mirror of
https://github.com/invoke-ai/InvokeAI
synced 2024-08-30 20:32:17 +00:00
Add Grounded SAM support (text prompt image segmentation) (#6701)
## Summary This PR enables Grounded SAM workflows (https://arxiv.org/pdf/2401.14159) via the following: - `GroundingDinoInvocation` for running a Grounding DINO model. - `SegmentAnythingModelInvocation` for running a SAM model. - `MaskTensorToImageInvocation` for convenient visualization. Other notes: - Uses the transformers implementation of Grounding DINO and SAM. - The new models are treated as 'utility models' meaning that they are not visible in the Models tab, and are downloaded automatically the first time that they are used. <img width="874" alt="image" src="https://github.com/user-attachments/assets/1cbaa97d-0e27-4943-86b1-dc7327ba8675"> ## Example Input image  Prompt: "wheels", all other configs default Result: 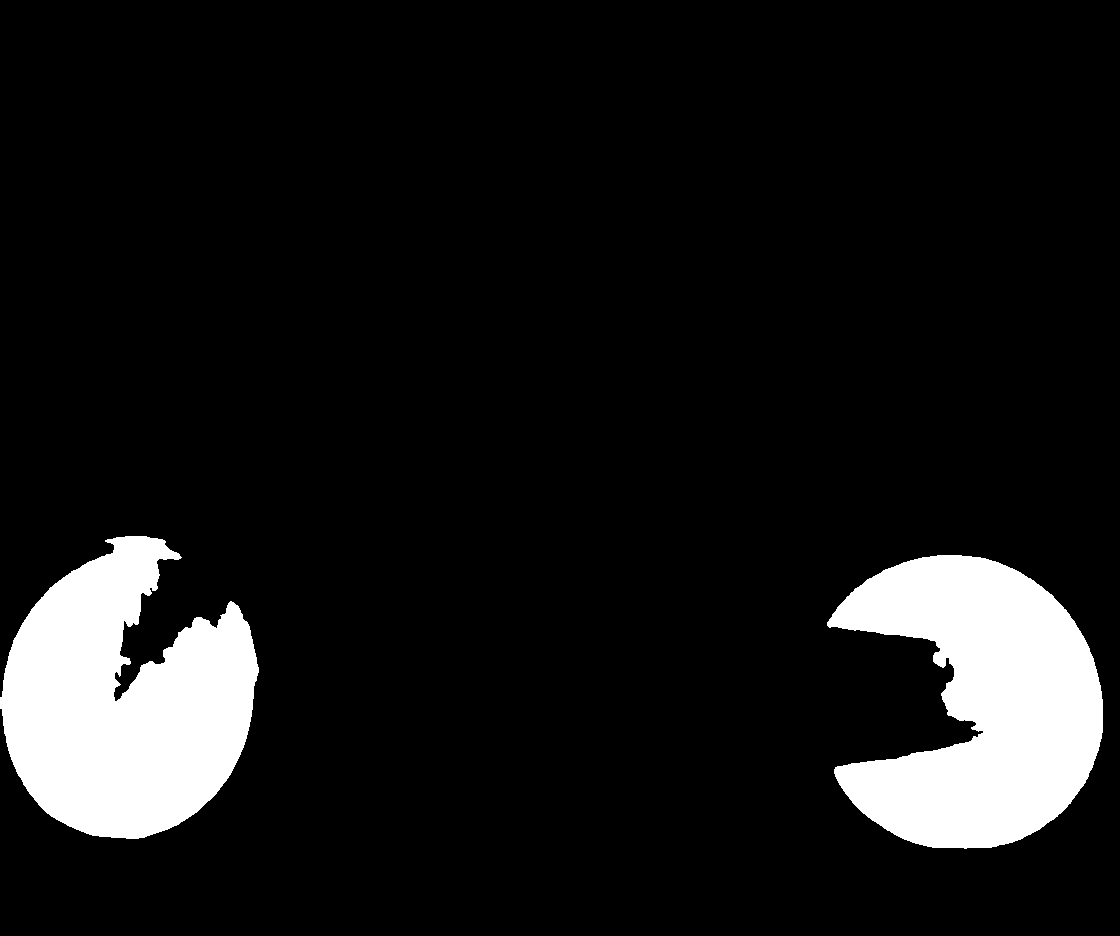 ## Related Issues / Discussions Thanks to @blessedcoolant for the initial draft here: https://github.com/invoke-ai/InvokeAI/pull/6678 ## QA Instructions Manual tests: - [ ] Test that default settings work well. - [ ] Test with / without apply_polygon_refinement - [ ] Test mask_filter options - [ ] Test detection_threshold values - [ ] Test RGB input image - [ ] Test RGBA input image - [ ] Test grayscale input image - [ ] Smoke test that an empty mask is returned when 0 objects are detected - [ ] Test on CPU - [ ] Test on MPS (Works on Mac OS, but had to force both models to run on CPU instead of MPS) Performance: - Peak GPU memory utilization with both Grounding DINO and SAM models loaded is ~4.5GB. (The models do not need to be loaded at the same time, so could be offloaded by the MM if needed.) - On an RTX4090, with the models already cached, node execution takes ~0.6 secs. - On my CPU, with the models cached, node execution takes ~10secs. ## Merge Plan No special instructions. ## Checklist - [x] _The PR has a short but descriptive title, suitable for a changelog_ - [ ] _Tests added / updated (if applicable)_ - [x] _Documentation added / updated (if applicable)_
This commit is contained in:
@ -1,7 +1,7 @@
|
|||||||
from enum import Enum
|
from enum import Enum
|
||||||
from typing import Any, Callable, Optional, Tuple
|
from typing import Any, Callable, Optional, Tuple
|
||||||
|
|
||||||
from pydantic import BaseModel, ConfigDict, Field, RootModel, TypeAdapter
|
from pydantic import BaseModel, ConfigDict, Field, RootModel, TypeAdapter, model_validator
|
||||||
from pydantic.fields import _Unset
|
from pydantic.fields import _Unset
|
||||||
from pydantic_core import PydanticUndefined
|
from pydantic_core import PydanticUndefined
|
||||||
|
|
||||||
@ -242,6 +242,31 @@ class ConditioningField(BaseModel):
|
|||||||
)
|
)
|
||||||
|
|
||||||
|
|
||||||
|
class BoundingBoxField(BaseModel):
|
||||||
|
"""A bounding box primitive value."""
