendored
128
invokeai/frontend/web/dist/assets/index-f83c2c5c.js
vendored
File diff suppressed because one or more lines are too long
File diff suppressed because one or more lines are too long
2
invokeai/frontend/web/dist/index.html
vendored
2
invokeai/frontend/web/dist/index.html
vendored
@ -12,7 +12,7 @@
|
||||
margin: 0;
|
||||
}
|
||||
</style>
|
||||
<script type="module" crossorigin src="./assets/index-f83c2c5c.js"></script>
|
||||
<script type="module" crossorigin src="./assets/index-f6c3f475.js"></script>
|
||||
</head>
|
||||
|
||||
<body dir="ltr">
|
||||
|
1588
invokeai/frontend/web/dist/locales/en.json
vendored
1588
invokeai/frontend/web/dist/locales/en.json
vendored
File diff suppressed because it is too large
Load Diff
@ -49,6 +49,7 @@
|
||||
"close": "Close",
|
||||
"communityLabel": "Community",
|
||||
"controlNet": "Controlnet",
|
||||
"ipAdapter": "IP Adapter",
|
||||
"darkMode": "Dark Mode",
|
||||
"discordLabel": "Discord",
|
||||
"dontAskMeAgain": "Don't ask me again",
|
||||
@ -191,7 +192,11 @@
|
||||
"showAdvanced": "Show Advanced",
|
||||
"toggleControlNet": "Toggle this ControlNet",
|
||||
"w": "W",
|
||||
"weight": "Weight"
|
||||
"weight": "Weight",
|
||||
"enableIPAdapter": "Enable IP Adapter",
|
||||
"ipAdapterModel": "Adapter Model",
|
||||
"resetIPAdapterImage": "Reset IP Adapter Image",
|
||||
"ipAdapterImageFallback": "No IP Adapter Image Selected"
|
||||
},
|
||||
"embedding": {
|
||||
"addEmbedding": "Add Embedding",
|
||||
@ -1036,6 +1041,7 @@
|
||||
"serverError": "Server Error",
|
||||
"setCanvasInitialImage": "Set as canvas initial image",
|
||||
"setControlImage": "Set as control image",
|
||||
"setIPAdapterImage": "Set as IP Adapter Image",
|
||||
"setInitialImage": "Set as initial image",
|
||||
"setNodeField": "Set as node field",
|
||||
"tempFoldersEmptied": "Temp Folder Emptied",
|
||||
|
@ -1,5 +1,8 @@
|
||||
import { resetCanvas } from 'features/canvas/store/canvasSlice';
|
||||
import { controlNetReset } from 'features/controlNet/store/controlNetSlice';
|
||||
import {
|
||||
controlNetReset,
|
||||
ipAdapterStateReset,
|
||||
} from 'features/controlNet/store/controlNetSlice';
|
||||
import { getImageUsage } from 'features/deleteImageModal/store/selectors';
|
||||
import { nodeEditorReset } from 'features/nodes/store/nodesSlice';
|
||||
import { clearInitialImage } from 'features/parameters/store/generationSlice';
|
||||
@ -18,6 +21,7 @@ export const addDeleteBoardAndImagesFulfilledListener = () => {
|
||||
let wasCanvasReset = false;
|
||||
let wasNodeEditorReset = false;
|
||||
let wasControlNetReset = false;
|
||||
let wasIPAdapterReset = false;
|
||||
|
||||
const state = getState();
|
||||
deleted_images.forEach((image_name) => {
|
||||
@ -42,6 +46,11 @@ export const addDeleteBoardAndImagesFulfilledListener = () => {
|
||||
dispatch(controlNetReset());
|
||||
wasControlNetReset = true;
|
||||
}
|
||||
|
||||
if (imageUsage.isIPAdapterImage && !wasIPAdapterReset) {
|
||||
dispatch(ipAdapterStateReset());
|
||||
wasIPAdapterReset = true;
|
||||
}
|
||||
});
|
||||
},
|
||||
});
|
||||
|
@ -3,6 +3,7 @@ import { resetCanvas } from 'features/canvas/store/canvasSlice';
|
||||
import {
|
||||
controlNetImageChanged,
|
||||
controlNetProcessedImageChanged,
|
||||
ipAdapterImageChanged,
|
||||
} from 'features/controlNet/store/controlNetSlice';
|
||||
import { imageDeletionConfirmed } from 'features/deleteImageModal/store/actions';
|
||||
import { isModalOpenChanged } from 'features/deleteImageModal/store/slice';
|
||||
@ -110,6 +111,14 @@ export const addRequestedSingleImageDeletionListener = () => {
|
||||
}
|
||||
});
|
||||
|
||||
// Remove IP Adapter Set Image if image is deleted.
|
||||
if (
|
||||
getState().controlNet.ipAdapterInfo.adapterImage?.image_name ===
|
||||
imageDTO.image_name
|
||||
) {
|
||||
dispatch(ipAdapterImageChanged(null));
|
||||
}
|
||||
|
||||
// reset nodes that use the deleted images
|
||||
getState().nodes.nodes.forEach((node) => {
|
||||
if (!isInvocationNode(node)) {
|
||||
@ -227,6 +236,14 @@ export const addRequestedMultipleImageDeletionListener = () => {
|
||||
}
|
||||
});
|
||||
|
||||
// Remove IP Adapter Set Image if image is deleted.
|
||||
if (
|
||||
getState().controlNet.ipAdapterInfo.adapterImage?.image_name ===
|
||||
imageDTO.image_name
|
||||
) {
|
||||
dispatch(ipAdapterImageChanged(null));
|
||||
}
|
||||
|
||||
// reset nodes that use the deleted images
|
||||
getState().nodes.nodes.forEach((node) => {
|
||||
if (!isInvocationNode(node)) {
|
||||
|
@ -1,7 +1,11 @@
|
||||
import { createAction } from '@reduxjs/toolkit';
|
||||
import { logger } from 'app/logging/logger';
|
||||
import { parseify } from 'common/util/serialize';
|
||||
import { setInitialCanvasImage } from 'features/canvas/store/canvasSlice';
|
||||
import { controlNetImageChanged } from 'features/controlNet/store/controlNetSlice';
|
||||
import {
|
||||
controlNetImageChanged,
|
||||
ipAdapterImageChanged,
|
||||
} from 'features/controlNet/store/controlNetSlice';
|
||||
import {
|
||||
TypesafeDraggableData,
|
||||
TypesafeDroppableData,
|
||||
@ -14,7 +18,6 @@ import {
|
||||
import { initialImageChanged } from 'features/parameters/store/generationSlice';
|
||||
import { imagesApi } from 'services/api/endpoints/images';
|
||||
import { startAppListening } from '../';
|
||||
import { parseify } from 'common/util/serialize';
|
||||
|
||||
export const dndDropped = createAction<{
|
||||
overData: TypesafeDroppableData;
|
||||
@ -99,6 +102,18 @@ export const addImageDroppedListener = () => {
|
||||
return;
|
||||
}
|
||||
|
||||
/**
|
||||
* Image dropped on IP Adapter image
|
||||
*/
|
||||
if (
|
||||
overData.actionType === 'SET_IP_ADAPTER_IMAGE' &&
|
||||
activeData.payloadType === 'IMAGE_DTO' &&
|
||||
activeData.payload.imageDTO
|
||||
) {
|
||||
dispatch(ipAdapterImageChanged(activeData.payload.imageDTO));
|
||||
return;
|
||||
}
|
||||
|
||||
/**
|
||||
* Image dropped on Canvas
|
||||
*/
|
||||
|
@ -19,6 +19,7 @@ export const addImageToDeleteSelectedListener = () => {
|
||||
imagesUsage.some((i) => i.isCanvasImage) ||
|
||||
imagesUsage.some((i) => i.isInitialImage) ||
|
||||
imagesUsage.some((i) => i.isControlNetImage) ||
|
||||
imagesUsage.some((i) => i.isIPAdapterImage) ||
|
||||
imagesUsage.some((i) => i.isNodesImage);
|
||||
|
||||
if (shouldConfirmOnDelete || isImageInUse) {
|
||||
|
@ -1,15 +1,18 @@
|
||||
import { UseToastOptions } from '@chakra-ui/react';
|
||||
import { logger } from 'app/logging/logger';
|
||||
import { setInitialCanvasImage } from 'features/canvas/store/canvasSlice';
|
||||
import { controlNetImageChanged } from 'features/controlNet/store/controlNetSlice';
|
||||
import {
|
||||
controlNetImageChanged,
|
||||
ipAdapterImageChanged,
|
||||
} from 'features/controlNet/store/controlNetSlice';
|
||||
import { fieldImageValueChanged } from 'features/nodes/store/nodesSlice';
|
||||
import { initialImageChanged } from 'features/parameters/store/generationSlice';
|
||||
import { addToast } from 'features/system/store/systemSlice';
|
||||
import { t } from 'i18next';
|
||||
import { omit } from 'lodash-es';
|
||||
import { boardsApi } from 'services/api/endpoints/boards';
|
||||
import { startAppListening } from '..';
|
||||
import { imagesApi } from '../../../../../services/api/endpoints/images';
|
||||
import { t } from 'i18next';
|
||||
|
||||
const DEFAULT_UPLOADED_TOAST: UseToastOptions = {
|
||||
title: t('toast.imageUploaded'),
|
||||
@ -99,6 +102,17 @@ export const addImageUploadedFulfilledListener = () => {
|
||||
return;
|
||||
}
|
||||
|
||||
if (postUploadAction?.type === 'SET_IP_ADAPTER_IMAGE') {
|
||||
dispatch(ipAdapterImageChanged(imageDTO));
|
||||
dispatch(
|
||||
addToast({
|
||||
...DEFAULT_UPLOADED_TOAST,
|
||||
description: t('toast.setIPAdapterImage'),
|
||||
})
|
||||
);
|
||||
return;
|
||||
}
|
||||
|
||||
if (postUploadAction?.type === 'SET_INITIAL_IMAGE') {
|
||||
dispatch(initialImageChanged(imageDTO));
|
||||
dispatch(
|
||||
|
@ -1,6 +1,9 @@
|
||||
import { logger } from 'app/logging/logger';
|
||||
import { setBoundingBoxDimensions } from 'features/canvas/store/canvasSlice';
|
||||
