mirror of
https://github.com/invoke-ai/InvokeAI
synced 2024-08-30 20:32:17 +00:00
Add control_mode parameter to ControlNet (#3535)
This PR adds the "control_mode" option to ControlNet implementation. Possible control_mode options are: - balanced -- this is the default, same as previous implementation without control_mode - more_prompt -- pays more attention to the prompt - more _control -- pays more attention to the ControlNet (in earlier implementations this was called "guess_mode") - unbalanced -- pays even more attention to the ControlNet balanced, more_prompt, and more_control should be nearly identical to the equivalent options in the [auto1111 sd-webui-controlnet extension](https://github.com/Mikubill/sd-webui-controlnet#more-control-modes-previously-called-guess-mode) The changes to enable balanced, more_prompt, and more_control are managed deeper in the code by two booleans, "soft_injection" and "cfg_injection". The three control mode options in sd-webui-controlnet map to these booleans like: !soft_injection && !cfg_injection ⇒ BALANCED soft_injection && cfg_injection ⇒ MORE_CONTROL soft_injection && !cfg_injection ⇒ MORE_PROMPT The "unbalanced" option simply exposes the fourth possible combination of these two booleans: !soft_injection && cfg_injection ⇒ UNBALANCED With "unbalanced" mode it is very easy to overdrive the controlnet inputs. It's recommended to use a cfg_scale between 2 and 4 to mitigate this, along with lowering controlnet weight and possibly lowering "end step percent". With those caveats, "unbalanced" can yield interesting results. Example of all four modes using Canny edge detection ControlNet with prompt "old man", identical params except for control_mode: 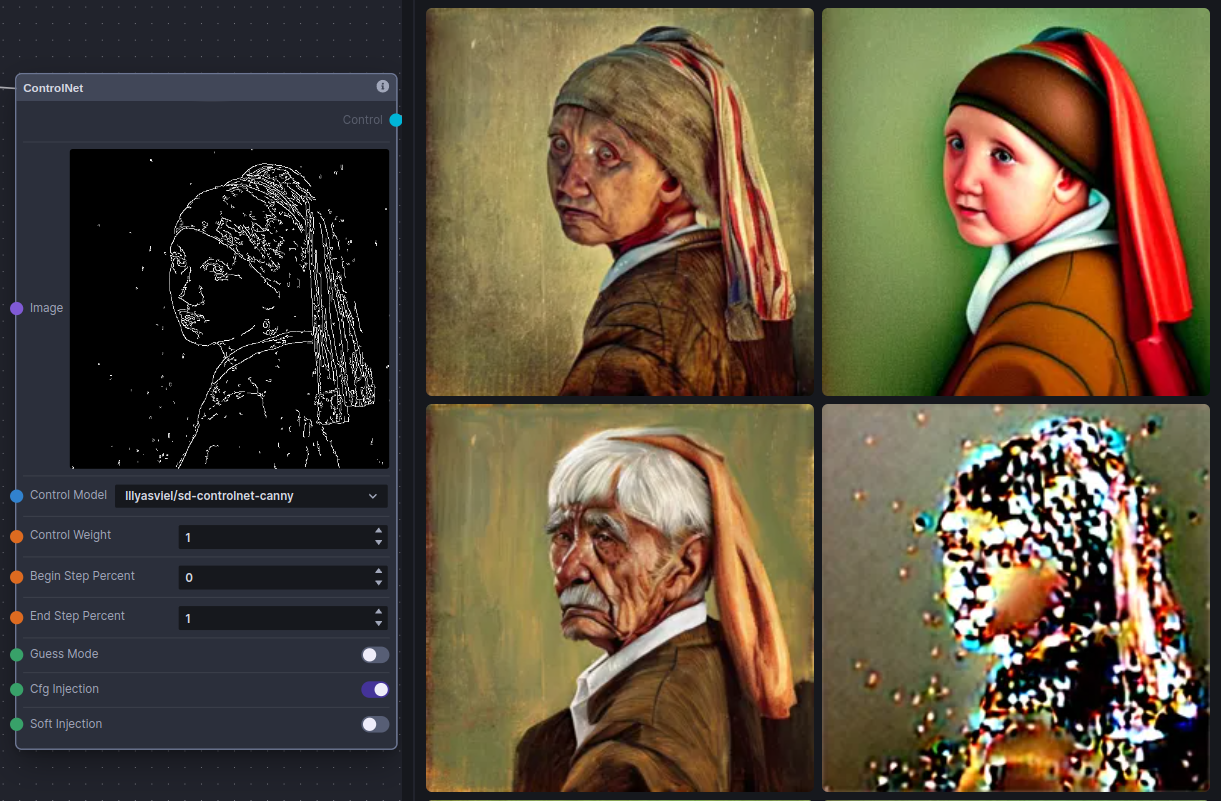 Top middle: BALANCED Top right: MORE_CONTROL Bottom middle: MORE_PROMPT Bottom right : UNBALANCED I kind of chose this seed because it shows pretty rough results with BALANCED (the default), but in my opinion better results with both MORE_CONTROL and MORE_PROMPT. And you can definitely see how MORE_PROMPT pays more attention to the prompt, and MORE_CONTROL pays more attention to the control image. And shows that UNBALANCED with default cfg_scale etc is unusable. But here are four examples from same series (same seed etc), all have control_mode = UNBALANCED but now cfg_scale is set to 3.  And param differences are: Top middle: prompt="old man", control_weight=0.3, end_step_percent=0.5 Top right: prompt="old man", control_weight=0.4, end_step_percent=1.0 Bottom middle: prompt=None, control_weight=0.3, end_step_percent=0.5 Bottom right: prompt=None, control_weight=0.4, end_step_percent=1.0 So with the right settings UNBALANCED seems useful.