|
||||||
|
|
||||||
|
x_min: int = Field(ge=0, description="The minimum x-coordinate of the bounding box (inclusive).")
|
||||||
|
x_max: int = Field(ge=0, description="The maximum x-coordinate of the bounding box (exclusive).")
|
||||||
|
y_min: int = Field(ge=0, description="The minimum y-coordinate of the bounding box (inclusive).")
|
||||||
|
y_max: int = Field(ge=0, description="The maximum y-coordinate of the bounding box (exclusive).")
|
||||||
|
|
||||||
|
score: Optional[float] = Field(
|
||||||
|
default=None,
|
||||||
|
ge=0.0,
|
||||||
|
le=1.0,
|
||||||
|
description="The score associated with the bounding box. In the range [0, 1]. This value is typically set "
|
||||||
|
"when the bounding box was produced by a detector and has an associated confidence score.",
|
||||||
|
)
|
||||||
|
|
||||||
|
@model_validator(mode="after")
|
||||||
|
def check_coords(self):
|
||||||
|
if self.x_min > self.x_max:
|
||||||
|
raise ValueError(f"x_min ({self.x_min}) is greater than x_max ({self.x_max}).")
|
||||||
|
if self.y_min > self.y_max:
|
||||||
|
raise ValueError(f"y_min ({self.y_min}) is greater than y_max ({self.y_max}).")
|
||||||
|
return self
|
||||||
|
|
||||||
|
|
||||||
class MetadataField(RootModel[dict[str, Any]]):
|
class MetadataField(RootModel[dict[str, Any]]):
|
||||||
"""
|
"""
|
||||||
Pydantic model for metadata with custom root of type dict[str, Any].
|
Pydantic model for metadata with custom root of type dict[str, Any].
|
||||||
|
100
invokeai/app/invocations/grounding_dino.py
Normal file
100
invokeai/app/invocations/grounding_dino.py
Normal file
@ -0,0 +1,100 @@
|
|||||||
|
from pathlib import Path
|
||||||
|
from typing import Literal
|
||||||
|
|
||||||
|
import torch
|
||||||
|
from PIL import Image
|
||||||
|
from transformers import pipeline
|
||||||
|
from transformers.pipelines import ZeroShotObjectDetectionPipeline
|
||||||
|
|
||||||
|
from invokeai.app.invocations.baseinvocation import BaseInvocation, invocation
|
||||||
|
from invokeai.app.invocations.fields import BoundingBoxField, ImageField, InputField
|
||||||
|
from invokeai.app.invocations.primitives import BoundingBoxCollectionOutput
|
||||||
|
from invokeai.app.services.shared.invocation_context import InvocationContext
|
||||||
|
from invokeai.backend.image_util.grounding_dino.detection_result import DetectionResult
|
||||||
|
from invokeai.backend.image_util.grounding_dino.grounding_dino_pipeline import GroundingDinoPipeline
|
||||||
|
|
||||||
|
GroundingDinoModelKey = Literal["grounding-dino-tiny", "grounding-dino-base"]
|
||||||
|
GROUNDING_DINO_MODEL_IDS: dict[GroundingDinoModelKey, str] = {
|
||||||
|
"grounding-dino-tiny": "IDEA-Research/grounding-dino-tiny",
|
||||||
|
"grounding-dino-base": "IDEA-Research/grounding-dino-base",
|
||||||
|
}
|
||||||
|
|
||||||
|
|
||||||
|
@invocation(
|
||||||
|
"grounding_dino",
|
||||||
|
title="Grounding DINO (Text Prompt Object Detection)",
|
||||||
|
tags=["prompt", "object detection"],
|
||||||
|
category="image",
|
||||||
|
version="1.0.0",
|
||||||
|
)
|
||||||
|
class GroundingDinoInvocation(BaseInvocation):
|
||||||
|
"""Runs a Grounding DINO model. Performs zero-shot bounding-box object detection from a text prompt."""