import { controlNetRemoved } from 'features/controlNet/store/controlNetSlice';
|
||||
import {
|
||||
controlNetRemoved,
|
||||
ipAdapterStateReset,
|
||||
} from 'features/controlNet/store/controlNetSlice';
|
||||
import { loraRemoved } from 'features/lora/store/loraSlice';
|
||||
import { modelSelected } from 'features/parameters/store/actions';
|
||||
import {
|
||||
@ -56,6 +59,7 @@ export const addModelSelectedListener = () => {
|
||||
modelsCleared += 1;
|
||||
}
|
||||
|
||||
// handle incompatible controlnets
|
||||
const { controlNets } = state.controlNet;
|
||||
forEach(controlNets, (controlNet, controlNetId) => {
|
||||
if (controlNet.model?.base_model !== base_model) {
|
||||
@ -64,6 +68,16 @@ export const addModelSelectedListener = () => {
|
||||
}
|
||||
});
|
||||
|
||||
// handle incompatible IP-Adapter
|
||||
const { ipAdapterInfo } = state.controlNet;
|
||||
if (
|
||||
ipAdapterInfo.model &&
|
||||
ipAdapterInfo.model.base_model !== base_model
|
||||
) {
|
||||
dispatch(ipAdapterStateReset());
|
||||
modelsCleared += 1;
|
||||
}
|
||||
|
||||
if (modelsCleared > 0) {
|
||||
dispatch(
|
||||
addToast(
|
||||
|
@ -86,7 +86,10 @@ export const store = configureStore({
|
||||
.concat(autoBatchEnhancer());
|
||||
},
|
||||
middleware: (getDefaultMiddleware) =>
|
||||
getDefaultMiddleware({ immutableCheck: false })
|
||||
getDefaultMiddleware({
|
||||
serializableCheck: false,
|
||||
immutableCheck: false,
|
||||
})
|
||||
.concat(api.middleware)
|
||||
.concat(dynamicMiddlewares)
|
||||
.prepend(listenerMiddleware.middleware),
|
||||
|
@ -18,6 +18,7 @@ import { defaultSelectorOptions } from 'app/store/util/defaultMemoizeOptions';
|
||||
import IAIIconButton from 'common/components/IAIIconButton';
|
||||
import IAISwitch from 'common/components/IAISwitch';
|
||||
import { activeTabNameSelector } from 'features/ui/store/uiSelectors';
|
||||
import { useTranslation } from 'react-i18next';
|
||||
import { useToggle } from 'react-use';
|
||||
import { v4 as uuidv4 } from 'uuid';
|
||||
import ControlNetImagePreview from './ControlNetImagePreview';
|
||||
@ -28,7 +29,6 @@ import ParamControlNetBeginEnd from './parameters/ParamControlNetBeginEnd';
|
||||
import ParamControlNetControlMode from './parameters/ParamControlNetControlMode';
|
||||
import ParamControlNetProcessorSelect from './parameters/ParamControlNetProcessorSelect';
|
||||
import ParamControlNetResizeMode from './parameters/ParamControlNetResizeMode';
|
||||
import { useTranslation } from 'react-i18next';
|
||||
|
||||
type ControlNetProps = {
|
||||
controlNet: ControlNetConfig;
|
||||
|
@ -0,0 +1,35 @@
|
||||
import { Flex } from '@chakra-ui/react';
|
||||
import { memo } from 'react';
|
||||
import ParamIPAdapterBeginEnd from './ParamIPAdapterBeginEnd';
|
||||
import ParamIPAdapterFeatureToggle from './ParamIPAdapterFeatureToggle';
|
||||
import ParamIPAdapterImage from './ParamIPAdapterImage';
|
||||
import ParamIPAdapterModelSelect from './ParamIPAdapterModelSelect';
|
||||
import ParamIPAdapterWeight from './ParamIPAdapterWeight';
|
||||
|
||||
const IPAdapterPanel = () => {
|
||||
return (
|
||||
<Flex
|
||||
sx={{
|
||||
flexDir: 'column',
|
||||
gap: 3,
|
||||
paddingInline: 3,
|
||||
paddingBlock: 2,
|
||||
paddingBottom: 5,
|
||||
borderRadius: 'base',
|
||||
position: 'relative',
|
||||
bg: 'base.250',
|
||||
_dark: {
|
||||
bg: 'base.750',
|
||||
},
|
||||
}}
|
||||
>
|
||||
<ParamIPAdapterFeatureToggle />
|
||||
<ParamIPAdapterImage />
|
||||
<ParamIPAdapterModelSelect />
|
||||
<ParamIPAdapterWeight />
|
||||
<ParamIPAdapterBeginEnd />
|
||||
</Flex>
|
||||
);
|
||||
};
|
||||
|
||||
export default memo(IPAdapterPanel);
|
@ -0,0 +1,100 @@
|
||||
import {
|
||||
FormControl,
|
||||
FormLabel,
|
||||
HStack,
|
||||
RangeSlider,
|
||||
RangeSliderFilledTrack,
|
||||
RangeSliderMark,
|
||||
RangeSliderThumb,
|
||||
RangeSliderTrack,
|
||||
Tooltip,
|
||||
} from '@chakra-ui/react';
|
||||
import { RootState } from 'app/store/store';
|
||||
import { useAppDispatch, useAppSelector } from 'app/store/storeHooks';
|
||||
import {
|
||||
ipAdapterBeginStepPctChanged,
|
||||
ipAdapterEndStepPctChanged,
|
||||
} from 'features/controlNet/store/controlNetSlice';
|
||||
import { memo, useCallback } from 'react';
|
||||
import { useTranslation } from 'react-i18next';
|
||||
|
||||
const formatPct = (v: number) => `${Math.round(v * 100)}%`;
|
||||
|
||||
const ParamIPAdapterBeginEnd = () => {
|
||||
const isEnabled = useAppSelector(
|
||||
(state: RootState) => state.controlNet.isIPAdapterEnabled
|
||||
);
|
||||
const beginStepPct = useAppSelector(
|
||||
(state: RootState) => state.controlNet.ipAdapterInfo.beginStepPct
|
||||
);
|
||||
const endStepPct = useAppSelector(
|
||||
(state: RootState) => state.controlNet.ipAdapterInfo.endStepPct
|
||||
);
|
||||
const dispatch = useAppDispatch();
|
||||
const { t } = useTranslation();
|
||||
|
||||
const handleStepPctChanged = useCallback(
|
||||
(v: number[]) => {
|
||||
dispatch(ipAdapterBeginStepPctChanged(v[0] as number));
|
||||
dispatch(ipAdapterEndStepPctChanged(v[1] as number));
|
||||
},
|
||||
[dispatch]
|
||||
);
|
||||
|
||||
return (
|
||||
<FormControl isDisabled={!isEnabled}>
|
||||
<FormLabel>{t('controlnet.beginEndStepPercent')}</FormLabel>
|
||||
<HStack w="100%" gap={2} alignItems="center">
|
||||
<RangeSlider
|
||||
aria-label={['Begin Step %', 'End Step %!']}
|
||||
value={[beginStepPct, endStepPct]}
|
||||
onChange={handleStepPctChanged}
|
||||
min={0}
|
||||
max={1}
|
||||
step={0.01}
|
||||
minStepsBetweenThumbs={5}
|
||||
isDisabled={!isEnabled}
|
||||
>
|
||||
<RangeSliderTrack>
|
||||
<RangeSliderFilledTrack />
|
||||
</RangeSliderTrack>
|
||||
<Tooltip label={formatPct(beginStepPct)} placement="top" hasArrow>
|
||||
<RangeSliderThumb index={0} />
|
||||
</Tooltip>
|
||||
<Tooltip label={formatPct(endStepPct)} placement="top" hasArrow>
|
||||
<RangeSliderThumb index={1} />
|
||||
</Tooltip>
|
||||
<RangeSliderMark
|
||||
value={0}
|
||||
sx={{
|
||||
insetInlineStart: '0 !important',
|
||||
insetInlineEnd: 'unset !important',
|
||||
}}
|
||||
>
|
||||
0%
|
||||
</RangeSliderMark>
|
||||
<RangeSliderMark
|
||||
value={0.5}
|
||||
sx={{
|
||||
insetInlineStart: '50% !important',
|
||||
transform: 'translateX(-50%)',
|
||||
}}
|
||||
>
|
||||
50%
|
||||
</RangeSliderMark>
|
||||
<RangeSliderMark
|
||||
value={1}
|
||||
sx={{
|
||||
insetInlineStart: 'unset !important',
|
||||
insetInlineEnd: '0 !important',
|
||||
}}
|
||||
>
|
||||
100%
|
||||
</RangeSliderMark>
|
||||
</RangeSlider>
|
||||
</HStack>
|
||||
</FormControl>
|
||||
);
|
||||
};
|
||||
|
||||
export default memo(ParamIPAdapterBeginEnd);
|
@ -0,0 +1,41 @@
|
||||
import { createSelector } from '@reduxjs/toolkit';
|
||||
import { stateSelector } from 'app/store/store';
|
||||
import { useAppDispatch, useAppSelector } from 'app/store/storeHooks';
|
||||
import { defaultSelectorOptions } from 'app/store/util/defaultMemoizeOptions';
|
||||
import IAISwitch from 'common/components/IAISwitch';
|
||||
import { isIPAdapterEnableToggled } from 'features/controlNet/store/controlNetSlice';
|
||||
import { memo, useCallback } from 'react';
|
||||
import { useTranslation } from 'react-i18next';
|
||||
|
||||
const selector = createSelector(
|
||||
stateSelector,
|
||||
(state) => {
|
||||
const { isIPAdapterEnabled } = state.controlNet;
|
||||
|
||||
return { isIPAdapterEnabled };
|
||||
},
|
||||
defaultSelectorOptions
|
||||
);
|
||||
|
||||
const ParamIPAdapterFeatureToggle = () => {
|
||||
const { isIPAdapterEnabled } = useAppSelector(selector);
|
||||
const dispatch = useAppDispatch();
|
||||
const { t } = useTranslation();
|
||||
|
||||
const handleChange = useCallback(() => {
|
||||
dispatch(isIPAdapterEnableToggled());
|
||||
}, [dispatch]);
|
||||
|
||||
return (
|
||||
<IAISwitch
|
||||
label={t('controlnet.enableIPAdapter')}
|
||||
isChecked={isIPAdapterEnabled}
|
||||
onChange={handleChange}
|
||||
formControlProps={{
|
||||
width: '100%',
|
||||
}}
|
||||
/>
|
||||
);
|
||||
};
|
||||
|
||||
export default memo(ParamIPAdapterFeatureToggle);
|
@ -0,0 +1,93 @@
|
||||
import { Flex } from '@chakra-ui/react';
|
||||
import { skipToken } from '@reduxjs/toolkit/dist/query';
