mirror of
https://github.com/invoke-ai/InvokeAI
synced 2024-08-30 20:32:17 +00:00
Replace SlicedAttnProcessor with patched to chunk memory on mps (#3868)
## What type of PR is this? (check all applicable) - [ ] Refactor - [ ] Feature - [x] Bug Fix - [ ] Optimization - [ ] Documentation Update - [ ] Community Node Submission ## Description On mps generating images with resolution above ~1536x1536 results in "fried" output. Main problem that such resolution results in tensors in size more then 4gb. Looks like that some of mps internals can't handle properly this, so to mitigate it I break attention calculation in chunks. ## QA Instructions, Screenshots, Recordings Example of bad output: 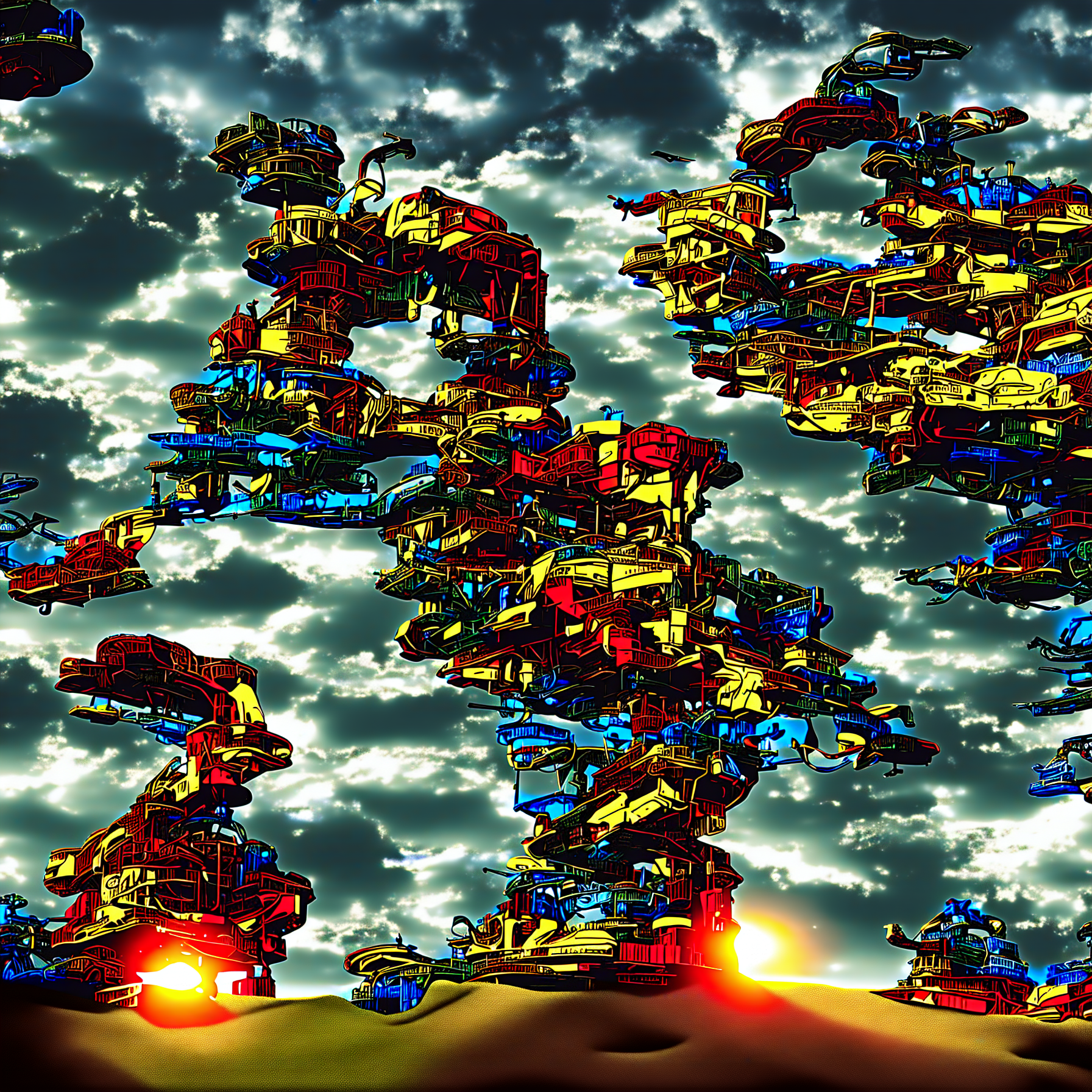
This commit is contained in:
commit
e06f2229ac
@ -1,4 +1,6 @@
|
|||||||
|
import math
|
||||||
import torch
|
import torch
|
||||||
|
import diffusers
|
||||||
|
|
||||||
|
|
||||||
if torch.backends.mps.is_available():
|
if torch.backends.mps.is_available():
|
||||||
@ -61,3 +63,150 @@ def new_torch_interpolate(input, size=None, scale_factor=None, mode='nearest', a
|
|||||||
return _torch_interpolate(input, size, scale_factor, mode, align_corners, recompute_scale_factor, antialias)
|
return _torch_interpolate(input, size, scale_factor, mode, align_corners, recompute_scale_factor, antialias)
|
||||||
|
|
||||||
torch.nn.functional.interpolate = new_torch_interpolate
|
torch.nn.functional.interpolate = new_torch_interpolate
|
||||||
|
|
||||||
|
# TODO: refactor it
|
||||||
|
_SlicedAttnProcessor = diffusers.models.attention_processor.SlicedAttnProcessor
|
||||||
|
class ChunkedSlicedAttnProcessor:
|
||||||
|
r"""
|
||||||
|
Processor for implementing sliced attention.