|
||||||
|
|
||||||
|
# Reference:
|
||||||
|
# - https://arxiv.org/pdf/2303.05499
|
||||||
|
# - https://huggingface.co/docs/transformers/v4.43.3/en/model_doc/grounding-dino#grounded-sam
|
||||||
|
# - https://github.com/NielsRogge/Transformers-Tutorials/blob/a39f33ac1557b02ebfb191ea7753e332b5ca933f/Grounding%20DINO/GroundingDINO_with_Segment_Anything.ipynb
|
||||||
|
|
||||||
|
model: GroundingDinoModelKey = InputField(description="The Grounding DINO model to use.")
|
||||||
|
prompt: str = InputField(description="The prompt describing the object to segment.")
|
||||||
|
image: ImageField = InputField(description="The image to segment.")
|
||||||
|
detection_threshold: float = InputField(
|
||||||
|
description="The detection threshold for the Grounding DINO model. All detected bounding boxes with scores above this threshold will be returned.",
|
||||||
|
ge=0.0,
|
||||||
|
le=1.0,
|
||||||
|
default=0.3,
|
||||||
|
)
|
||||||
|
|
||||||
|
@torch.no_grad()
|
||||||
|
def invoke(self, context: InvocationContext) -> BoundingBoxCollectionOutput:
|
||||||
|
# The model expects a 3-channel RGB image.
|
||||||
|
image_pil = context.images.get_pil(self.image.image_name, mode="RGB")
|
||||||
|
|
||||||
|
detections = self._detect(
|
||||||
|
context=context, image=image_pil, labels=[self.prompt], threshold=self.detection_threshold
|
||||||
|
)
|
||||||
|
|
||||||
|
# Convert detections to BoundingBoxCollectionOutput.
|
||||||
|
bounding_boxes: list[BoundingBoxField] = []
|
||||||
|
for detection in detections:
|
||||||
|
bounding_boxes.append(
|
||||||
|
BoundingBoxField(
|
||||||
|
x_min=detection.box.xmin,
|
||||||
|
x_max=detection.box.xmax,
|
||||||
|
y_min=detection.box.ymin,
|
||||||
|
y_max=detection.box.ymax,
|
||||||
|
score=detection.score,
|
||||||
|
)
|
||||||
|
)
|
||||||
|
return BoundingBoxCollectionOutput(collection=bounding_boxes)
|
||||||
|
|
||||||
|
@staticmethod
|
||||||
|
def _load_grounding_dino(model_path: Path):
|
||||||
|
grounding_dino_pipeline = pipeline(
|
||||||
|
model=str(model_path),
|
||||||
|
task="zero-shot-object-detection",
|
||||||
|
local_files_only=True,
|
||||||
|
# TODO(ryand): Setting the torch_dtype here doesn't work. Investigate whether fp16 is supported by the
|
||||||
|
# model, and figure out how to make it work in the pipeline.
|
||||||
|
# torch_dtype=TorchDevice.choose_torch_dtype(),
|
||||||
|
)
|
||||||
|
assert isinstance(grounding_dino_pipeline, ZeroShotObjectDetectionPipeline)
|
||||||
|
return GroundingDinoPipeline(grounding_dino_pipeline)
|
||||||
|
|
||||||
|
def _detect(
|
||||||
|
self,
|
||||||
|
context: InvocationContext,
|
||||||
|
image: Image.Image,
|
||||||
|
labels: list[str],
|
||||||
|
threshold: float = 0.3,
|
||||||
|
) -> list[DetectionResult]:
|
||||||
|
"""Use Grounding DINO to detect bounding boxes for a set of labels in an image."""
|
||||||
|
# TODO(ryand): I copied this "."-handling logic from the transformers example code. Test it and see if it
|
||||||
|
# actually makes a difference.
|
||||||
|
labels = [label if label.endswith(".") else label + "." for label in labels]
|
||||||
|
|
||||||
|
with context.models.load_remote_model(
|
||||||
|
source=GROUNDING_DINO_MODEL_IDS[self.model], loader=GroundingDinoInvocation._load_grounding_dino
|
||||||
|
) as detector:
|
||||||
|
assert isinstance(detector, GroundingDinoPipeline)
|
||||||
|
return detector.detect(image=image, candidate_labels=labels, threshold=threshold)
|
@ -1,9 +1,10 @@
|
|||||||
import numpy as np
|
import numpy as np
|
||||||
import torch
|
import torch
|
||||||
|
from PIL import Image
|
||||||
|
|
||||||
from invokeai.app.invocations.baseinvocation import BaseInvocation, Classification, InvocationContext, invocation
|
from invokeai.app.invocations.baseinvocation import BaseInvocation, Classification, InvocationContext, invocation
|
||||||
from invokeai.app.invocations.fields import ImageField, InputField, TensorField, WithMetadata
|
from invokeai.app.invocations.fields import ImageField, InputField, TensorField, WithBoard, WithMetadata
|
||||||
from invokeai.app.invocations.primitives import MaskOutput
|
from invokeai.app.invocations.primitives import ImageOutput, MaskOutput
|
||||||
|
|
||||||
|
|
||||||
@invocation(
|
@invocation(
|
||||||
@ -118,3 +119,27 @@ class ImageMaskToTensorInvocation(BaseInvocation, WithMetadata):
|
|||||||
height=mask.shape[1],
|
height=mask.shape[1],
|
||||||
width=mask.shape[2],
|
width=mask.shape[2],
|
||||||
)
|
)
|
||||||
|
|
||||||
|
|
||||||
|
@invocation(
|
||||||
|
"tensor_mask_to_image",
|
||||||
|
title="Tensor Mask to Image",
|
||||||
|
tags=["mask"],
|
||||||
|
category="mask",
|
||||||
|
version="1.0.0",
|
||||||
|
)
|
||||||
|
class MaskTensorToImageInvocation(BaseInvocation, WithMetadata, WithBoard):
|
||||||
|
"""Convert a mask tensor to an image."""