|
||||
import { RootState } from 'app/store/store';
|
||||
import { useAppDispatch, useAppSelector } from 'app/store/storeHooks';
|
||||
import IAIDndImage from 'common/components/IAIDndImage';
|
||||
import IAIDndImageIcon from 'common/components/IAIDndImageIcon';
|
||||
import { IAINoContentFallback } from 'common/components/IAIImageFallback';
|
||||
import { ipAdapterImageChanged } from 'features/controlNet/store/controlNetSlice';
|
||||
import {
|
||||
TypesafeDraggableData,
|
||||
TypesafeDroppableData,
|
||||
} from 'features/dnd/types';
|
||||
import { memo, useMemo } from 'react';
|
||||
import { useTranslation } from 'react-i18next';
|
||||
import { FaUndo } from 'react-icons/fa';
|
||||
import { useGetImageDTOQuery } from 'services/api/endpoints/images';
|
||||
import { PostUploadAction } from 'services/api/types';
|
||||
|
||||
const ParamIPAdapterImage = () => {
|
||||
const ipAdapterInfo = useAppSelector(
|
||||
(state: RootState) => state.controlNet.ipAdapterInfo
|
||||
);
|
||||
|
||||
const isIPAdapterEnabled = useAppSelector(
|
||||
(state: RootState) => state.controlNet.isIPAdapterEnabled
|
||||
);
|
||||
|
||||
const dispatch = useAppDispatch();
|
||||
const { t } = useTranslation();
|
||||
|
||||
const { currentData: imageDTO } = useGetImageDTOQuery(
|
||||
ipAdapterInfo.adapterImage?.image_name ?? skipToken
|
||||
);
|
||||
|
||||
const draggableData = useMemo<TypesafeDraggableData | undefined>(() => {
|
||||
if (imageDTO) {
|
||||
return {
|
||||
id: 'ip-adapter-image',
|
||||
payloadType: 'IMAGE_DTO',
|
||||
payload: { imageDTO },
|
||||
};
|
||||
}
|
||||
}, [imageDTO]);
|
||||
|
||||
const droppableData = useMemo<TypesafeDroppableData | undefined>(
|
||||
() => ({
|
||||
id: 'ip-adapter-image',
|
||||
actionType: 'SET_IP_ADAPTER_IMAGE',
|
||||
}),
|
||||
[]
|
||||
);
|
||||
|
||||
const postUploadAction = useMemo<PostUploadAction>(
|
||||
() => ({
|
||||
type: 'SET_IP_ADAPTER_IMAGE',
|
||||
}),
|
||||
[]
|
||||
);
|
||||
|
||||
return (
|
||||
<Flex
|
||||
sx={{
|
||||
position: 'relative',
|
||||
w: 'full',
|
||||
alignItems: 'center',
|
||||
justifyContent: 'center',
|
||||
}}
|
||||
>
|
||||
<IAIDndImage
|
||||
imageDTO={imageDTO}
|
||||
droppableData={droppableData}
|
||||
draggableData={draggableData}
|
||||
postUploadAction={postUploadAction}
|
||||
isUploadDisabled={!isIPAdapterEnabled}
|
||||
isDropDisabled={!isIPAdapterEnabled}
|
||||
dropLabel={t('toast.setIPAdapterImage')}
|
||||
noContentFallback={
|
||||
<IAINoContentFallback
|
||||
label={t('controlnet.ipAdapterImageFallback')}
|
||||
/>
|
||||
}
|
||||
/>
|
||||
|
||||
<IAIDndImageIcon
|
||||
onClick={() => dispatch(ipAdapterImageChanged(null))}
|
||||
icon={ipAdapterInfo.adapterImage ? <FaUndo /> : undefined}
|
||||
tooltip={t('controlnet.resetIPAdapterImage')}
|
||||
/>
|
||||
</Flex>
|
||||
);
|
||||
};
|
||||
|
||||
export default memo(ParamIPAdapterImage);
|
@ -0,0 +1,97 @@
|
||||
import { SelectItem } from '@mantine/core';
|
||||
import { RootState } from 'app/store/store';
|
||||
import { useAppDispatch, useAppSelector } from 'app/store/storeHooks';
|
||||
import IAIMantineSelect from 'common/components/IAIMantineSelect';
|
||||
import { ipAdapterModelChanged } from 'features/controlNet/store/controlNetSlice';
|
||||
import { MODEL_TYPE_MAP } from 'features/parameters/types/constants';
|
||||
import { modelIdToIPAdapterModelParam } from 'features/parameters/util/modelIdToIPAdapterModelParams';
|
||||
import { forEach } from 'lodash-es';
|
||||
import { memo, useCallback, useMemo } from 'react';
|
||||
import { useTranslation } from 'react-i18next';
|
||||
import { useGetIPAdapterModelsQuery } from 'services/api/endpoints/models';
|
||||
|
||||
const ParamIPAdapterModelSelect = () => {
|
||||
const ipAdapterModel = useAppSelector(
|
||||
(state: RootState) => state.controlNet.ipAdapterInfo.model
|
||||
);
|
||||
const model = useAppSelector((state: RootState) => state.generation.model);
|
||||
|
||||
const dispatch = useAppDispatch();
|
||||
const { t } = useTranslation();
|
||||
|
||||
const { data: ipAdapterModels } = useGetIPAdapterModelsQuery();
|
||||
|
||||
// grab the full model entity from the RTK Query cache
|
||||
const selectedModel = useMemo(
|
||||
() =>
|
||||
ipAdapterModels?.entities[
|
||||
`${ipAdapterModel?.base_model}/ip_adapter/${ipAdapterModel?.model_name}`
|
||||
] ?? null,
|
||||
[
|
||||
ipAdapterModel?.base_model,
|
||||
ipAdapterModel?.model_name,
|
||||
ipAdapterModels?.entities,
|
||||
]
|
||||
);
|
||||
|
||||
const data = useMemo(() => {
|
||||
if (!ipAdapterModels) {
|
||||
return [];
|
||||
}
|
||||
|
||||
const data: SelectItem[] = [];
|
||||
|
||||
forEach(ipAdapterModels.entities, (ipAdapterModel, id) => {
|
||||
if (!ipAdapterModel) {
|
||||
return;
|
||||
}
|
||||
|
||||
const disabled = model?.base_model !== ipAdapterModel.base_model;
|
||||
|
||||
data.push({
|
||||
value: id,
|
||||
label: ipAdapterModel.model_name,
|
||||
group: MODEL_TYPE_MAP[ipAdapterModel.base_model],
|
||||
disabled,
|
||||
tooltip: disabled
|
||||
? `Incompatible base model: ${ipAdapterModel.base_model}`
|
||||
: undefined,
|
||||
});
|
||||
});
|
||||
|
||||
return data.sort((a, b) => (a.disabled && !b.disabled ? 1 : -1));
|
||||
}, [ipAdapterModels, model?.base_model]);
|
||||
|
||||
const handleValueChanged = useCallback(
|
||||
(v: string | null) => {
|
||||
if (!v) {
|
||||
return;
|
||||
}
|
||||
|
||||
const newIPAdapterModel = modelIdToIPAdapterModelParam(v);
|
||||
|
||||
if (!newIPAdapterModel) {
|
||||
return;
|
||||
}
|
||||
|
||||
dispatch(ipAdapterModelChanged(newIPAdapterModel));
|
||||
},
|
||||
[dispatch]
|
||||
);
|
||||
|
||||
return (
|
||||
<IAIMantineSelect
|
||||
label={t('controlnet.ipAdapterModel')}
|
||||
className="nowheel nodrag"
|
||||
tooltip={selectedModel?.description}
|
||||
value={selectedModel?.id ?? null}
|
||||
placeholder="Pick one"
|
||||
error={!selectedModel}
|
||||
data={data}
|
||||
onChange={handleValueChanged}
|
||||
sx={{ width: '100%' }}
|
||||
/>
|
||||
);
|
||||
};
|
||||
|
||||
export default memo(ParamIPAdapterModelSelect);
|
@ -0,0 +1,46 @@
|
||||
import { RootState } from 'app/store/store';
|
||||
import { useAppDispatch, useAppSelector } from 'app/store/storeHooks';
|
||||
import IAISlider from 'common/components/IAISlider';
|
||||
import { ipAdapterWeightChanged } from 'features/controlNet/store/controlNetSlice';
|
||||
import { memo, useCallback } from 'react';
|
||||
import { useTranslation } from 'react-i18next';
|
||||
|
||||
const ParamIPAdapterWeight = () => {
|
||||
const isIpAdapterEnabled = useAppSelector(
|
||||
(state: RootState) => state.controlNet.isIPAdapterEnabled
|
||||
);
|
||||
const ipAdapterWeight = useAppSelector(
|
||||
(state: RootState) => state.controlNet.ipAdapterInfo.weight
|
||||
);
|
||||
const dispatch = useAppDispatch();
|
||||
const { t } = useTranslation();
|
||||
|
||||
const handleWeightChanged = useCallback(
|
||||
(weight: number) => {
|
||||
dispatch(ipAdapterWeightChanged(weight));
|
||||
},
|
||||
[dispatch]
|
||||
);
|
||||
|
||||
const handleWeightReset = useCallback(() => {
|
||||
dispatch(ipAdapterWeightChanged(1));
|
||||
}, [dispatch]);
|
||||
|
||||
return (
|
||||
<IAISlider
|
||||
isDisabled={!isIpAdapterEnabled}
|
||||
label={t('controlnet.weight')}
|
||||
value={ipAdapterWeight}
|
||||
onChange={handleWeightChanged}
|
||||
min={0}
|
||||
max={2}
|
||||
step={0.01}
|
||||
withSliderMarks
|
||||
sliderMarks={[0, 1, 2]}
|
||||
withReset
|
||||
handleReset={handleWeightReset}
|
||||
/>
|
||||
);
|
||||
};
|
||||
|
||||
export default memo(ParamIPAdapterWeight);
|
@ -1,9 +1,13 @@
|
||||
import { PayloadAction, createSlice } from '@reduxjs/toolkit';
|
||||
import { ControlNetModelParam } from 'features/parameters/types/parameterSchemas';
|
||||
import {
|
||||
ControlNetModelParam,
|
||||
IPAdapterModelParam,
|
||||
} from 'features/parameters/types/parameterSchemas';
|
||||
import { cloneDeep, forEach } from 'lodash-es';
|
||||
import { imagesApi } from 'services/api/endpoints/images';
|
||||
import { components } from 'services/api/schema';
|
||||
import { isAnySessionRejected } from 'services/api/thunks/session';