This commit is contained in:
commit
922468b836
@ -1,7 +1,7 @@
|
||||
# InvokeAI nodes for ControlNet image preprocessors
|
||||
# initial implementation by Gregg Helt, 2023
|
||||
# heavily leverages controlnet_aux package: https://github.com/patrickvonplaten/controlnet_aux
|
||||
from builtins import float
|
||||
from builtins import float, bool
|
||||
|
||||
import numpy as np
|
||||
from typing import Literal, Optional, Union, List
|
||||
@ -94,6 +94,7 @@ CONTROLNET_DEFAULT_MODELS = [
|
||||
]
|
||||
|
||||
CONTROLNET_NAME_VALUES = Literal[tuple(CONTROLNET_DEFAULT_MODELS)]
|
||||
CONTROLNET_MODE_VALUES = Literal[tuple(["balanced", "more_prompt", "more_control", "unbalanced"])]
|
||||
|
||||
class ControlField(BaseModel):
|
||||
image: ImageField = Field(default=None, description="The control image")
|
||||
@ -104,6 +105,8 @@ class ControlField(BaseModel):
|
||||
description="When the ControlNet is first applied (% of total steps)")
|
||||
end_step_percent: float = Field(default=1, ge=0, le=1,
|
||||
description="When the ControlNet is last applied (% of total steps)")
|
||||
control_mode: CONTROLNET_MODE_VALUES = Field(default="balanced", description="The contorl mode to use")
|
||||
|
||||
@validator("control_weight")
|
||||
def abs_le_one(cls, v):
|
||||
"""validate that all abs(values) are <=1"""
|
||||
@ -144,11 +147,11 @@ class ControlNetInvocation(BaseInvocation):
|
||||
control_model: CONTROLNET_NAME_VALUES = Field(default="lllyasviel/sd-controlnet-canny",
|
||||
description="control model used")
|
||||
control_weight: Union[float, List[float]] = Field(default=1.0, description="The weight given to the ControlNet")
|
||||
# TODO: add support in backend core for begin_step_percent, end_step_percent, guess_mode
|
||||
begin_step_percent: float = Field(default=0, ge=0, le=1,
|
||||
description="When the ControlNet is first applied (% of total steps)")
|
||||
end_step_percent: float = Field(default=1, ge=0, le=1,
|
||||
description="When the ControlNet is last applied (% of total steps)")
|
||||
control_mode: CONTROLNET_MODE_VALUES = Field(default="balanced", description="The control mode used")
|
||||
# fmt: on
|
||||
|
||||
class Config(InvocationConfig):
|
||||
@ -166,7 +169,6 @@ class ControlNetInvocation(BaseInvocation):
|
||||
}
|
||||
|
||||
def invoke(self, context: InvocationContext) -> ControlOutput:
|
||||
|
||||
return ControlOutput(
|
||||
control=ControlField(
|
||||
image=self.image,
|
||||
@ -174,6 +176,7 @@ class ControlNetInvocation(BaseInvocation):
|
||||
control_weight=self.control_weight,
|
||||
begin_step_percent=self.begin_step_percent,
|
||||
end_step_percent=self.end_step_percent,
|
||||
control_mode=self.control_mode,
|
||||
),
|
||||
)
|
||||
|
||||
|
@ -287,19 +287,14 @@ class TextToLatentsInvocation(BaseInvocation):
|
||||
control_height_resize = latents_shape[2] * 8
|
||||
control_width_resize = latents_shape[3] * 8
|
||||
if control_input is None:
|
||||
# print("control input is None")
|
||||
control_list = None
|
||||
elif isinstance(control_input, list) and len(control_input) == 0:
|
||||
# print("control input is empty list")
|
||||
control_list = None
|
||||
elif isinstance(control_input, ControlField):
|
||||
# print("control input is ControlField")
|
||||
control_list = [control_input]
|
||||
elif isinstance(control_input, list) and len(control_input) > 0 and isinstance(control_input[0], ControlField):
|
||||
# print("control input is list[ControlField]")
|
||||
control_list = control_input
|
||||
else:
|
||||
# print("input control is unrecognized:", type(self.control))
|
||||
control_list = None
|
||||
if (control_list is None):
|
||||
control_data = None
|
||||
@ -341,12 +336,15 @@ class TextToLatentsInvocation(BaseInvocation):
|
||||
# num_images_per_prompt=num_images_per_prompt,
|
||||
device=control_model.device,
|
||||
dtype=control_model.dtype,
|
||||
control_mode=control_info.control_mode,
|
||||
)
|
||||
control_item = ControlNetData(model=control_model,
|
||||
image_tensor=control_image,
|
||||
weight=control_info.control_weight,
|
||||
begin_step_percent=control_info.begin_step_percent,
|
||||
end_step_percent=control_info.end_step_percent)
|
||||
end_step_percent=control_info.end_step_percent,
|
||||
control_mode=control_info.control_mode,
|
||||
)
|
||||
control_data.append(control_item)
|
||||
# MultiControlNetModel has been refactored out, just need list[ControlNetData]
|
||||
return control_data
|
||||
|
@ -219,6 +219,8 @@ class ControlNetData:
|
||||
weight: Union[float, List[float]] = Field(default=1.0)
|
||||
begin_step_percent: float = Field(default=0.0)
|
||||
end_step_percent: float = Field(default=1.0)
|
||||
control_mode: str = Field(default="balanced")
|
||||
|
||||
|
||||
@dataclass(frozen=True)
|
||||
class ConditioningData:
|
||||
@ -599,48 +601,68 @@ class StableDiffusionGeneratorPipeline(StableDiffusionPipeline):