|
||||||
|
|
||||||
|
mask: TensorField = InputField(description="The mask tensor to convert.")
|
||||||
|
|
||||||
|
def invoke(self, context: InvocationContext) -> ImageOutput:
|
||||||
|
mask = context.tensors.load(self.mask.tensor_name)
|
||||||
|
# Ensure that the mask is binary.
|
||||||
|
if mask.dtype != torch.bool:
|
||||||
|
mask = mask > 0.5
|
||||||
|
mask_np = (mask.float() * 255).byte().cpu().numpy()
|
||||||
|
|
||||||
|
mask_pil = Image.fromarray(mask_np, mode="L")
|
||||||
|
image_dto = context.images.save(image=mask_pil)
|
||||||
|
return ImageOutput.build(image_dto)
|
||||||
|
@ -7,6 +7,7 @@ import torch
|
|||||||
from invokeai.app.invocations.baseinvocation import BaseInvocation, BaseInvocationOutput, invocation, invocation_output
|
from invokeai.app.invocations.baseinvocation import BaseInvocation, BaseInvocationOutput, invocation, invocation_output
|
||||||
from invokeai.app.invocations.constants import LATENT_SCALE_FACTOR
|
from invokeai.app.invocations.constants import LATENT_SCALE_FACTOR
|
||||||
from invokeai.app.invocations.fields import (
|
from invokeai.app.invocations.fields import (
|
||||||
|
BoundingBoxField,
|
||||||
ColorField,
|
ColorField,
|
||||||
ConditioningField,
|
ConditioningField,
|
||||||
DenoiseMaskField,
|
DenoiseMaskField,
|
||||||
@ -469,3 +470,42 @@ class ConditioningCollectionInvocation(BaseInvocation):
|
|||||||
|
|
||||||
|
|
||||||
# endregion
|
# endregion
|
||||||
|
|
||||||
|
# region BoundingBox
|
||||||
|
|
||||||
|
|
||||||
|
@invocation_output("bounding_box_output")
|
||||||
|
class BoundingBoxOutput(BaseInvocationOutput):
|
||||||
|
"""Base class for nodes that output a single bounding box"""
|
||||||
|
|
||||||
|
bounding_box: BoundingBoxField = OutputField(description="The output bounding box.")
|
||||||
|
|
||||||
|
|
||||||
|
@invocation_output("bounding_box_collection_output")
|
||||||
|
class BoundingBoxCollectionOutput(BaseInvocationOutput):
|
||||||
|
"""Base class for nodes that output a collection of bounding boxes"""
|
||||||
|
|
||||||
|
collection: list[BoundingBoxField] = OutputField(description="The output bounding boxes.", title="Bounding Boxes")
|
||||||
|
|
||||||
|
|
||||||
|
@invocation(
|
||||||
|
"bounding_box",
|
||||||
|
title="Bounding Box",
|
||||||
|
tags=["primitives", "segmentation", "collection", "bounding box"],
|
||||||
|
category="primitives",
|
||||||
|
version="1.0.0",
|
||||||
|
)
|
||||||
|
class BoundingBoxInvocation(BaseInvocation):
|
||||||
|
"""Create a bounding box manually by supplying box coordinates"""
|
||||||
|
|
||||||
|
x_min: int = InputField(default=0, description="x-coordinate of the bounding box's top left vertex")
|
||||||
|
y_min: int = InputField(default=0, description="y-coordinate of the bounding box's top left vertex")
|
||||||
|
x_max: int = InputField(default=0, description="x-coordinate of the bounding box's bottom right vertex")
|
||||||
|
y_max: int = InputField(default=0, description="y-coordinate of the bounding box's bottom right vertex")
|
||||||
|
|
||||||
|
def invoke(self, context: InvocationContext) -> BoundingBoxOutput:
|
||||||
|
bounding_box = BoundingBoxField(x_min=self.x_min, y_min=self.y_min, x_max=self.x_max, y_max=self.y_max)
|
||||||
|
return BoundingBoxOutput(bounding_box=bounding_box)
|
||||||
|
|
||||||
|
|
||||||
|
# endregion
|
||||||
|
161
invokeai/app/invocations/segment_anything.py
Normal file
161
invokeai/app/invocations/segment_anything.py
Normal file
@ -0,0 +1,161 @@
|
|||||||
|
from pathlib import Path
|
||||||
|
from typing import Literal
|
||||||
|
|
||||||
|
import numpy as np
|
||||||
|
import torch
|
||||||
|
from PIL import Image
|
||||||
|
from transformers import AutoModelForMaskGeneration, AutoProcessor
|
||||||
|
from transformers.models.sam import SamModel
|
||||||
|
from transformers.models.sam.processing_sam import SamProcessor
|
||||||
|
|
||||||
|
from invokeai.app.invocations.baseinvocation import BaseInvocation, invocation
|
||||||
|
from invokeai.app.invocations.fields import BoundingBoxField, ImageField, InputField, TensorField
|
||||||
|
from invokeai.app.invocations.primitives import MaskOutput
|
||||||
|
from invokeai.app.services.shared.invocation_context import InvocationContext
|
||||||
|
from invokeai.backend.image_util.segment_anything.mask_refinement import mask_to_polygon, polygon_to_mask
|
||||||
|
from invokeai.backend.image_util.segment_anything.segment_anything_pipeline import SegmentAnythingPipeline
|
||||||
|
|
||||||
|
SegmentAnythingModelKey = Literal["segment-anything-base", "segment-anything-large", "segment-anything-huge"]
|
||||||
|
SEGMENT_ANYTHING_MODEL_IDS: dict[SegmentAnythingModelKey, str] = {
|
||||||
|
"segment-anything-base": "facebook/sam-vit-base",
|
||||||
|
"segment-anything-large": "facebook/sam-vit-large",
|
||||||
|
"segment-anything-huge": "facebook/sam-vit-huge",
|
||||||
|
}
|
||||||
|
|
||||||
|
|
||||||
|
@invocation(
|
||||||
|
"segment_anything",
|
||||||
|
title="Segment Anything",
|
||||||
|
tags=["prompt", "segmentation"],
|
||||||
|
category="segmentation",
|
||||||
|
version="1.0.0",
|
||||||
|
)
|
||||||
|
class SegmentAnythingInvocation(BaseInvocation):
|
||||||
|
"""Runs a Segment Anything Model."""