|
||||
import { ImageDTO } from 'services/api/types';
|
||||
import { appSocketInvocationError } from 'services/events/actions';
|
||||
import { controlNetImageProcessed } from './actions';
|
||||
import {
|
||||
@ -56,16 +60,36 @@ export type ControlNetConfig = {
|
||||
shouldAutoConfig: boolean;
|
||||
};
|
||||
|
||||
export type IPAdapterConfig = {
|
||||
adapterImage: ImageDTO | null;
|
||||
model: IPAdapterModelParam | null;
|
||||
weight: number;
|
||||
beginStepPct: number;
|
||||
endStepPct: number;
|
||||
};
|
||||
|
||||
export type ControlNetState = {
|
||||
controlNets: Record<string, ControlNetConfig>;
|
||||
isEnabled: boolean;
|
||||
pendingControlImages: string[];
|
||||
isIPAdapterEnabled: boolean;
|
||||
ipAdapterInfo: IPAdapterConfig;
|
||||
};
|
||||
|
||||
export const initialIPAdapterState: IPAdapterConfig = {
|
||||
adapterImage: null,
|
||||
model: null,
|
||||
weight: 1,
|
||||
beginStepPct: 0,
|
||||
endStepPct: 1,
|
||||
};
|
||||
|
||||
export const initialControlNetState: ControlNetState = {
|
||||
controlNets: {},
|
||||
isEnabled: false,
|
||||
pendingControlImages: [],
|
||||
isIPAdapterEnabled: false,
|
||||
ipAdapterInfo: { ...initialIPAdapterState },
|
||||
};
|
||||
|
||||
export const controlNetSlice = createSlice({
|
||||
@ -353,6 +377,31 @@ export const controlNetSlice = createSlice({
|
||||
controlNetReset: () => {
|
||||
return { ...initialControlNetState };
|
||||
},
|
||||
isIPAdapterEnableToggled: (state) => {
|
||||
state.isIPAdapterEnabled = !state.isIPAdapterEnabled;
|
||||
},
|
||||
ipAdapterImageChanged: (state, action: PayloadAction<ImageDTO | null>) => {
|
||||
state.ipAdapterInfo.adapterImage = action.payload;
|
||||
},
|
||||
ipAdapterWeightChanged: (state, action: PayloadAction<number>) => {
|
||||
state.ipAdapterInfo.weight = action.payload;
|
||||
},
|
||||
ipAdapterModelChanged: (
|
||||
state,
|
||||
action: PayloadAction<IPAdapterModelParam | null>
|
||||
) => {
|
||||
state.ipAdapterInfo.model = action.payload;
|
||||
},
|
||||
ipAdapterBeginStepPctChanged: (state, action: PayloadAction<number>) => {
|
||||
state.ipAdapterInfo.beginStepPct = action.payload;
|
||||
},
|
||||
ipAdapterEndStepPctChanged: (state, action: PayloadAction<number>) => {
|
||||
state.ipAdapterInfo.endStepPct = action.payload;
|
||||
},
|
||||
ipAdapterStateReset: (state) => {
|
||||
state.isIPAdapterEnabled = false;
|
||||
state.ipAdapterInfo = { ...initialIPAdapterState };
|
||||
},
|
||||
},
|
||||
extraReducers: (builder) => {
|
||||
builder.addCase(controlNetImageProcessed, (state, action) => {
|
||||
@ -412,6 +461,13 @@ export const {
|
||||
controlNetProcessorTypeChanged,
|
||||
controlNetReset,
|
||||
controlNetAutoConfigToggled,
|
||||
isIPAdapterEnableToggled,
|
||||
ipAdapterImageChanged,
|
||||
ipAdapterWeightChanged,
|
||||
ipAdapterModelChanged,
|
||||
ipAdapterBeginStepPctChanged,
|
||||
ipAdapterEndStepPctChanged,
|
||||
ipAdapterStateReset,
|
||||
} = controlNetSlice.actions;
|
||||
|
||||
export default controlNetSlice.reducer;
|
||||
|
@ -10,20 +10,20 @@ import {
|
||||
Text,
|
||||
} from '@chakra-ui/react';
|
||||
import { createSelector } from '@reduxjs/toolkit';
|
||||
import { stateSelector } from 'app/store/store';
|
||||
import { useAppDispatch, useAppSelector } from 'app/store/storeHooks';
|
||||
import { defaultSelectorOptions } from 'app/store/util/defaultMemoizeOptions';
|
||||
import IAIButton from 'common/components/IAIButton';
|
||||
import IAISwitch from 'common/components/IAISwitch';
|
||||
import { setShouldConfirmOnDelete } from 'features/system/store/systemSlice';
|
||||
import { stateSelector } from 'app/store/store';
|
||||
import { some } from 'lodash-es';
|
||||
import { ChangeEvent, memo, useCallback, useRef } from 'react';
|
||||
import { useTranslation } from 'react-i18next';
|
||||
import { imageDeletionConfirmed } from '../store/actions';
|
||||
import { getImageUsage, selectImageUsage } from '../store/selectors';
|
||||
import { imageDeletionCanceled, isModalOpenChanged } from '../store/slice';
|
||||
import ImageUsageMessage from './ImageUsageMessage';
|
||||
import { ImageUsage } from '../store/types';
|
||||
import ImageUsageMessage from './ImageUsageMessage';
|
||||
|
||||
const selector = createSelector(
|
||||
[stateSelector, selectImageUsage],
|
||||
@ -42,6 +42,7 @@ const selector = createSelector(
|
||||
isCanvasImage: some(allImageUsage, (i) => i.isCanvasImage),
|
||||
isNodesImage: some(allImageUsage, (i) => i.isNodesImage),
|
||||
isControlNetImage: some(allImageUsage, (i) => i.isControlNetImage),
|
||||
isIPAdapterImage: some(allImageUsage, (i) => i.isIPAdapterImage),
|
||||
};
|
||||
|
||||
return {
|
||||
|
@ -1,8 +1,8 @@
|
||||
import { ListItem, Text, UnorderedList } from '@chakra-ui/react';
|
||||
import { some } from 'lodash-es';
|
||||
import { memo } from 'react';
|
||||
import { ImageUsage } from '../store/types';
|
||||
import { useTranslation } from 'react-i18next';
|
||||
import { ImageUsage } from '../store/types';
|
||||
|
||||
type Props = {
|
||||
imageUsage?: ImageUsage;
|
||||
@ -38,6 +38,9 @@ const ImageUsageMessage = (props: Props) => {
|
||||
{imageUsage.isControlNetImage && (
|
||||
<ListItem>{t('common.controlNet')}</ListItem>
|
||||
)}
|
||||
{imageUsage.isIPAdapterImage && (
|
||||
<ListItem>{t('common.ipAdapter')}</ListItem>
|
||||
)}
|
||||
{imageUsage.isNodesImage && (
|
||||
<ListItem>{t('common.nodeEditor')}</ListItem>
|
||||
)}
|
||||
|
@ -1,9 +1,9 @@
|
||||
import { createSelector } from '@reduxjs/toolkit';
|
||||
import { RootState } from 'app/store/store';
|
||||
import { defaultSelectorOptions } from 'app/store/util/defaultMemoizeOptions';
|
||||
import { isInvocationNode } from 'features/nodes/types/types';
|
||||
import { some } from 'lodash-es';
|
||||
import { ImageUsage } from './types';
|
||||
import { isInvocationNode } from 'features/nodes/types/types';
|
||||
|
||||
export const getImageUsage = (state: RootState, image_name: string) => {
|
||||
const { generation, canvas, nodes, controlNet } = state;
|
||||
@ -27,11 +27,15 @@ export const getImageUsage = (state: RootState, image_name: string) => {
|
||||
c.controlImage === image_name || c.processedControlImage === image_name
|
||||
);
|
||||
|
||||
const isIPAdapterImage =
|
||||
controlNet.ipAdapterInfo.adapterImage?.image_name === image_name;
|
||||
|
||||
const imageUsage: ImageUsage = {
|
||||
isInitialImage,
|
||||
isCanvasImage,
|
||||
isNodesImage,
|
||||
isControlNetImage,
|
||||
isIPAdapterImage,
|
||||
};
|
||||
|
||||
return imageUsage;
|
||||
|
@ -10,4 +10,5 @@ export type ImageUsage = {
|
||||
isCanvasImage: boolean;
|
||||
isNodesImage: boolean;
|
||||
isControlNetImage: boolean;
|
||||
isIPAdapterImage: boolean;
|
||||
};
|
||||
|
@ -35,6 +35,10 @@ export type ControlNetDropData = BaseDropData & {
|
||||
};
|
||||
};
|
||||
|
||||
export type IPAdapterImageDropData = BaseDropData & {
|
||||
actionType: 'SET_IP_ADAPTER_IMAGE';
|
||||
};
|
||||
|
||||
export type CanvasInitialImageDropData = BaseDropData & {
|
||||
actionType: 'SET_CANVAS_INITIAL_IMAGE';
|
||||
};
|
||||
@ -73,6 +77,7 @@ export type TypesafeDroppableData =
|
||||
| CurrentImageDropData
|
||||
| InitialImageDropData
|
||||
| ControlNetDropData
|
||||
| IPAdapterImageDropData
|
||||
| CanvasInitialImageDropData
|
||||
| NodesImageDropData
|
||||
| AddToBatchDropData
|
||||
|
@ -24,6 +24,8 @@ export const isValidDrop = (
|
||||
return payloadType === 'IMAGE_DTO';
|
||||
case 'SET_CONTROLNET_IMAGE':
|
||||
return payloadType === 'IMAGE_DTO';
|
||||
case 'SET_IP_ADAPTER_IMAGE':
|
||||
return payloadType === 'IMAGE_DTO';
|
||||
case 'SET_CANVAS_INITIAL_IMAGE':
|
||||
return payloadType === 'IMAGE_DTO';
|
||||
case 'SET_NODES_IMAGE':
|
||||
|
@ -53,6 +53,7 @@ const DeleteBoardModal = (props: Props) => {
|
||||
isCanvasImage: some(allImageUsage, (i) => i.isCanvasImage),
|
||||
isNodesImage: some(allImageUsage, (i) => i.isNodesImage),
|
||||
isControlNetImage: some(allImageUsage, (i) => i.isControlNetImage),
|
||||
isIPAdapterImage: some(allImageUsage, (i) => i.isIPAdapterImage),
|
||||
};
|
||||
return { imageUsageSummary };
|
||||
}),
|
||||
|