|
||||||
|
|
||||||
|
Args:
|
||||||
|
slice_size (`int`, *optional*):
|
||||||
|
The number of steps to compute attention. Uses as many slices as `attention_head_dim // slice_size`, and
|
||||||
|
`attention_head_dim` must be a multiple of the `slice_size`.
|
||||||
|
"""
|
||||||
|
|
||||||
|
def __init__(self, slice_size):
|
||||||
|
assert isinstance(slice_size, int)
|
||||||
|
slice_size = 1 # TODO: maybe implement chunking in batches too when enough memory
|
||||||
|
self.slice_size = slice_size
|
||||||
|
self._sliced_attn_processor = _SlicedAttnProcessor(slice_size)
|
||||||
|
|
||||||
|
def __call__(self, attn, hidden_states, encoder_hidden_states=None, attention_mask=None):
|
||||||
|
if self.slice_size != 1:
|
||||||
|
return self._sliced_attn_processor(attn, hidden_states, encoder_hidden_states, attention_mask)
|
||||||
|
|
||||||
|
residual = hidden_states
|
||||||
|
|
||||||
|
input_ndim = hidden_states.ndim
|
||||||
|
|
||||||
|
if input_ndim == 4:
|
||||||
|
batch_size, channel, height, width = hidden_states.shape
|
||||||
|
hidden_states = hidden_states.view(batch_size, channel, height * width).transpose(1, 2)
|
||||||
|
|
||||||
|
batch_size, sequence_length, _ = (
|
||||||
|
hidden_states.shape if encoder_hidden_states is None else encoder_hidden_states.shape
|
||||||
|
)
|
||||||
|
attention_mask = attn.prepare_attention_mask(attention_mask, sequence_length, batch_size)
|
||||||
|
|
||||||
|
if attn.group_norm is not None:
|
||||||
|
hidden_states = attn.group_norm(hidden_states.transpose(1, 2)).transpose(1, 2)
|
||||||
|
|
||||||
|
query = attn.to_q(hidden_states)
|
||||||
|
dim = query.shape[-1]
|
||||||
|
query = attn.head_to_batch_dim(query)
|
||||||
|
|
||||||
|
if encoder_hidden_states is None:
|
||||||
|
encoder_hidden_states = hidden_states
|
||||||
|
elif attn.norm_cross:
|
||||||
|
encoder_hidden_states = attn.norm_encoder_hidden_states(encoder_hidden_states)
|
||||||
|
|
||||||
|
key = attn.to_k(encoder_hidden_states)
|
||||||
|
value = attn.to_v(encoder_hidden_states)
|
||||||
|
key = attn.head_to_batch_dim(key)
|
||||||
|
value = attn.head_to_batch_dim(value)
|
||||||
|
|
||||||
|
batch_size_attention, query_tokens, _ = query.shape
|
||||||
|
hidden_states = torch.zeros(
|
||||||
|
(batch_size_attention, query_tokens, dim // attn.heads), device=query.device, dtype=query.dtype
|
||||||
|
)
|
||||||
|
|
||||||
|
chunk_tmp_tensor = torch.empty(self.slice_size, query.shape[1], key.shape[1], dtype=query.dtype, device=query.device)
|
||||||
|
|
||||||
|
for i in range(batch_size_attention // self.slice_size):
|
||||||
|
start_idx = i * self.slice_size
|
||||||
|
end_idx = (i + 1) * self.slice_size
|
||||||
|
|
||||||
|
query_slice = query[start_idx:end_idx]
|
||||||
|
key_slice = key[start_idx:end_idx]
|
||||||
|
attn_mask_slice = attention_mask[start_idx:end_idx] if attention_mask is not None else None
|
||||||
|
|
||||||
|
self.get_attention_scores_chunked(attn, query_slice, key_slice, attn_mask_slice, hidden_states[start_idx:end_idx], value[start_idx:end_idx], chunk_tmp_tensor)