|
||||||
|
|
||||||
|
# Reference:
|
||||||
|
# - https://arxiv.org/pdf/2304.02643
|
||||||
|
# - https://huggingface.co/docs/transformers/v4.43.3/en/model_doc/grounding-dino#grounded-sam
|
||||||
|
# - https://github.com/NielsRogge/Transformers-Tutorials/blob/a39f33ac1557b02ebfb191ea7753e332b5ca933f/Grounding%20DINO/GroundingDINO_with_Segment_Anything.ipynb
|
||||||
|
|
||||||
|
model: SegmentAnythingModelKey = InputField(description="The Segment Anything model to use.")
|
||||||
|
image: ImageField = InputField(description="The image to segment.")
|
||||||
|
bounding_boxes: list[BoundingBoxField] = InputField(description="The bounding boxes to prompt the SAM model with.")
|
||||||
|
apply_polygon_refinement: bool = InputField(
|
||||||
|
description="Whether to apply polygon refinement to the masks. This will smooth the edges of the masks slightly and ensure that each mask consists of a single closed polygon (before merging).",
|
||||||
|
default=True,
|
||||||
|
)
|
||||||
|
mask_filter: Literal["all", "largest", "highest_box_score"] = InputField(
|
||||||
|
description="The filtering to apply to the detected masks before merging them into a final output.",
|
||||||
|
default="all",
|
||||||
|
)
|
||||||
|
|
||||||
|
@torch.no_grad()
|
||||||
|
def invoke(self, context: InvocationContext) -> MaskOutput:
|
||||||
|
# The models expect a 3-channel RGB image.
|
||||||
|
image_pil = context.images.get_pil(self.image.image_name, mode="RGB")
|
||||||
|
|
||||||
|
if len(self.bounding_boxes) == 0:
|
||||||
|
combined_mask = torch.zeros(image_pil.size[::-1], dtype=torch.bool)
|
||||||
|
else:
|
||||||
|
masks = self._segment(context=context, image=image_pil)
|
||||||
|
masks = self._filter_masks(masks=masks, bounding_boxes=self.bounding_boxes)
|
||||||
|
|
||||||
|
# masks contains bool values, so we merge them via max-reduce.
|
||||||
|
combined_mask, _ = torch.stack(masks).max(dim=0)
|
||||||
|
|
||||||
|
mask_tensor_name = context.tensors.save(combined_mask)
|
||||||
|
height, width = combined_mask.shape
|
||||||
|
return MaskOutput(mask=TensorField(tensor_name=mask_tensor_name), width=width, height=height)
|
||||||
|
|
||||||
|
@staticmethod
|
||||||
|
def _load_sam_model(model_path: Path):
|
||||||
|
sam_model = AutoModelForMaskGeneration.from_pretrained(
|
||||||
|
model_path,
|
||||||
|
local_files_only=True,
|
||||||
|
# TODO(ryand): Setting the torch_dtype here doesn't work. Investigate whether fp16 is supported by the
|
||||||
|
# model, and figure out how to make it work in the pipeline.
|
||||||
|
# torch_dtype=TorchDevice.choose_torch_dtype(),
|
||||||
|
)
|
||||||
|
assert isinstance(sam_model, SamModel)
|
||||||
|
|
||||||
|
sam_processor = AutoProcessor.from_pretrained(model_path, local_files_only=True)
|
||||||
|
assert isinstance(sam_processor, SamProcessor)
|
||||||
|
return SegmentAnythingPipeline(sam_model=sam_model, sam_processor=sam_processor)
|
||||||
|
|
||||||
|
def _segment(
|
||||||
|
self,
|
||||||
|
context: InvocationContext,
|
||||||
|
image: Image.Image,
|
||||||
|
) -> list[torch.Tensor]:
|
||||||
|
"""Use Segment Anything (SAM) to generate masks given an image + a set of bounding boxes."""