@ -15,6 +15,7 @@ import SDXLMainModelInputField from './inputs/SDXLMainModelInputField';
|
||||
import SchedulerInputField from './inputs/SchedulerInputField';
|
||||
import StringInputField from './inputs/StringInputField';
|
||||
import VaeModelInputField from './inputs/VaeModelInputField';
|
||||
import IPAdapterModelInputField from './inputs/IPAdapterModelInputField';
|
||||
|
||||
type InputFieldProps = {
|
||||
nodeId: string;
|
||||
@ -147,6 +148,19 @@ const InputFieldRenderer = ({ nodeId, fieldName }: InputFieldProps) => {
|
||||
);
|
||||
}
|
||||
|
||||
if (
|
||||
field?.type === 'IPAdapterModelField' &&
|
||||
fieldTemplate?.type === 'IPAdapterModelField'
|
||||
) {
|
||||
return (
|
||||
<IPAdapterModelInputField
|
||||
nodeId={nodeId}
|
||||
field={field}
|
||||
fieldTemplate={fieldTemplate}
|
||||
/>
|
||||
);
|
||||
}
|
||||
|
||||
if (field?.type === 'ColorField' && fieldTemplate?.type === 'ColorField') {
|
||||
return (
|
||||
<ColorInputField
|
||||
|
@ -0,0 +1,17 @@
|
||||
import {
|
||||
IPAdapterInputFieldTemplate,
|
||||
IPAdapterInputFieldValue,
|
||||
FieldComponentProps,
|
||||
} from 'features/nodes/types/types';
|
||||
import { memo } from 'react';
|
||||
|
||||
const IPAdapterInputFieldComponent = (
|
||||
_props: FieldComponentProps<
|
||||
IPAdapterInputFieldValue,
|
||||
IPAdapterInputFieldTemplate
|
||||
>
|
||||
) => {
|
||||
return null;
|
||||
};
|
||||
|
||||
export default memo(IPAdapterInputFieldComponent);
|
@ -0,0 +1,100 @@
|
||||
import { SelectItem } from '@mantine/core';
|
||||
import { useAppDispatch } from 'app/store/storeHooks';
|
||||
import IAIMantineSelect from 'common/components/IAIMantineSelect';
|
||||
import { fieldIPAdapterModelValueChanged } from 'features/nodes/store/nodesSlice';
|
||||
import {
|
||||
IPAdapterModelInputFieldTemplate,
|
||||
IPAdapterModelInputFieldValue,
|
||||
FieldComponentProps,
|
||||
} from 'features/nodes/types/types';
|
||||
import { MODEL_TYPE_MAP } from 'features/parameters/types/constants';
|
||||
import { modelIdToIPAdapterModelParam } from 'features/parameters/util/modelIdToIPAdapterModelParams';
|
||||
import { forEach } from 'lodash-es';
|
||||
import { memo, useCallback, useMemo } from 'react';
|
||||
import { useGetIPAdapterModelsQuery } from 'services/api/endpoints/models';
|
||||
|
||||
const IPAdapterModelInputFieldComponent = (
|
||||
props: FieldComponentProps<
|
||||
IPAdapterModelInputFieldValue,
|
||||
IPAdapterModelInputFieldTemplate
|
||||
>
|
||||
) => {
|
||||
const { nodeId, field } = props;
|
||||
const ipAdapterModel = field.value;
|
||||
const dispatch = useAppDispatch();
|
||||
|
||||
const { data: ipAdapterModels } = useGetIPAdapterModelsQuery();
|
||||
|
||||
// grab the full model entity from the RTK Query cache
|
||||
const selectedModel = useMemo(
|
||||
() =>
|
||||
ipAdapterModels?.entities[
|
||||
`${ipAdapterModel?.base_model}/ip_adapter/${ipAdapterModel?.model_name}`
|
||||
] ?? null,
|
||||
[
|
||||
ipAdapterModel?.base_model,
|
||||
ipAdapterModel?.model_name,
|
||||
ipAdapterModels?.entities,
|
||||
]
|
||||
);
|
||||
|
||||
const data = useMemo(() => {
|
||||
if (!ipAdapterModels) {
|
||||
return [];
|
||||
}
|
||||
|
||||
const data: SelectItem[] = [];
|
||||
|
||||
forEach(ipAdapterModels.entities, (model, id) => {
|
||||
if (!model) {
|
||||
return;
|
||||
}
|
||||
|
||||
data.push({
|
||||
value: id,
|
||||
label: model.model_name,
|
||||
group: MODEL_TYPE_MAP[model.base_model],
|
||||
});
|
||||
});
|
||||
|
||||
return data;
|
||||
}, [ipAdapterModels]);
|
||||
|
||||
const handleValueChanged = useCallback(
|
||||
(v: string | null) => {
|
||||
if (!v) {
|
||||
return;
|
||||
}
|
||||
|
||||
const newIPAdapterModel = modelIdToIPAdapterModelParam(v);
|
||||
|
||||
if (!newIPAdapterModel) {
|
||||
return;
|
||||
}
|
||||
|
||||
dispatch(
|
||||
fieldIPAdapterModelValueChanged({
|
||||
nodeId,
|
||||
fieldName: field.name,
|
||||
value: newIPAdapterModel,
|
||||
})
|
||||
);
|
||||
},
|
||||
[dispatch, field.name, nodeId]
|
||||
);
|
||||
|
||||
return (
|
||||
<IAIMantineSelect
|
||||
className="nowheel nodrag"
|
||||
tooltip={selectedModel?.description}
|
||||
value={selectedModel?.id ?? null}
|
||||
placeholder="Pick one"
|
||||
error={!selectedModel}
|
||||
data={data}
|
||||
onChange={handleValueChanged}
|
||||
sx={{ width: '100%' }}
|
||||
/>
|
||||
);
|
||||
};
|
||||
|
||||
export default memo(IPAdapterModelInputFieldComponent);
|
@ -41,6 +41,7 @@ import {
|
||||
IntegerInputFieldValue,
|
||||
InvocationNodeData,
|
||||
InvocationTemplate,
|
||||
IPAdapterModelInputFieldValue,
|
||||
isInvocationNode,
|
||||
isNotesNode,
|
||||
LoRAModelInputFieldValue,
|
||||
@ -520,6 +521,12 @@ const nodesSlice = createSlice({
|
||||
) => {
|
||||
fieldValueReducer(state, action);
|
||||
},
|
||||
fieldIPAdapterModelValueChanged: (
|
||||
state,
|
||||
action: FieldValueAction<IPAdapterModelInputFieldValue>
|
||||
) => {
|
||||
fieldValueReducer(state, action);
|
||||
},
|
||||
fieldEnumModelValueChanged: (
|
||||
state,
|
||||
action: FieldValueAction<EnumInputFieldValue>
|
||||
@ -866,6 +873,7 @@ export const {
|
||||
fieldLoRAModelValueChanged,
|
||||
fieldEnumModelValueChanged,
|
||||
fieldControlNetModelValueChanged,
|
||||
fieldIPAdapterModelValueChanged,
|
||||
fieldRefinerModelValueChanged,
|
||||
fieldSchedulerValueChanged,
|
||||
nodeIsOpenChanged,
|
||||
|
@ -41,6 +41,7 @@ export const POLYMORPHIC_TYPES = [
|
||||
];
|
||||
|
||||
export const MODEL_TYPES = [
|
||||
'IPAdapterModelField',
|
||||
'ControlNetModelField',
|
||||
'LoRAModelField',
|
||||
'MainModelField',
|
||||
@ -236,6 +237,16 @@ export const FIELDS: Record<FieldType, FieldUIConfig> = {
|
||||
description: t('nodes.integerPolymorphicDescription'),
|
||||
title: t('nodes.integerPolymorphic'),
|
||||
},
|
||||
IPAdapterField: {
|
||||
color: 'green.300',
|
||||
description: 'IP-Adapter info passed between nodes.',
|
||||
title: 'IP-Adapter',
|
||||
},
|
||||
IPAdapterModelField: {
|
||||
color: 'teal.500',
|
||||
description: 'IP-Adapter model',
|
||||
title: 'IP-Adapter Model',
|
||||
},
|
||||
LatentsCollection: {
|
||||
color: 'pink.500',
|
||||
description: t('nodes.latentsCollectionDescription'),
|
||||
|
@ -94,6 +94,8 @@ export const zFieldType = z.enum([
|
||||
'integer',
|
||||
'IntegerCollection',
|
||||
'IntegerPolymorphic',
|
||||
'IPAdapterField',
|
||||
'IPAdapterModelField',
|
||||
'LatentsCollection',
|
||||
'LatentsField',
|
||||
'LatentsPolymorphic',
|
||||
@ -389,6 +391,25 @@ export type ControlCollectionInputFieldValue = z.infer<
|
||||
typeof zControlCollectionInputFieldValue
|
||||
>;
|
||||
|
||||
export const zIPAdapterModel = zModelIdentifier;
|
||||
export type IPAdapterModel = z.infer<typeof zIPAdapterModel>;
|
||||
|
||||
export const zIPAdapterField = z.object({
|
||||
image: zImageField,
|
||||
ip_adapter_model: zIPAdapterModel,
|
||||
image_encoder_model: z.string().trim().min(1),
|
||||
weight: z.number(),
|
||||
});
|
||||
export type IPAdapterField = z.infer<typeof zIPAdapterField>;
|
||||
|
||||
export const zIPAdapterInputFieldValue = zInputFieldValueBase.extend({
|
||||
type: z.literal('IPAdapterField'),
|
||||
value: zIPAdapterField.optional(),
|
||||
});
|
||||
export type IPAdapterInputFieldValue = z.infer<
|
||||
typeof zIPAdapterInputFieldValue
|
||||
>;
|
||||
|
||||
export const zModelType = z.enum([
|
||||
'onnx',
|
||||
'main',
|
||||
@ -538,6 +559,17 @@ export type ControlNetModelInputFieldValue = z.infer<
|
||||
typeof zControlNetModelInputFieldValue
|
||||
>;
|
||||
|
||||
export const zIPAdapterModelField = zModelIdentifier;
|
||||
export type IPAdapterModelField = z.infer<typeof zIPAdapterModelField>;
|
||||
|
||||
export const zIPAdapterModelInputFieldValue = zInputFieldValueBase.extend({
|
||||
type: z.literal('IPAdapterModelField'),
|
||||
value: zIPAdapterModelField.optional(),
|
||||
});
|
||||
export type IPAdapterModelInputFieldValue = z.infer<
|
||||
typeof zIPAdapterModelInputFieldValue
|
||||
>;
|
||||
|
||||
export const zCollectionInputFieldValue = zInputFieldValueBase.extend({
|
||||
type: z.literal('Collection'),
|
||||
value: z.array(z.any()).optional(), // TODO: should this field ever have a value?