|
||||
|
||||
# TODO: should this scaling happen here or inside self._unet_forward?
|
||||
# i.e. before or after passing it to InvokeAIDiffuserComponent
|
||||
latent_model_input = self.scheduler.scale_model_input(latents, timestep)
|
||||
unet_latent_input = self.scheduler.scale_model_input(latents, timestep)
|
||||
|
||||
# default is no controlnet, so set controlnet processing output to None
|
||||
down_block_res_samples, mid_block_res_sample = None, None
|
||||
|
||||
if control_data is not None:
|
||||
# FIXME: make sure guidance_scale < 1.0 is handled correctly if doing per-step guidance setting
|
||||
# if conditioning_data.guidance_scale > 1.0:
|
||||
if conditioning_data.guidance_scale is not None:
|
||||
# expand the latents input to control model if doing classifier free guidance
|
||||
# (which I think for now is always true, there is conditional elsewhere that stops execution if
|
||||
# classifier_free_guidance is <= 1.0 ?)
|
||||
latent_control_input = torch.cat([latent_model_input] * 2)
|
||||
else:
|
||||
latent_control_input = latent_model_input
|
||||
# control_data should be type List[ControlNetData]
|
||||
# this loop covers both ControlNet (one ControlNetData in list)
|
||||
# and MultiControlNet (multiple ControlNetData in list)
|
||||
for i, control_datum in enumerate(control_data):
|
||||
# print("controlnet", i, "==>", type(control_datum))
|
||||
control_mode = control_datum.control_mode
|
||||
# soft_injection and cfg_injection are the two ControlNet control_mode booleans
|
||||
# that are combined at higher level to make control_mode enum
|
||||
# soft_injection determines whether to do per-layer re-weighting adjustment (if True)
|
||||
# or default weighting (if False)
|
||||
soft_injection = (control_mode == "more_prompt" or control_mode == "more_control")
|
||||
# cfg_injection = determines whether to apply ControlNet to only the conditional (if True)
|
||||
# or the default both conditional and unconditional (if False)
|
||||
cfg_injection = (control_mode == "more_control" or control_mode == "unbalanced")
|
||||
|
||||
first_control_step = math.floor(control_datum.begin_step_percent * total_step_count)
|
||||
last_control_step = math.ceil(control_datum.end_step_percent * total_step_count)
|
||||
# only apply controlnet if current step is within the controlnet's begin/end step range
|
||||
if step_index >= first_control_step and step_index <= last_control_step:
|
||||
# print("running controlnet", i, "for step", step_index)
|
||||
|
||||
if cfg_injection:
|
||||
control_latent_input = unet_latent_input
|
||||
else:
|
||||
# expand the latents input to control model if doing classifier free guidance
|
||||
# (which I think for now is always true, there is conditional elsewhere that stops execution if
|
||||
# classifier_free_guidance is <= 1.0 ?)
|
||||
control_latent_input = torch.cat([unet_latent_input] * 2)
|
||||
|
||||
if cfg_injection: # only applying ControlNet to conditional instead of in unconditioned
|
||||
encoder_hidden_states = torch.cat([conditioning_data.unconditioned_embeddings])
|
||||
else:
|
||||
encoder_hidden_states = torch.cat([conditioning_data.unconditioned_embeddings,
|
||||
conditioning_data.text_embeddings])
|
||||
if isinstance(control_datum.weight, list):
|
||||
# if controlnet has multiple weights, use the weight for the current step
|
||||
controlnet_weight = control_datum.weight[step_index]
|
||||
else:
|
||||
# if controlnet has a single weight, use it for all steps
|
||||
controlnet_weight = control_datum.weight
|
||||
|
||||
# controlnet(s) inference
|
||||
down_samples, mid_sample = control_datum.model(
|
||||
sample=latent_control_input,
|
||||
sample=control_latent_input,
|
||||
timestep=timestep,
|
||||
encoder_hidden_states=torch.cat([conditioning_data.unconditioned_embeddings,
|
||||
conditioning_data.text_embeddings]),
|
||||
encoder_hidden_states=encoder_hidden_states,
|
||||
controlnet_cond=control_datum.image_tensor,
|
||||
conditioning_scale=controlnet_weight,
|
||||
# cross_attention_kwargs,
|
||||
guess_mode=False,
|
||||
conditioning_scale=controlnet_weight, # controlnet specific, NOT the guidance scale
|
||||
guess_mode=soft_injection, # this is still called guess_mode in diffusers ControlNetModel
|
||||
return_dict=False,
|
||||
)
|
||||
if cfg_injection:
|
||||
# Inferred ControlNet only for the conditional batch.
|
||||
# To apply the output of ControlNet to both the unconditional and conditional batches,
|
||||
# add 0 to the unconditional batch to keep it unchanged.
|
||||
down_samples = [torch.cat([torch.zeros_like(d), d]) for d in down_samples]
|
||||
mid_sample = torch.cat([torch.zeros_like(mid_sample), mid_sample])
|
||||
|
||||
if down_block_res_samples is None and mid_block_res_sample is None:
|
||||
down_block_res_samples, mid_block_res_sample = down_samples, mid_sample
|
||||
else:
|
||||
@ -653,11 +675,11 @@ class StableDiffusionGeneratorPipeline(StableDiffusionPipeline):
|
||||
|
||||
# predict the noise residual
|
||||
noise_pred = self.invokeai_diffuser.do_diffusion_step(
|
||||
latent_model_input,
|
||||
t,
|
||||
conditioning_data.unconditioned_embeddings,
|
||||
conditioning_data.text_embeddings,
|
||||
conditioning_data.guidance_scale,
|
||||
x=unet_latent_input,
|
||||
sigma=t,
|
||||
unconditioning=conditioning_data.unconditioned_embeddings,
|
||||
conditioning=conditioning_data.text_embeddings,
|
||||
unconditional_guidance_scale=conditioning_data.guidance_scale,
|
||||
step_index=step_index,
|
||||
total_step_count=total_step_count,
|
||||
down_block_additional_residuals=down_block_res_samples, # from controlnet(s)
|
||||
@ -962,6 +984,7 @@ class StableDiffusionGeneratorPipeline(StableDiffusionPipeline):
|
||||
device="cuda",
|
||||
dtype=torch.float16,
|
||||
do_classifier_free_guidance=True,
|
||||
control_mode="balanced"
|
||||
):
|
||||
|
||||
if not isinstance(image, torch.Tensor):
|
||||
@ -992,6 +1015,7 @@ class StableDiffusionGeneratorPipeline(StableDiffusionPipeline):
|
||||
repeat_by = num_images_per_prompt
|
||||
image = image.repeat_interleave(repeat_by, dim=0)
|
||||
image = image.to(device=device, dtype=dtype)
|
||||
if do_classifier_free_guidance:
|
||||
cfg_injection = (control_mode == "more_control" or control_mode == "unbalanced")
|
||||
if do_classifier_free_guidance and not cfg_injection:
|
||||
image = torch.cat([image] * 2)
|
||||
return image
|
||||
|
@ -23,7 +23,7 @@
|
||||
"dev": "concurrently \"vite dev\" \"yarn run theme:watch\"",
|
||||
"dev:host": "concurrently \"vite dev --host\" \"yarn run theme:watch\"",
|
||||
"build": "yarn run lint && vite build",
|
||||
"typegen": "npx openapi-typescript http://localhost:9090/openapi.json --output src/services/schema.d.ts -t",
|
||||
"typegen": "npx openapi-typescript http://localhost:9090/openapi.json --output src/services/api/schema.d.ts -t",
|
||||
"preview": "vite preview",
|
||||
"lint:madge": "madge --circular src/main.tsx",
|
||||
"lint:eslint": "eslint --max-warnings=0 .",
|
||||
|
@ -524,7 +524,8 @@
|
||||
"initialImage": "Initial Image",
|
||||
"showOptionsPanel": "Show Options Panel",
|
||||
"hidePreview": "Hide Preview",
|
||||
"showPreview": "Show Preview"
|
||||
"showPreview": "Show Preview",
|
||||
"controlNetControlMode": "Control Mode"
|
||||
},
|
||||
"settings": {
|
||||
"models": "Models",
|
||||
|
@ -1,26 +1,27 @@
|
||||
import { Box, ChakraProps, Flex } from '@chakra-ui/react';
|
||||
import { useAppDispatch } from 'app/store/storeHooks';
|
||||
import { memo, useCallback } from 'react';
|
||||
import { FaCopy, FaTrash } from 'react-icons/fa';
|
||||
import {
|
||||
ControlNetConfig,
|
||||
controlNetAdded,
|
||||
controlNetRemoved,
|
||||
controlNetToggled,
|
||||
} from '../store/controlNetSlice';
|
||||
import { useAppDispatch } from 'app/store/storeHooks';
|
||||
import ParamControlNetModel from './parameters/ParamControlNetModel';
|
||||
import ParamControlNetWeight from './parameters/ParamControlNetWeight';
|
||||
import { Flex, Box, ChakraProps } from '@chakra-ui/react';
|
||||
import { FaCopy, FaTrash } from 'react-icons/fa';
|
||||
|
||||
import ParamControlNetBeginEnd from './parameters/ParamControlNetBeginEnd';
|
||||
import ControlNetImagePreview from './ControlNetImagePreview';
|
||||
import IAIIconButton from 'common/components/IAIIconButton';
|
||||
import { v4 as uuidv4 } from 'uuid';
|
||||
import { useToggle } from 'react-use';
|
||||
import ParamControlNetProcessorSelect from './parameters/ParamControlNetProcessorSelect';
|
||||
import ControlNetProcessorComponent from './ControlNetProcessorComponent';
|
||||
import IAISwitch from 'common/components/IAISwitch';
|
||||
import { ChevronUpIcon } from '@chakra-ui/icons';
|
||||
import IAIIconButton from 'common/components/IAIIconButton';
|
||||