|
||||||
|
# Convert the bounding boxes to the SAM input format.
|
||||||
|
sam_bounding_boxes = [[bb.x_min, bb.y_min, bb.x_max, bb.y_max] for bb in self.bounding_boxes]
|
||||||
|
|
||||||
|
with (
|
||||||
|
context.models.load_remote_model(
|
||||||
|
source=SEGMENT_ANYTHING_MODEL_IDS[self.model], loader=SegmentAnythingInvocation._load_sam_model
|
||||||
|
) as sam_pipeline,
|
||||||
|
):
|
||||||
|
assert isinstance(sam_pipeline, SegmentAnythingPipeline)
|
||||||
|
masks = sam_pipeline.segment(image=image, bounding_boxes=sam_bounding_boxes)
|
||||||
|
|
||||||
|
masks = self._process_masks(masks)
|
||||||
|
if self.apply_polygon_refinement:
|
||||||
|
masks = self._apply_polygon_refinement(masks)
|
||||||
|
|
||||||
|
return masks
|
||||||
|
|
||||||
|
def _process_masks(self, masks: torch.Tensor) -> list[torch.Tensor]:
|
||||||
|
"""Convert the tensor output from the Segment Anything model from a tensor of shape
|
||||||
|
[num_masks, channels, height, width] to a list of tensors of shape [height, width].
|
||||||
|
"""
|
||||||
|
assert masks.dtype == torch.bool
|
||||||
|
# [num_masks, channels, height, width] -> [num_masks, height, width]
|
||||||
|
masks, _ = masks.max(dim=1)
|
||||||
|
# Split the first dimension into a list of masks.
|
||||||
|
return list(masks.cpu().unbind(dim=0))
|
||||||
|
|
||||||
|
def _apply_polygon_refinement(self, masks: list[torch.Tensor]) -> list[torch.Tensor]:
|
||||||
|
"""Apply polygon refinement to the masks.
|
||||||
|
|
||||||
|
Convert each mask to a polygon, then back to a mask. This has the following effect:
|
||||||
|
- Smooth the edges of the mask slightly.
|
||||||
|
- Ensure that each mask consists of a single closed polygon
|
||||||
|
- Removes small mask pieces.
|
||||||
|
- Removes holes from the mask.
|
||||||
|
"""
|
||||||
|
# Convert tensor masks to np masks.
|
||||||
|
np_masks = [mask.cpu().numpy().astype(np.uint8) for mask in masks]
|
||||||
|
|
||||||
|
# Apply polygon refinement.
|
||||||
|
for idx, mask in enumerate(np_masks):
|
||||||
|
shape = mask.shape
|
||||||
|
assert len(shape) == 2 # Assert length to satisfy type checker.
|
||||||
|
polygon = mask_to_polygon(mask)
|
||||||
|
mask = polygon_to_mask(polygon, shape)
|
||||||
|
np_masks[idx] = mask
|
||||||
|
|
||||||
|
# Convert np masks back to tensor masks.
|
||||||
|
masks = [torch.tensor(mask, dtype=torch.bool) for mask in np_masks]
|
||||||
|
|
||||||
|
return masks
|
||||||
|
|
||||||
|
def _filter_masks(self, masks: list[torch.Tensor], bounding_boxes: list[BoundingBoxField]) -> list[torch.Tensor]:
|
||||||
|
"""Filter the detected masks based on the specified mask filter."""
|
||||||
|
assert len(masks) == len(bounding_boxes)
|
||||||
|
|
||||||
|
if self.mask_filter == "all":
|
||||||
|
return masks
|
||||||
|
elif self.mask_filter == "largest":
|
||||||
|
# Find the largest mask.
|
||||||
|
return [max(masks, key=lambda x: float(x.sum()))]
|
||||||
|
elif self.mask_filter == "highest_box_score":
|
||||||
|
# Find the index of the bounding box with the highest score.
|
||||||
|
# Note that we fallback to -1.0 if the score is None. This is mainly to satisfy the type checker. In most
|
||||||
|
# cases the scores should all be non-None when using this filtering mode. That being said, -1.0 is a
|
||||||
|
# reasonable fallback since the expected score range is [0.0, 1.0].
|
||||||
|
max_score_idx = max(range(len(bounding_boxes)), key=lambda i: bounding_boxes[i].score or -1.0)
|
||||||
|
return [masks[max_score_idx]]
|
||||||
|
else:
|
||||||
|
raise ValueError(f"Invalid mask filter: {self.mask_filter}")
|
@ -0,0 +1,22 @@
|
|||||||
|
from pydantic import BaseModel, ConfigDict
|
||||||
|
|
||||||
|
|
||||||
|
class BoundingBox(BaseModel):
|
||||||
|
"""Bounding box helper class."""
|
||||||
|
|
||||||
|
xmin: int
|
||||||
|
ymin: int
|
||||||
|
xmax: int
|
||||||
|
ymax: int
|
||||||
|
|
||||||
|
|
||||||
|
class DetectionResult(BaseModel):
|
||||||
|
"""Detection result from Grounding DINO."""