|
||||
@ -620,6 +652,8 @@ export const zInputFieldValue = z.discriminatedUnion('type', [
|
||||
zIntegerCollectionInputFieldValue,
|
||||
zIntegerPolymorphicInputFieldValue,
|
||||
zIntegerInputFieldValue,
|
||||
zIPAdapterInputFieldValue,
|
||||
zIPAdapterModelInputFieldValue,
|
||||
zLatentsInputFieldValue,
|
||||
zLatentsCollectionInputFieldValue,
|
||||
zLatentsPolymorphicInputFieldValue,
|
||||
@ -822,6 +856,11 @@ export type ControlPolymorphicInputFieldTemplate = Omit<
|
||||
type: 'ControlPolymorphic';
|
||||
};
|
||||
|
||||
export type IPAdapterInputFieldTemplate = InputFieldTemplateBase & {
|
||||
default: undefined;
|
||||
type: 'IPAdapterField';
|
||||
};
|
||||
|
||||
export type EnumInputFieldTemplate = InputFieldTemplateBase & {
|
||||
default: string;
|
||||
type: 'enum';
|
||||
@ -859,6 +898,11 @@ export type ControlNetModelInputFieldTemplate = InputFieldTemplateBase & {
|
||||
type: 'ControlNetModelField';
|
||||
};
|
||||
|
||||
export type IPAdapterModelInputFieldTemplate = InputFieldTemplateBase & {
|
||||
default: string;
|
||||
type: 'IPAdapterModelField';
|
||||
};
|
||||
|
||||
export type CollectionInputFieldTemplate = InputFieldTemplateBase & {
|
||||
default: [];
|
||||
type: 'Collection';
|
||||
@ -930,6 +974,8 @@ export type InputFieldTemplate =
|
||||
| IntegerCollectionInputFieldTemplate
|
||||
| IntegerPolymorphicInputFieldTemplate
|
||||
| IntegerInputFieldTemplate
|
||||
| IPAdapterInputFieldTemplate
|
||||
| IPAdapterModelInputFieldTemplate
|
||||
| LatentsInputFieldTemplate
|
||||
| LatentsCollectionInputFieldTemplate
|
||||
| LatentsPolymorphicInputFieldTemplate
|
||||
|
@ -60,6 +60,8 @@ import {
|
||||
ImageField,
|
||||
LatentsField,
|
||||
ConditioningField,
|
||||
IPAdapterInputFieldTemplate,
|
||||
IPAdapterModelInputFieldTemplate,
|
||||
} from '../types/types';
|
||||
import { ControlField } from 'services/api/types';
|
||||
|
||||
@ -435,6 +437,19 @@ const buildControlNetModelInputFieldTemplate = ({
|
||||
return template;
|
||||
};
|
||||
|
||||
const buildIPAdapterModelInputFieldTemplate = ({
|
||||
schemaObject,
|
||||
baseField,
|
||||
}: BuildInputFieldArg): IPAdapterModelInputFieldTemplate => {
|
||||
const template: IPAdapterModelInputFieldTemplate = {
|
||||
...baseField,
|
||||
type: 'IPAdapterModelField',
|
||||
default: schemaObject.default ?? undefined,
|
||||
};
|
||||
|
||||
return template;
|
||||
};
|
||||
|
||||
const buildImageInputFieldTemplate = ({
|
||||
schemaObject,
|
||||
baseField,
|
||||
@ -648,6 +663,19 @@ const buildControlCollectionInputFieldTemplate = ({
|
||||
return template;
|
||||
};
|
||||
|
||||
const buildIPAdapterInputFieldTemplate = ({
|
||||
schemaObject,
|
||||
baseField,
|
||||
}: BuildInputFieldArg): IPAdapterInputFieldTemplate => {
|
||||
const template: IPAdapterInputFieldTemplate = {
|
||||
...baseField,
|
||||
type: 'IPAdapterField',
|
||||
default: schemaObject.default ?? undefined,
|
||||
};
|
||||
|
||||
return template;
|
||||
};
|
||||
|
||||
const buildEnumInputFieldTemplate = ({
|
||||
schemaObject,
|
||||
baseField,
|
||||
@ -851,6 +879,8 @@ const TEMPLATE_BUILDER_MAP = {
|
||||
integer: buildIntegerInputFieldTemplate,
|
||||
IntegerCollection: buildIntegerCollectionInputFieldTemplate,
|
||||
IntegerPolymorphic: buildIntegerPolymorphicInputFieldTemplate,
|
||||
IPAdapterField: buildIPAdapterInputFieldTemplate,
|
||||
IPAdapterModelField: buildIPAdapterModelInputFieldTemplate,
|
||||
LatentsCollection: buildLatentsCollectionInputFieldTemplate,
|
||||
LatentsField: buildLatentsInputFieldTemplate,
|
||||
LatentsPolymorphic: buildLatentsPolymorphicInputFieldTemplate,
|
||||
|
@ -28,6 +28,8 @@ const FIELD_VALUE_FALLBACK_MAP = {
|
||||
integer: 0,
|
||||
IntegerCollection: [],
|
||||
IntegerPolymorphic: 0,
|
||||
IPAdapterField: undefined,
|
||||
IPAdapterModelField: undefined,
|
||||
LatentsCollection: [],
|
||||
LatentsField: undefined,
|
||||
LatentsPolymorphic: undefined,
|
||||
|
@ -0,0 +1,59 @@
|
||||
import { RootState } from 'app/store/store';
|
||||
import { IPAdapterInvocation } from 'services/api/types';
|
||||
import { NonNullableGraph } from '../../types/types';
|
||||
import { IP_ADAPTER } from './constants';
|
||||
|
||||
export const addIPAdapterToLinearGraph = (
|
||||
state: RootState,
|
||||
graph: NonNullableGraph,
|
||||
baseNodeId: string
|
||||
): void => {
|
||||
const { isIPAdapterEnabled, ipAdapterInfo } = state.controlNet;
|
||||
|
||||
// const metadataAccumulator = graph.nodes[METADATA_ACCUMULATOR] as
|
||||
// | MetadataAccumulatorInvocation
|
||||
// | undefined;
|
||||
|
||||
if (isIPAdapterEnabled && ipAdapterInfo.model) {
|
||||
const ipAdapterNode: IPAdapterInvocation = {
|
||||
id: IP_ADAPTER,
|
||||
type: 'ip_adapter',
|
||||
is_intermediate: true,
|
||||
weight: ipAdapterInfo.weight,
|
||||
ip_adapter_model: {
|
||||
base_model: ipAdapterInfo.model?.base_model,
|
||||
model_name: ipAdapterInfo.model?.model_name,
|
||||
},
|
||||
begin_step_percent: ipAdapterInfo.beginStepPct,
|
||||
end_step_percent: ipAdapterInfo.endStepPct,
|
||||
};
|
||||
|
||||
if (ipAdapterInfo.adapterImage) {
|
||||
ipAdapterNode.image = {
|
||||
image_name: ipAdapterInfo.adapterImage.image_name,
|
||||
};
|
||||
} else {
|
||||
return;
|
||||
}
|
||||
|
||||
graph.nodes[ipAdapterNode.id] = ipAdapterNode as IPAdapterInvocation;
|
||||
|
||||
// if (metadataAccumulator?.ip_adapters) {
|
||||
// // metadata accumulator only needs the ip_adapter field - not the whole node
|
||||
// // extract what we need and add to the accumulator
|
||||
// const ipAdapterField = omit(ipAdapterNode, [
|
||||
// 'id',
|
||||
// 'type',
|
||||
// ]) as IPAdapterField;
|
||||
// metadataAccumulator.ip_adapters.push(ipAdapterField);
|
||||
// }
|
||||
|
||||
graph.edges.push({
|
||||
source: { node_id: ipAdapterNode.id, field: 'ip_adapter' },
|
||||
destination: {
|
||||
node_id: baseNodeId,
|
||||
field: 'ip_adapter',
|
||||
},
|
||||
});
|
||||
}
|
||||
};
|
@ -5,6 +5,7 @@ import { initialGenerationState } from 'features/parameters/store/generationSlic
|
||||
import { ImageDTO, ImageToLatentsInvocation } from 'services/api/types';
|
||||
import { addControlNetToLinearGraph } from './addControlNetToLinearGraph';
|
||||
import { addDynamicPromptsToGraph } from './addDynamicPromptsToGraph';
|
||||
import { addIPAdapterToLinearGraph } from './addIPAdapterToLinearGraph';
|
||||
import { addLoRAsToGraph } from './addLoRAsToGraph';
|
||||
import { addNSFWCheckerToGraph } from './addNSFWCheckerToGraph';
|
||||
import { addSeamlessToLinearGraph } from './addSeamlessToLinearGraph';
|
||||
@ -366,6 +367,9 @@ export const buildCanvasImageToImageGraph = (
|
||||
// add controlnet, mutating `graph`
|
||||
addControlNetToLinearGraph(state, graph, DENOISE_LATENTS);
|
||||
|
||||
// Add IP Adapter
|
||||
addIPAdapterToLinearGraph(state, graph, DENOISE_LATENTS);
|
||||
|
||||
// NSFW & watermark - must be last thing added to graph
|
||||
if (state.system.shouldUseNSFWChecker) {
|
||||
// must add before watermarker!
|
||||
|
@ -12,6 +12,7 @@ import {
|
||||
RangeOfSizeInvocation,
|
||||
} from 'services/api/types';
|
||||
import { addControlNetToLinearGraph } from './addControlNetToLinearGraph';
|
||||
import { addIPAdapterToLinearGraph } from './addIPAdapterToLinearGraph';
|
||||
import { addLoRAsToGraph } from './addLoRAsToGraph';
|
||||
import { addNSFWCheckerToGraph } from './addNSFWCheckerToGraph';
|
||||
import { addSeamlessToLinearGraph } from './addSeamlessToLinearGraph';
|
||||
@ -736,6 +737,9 @@ export const buildCanvasInpaintGraph = (
|
||||
// add controlnet, mutating `graph`
|
||||
addControlNetToLinearGraph(state, graph, DENOISE_LATENTS);
|
||||
|
||||
// Add IP Adapter
|
||||
addIPAdapterToLinearGraph(state, graph, DENOISE_LATENTS);
|
||||
|
||||
// NSFW & watermark - must be last thing added to graph
|
||||
if (state.system.shouldUseNSFWChecker) {
|
||||
// must add before watermarker!
|
||||
|
@ -11,6 +11,7 @@ import {
|
||||
RangeOfSizeInvocation,
|
||||
} from 'services/api/types';
|
||||
import { addControlNetToLinearGraph } from './addControlNetToLinearGraph';
|
||||
import { addIPAdapterToLinearGraph } from './addIPAdapterToLinearGraph';
|
||||
import { addLoRAsToGraph } from './addLoRAsToGraph';
|
||||
import { addNSFWCheckerToGraph } from './addNSFWCheckerToGraph';
|
||||
import { addSeamlessToLinearGraph } from './addSeamlessToLinearGraph';
|
||||
@ -838,6 +839,9 @@ export const buildCanvasOutpaintGraph = (
|
||||
// add controlnet, mutating `graph`
|
||||
addControlNetToLinearGraph(state, graph, DENOISE_LATENTS);
|
||||
|
||||
// Add IP Adapter
|
||||
addIPAdapterToLinearGraph(state, graph, DENOISE_LATENTS);
|
||||
|
||||
// NSFW & watermark - must be last thing added to graph
|
||||
if (state.system.shouldUseNSFWChecker) {
|
||||
// must add before watermarker!
|
||||
|
@ -5,6 +5,7 @@ import { initialGenerationState } from 'features/parameters/store/generationSlic
|
||||
import { ImageDTO, ImageToLatentsInvocation } from 'services/api/types';
|
||||
import { addControlNetToLinearGraph } from './addControlNetToLinearGraph';
|
||||
import { addDynamicPromptsToGraph } from './addDynamicPromptsToGraph';
|
||||
import { addIPAdapterToLinearGraph } from './addIPAdapterToLinearGraph';
|
||||
import { addNSFWCheckerToGraph } from './addNSFWCheckerToGraph';
|
||||
import { addSDXLLoRAsToGraph } from './addSDXLLoRAstoGraph';
|
||||
import { addSDXLRefinerToGraph } from './addSDXLRefinerToGraph';
|
||||
@ -392,6 +393,9 @@ export const buildCanvasSDXLImageToImageGraph = (
|
||||
// add controlnet, mutating `graph`
|
||||
addControlNetToLinearGraph(state, graph, SDXL_DENOISE_LATENTS);
|
||||
|
||||
// Add IP Adapter
|
||||
addIPAdapterToLinearGraph(state, graph, SDXL_DENOISE_LATENTS);
|
||||
|
||||
// NSFW & watermark - must be last thing added to graph
|
||||
if (state.system.shouldUseNSFWChecker) {
|
||||
// must add before watermarker!
|
||||
|
@ -46,6 +46,7 @@ import {
|
||||
SEAMLESS,
|
||||
} from './constants';
|
||||
import { craftSDXLStylePrompt } from './helpers/craftSDXLStylePrompt';
|
||||
import { addIPAdapterToLinearGraph } from './addIPAdapterToLinearGraph';
|
||||
|
||||
/**
|
||||
* Builds the Canvas tab's Inpaint graph.
|
||||
@ -765,6 +766,9 @@ export const buildCanvasSDXLInpaintGraph = (
|
||||
// add controlnet, mutating `graph`
|
||||
addControlNetToLinearGraph(state, graph, SDXL_DENOISE_LATENTS);
|
||||
|
||||
// Add IP Adapter
|
||||
addIPAdapterToLinearGraph(state, graph, SDXL_DENOISE_LATENTS);
|
||||
|
||||
// NSFW & watermark - must be last thing added to graph
|
||||
if (state.system.shouldUseNSFWChecker) {
|
||||
// must add before watermarker!