import IAISwitch from 'common/components/IAISwitch';
|
||||
import { useToggle } from 'react-use';
|
||||
import { v4 as uuidv4 } from 'uuid';
|
||||
import ControlNetImagePreview from './ControlNetImagePreview';
|
||||
import ControlNetProcessorComponent from './ControlNetProcessorComponent';
|
||||
import ParamControlNetShouldAutoConfig from './ParamControlNetShouldAutoConfig';
|
||||
import ParamControlNetBeginEnd from './parameters/ParamControlNetBeginEnd';
|
||||
import ParamControlNetControlMode from './parameters/ParamControlNetControlMode';
|
||||
import ParamControlNetProcessorSelect from './parameters/ParamControlNetProcessorSelect';
|
||||
|
||||
const expandedControlImageSx: ChakraProps['sx'] = { maxH: 96 };
|
||||
|
||||
@ -36,6 +37,7 @@ const ControlNet = (props: ControlNetProps) => {
|
||||
weight,
|
||||
beginStepPct,
|
||||
endStepPct,
|
||||
controlMode,
|
||||
controlImage,
|
||||
processedControlImage,
|
||||
processorNode,
|
||||
@ -137,13 +139,13 @@ const ControlNet = (props: ControlNetProps) => {
|
||||
</Flex>
|
||||
{isEnabled && (
|
||||
<>
|
||||
<Flex sx={{ w: 'full', flexDirection: 'column' }}>
|
||||
<Flex sx={{ gap: 4, w: 'full' }}>
|
||||
<Flex
|
||||
sx={{
|
||||
flexDir: 'column',
|
||||
gap: 2,
|
||||
gap: 3,
|
||||
w: 'full',
|
||||
h: isExpanded ? 28 : 24,
|
||||
paddingInlineStart: 1,
|
||||
paddingInlineEnd: isExpanded ? 1 : 0,
|
||||
pb: 2,
|
||||
@ -172,13 +174,16 @@ const ControlNet = (props: ControlNetProps) => {
|
||||
aspectRatio: '1/1',
|
||||
}}
|
||||
>
|
||||
<ControlNetImagePreview
|
||||
controlNet={props.controlNet}
|
||||
height={24}
|
||||
/>
|
||||
<ControlNetImagePreview controlNet={props.controlNet} />
|
||||
</Flex>
|
||||
)}
|
||||
</Flex>
|
||||
<ParamControlNetControlMode
|
||||
controlNetId={controlNetId}
|
||||
controlMode={controlMode}
|
||||
/>
|
||||
</Flex>
|
||||
|
||||
{isExpanded && (
|
||||
<>
|
||||
<Box mt={2}>
|
||||
|
@ -0,0 +1,45 @@
|
||||
import { useAppDispatch } from 'app/store/storeHooks';
|
||||
import IAIMantineSelect from 'common/components/IAIMantineSelect';
|
||||
import {
|
||||
ControlModes,
|
||||
controlNetControlModeChanged,
|
||||
} from 'features/controlNet/store/controlNetSlice';
|
||||
import { useCallback } from 'react';
|
||||
import { useTranslation } from 'react-i18next';
|
||||
|
||||
type ParamControlNetControlModeProps = {
|
||||
controlNetId: string;
|
||||
controlMode: string;
|
||||
};
|
||||
|
||||
const CONTROL_MODE_DATA = [
|
||||
{ label: 'Balanced', value: 'balanced' },
|
||||
{ label: 'Prompt', value: 'more_prompt' },
|
||||
{ label: 'Control', value: 'more_control' },
|
||||
{ label: 'Mega Control', value: 'unbalanced' },
|
||||
];
|
||||
|
||||
export default function ParamControlNetControlMode(
|
||||
props: ParamControlNetControlModeProps
|
||||
) {
|
||||
const { controlNetId, controlMode = false } = props;
|
||||
const dispatch = useAppDispatch();
|
||||
|
||||
const { t } = useTranslation();
|
||||
|
||||
const handleControlModeChange = useCallback(
|
||||
(controlMode: ControlModes) => {
|
||||
dispatch(controlNetControlModeChanged({ controlNetId, controlMode }));
|
||||
},
|
||||
[controlNetId, dispatch]
|
||||
);
|
||||
|
||||
return (
|
||||
<IAIMantineSelect
|
||||
label={t('parameters.controlNetControlMode')}
|
||||
data={CONTROL_MODE_DATA}
|
||||
value={String(controlMode)}
|
||||
onChange={handleControlModeChange}
|
||||
/>
|
||||
);
|
||||
}
|
@ -1,6 +1,5 @@
|
||||
import {
|
||||
ControlNetProcessorType,
|
||||
RequiredCannyImageProcessorInvocation,
|
||||
RequiredControlNetProcessorNode,
|
||||
} from './types';
|
||||
|
||||
@ -23,7 +22,7 @@ type ControlNetProcessorsDict = Record<
|
||||
*
|
||||
* TODO: Generate from the OpenAPI schema
|
||||
*/
|
||||
export const CONTROLNET_PROCESSORS = {
|
||||
export const CONTROLNET_PROCESSORS: ControlNetProcessorsDict = {
|
||||
none: {
|
||||
type: 'none',
|
||||
label: 'none',
|
||||
@ -174,6 +173,8 @@ export const CONTROLNET_PROCESSORS = {
|
||||
},
|
||||
};
|
||||
|
||||
type ControlNetModelsDict = Record<string, ControlNetModel>;
|
||||
|
||||
type ControlNetModel = {
|
||||
type: string;
|
||||
label: string;
|
||||
@ -181,7 +182,7 @@ type ControlNetModel = {
|
||||
defaultProcessor?: ControlNetProcessorType;
|
||||
};
|
||||
|
||||
export const CONTROLNET_MODELS = {
|
||||
export const CONTROLNET_MODELS: ControlNetModelsDict = {
|
||||
'lllyasviel/control_v11p_sd15_canny': {
|
||||
type: 'lllyasviel/control_v11p_sd15_canny',