|
||||||
|
|
||||||
|
hidden_states = attn.batch_to_head_dim(hidden_states)
|
||||||
|
|
||||||
|
# linear proj
|
||||||
|
hidden_states = attn.to_out[0](hidden_states)
|
||||||
|
# dropout
|
||||||
|
hidden_states = attn.to_out[1](hidden_states)
|
||||||
|
|
||||||
|
if input_ndim == 4:
|
||||||
|
hidden_states = hidden_states.transpose(-1, -2).reshape(batch_size, channel, height, width)
|
||||||
|
|
||||||
|
if attn.residual_connection:
|
||||||
|
hidden_states = hidden_states + residual
|
||||||
|
|
||||||
|
hidden_states = hidden_states / attn.rescale_output_factor
|
||||||
|
|
||||||
|
return hidden_states
|
||||||
|
|
||||||
|
|
||||||
|
def get_attention_scores_chunked(self, attn, query, key, attention_mask, hidden_states, value, chunk):
|
||||||
|
# batch size = 1
|
||||||
|
assert query.shape[0] == 1
|
||||||
|
assert key.shape[0] == 1
|
||||||
|
assert value.shape[0] == 1
|
||||||
|
assert hidden_states.shape[0] == 1
|
||||||
|
|
||||||
|
dtype = query.dtype
|
||||||
|
if attn.upcast_attention:
|
||||||
|
query = query.float()
|
||||||
|
key = key.float()
|
||||||
|
|
||||||
|
#out_item_size = query.dtype.itemsize
|
||||||
|
#if attn.upcast_attention:
|
||||||
|
# out_item_size = torch.float32.itemsize
|
||||||
|
out_item_size = query.element_size()
|
||||||
|
if attn.upcast_attention:
|
||||||
|
out_item_size = 4
|
||||||
|
|
||||||
|
chunk_size = 2 ** 29
|
||||||
|
|
||||||
|
out_size = query.shape[1] * key.shape[1] * out_item_size
|
||||||
|
chunks_count = min(query.shape[1], math.ceil((out_size - 1) / chunk_size))
|
||||||
|
chunk_step = max(1, int(query.shape[1] / chunks_count))
|
||||||
|
|
||||||
|
key = key.transpose(-1, -2)
|
||||||
|
|
||||||
|
def _get_chunk_view(tensor, start, length):
|
||||||
|
if start + length > tensor.shape[1]:
|
||||||
|
length = tensor.shape[1] - start
|
||||||
|
#print(f"view: [{tensor.shape[0]},{tensor.shape[1]},{tensor.shape[2]}] - start: {start}, length: {length}")
|
||||||
|
return tensor[:,start:start+length]
|
||||||
|
|
||||||
|
for chunk_pos in range(0, query.shape[1], chunk_step):
|
||||||
|
if attention_mask is not None:
|
||||||
|
torch.baddbmm(
|
||||||
|
_get_chunk_view(attention_mask, chunk_pos, chunk_step),
|
||||||
|
_get_chunk_view(query, chunk_pos, chunk_step),
|
||||||
|
key,
|
||||||
|
beta=1,
|
||||||
|
alpha=attn.scale,
|
||||||
|
out=chunk,
|
||||||
|
)
|
||||||
|
else:
|
||||||
|
torch.baddbmm(
|
||||||
|
torch.zeros((1,1,1), device=query.device, dtype=query.dtype),
|
||||||
|
_get_chunk_view(query, chunk_pos, chunk_step),
|
||||||
|
key,
|
||||||
|
beta=0,
|
||||||
|
alpha=attn.scale,
|
||||||
|
out=chunk,
|
||||||
|
)
|
||||||
|
chunk = chunk.softmax(dim=-1)
|
||||||
|
torch.bmm(chunk, value, out=_get_chunk_view(hidden_states, chunk_pos, chunk_step))
|
||||||
|
|
||||||
|
#del chunk
|
||||||
|
|
||||||
|
|
||||||
|
diffusers.models.attention_processor.SlicedAttnProcessor = ChunkedSlicedAttnProcessor
|
||||||
|
Loading…
Reference in New Issue
Block a user