|
||||||
|
|
||||||
|
score: float
|
||||||
|
label: str
|
||||||
|
box: BoundingBox
|
||||||
|
model_config = ConfigDict(
|
||||||
|
# Allow arbitrary types for mask, since it will be a numpy array.
|
||||||
|
arbitrary_types_allowed=True
|
||||||
|
)
|
@ -0,0 +1,37 @@
|
|||||||
|
from typing import Optional
|
||||||
|
|
||||||
|
import torch
|
||||||
|
from PIL import Image
|
||||||
|
from transformers.pipelines import ZeroShotObjectDetectionPipeline
|
||||||
|
|
||||||
|
from invokeai.backend.image_util.grounding_dino.detection_result import DetectionResult
|
||||||
|
from invokeai.backend.raw_model import RawModel
|
||||||
|
|
||||||
|
|
||||||
|
class GroundingDinoPipeline(RawModel):
|
||||||
|
"""A wrapper class for a ZeroShotObjectDetectionPipeline that makes it compatible with the model manager's memory
|
||||||
|
management system.
|
||||||
|
"""
|
||||||
|
|
||||||
|
def __init__(self, pipeline: ZeroShotObjectDetectionPipeline):
|
||||||
|
self._pipeline = pipeline
|
||||||
|
|
||||||
|
def detect(self, image: Image.Image, candidate_labels: list[str], threshold: float = 0.1) -> list[DetectionResult]:
|
||||||
|
results = self._pipeline(image=image, candidate_labels=candidate_labels, threshold=threshold)
|
||||||
|
assert results is not None
|
||||||
|
results = [DetectionResult.model_validate(result) for result in results]
|
||||||
|
return results
|
||||||
|
|
||||||
|
def to(self, device: Optional[torch.device] = None, dtype: Optional[torch.dtype] = None):
|
||||||
|
# HACK(ryand): The GroundingDinoPipeline does not work on MPS devices. We only allow it to be moved to CPU or
|
||||||
|
# CUDA.
|
||||||
|
if device is not None and device.type not in {"cpu", "cuda"}:
|
||||||
|
device = None
|
||||||
|
self._pipeline.model.to(device=device, dtype=dtype)
|
||||||
|
self._pipeline.device = self._pipeline.model.device
|
||||||
|
|
||||||
|
def calc_size(self) -> int:
|
||||||
|
# HACK(ryand): Fix the circular import issue.
|
||||||
|
from invokeai.backend.model_manager.load.model_util import calc_module_size
|
||||||
|
|
||||||
|
return calc_module_size(self._pipeline.model)
|
@ -0,0 +1,50 @@
|
|||||||
|
# This file contains utilities for Grounded-SAM mask refinement based on:
|
||||||
|
# https://github.com/NielsRogge/Transformers-Tutorials/blob/a39f33ac1557b02ebfb191ea7753e332b5ca933f/Grounding%20DINO/GroundingDINO_with_Segment_Anything.ipynb
|
||||||
|
|
||||||
|
|
||||||
|
import cv2
|
||||||
|
import numpy as np
|
||||||
|
import numpy.typing as npt
|
||||||
|
|
||||||
|
|
||||||
|
def mask_to_polygon(mask: npt.NDArray[np.uint8]) -> list[tuple[int, int]]:
|
||||||
|
"""Convert a binary mask to a polygon.
|
||||||
|
|
||||||
|
Returns:
|
||||||
|
list[list[int]]: List of (x, y) coordinates representing the vertices of the polygon.
|
||||||
|
"""
|
||||||
|
# Find contours in the binary mask.
|
||||||
|
contours, _ = cv2.findContours(mask.astype(np.uint8), cv2.RETR_EXTERNAL, cv2.CHAIN_APPROX_SIMPLE)
|
||||||
|
|
||||||
|
# Find the contour with the largest area.
|
||||||
|
largest_contour = max(contours, key=cv2.contourArea)
|
||||||
|
|
||||||
|
# Extract the vertices of the contour.
|
||||||
|
polygon = largest_contour.reshape(-1, 2).tolist()
|
||||||
|
|
||||||
|
return polygon
|
||||||
|
|
||||||
|
|
||||||
|
def polygon_to_mask(
|
||||||
|
polygon: list[tuple[int, int]], image_shape: tuple[int, int], fill_value: int = 1
|
||||||
|
) -> npt.NDArray[np.uint8]:
|
||||||
|
"""Convert a polygon to a segmentation mask.
|
||||||
|
|
||||||
|
Args:
|
||||||
|
polygon (list): List of (x, y) coordinates representing the vertices of the polygon.
|
||||||
|
image_shape (tuple): Shape of the image (height, width) for the mask.
|
||||||
|
fill_value (int): Value to fill the polygon with.
|
||||||
|
|
||||||
|
Returns:
|
||||||
|
np.ndarray: Segmentation mask with the polygon filled (with value 255).
|
||||||
|
"""
|
||||||
|
# Create an empty mask.
|
||||||
|
mask = np.zeros(image_shape, dtype=np.uint8)
|
||||||
|
|
||||||
|
# Convert polygon to an array of points.
|
||||||
|
pts = np.array(polygon, dtype=np.int32)
|
||||||
|
|
||||||
|
# Fill the polygon with white color (255).
|
||||||
|
cv2.fillPoly(mask, [pts], color=(fill_value,))
|
||||||
|
|
||||||
|
return mask
|
@ -0,0 +1,53 @@
|
|||||||
|
from typing import Optional
|
||||||
|
|
||||||
|
import torch
|
||||||
|
from PIL import Image
|
||||||
|
from transformers.models.sam import SamModel
|
||||||
|
from transformers.models.sam.processing_sam import SamProcessor
|
||||||
|
|
||||||
|
from invokeai.backend.raw_model import RawModel
|
||||||
|
|
||||||
|
|
||||||
|
class SegmentAnythingPipeline(RawModel):
|
||||||
|
"""A wrapper class for the transformers SAM model and processor that makes it compatible with the model manager."""