|
||||
label: 'Canny',
|
||||
@ -190,6 +191,7 @@ export const CONTROLNET_MODELS = {
|
||||
'lllyasviel/control_v11p_sd15_inpaint': {
|
||||
type: 'lllyasviel/control_v11p_sd15_inpaint',
|
||||
label: 'Inpaint',
|
||||
defaultProcessor: 'none',
|
||||
},
|
||||
'lllyasviel/control_v11p_sd15_mlsd': {
|
||||
type: 'lllyasviel/control_v11p_sd15_mlsd',
|
||||
@ -209,6 +211,7 @@ export const CONTROLNET_MODELS = {
|
||||
'lllyasviel/control_v11p_sd15_seg': {
|
||||
type: 'lllyasviel/control_v11p_sd15_seg',
|
||||
label: 'Segmentation',
|
||||
defaultProcessor: 'none',
|
||||
},
|
||||
'lllyasviel/control_v11p_sd15_lineart': {
|
||||
type: 'lllyasviel/control_v11p_sd15_lineart',
|
||||
@ -223,6 +226,7 @@ export const CONTROLNET_MODELS = {
|
||||
'lllyasviel/control_v11p_sd15_scribble': {
|
||||
type: 'lllyasviel/control_v11p_sd15_scribble',
|
||||
label: 'Scribble',
|
||||
defaultProcessor: 'none',
|
||||
},
|
||||
'lllyasviel/control_v11p_sd15_softedge': {
|
||||
type: 'lllyasviel/control_v11p_sd15_softedge',
|
||||
@ -242,10 +246,12 @@ export const CONTROLNET_MODELS = {
|
||||
'lllyasviel/control_v11f1e_sd15_tile': {
|
||||
type: 'lllyasviel/control_v11f1e_sd15_tile',
|
||||
label: 'Tile (experimental)',
|
||||
defaultProcessor: 'none',
|
||||
},
|
||||
'lllyasviel/control_v11e_sd15_ip2p': {
|
||||
type: 'lllyasviel/control_v11e_sd15_ip2p',
|
||||
label: 'Pix2Pix (experimental)',
|
||||
defaultProcessor: 'none',
|
||||
},
|
||||
'CrucibleAI/ControlNetMediaPipeFace': {
|
||||
type: 'CrucibleAI/ControlNetMediaPipeFace',
|
||||
|
@ -18,12 +18,19 @@ import { forEach } from 'lodash-es';
|
||||
import { isAnySessionRejected } from 'services/api/thunks/session';
|
||||
import { appSocketInvocationError } from 'services/events/actions';
|
||||
|
||||
export type ControlModes =
|
||||
| 'balanced'
|
||||
| 'more_prompt'
|
||||
| 'more_control'
|
||||
| 'unbalanced';
|
||||
|
||||
export const initialControlNet: Omit<ControlNetConfig, 'controlNetId'> = {
|
||||
isEnabled: true,
|
||||
model: CONTROLNET_MODELS['lllyasviel/control_v11p_sd15_canny'].type,
|
||||
weight: 1,
|
||||
beginStepPct: 0,
|
||||
endStepPct: 1,
|
||||
controlMode: 'balanced',
|
||||
controlImage: null,
|
||||
processedControlImage: null,
|
||||
processorType: 'canny_image_processor',
|
||||
@ -39,6 +46,7 @@ export type ControlNetConfig = {
|
||||
weight: number;
|
||||
beginStepPct: number;
|
||||
endStepPct: number;
|
||||
controlMode: ControlModes;
|
||||
controlImage: string | null;
|
||||
processedControlImage: string | null;
|
||||
processorType: ControlNetProcessorType;
|
||||
@ -181,6 +189,13 @@ export const controlNetSlice = createSlice({
|
||||
const { controlNetId, endStepPct } = action.payload;
|
||||
state.controlNets[controlNetId].endStepPct = endStepPct;
|
||||
},
|
||||
controlNetControlModeChanged: (
|
||||
state,
|
||||
action: PayloadAction<{ controlNetId: string; controlMode: ControlModes }>
|
||||
) => {
|
||||
const { controlNetId, controlMode } = action.payload;
|
||||
state.controlNets[controlNetId].controlMode = controlMode;
|
||||
},
|
||||
controlNetProcessorParamsChanged: (
|
||||
state,
|
||||
action: PayloadAction<{
|
||||
@ -307,6 +322,7 @@ export const {
|
||||
controlNetWeightChanged,
|
||||
controlNetBeginStepPctChanged,
|
||||
controlNetEndStepPctChanged,
|
||||
controlNetControlModeChanged,
|
||||
controlNetProcessorParamsChanged,
|
||||
controlNetProcessorTypeChanged,
|
||||
controlNetReset,
|
||||
|
@ -44,6 +44,7 @@ export const addControlNetToLinearGraph = (
|
||||
processedControlImage,
|
||||
beginStepPct,
|
||||
endStepPct,
|
||||
controlMode,
|
||||
model,
|
||||
processorType,
|
||||
weight,
|
||||
@ -59,6 +60,7 @@ export const addControlNetToLinearGraph = (
|
||||
type: 'controlnet',
|
||||
begin_step_percent: beginStepPct,
|
||||
end_step_percent: endStepPct,
|
||||
control_mode: controlMode,
|
||||
control_model: model as ControlNetInvocation['control_model'],
|
||||
control_weight: weight,
|
||||
};
|
||||
|
@ -0,0 +1,36 @@
|
||||
/* istanbul ignore file */
|
||||
/* tslint:disable */
|
||||
/* eslint-disable */
|
||||
|
||||
import type { ImageField } from './ImageField';
|
||||
|
||||
/**
|
||||
* Applies HED edge detection to image
|
||||
*/
|
||||
export type HedImageProcessorInvocation = {
|
||||
/**
|
||||
* The id of this node. Must be unique among all nodes.
|
||||
*/
|
||||
id: string;
|
||||
/**
|
||||
* Whether or not this node is an intermediate node.