|
||||
|
@ -11,6 +11,7 @@ import {
|
||||
RangeOfSizeInvocation,
|
||||
} from 'services/api/types';
|
||||
import { addControlNetToLinearGraph } from './addControlNetToLinearGraph';
|
||||
import { addIPAdapterToLinearGraph } from './addIPAdapterToLinearGraph';
|
||||
import { addNSFWCheckerToGraph } from './addNSFWCheckerToGraph';
|
||||
import { addSDXLLoRAsToGraph } from './addSDXLLoRAstoGraph';
|
||||
import { addSDXLRefinerToGraph } from './addSDXLRefinerToGraph';
|
||||
@ -868,6 +869,9 @@ export const buildCanvasSDXLOutpaintGraph = (
|
||||
// add controlnet, mutating `graph`
|
||||
addControlNetToLinearGraph(state, graph, SDXL_DENOISE_LATENTS);
|
||||
|
||||
// Add IP Adapter
|
||||
addIPAdapterToLinearGraph(state, graph, SDXL_DENOISE_LATENTS);
|
||||
|
||||
// NSFW & watermark - must be last thing added to graph
|
||||
if (state.system.shouldUseNSFWChecker) {
|
||||
// must add before watermarker!
|
||||
|
@ -8,6 +8,7 @@ import {
|
||||
} from 'services/api/types';
|
||||
import { addControlNetToLinearGraph } from './addControlNetToLinearGraph';
|
||||
import { addDynamicPromptsToGraph } from './addDynamicPromptsToGraph';
|
||||
import { addIPAdapterToLinearGraph } from './addIPAdapterToLinearGraph';
|
||||
import { addNSFWCheckerToGraph } from './addNSFWCheckerToGraph';
|
||||
import { addSDXLLoRAsToGraph } from './addSDXLLoRAstoGraph';
|
||||
import { addSDXLRefinerToGraph } from './addSDXLRefinerToGraph';
|
||||
@ -372,6 +373,9 @@ export const buildCanvasSDXLTextToImageGraph = (
|
||||
// add controlnet, mutating `graph`
|
||||
addControlNetToLinearGraph(state, graph, SDXL_DENOISE_LATENTS);
|
||||
|
||||
// Add IP Adapter
|
||||
addIPAdapterToLinearGraph(state, graph, SDXL_DENOISE_LATENTS);
|
||||
|
||||
// NSFW & watermark - must be last thing added to graph
|
||||
if (state.system.shouldUseNSFWChecker) {
|
||||
// must add before watermarker!
|
||||
|
@ -8,6 +8,7 @@ import {
|
||||
} from 'services/api/types';
|
||||
import { addControlNetToLinearGraph } from './addControlNetToLinearGraph';
|
||||
import { addDynamicPromptsToGraph } from './addDynamicPromptsToGraph';
|
||||
import { addIPAdapterToLinearGraph } from './addIPAdapterToLinearGraph';
|
||||
import { addLoRAsToGraph } from './addLoRAsToGraph';
|
||||
import { addNSFWCheckerToGraph } from './addNSFWCheckerToGraph';
|
||||
import { addSeamlessToLinearGraph } from './addSeamlessToLinearGraph';
|
||||
@ -345,6 +346,9 @@ export const buildCanvasTextToImageGraph = (
|
||||
// add controlnet, mutating `graph`
|
||||
addControlNetToLinearGraph(state, graph, DENOISE_LATENTS);
|
||||
|
||||
// Add IP Adapter
|
||||
addIPAdapterToLinearGraph(state, graph, DENOISE_LATENTS);
|
||||
|
||||
// NSFW & watermark - must be last thing added to graph
|
||||
if (state.system.shouldUseNSFWChecker) {
|
||||
// must add before watermarker!
|
||||
|
@ -8,6 +8,7 @@ import {
|
||||
} from 'services/api/types';
|
||||
import { addControlNetToLinearGraph } from './addControlNetToLinearGraph';
|
||||
import { addDynamicPromptsToGraph } from './addDynamicPromptsToGraph';
|
||||
import { addIPAdapterToLinearGraph } from './addIPAdapterToLinearGraph';
|
||||
import { addLoRAsToGraph } from './addLoRAsToGraph';
|
||||
import { addNSFWCheckerToGraph } from './addNSFWCheckerToGraph';
|
||||
import { addSeamlessToLinearGraph } from './addSeamlessToLinearGraph';
|
||||
@ -364,6 +365,9 @@ export const buildLinearImageToImageGraph = (
|
||||
// add controlnet, mutating `graph`
|
||||
addControlNetToLinearGraph(state, graph, DENOISE_LATENTS);
|
||||
|
||||
// Add IP Adapter
|
||||
addIPAdapterToLinearGraph(state, graph, DENOISE_LATENTS);
|
||||
|
||||
// NSFW & watermark - must be last thing added to graph
|
||||
if (state.system.shouldUseNSFWChecker) {
|
||||
// must add before watermarker!
|
||||
|
@ -8,6 +8,7 @@ import {
|
||||
} from 'services/api/types';
|
||||
import { addControlNetToLinearGraph } from './addControlNetToLinearGraph';
|
||||
import { addDynamicPromptsToGraph } from './addDynamicPromptsToGraph';
|
||||
import { addIPAdapterToLinearGraph } from './addIPAdapterToLinearGraph';
|
||||
import { addNSFWCheckerToGraph } from './addNSFWCheckerToGraph';
|
||||
import { addSDXLLoRAsToGraph } from './addSDXLLoRAstoGraph';
|
||||
import { addSDXLRefinerToGraph } from './addSDXLRefinerToGraph';
|
||||
@ -384,6 +385,9 @@ export const buildLinearSDXLImageToImageGraph = (
|
||||
// add controlnet, mutating `graph`
|
||||
addControlNetToLinearGraph(state, graph, SDXL_DENOISE_LATENTS);
|
||||
|
||||
// Add IP Adapter
|
||||
addIPAdapterToLinearGraph(state, graph, SDXL_DENOISE_LATENTS);
|
||||
|
||||
// add dynamic prompts - also sets up core iteration and seed
|
||||
addDynamicPromptsToGraph(state, graph);
|
||||
|
||||
|
@ -4,6 +4,7 @@ import { NonNullableGraph } from 'features/nodes/types/types';
|
||||
import { initialGenerationState } from 'features/parameters/store/generationSlice';
|
||||
import { addControlNetToLinearGraph } from './addControlNetToLinearGraph';
|
||||
import { addDynamicPromptsToGraph } from './addDynamicPromptsToGraph';
|
||||
import { addIPAdapterToLinearGraph } from './addIPAdapterToLinearGraph';
|
||||
import { addNSFWCheckerToGraph } from './addNSFWCheckerToGraph';
|
||||
import { addSDXLLoRAsToGraph } from './addSDXLLoRAstoGraph';
|
||||
import { addSDXLRefinerToGraph } from './addSDXLRefinerToGraph';
|
||||
@ -277,6 +278,9 @@ export const buildLinearSDXLTextToImageGraph = (
|
||||
// add controlnet, mutating `graph`
|
||||
addControlNetToLinearGraph(state, graph, SDXL_DENOISE_LATENTS);
|
||||
|
||||
// add IP Adapter
|
||||
addIPAdapterToLinearGraph(state, graph, SDXL_DENOISE_LATENTS);
|
||||
|
||||
// add dynamic prompts - also sets up core iteration and seed
|
||||
addDynamicPromptsToGraph(state, graph);
|
||||
|
||||
|
@ -8,6 +8,7 @@ import {
|
||||
} from 'services/api/types';
|
||||
import { addControlNetToLinearGraph } from './addControlNetToLinearGraph';
|
||||
import { addDynamicPromptsToGraph } from './addDynamicPromptsToGraph';
|
||||
import { addIPAdapterToLinearGraph } from './addIPAdapterToLinearGraph';
|
||||
import { addLoRAsToGraph } from './addLoRAsToGraph';
|
||||
import { addNSFWCheckerToGraph } from './addNSFWCheckerToGraph';
|
||||
import { addSeamlessToLinearGraph } from './addSeamlessToLinearGraph';
|
||||
@ -282,6 +283,9 @@ export const buildLinearTextToImageGraph = (
|
||||
// add controlnet, mutating `graph`
|
||||
addControlNetToLinearGraph(state, graph, DENOISE_LATENTS);
|
||||
|
||||
// add IP Adapter
|
||||
addIPAdapterToLinearGraph(state, graph, DENOISE_LATENTS);
|
||||
|
||||
// NSFW & watermark - must be last thing added to graph
|
||||
if (state.system.shouldUseNSFWChecker) {
|
||||
// must add before watermarker!