|
||||||
|
|
||||||
|
def __init__(self, sam_model: SamModel, sam_processor: SamProcessor):
|
||||||
|
self._sam_model = sam_model
|
||||||
|
self._sam_processor = sam_processor
|
||||||
|
|
||||||
|
def to(self, device: Optional[torch.device] = None, dtype: Optional[torch.dtype] = None):
|
||||||
|
# HACK(ryand): The SAM pipeline does not work on MPS devices. We only allow it to be moved to CPU or CUDA.
|
||||||
|
if device is not None and device.type not in {"cpu", "cuda"}:
|
||||||
|
device = None
|
||||||
|
self._sam_model.to(device=device, dtype=dtype)
|
||||||
|
|
||||||
|
def calc_size(self) -> int:
|
||||||
|
# HACK(ryand): Fix the circular import issue.
|
||||||
|
from invokeai.backend.model_manager.load.model_util import calc_module_size
|
||||||
|
|
||||||
|
return calc_module_size(self._sam_model)
|
||||||
|
|
||||||
|
def segment(self, image: Image.Image, bounding_boxes: list[list[int]]) -> torch.Tensor:
|
||||||
|
"""Run the SAM model.
|
||||||
|
|
||||||
|
Args:
|
||||||
|
image (Image.Image): The image to segment.
|
||||||
|
bounding_boxes (list[list[int]]): The bounding box prompts. Each bounding box is in the format
|
||||||
|
[xmin, ymin, xmax, ymax].
|
||||||
|
|
||||||
|
Returns:
|
||||||
|
torch.Tensor: The segmentation masks. dtype: torch.bool. shape: [num_masks, channels, height, width].
|
||||||
|
"""
|
||||||
|
# Add batch dimension of 1 to the bounding boxes.
|
||||||
|
boxes = [bounding_boxes]
|
||||||
|
inputs = self._sam_processor(images=image, input_boxes=boxes, return_tensors="pt").to(self._sam_model.device)
|
||||||
|
outputs = self._sam_model(**inputs)
|
||||||
|
masks = self._sam_processor.post_process_masks(
|
||||||
|
masks=outputs.pred_masks,
|
||||||
|
original_sizes=inputs.original_sizes,
|
||||||
|
reshaped_input_sizes=inputs.reshaped_input_sizes,
|
||||||
|
)
|
||||||
|
|
||||||
|
# There should be only one batch.
|
||||||
|
assert len(masks) == 1
|
||||||
|
return masks[0]
|
@ -11,6 +11,8 @@ from diffusers.pipelines.pipeline_utils import DiffusionPipeline
|
|||||||
from diffusers.schedulers.scheduling_utils import SchedulerMixin
|
from diffusers.schedulers.scheduling_utils import SchedulerMixin
|
||||||
from transformers import CLIPTokenizer
|
from transformers import CLIPTokenizer
|
||||||
|
|
||||||
|
from invokeai.backend.image_util.grounding_dino.grounding_dino_pipeline import GroundingDinoPipeline
|
||||||
|
from invokeai.backend.image_util.segment_anything.segment_anything_pipeline import SegmentAnythingPipeline
|
||||||
from invokeai.backend.ip_adapter.ip_adapter import IPAdapter
|
from invokeai.backend.ip_adapter.ip_adapter import IPAdapter
|
||||||
from invokeai.backend.lora import LoRAModelRaw
|
from invokeai.backend.lora import LoRAModelRaw
|
||||||
from invokeai.backend.model_manager.config import AnyModel
|
from invokeai.backend.model_manager.config import AnyModel
|
||||||
@ -34,7 +36,17 @@ def calc_model_size_by_data(logger: logging.Logger, model: AnyModel) -> int:
|
|||||||
elif isinstance(model, CLIPTokenizer):
|
elif isinstance(model, CLIPTokenizer):
|
||||||
# TODO(ryand): Accurately calculate the tokenizer's size. It's small enough that it shouldn't matter for now.
|
# TODO(ryand): Accurately calculate the tokenizer's size. It's small enough that it shouldn't matter for now.
|
||||||
return 0
|
return 0
|
||||||
elif isinstance(model, (TextualInversionModelRaw, IPAdapter, LoRAModelRaw, SpandrelImageToImageModel)):
|
elif isinstance(
|
||||||
|
model,
|
||||||
|
(
|
||||||
|
TextualInversionModelRaw,
|
||||||
|
IPAdapter,
|
||||||
|
LoRAModelRaw,
|
||||||
|
SpandrelImageToImageModel,
|
||||||
|
GroundingDinoPipeline,
|
||||||
|
SegmentAnythingPipeline,
|
||||||
|
),
|
||||||
|
):
|
||||||
return model.calc_size()
|
return model.calc_size()
|
||||||
else:
|
else:
|
||||||
# TODO(ryand): Promote this from a log to an exception once we are confident that we are handling all of the
|
# TODO(ryand): Promote this from a log to an exception once we are confident that we are handling all of the
|
||||||
|
Reference in New Issue
Block a user