|
||||
*/
|
||||
is_intermediate?: boolean;
|
||||
type?: 'hed_image_processor';
|
||||
/**
|
||||
* The image to process
|
||||
*/
|
||||
image?: ImageField;
|
||||
/**
|
||||
* The pixel resolution for detection
|
||||
*/
|
||||
detect_resolution?: number;
|
||||
/**
|
||||
* The pixel resolution for the output image
|
||||
*/
|
||||
image_resolution?: number;
|
||||
/**
|
||||
* Whether to use scribble mode
|
||||
*/
|
||||
scribble?: boolean;
|
||||
};
|
@ -648,6 +648,13 @@ export type components = {
|
||||
* @default 1
|
||||
*/
|
||||
end_step_percent: number;
|
||||
/**
|
||||
* Control Mode
|
||||
* @description The contorl mode to use
|
||||
* @default balanced
|
||||
* @enum {string}
|
||||
*/
|
||||
control_mode?: "balanced" | "more_prompt" | "more_control" | "unbalanced";
|
||||
};
|
||||
/**
|
||||
* ControlNetInvocation
|
||||
@ -701,6 +708,13 @@ export type components = {
|
||||
* @default 1
|
||||
*/
|
||||
end_step_percent?: number;
|
||||
/**
|
||||
* Control Mode
|
||||
* @description The control mode used
|
||||
* @default balanced
|
||||
* @enum {string}
|
||||
*/
|
||||
control_mode?: "balanced" | "more_prompt" | "more_control" | "unbalanced";
|
||||
};
|
||||
/** ControlNetModelConfig */
|
||||
ControlNetModelConfig: {
|
||||
@ -2903,7 +2917,7 @@ export type components = {
|
||||
/** ModelsList */
|
||||
ModelsList: {
|
||||
/** Models */
|
||||
models: (components["schemas"]["StableDiffusion1ModelCheckpointConfig"] | components["schemas"]["StableDiffusion1ModelDiffusersConfig"] | components["schemas"]["VaeModelConfig"] | components["schemas"]["LoRAModelConfig"] | components["schemas"]["ControlNetModelConfig"] | components["schemas"]["TextualInversionModelConfig"] | components["schemas"]["StableDiffusion2ModelDiffusersConfig"] | components["schemas"]["StableDiffusion2ModelCheckpointConfig"])[];
|
||||
models: (components["schemas"]["StableDiffusion1ModelDiffusersConfig"] | components["schemas"]["StableDiffusion1ModelCheckpointConfig"] | components["schemas"]["VaeModelConfig"] | components["schemas"]["LoRAModelConfig"] | components["schemas"]["ControlNetModelConfig"] | components["schemas"]["TextualInversionModelConfig"] | components["schemas"]["StableDiffusion2ModelCheckpointConfig"] | components["schemas"]["StableDiffusion2ModelDiffusersConfig"])[];
|
||||
};
|
||||
/**
|
||||
* MultiplyInvocation
|
||||
@ -4163,18 +4177,18 @@ export type components = {
|
||||
*/
|
||||
image?: components["schemas"]["ImageField"];
|
||||
};
|
||||
/**
|
||||
* StableDiffusion1ModelFormat
|
||||
* @description An enumeration.
|
||||
* @enum {string}
|
||||
*/
|
||||
StableDiffusion1ModelFormat: "checkpoint" | "diffusers";
|
||||
/**
|
||||
* StableDiffusion2ModelFormat
|
||||
* @description An enumeration.
|
||||
* @enum {string}
|
||||
*/
|
||||
StableDiffusion2ModelFormat: "checkpoint" | "diffusers";
|
||||
/**
|
||||
* StableDiffusion1ModelFormat
|
||||
* @description An enumeration.
|
||||
* @enum {string}
|
||||
*/
|
||||
StableDiffusion1ModelFormat: "checkpoint" | "diffusers";
|
||||
};
|
||||
responses: never;
|
||||
parameters: never;
|
||||
|
@ -1,81 +1,78 @@
|
||||
import { components } from './schema';
|
||||
|
||||
type schemas = components['schemas'];
|
||||
|
||||
/**
|
||||
* Types from the API, re-exported from the types generated by `openapi-typescript`.
|
||||
*/
|
||||
|
||||
// Images
|
||||
export type ImageDTO = components['schemas']['ImageDTO'];
|
||||
export type BoardDTO = components['schemas']['BoardDTO'];
|
||||
export type BoardChanges = components['schemas']['BoardChanges'];
|
||||
export type ImageChanges = components['schemas']['ImageRecordChanges'];
|
||||
export type ImageCategory = components['schemas']['ImageCategory'];
|
||||
export type ResourceOrigin = components['schemas']['ResourceOrigin'];
|
||||
export type ImageField = components['schemas']['ImageField'];
|
||||
export type ImageDTO = schemas['ImageDTO'];
|
||||
export type BoardDTO = schemas['BoardDTO'];
|
||||
export type BoardChanges = schemas['BoardChanges'];
|
||||
export type ImageChanges = schemas['ImageRecordChanges'];
|
||||
export type ImageCategory = schemas['ImageCategory'];
|
||||
export type ResourceOrigin = schemas['ResourceOrigin'];
|
||||
export type ImageField = schemas['ImageField'];
|
||||
export type OffsetPaginatedResults_BoardDTO_ =
|
||||
components['schemas']['OffsetPaginatedResults_BoardDTO_'];
|
||||
schemas['OffsetPaginatedResults_BoardDTO_'];
|
||||
export type OffsetPaginatedResults_ImageDTO_ =
|
||||
components['schemas']['OffsetPaginatedResults_ImageDTO_'];
|
||||
schemas['OffsetPaginatedResults_ImageDTO_'];
|
||||
|
||||
// Models
|
||||
export type ModelType = components['schemas']['ModelType'];
|
||||
export type BaseModelType = components['schemas']['BaseModelType'];
|
||||
export type PipelineModelField = components['schemas']['PipelineModelField'];
|
||||
export type ModelsList = components['schemas']['ModelsList'];
|
||||
export type ModelType = schemas['ModelType'];
|
||||
export type BaseModelType = schemas['BaseModelType'];
|
||||
export type PipelineModelField = schemas['PipelineModelField'];
|
||||
export type ModelsList = schemas['ModelsList'];