|
||||
|
@ -45,6 +45,7 @@ export const MASK_RESIZE_DOWN = 'mask_resize_down';
|
||||
export const COLOR_CORRECT = 'color_correct';
|
||||
export const PASTE_IMAGE = 'img_paste';
|
||||
export const CONTROL_NET_COLLECT = 'control_net_collect';
|
||||
export const IP_ADAPTER = 'ip_adapter';
|
||||
export const DYNAMIC_PROMPT = 'dynamic_prompt';
|
||||
export const IMAGE_COLLECTION = 'image_collection';
|
||||
export const IMAGE_COLLECTION_ITERATE = 'image_collection_iterate';
|
||||
|
@ -6,6 +6,7 @@ import { defaultSelectorOptions } from 'app/store/util/defaultMemoizeOptions';
|
||||
import IAICollapse from 'common/components/IAICollapse';
|
||||
import IAIIconButton from 'common/components/IAIIconButton';
|
||||
import ControlNet from 'features/controlNet/components/ControlNet';
|
||||
import IPAdapterPanel from 'features/controlNet/components/ipAdapter/IPAdapterPanel';
|
||||
import ParamControlNetFeatureToggle from 'features/controlNet/components/parameters/ParamControlNetFeatureToggle';
|
||||
import {
|
||||
controlNetAdded,
|
||||
@ -25,14 +26,23 @@ import { v4 as uuidv4 } from 'uuid';
|
||||
const selector = createSelector(
|
||||
[stateSelector],
|
||||
({ controlNet }) => {
|
||||
const { controlNets, isEnabled } = controlNet;
|
||||
const { controlNets, isEnabled, isIPAdapterEnabled } = controlNet;
|
||||
|
||||
const validControlNets = getValidControlNets(controlNets);
|
||||
|
||||
const activeLabel =
|
||||
isEnabled && validControlNets.length > 0
|
||||
? `${validControlNets.length} Active`
|
||||
: undefined;
|
||||
let activeLabel = undefined;
|
||||
|
||||
if (isEnabled && validControlNets.length > 0) {
|
||||
activeLabel = `${validControlNets.length} ControlNet`;
|
||||
}
|
||||
|
||||
if (isIPAdapterEnabled) {
|
||||
if (activeLabel) {
|
||||
activeLabel = `${activeLabel}, IP Adapter`;
|
||||
} else {
|
||||
activeLabel = 'IP Adapter';
|
||||
}
|
||||
}
|
||||
|
||||
return { controlNetsArray: map(controlNets), activeLabel };
|
||||
},
|
||||
@ -101,6 +111,7 @@ const ParamControlNetCollapse = () => {
|
||||
<ControlNet controlNet={c} />
|
||||
</Fragment>
|
||||
))}
|
||||
<IPAdapterPanel />
|
||||
</Flex>
|
||||
</IAICollapse>
|
||||
);
|
||||
|
@ -1,6 +1,7 @@
|
||||
import { components } from 'services/api/schema';
|
||||
|
||||
export const MODEL_TYPE_MAP = {
|
||||
any: 'Any',
|
||||
'sd-1': 'Stable Diffusion 1.x',
|
||||
'sd-2': 'Stable Diffusion 2.x',
|
||||
sdxl: 'Stable Diffusion XL',
|
||||
@ -8,6 +9,7 @@ export const MODEL_TYPE_MAP = {
|
||||
};
|
||||
|
||||
export const MODEL_TYPE_SHORT_MAP = {
|
||||
any: 'Any',
|
||||
'sd-1': 'SD1',
|
||||
'sd-2': 'SD2',
|
||||
sdxl: 'SDXL',
|
||||
@ -15,6 +17,10 @@ export const MODEL_TYPE_SHORT_MAP = {
|
||||
};
|
||||
|
||||
export const clipSkipMap = {
|
||||
any: {
|
||||
maxClip: 0,
|
||||
markers: [],
|
||||
},
|
||||
'sd-1': {
|
||||
maxClip: 12,
|
||||
markers: [0, 1, 2, 3, 4, 8, 12],
|
||||
|
@ -210,7 +210,13 @@ export type HeightParam = z.infer<typeof zHeight>;
|
||||
export const isValidHeight = (val: unknown): val is HeightParam =>
|
||||
zHeight.safeParse(val).success;
|
||||
|
||||
export const zBaseModel = z.enum(['sd-1', 'sd-2', 'sdxl', 'sdxl-refiner']);
|
||||
export const zBaseModel = z.enum([
|
||||
'any',
|
||||
'sd-1',
|
||||
'sd-2',
|
||||
'sdxl',
|
||||
'sdxl-refiner',
|
||||
]);
|
||||
|
||||
export type BaseModelParam = z.infer<typeof zBaseModel>;
|
||||
|
||||
@ -323,7 +329,17 @@ export type ControlNetModelParam = z.infer<typeof zLoRAModel>;
|
||||
export const isValidControlNetModel = (
|
||||
val: unknown
|
||||
): val is ControlNetModelParam => zControlNetModel.safeParse(val).success;
|
||||
|
||||
/**
|
||||
* Zod schema for IP-Adapter models
|
||||
*/
|
||||
export const zIPAdapterModel = z.object({
|
||||
model_name: z.string().min(1),
|
||||
base_model: zBaseModel,
|
||||
});
|
||||
/**
|
||||
* Type alias for model parameter, inferred from its zod schema
|
||||
*/
|
||||
export type IPAdapterModelParam = z.infer<typeof zIPAdapterModel>;
|
||||
/**
|
||||
* Zod schema for l2l strength parameter
|
||||
*/
|
||||
|
@ -0,0 +1,29 @@
|
||||
import { logger } from 'app/logging/logger';
|
||||
import { zIPAdapterModel } from 'features/parameters/types/parameterSchemas';
|
||||
import { IPAdapterModelField } from 'services/api/types';
|
||||
|
||||
export const modelIdToIPAdapterModelParam = (
|
||||
ipAdapterModelId: string
|
||||
): IPAdapterModelField | undefined => {
|
||||
const log = logger('models');
|
||||
const [base_model, _model_type, model_name] = ipAdapterModelId.split('/');
|
||||
|
||||
const result = zIPAdapterModel.safeParse({
|
||||
base_model,
|
||||
model_name,
|
||||
});
|
||||
|
||||
if (!result.success) {
|
||||
log.error(
|
||||
{
|
||||
ipAdapterModelId,
|
||||
errors: result.error.format(),
|
||||
},
|
||||
'Failed to parse IP-Adapter model id'
|
||||
);
|
||||
|
||||
return;
|
||||
}
|
||||
|
||||
return result.data;
|
||||
};
|
@ -5,6 +5,7 @@ import {
|
||||
BaseModelType,
|
||||
CheckpointModelConfig,
|
||||
ControlNetModelConfig,
|
||||
IPAdapterModelConfig,
|
||||
DiffusersModelConfig,
|
||||
ImportModelConfig,
|
||||
LoRAModelConfig,
|
||||
@ -36,6 +37,10 @@ export type ControlNetModelConfigEntity = ControlNetModelConfig & {
|
||||
id: string;
|
||||
};
|
||||
|
||||
export type IPAdapterModelConfigEntity = IPAdapterModelConfig & {
|
||||
id: string;
|
||||
};
|
||||
|
||||
export type TextualInversionModelConfigEntity = TextualInversionModelConfig & {
|
||||
id: string;
|
||||
};
|
||||
@ -47,6 +52,7 @@ type AnyModelConfigEntity =
|
||||
| OnnxModelConfigEntity
|
||||
| LoRAModelConfigEntity
|
||||
| ControlNetModelConfigEntity
|
||||
| IPAdapterModelConfigEntity
|
||||
| TextualInversionModelConfigEntity
|
||||
| VaeModelConfigEntity;
|
||||
|
||||
@ -135,6 +141,10 @@ export const controlNetModelsAdapter =
|
||||
createEntityAdapter<ControlNetModelConfigEntity>({
|
||||
sortComparer: (a, b) => a.model_name.localeCompare(b.model_name),
|
||||
});
|
||||
export const ipAdapterModelsAdapter =
|
||||
createEntityAdapter<IPAdapterModelConfigEntity>({
|
||||
sortComparer: (a, b) => a.model_name.localeCompare(b.model_name),
|
||||
});
|
||||
export const textualInversionModelsAdapter =
|
||||
createEntityAdapter<TextualInversionModelConfigEntity>({
|
||||
sortComparer: (a, b) => a.model_name.localeCompare(b.model_name),
|
||||
@ -435,6 +445,37 @@ export const modelsApi = api.injectEndpoints({
|
||||
);
|
||||
},
|
||||
}),
|
||||
getIPAdapterModels: build.query<
|
||||
EntityState<IPAdapterModelConfigEntity>,
|
||||
void
|
||||
>({
|
||||
query: () => ({ url: 'models/', params: { model_type: 'ip_adapter' } }),
|
||||
providesTags: (result) => {
|
||||
const tags: ApiFullTagDescription[] = [
|
||||
{ type: 'IPAdapterModel', id: LIST_TAG },
|
||||
];
|
||||
|
||||
if (result) {
|
||||
tags.push(
|
||||
...result.ids.map((id) => ({
|
||||
type: 'IPAdapterModel' as const,
|
||||
id,
|
||||
}))
|
||||
);
|
||||
}
|
||||
|
||||
return tags;
|
||||
},
|
||||
transformResponse: (response: { models: IPAdapterModelConfig[] }) => {
|
||||
const entities = createModelEntities<IPAdapterModelConfigEntity>(
|
||||
response.models
|
||||
);
|
||||
return ipAdapterModelsAdapter.setAll(
|
||||
ipAdapterModelsAdapter.getInitialState(),
|
||||
entities
|
||||
);
|
||||
},
|
||||
}),
|
||||
getVaeModels: build.query<EntityState<VaeModelConfigEntity>, void>({
|
||||
query: () => ({ url: 'models/', params: { model_type: 'vae' } }),
|
||||
providesTags: (result) => {
|
||||
@ -533,6 +574,7 @@ export const {
|
||||
useGetMainModelsQuery,
|
||||
useGetOnnxModelsQuery,
|
||||
useGetControlNetModelsQuery,
|
||||
useGetIPAdapterModelsQuery,
|
||||
useGetLoRAModelsQuery,
|
||||
useGetTextualInversionModelsQuery,
|
||||
useGetVaeModelsQuery,
|
||||
|
498
invokeai/frontend/web/src/services/api/schema.d.ts
vendored
498
invokeai/frontend/web/src/services/api/schema.d.ts
vendored
File diff suppressed because one or more lines are too long
@ -60,8 +60,10 @@ export type OnnxModelField = s['OnnxModelField'];
|
||||
export type VAEModelField = s['VAEModelField'];
|
||||
export type LoRAModelField = s['LoRAModelField'];
|
||||
export type ControlNetModelField = s['ControlNetModelField'];
|
||||
export type IPAdapterModelField = s['IPAdapterModelField'];
|
||||
export type ModelsList = s['ModelsList'];
|
||||
export type ControlField = s['ControlField'];
|
||||
export type IPAdapterField = s['IPAdapterField'];
|
||||
|
||||
// Model Configs
|
||||
export type LoRAModelConfig = s['LoRAModelConfig'];
|
||||
@ -73,6 +75,8 @@ export type ControlNetModelDiffusersConfig =
|
||||
export type ControlNetModelConfig =
|
||||
| ControlNetModelCheckpointConfig
|
||||
| ControlNetModelDiffusersConfig;
|
||||
export type IPAdapterModelInvokeAIConfig = s['IPAdapterModelInvokeAIConfig'];
|
||||
export type IPAdapterModelConfig = IPAdapterModelInvokeAIConfig;
|
||||
export type TextualInversionModelConfig = s['TextualInversionModelConfig'];
|
||||
export type DiffusersModelConfig =
|
||||
| s['StableDiffusion1ModelDiffusersConfig']
|
||||
@ -88,6 +92,7 @@ export type AnyModelConfig =
|
||||
| LoRAModelConfig
|
||||
| VaeModelConfig
|
||||
| ControlNetModelConfig
|
||||
| IPAdapterModelConfig
|
||||
| TextualInversionModelConfig
|
||||
| MainModelConfig
|
||||
| OnnxModelConfig;
|
||||
@ -135,6 +140,7 @@ export type SeamlessModeInvocation = s['SeamlessModeInvocation'];
|
||||
|
||||
// ControlNet Nodes
|
||||
export type ControlNetInvocation = s['ControlNetInvocation'];
|
||||
export type IPAdapterInvocation = s['IPAdapterInvocation'];
|
||||
export type CannyImageProcessorInvocation = s['CannyImageProcessorInvocation'];
|
||||
export type ContentShuffleImageProcessorInvocation =
|
||||
s['ContentShuffleImageProcessorInvocation'];
|
||||
@ -173,6 +179,10 @@ export type ControlNetAction = {
|
||||
controlNetId: string;
|
||||
};
|
||||
|
||||
export type IPAdapterAction = {
|
||||
type: 'SET_IP_ADAPTER_IMAGE';
|
||||
};
|
||||
|
||||
export type InitialImageAction = {
|
||||
type: 'SET_INITIAL_IMAGE';
|
||||
};
|
||||
@ -198,6 +208,7 @@ export type AddToBatchAction = {
|
||||
|
||||
export type PostUploadAction =
|
||||
| ControlNetAction
|
||||
| IPAdapterAction
|
||||
| InitialImageAction
|
||||
| NodesAction
|
||||
| CanvasInitialImageAction
|
||||
|
Loading…
x
Reference in New Issue
Block a user