|
||||
|
||||
// Graphs
|
||||
export type Graph = components['schemas']['Graph'];
|
||||
export type Edge = components['schemas']['Edge'];
|
||||
export type GraphExecutionState = components['schemas']['GraphExecutionState'];
|
||||
export type Graph = schemas['Graph'];
|
||||
export type Edge = schemas['Edge'];
|
||||
export type GraphExecutionState = schemas['GraphExecutionState'];
|
||||
|
||||
// General nodes
|
||||
export type CollectInvocation = components['schemas']['CollectInvocation'];
|
||||
export type IterateInvocation = components['schemas']['IterateInvocation'];
|
||||
export type RangeInvocation = components['schemas']['RangeInvocation'];
|
||||
export type RandomRangeInvocation =
|
||||
components['schemas']['RandomRangeInvocation'];
|
||||
export type RangeOfSizeInvocation =
|
||||
components['schemas']['RangeOfSizeInvocation'];
|
||||
export type InpaintInvocation = components['schemas']['InpaintInvocation'];
|
||||
export type ImageResizeInvocation =
|
||||
components['schemas']['ImageResizeInvocation'];
|
||||
export type RandomIntInvocation = components['schemas']['RandomIntInvocation'];
|
||||
export type CompelInvocation = components['schemas']['CompelInvocation'];
|
||||
export type CollectInvocation = schemas['CollectInvocation'];
|
||||
export type IterateInvocation = schemas['IterateInvocation'];
|
||||
export type RangeInvocation = schemas['RangeInvocation'];
|
||||
export type RandomRangeInvocation = schemas['RandomRangeInvocation'];
|
||||
export type RangeOfSizeInvocation = schemas['RangeOfSizeInvocation'];
|
||||
export type InpaintInvocation = schemas['InpaintInvocation'];
|
||||
export type ImageResizeInvocation = schemas['ImageResizeInvocation'];
|
||||
export type RandomIntInvocation = schemas['RandomIntInvocation'];
|
||||
export type CompelInvocation = schemas['CompelInvocation'];
|
||||
|
||||
// ControlNet Nodes
|
||||
export type ControlNetInvocation = schemas['ControlNetInvocation'];
|
||||
export type CannyImageProcessorInvocation =
|
||||
components['schemas']['CannyImageProcessorInvocation'];
|
||||
schemas['CannyImageProcessorInvocation'];
|
||||
export type ContentShuffleImageProcessorInvocation =
|
||||
components['schemas']['ContentShuffleImageProcessorInvocation'];
|
||||
schemas['ContentShuffleImageProcessorInvocation'];
|
||||
export type HedImageProcessorInvocation =
|
||||
components['schemas']['HedImageProcessorInvocation'];
|
||||
schemas['HedImageProcessorInvocation'];
|
||||
export type LineartAnimeImageProcessorInvocation =
|
||||
components['schemas']['LineartAnimeImageProcessorInvocation'];
|
||||
schemas['LineartAnimeImageProcessorInvocation'];
|
||||
export type LineartImageProcessorInvocation =
|
||||
components['schemas']['LineartImageProcessorInvocation'];
|
||||
schemas['LineartImageProcessorInvocation'];
|
||||
export type MediapipeFaceProcessorInvocation =
|
||||
components['schemas']['MediapipeFaceProcessorInvocation'];
|
||||
schemas['MediapipeFaceProcessorInvocation'];
|
||||
export type MidasDepthImageProcessorInvocation =
|
||||
components['schemas']['MidasDepthImageProcessorInvocation'];
|
||||
schemas['MidasDepthImageProcessorInvocation'];
|
||||
export type MlsdImageProcessorInvocation =
|
||||
components['schemas']['MlsdImageProcessorInvocation'];
|
||||
schemas['MlsdImageProcessorInvocation'];
|
||||
export type NormalbaeImageProcessorInvocation =
|
||||
components['schemas']['NormalbaeImageProcessorInvocation'];
|
||||
schemas['NormalbaeImageProcessorInvocation'];
|
||||
export type OpenposeImageProcessorInvocation =
|
||||
components['schemas']['OpenposeImageProcessorInvocation'];
|
||||
schemas['OpenposeImageProcessorInvocation'];
|
||||
export type PidiImageProcessorInvocation =
|
||||
components['schemas']['PidiImageProcessorInvocation'];
|
||||
schemas['PidiImageProcessorInvocation'];
|
||||
export type ZoeDepthImageProcessorInvocation =
|
||||
components['schemas']['ZoeDepthImageProcessorInvocation'];
|
||||
schemas['ZoeDepthImageProcessorInvocation'];
|
||||
|
||||
// Node Outputs
|
||||
export type ImageOutput = components['schemas']['ImageOutput'];
|
||||
export type MaskOutput = components['schemas']['MaskOutput'];
|
||||
export type PromptOutput = components['schemas']['PromptOutput'];
|
||||
export type IterateInvocationOutput =
|
||||
components['schemas']['IterateInvocationOutput'];
|
||||
export type CollectInvocationOutput =
|
||||
components['schemas']['CollectInvocationOutput'];
|
||||
export type LatentsOutput = components['schemas']['LatentsOutput'];
|
||||
export type GraphInvocationOutput =
|
||||
components['schemas']['GraphInvocationOutput'];
|
||||
export type ImageOutput = schemas['ImageOutput'];
|
||||
export type MaskOutput = schemas['MaskOutput'];
|
||||
export type PromptOutput = schemas['PromptOutput'];
|
||||
export type IterateInvocationOutput = schemas['IterateInvocationOutput'];
|
||||
export type CollectInvocationOutput = schemas['CollectInvocationOutput'];
|
||||
export type LatentsOutput = schemas['LatentsOutput'];
|
||||
export type GraphInvocationOutput = schemas['GraphInvocationOutput'];
|
||||
|
Loading…
Reference in New Issue
Block a user