mirror of
https://github.com/invoke-ai/InvokeAI
synced 2024-08-30 20:32:17 +00:00
Merge branch 'main' into bugfix/set-vram-on-macs
This commit is contained in:
commit
c54c1f603b
36
.github/CODEOWNERS
vendored
36
.github/CODEOWNERS
vendored
@ -1,34 +1,34 @@
|
||||
# continuous integration
|
||||
/.github/workflows/ @lstein @blessedcoolant
|
||||
/.github/workflows/ @lstein @blessedcoolant @hipsterusername
|
||||
|
||||
# documentation
|
||||
/docs/ @lstein @blessedcoolant @hipsterusername @Millu
|
||||
/mkdocs.yml @lstein @blessedcoolant
|
||||
/mkdocs.yml @lstein @blessedcoolant @hipsterusername @Millu
|
||||
|
||||
# nodes
|
||||
/invokeai/app/ @Kyle0654 @blessedcoolant @psychedelicious @brandonrising
|
||||
/invokeai/app/ @Kyle0654 @blessedcoolant @psychedelicious @brandonrising @hipsterusername
|
||||
|
||||
# installation and configuration
|
||||
/pyproject.toml @lstein @blessedcoolant
|
||||
/docker/ @lstein @blessedcoolant
|
||||
/scripts/ @ebr @lstein
|
||||
/installer/ @lstein @ebr
|
||||
/invokeai/assets @lstein @ebr
|
||||
/invokeai/configs @lstein
|
||||
/invokeai/version @lstein @blessedcoolant
|
||||
/pyproject.toml @lstein @blessedcoolant @hipsterusername
|
||||
/docker/ @lstein @blessedcoolant @hipsterusername
|
||||
/scripts/ @ebr @lstein @hipsterusername
|
||||
/installer/ @lstein @ebr @hipsterusername
|
||||
/invokeai/assets @lstein @ebr @hipsterusername
|
||||
/invokeai/configs @lstein @hipsterusername
|
||||
/invokeai/version @lstein @blessedcoolant @hipsterusername
|
||||
|
||||
# web ui
|
||||
/invokeai/frontend @blessedcoolant @psychedelicious @lstein @maryhipp
|
||||
/invokeai/backend @blessedcoolant @psychedelicious @lstein @maryhipp
|
||||
/invokeai/frontend @blessedcoolant @psychedelicious @lstein @maryhipp @hipsterusername
|
||||
/invokeai/backend @blessedcoolant @psychedelicious @lstein @maryhipp @hipsterusername
|
||||
|
||||
# generation, model management, postprocessing
|
||||
/invokeai/backend @damian0815 @lstein @blessedcoolant @gregghelt2 @StAlKeR7779 @brandonrising @ryanjdick
|
||||
/invokeai/backend @damian0815 @lstein @blessedcoolant @gregghelt2 @StAlKeR7779 @brandonrising @ryanjdick @hipsterusername
|
||||
|
||||
# front ends
|
||||
/invokeai/frontend/CLI @lstein
|
||||
/invokeai/frontend/install @lstein @ebr
|
||||
/invokeai/frontend/merge @lstein @blessedcoolant
|
||||
/invokeai/frontend/training @lstein @blessedcoolant
|
||||
/invokeai/frontend/web @psychedelicious @blessedcoolant @maryhipp
|
||||
/invokeai/frontend/CLI @lstein @hipsterusername
|
||||
/invokeai/frontend/install @lstein @ebr @hipsterusername
|
||||
/invokeai/frontend/merge @lstein @blessedcoolant @hipsterusername
|
||||
/invokeai/frontend/training @lstein @blessedcoolant @hipsterusername
|
||||
/invokeai/frontend/web @psychedelicious @blessedcoolant @maryhipp @hipsterusername
|
||||
|
||||
|
||||
|
@ -244,8 +244,12 @@ copy-paste the template above.
|
||||
We can use the `@invocation` decorator to provide some additional info to the
|
||||
UI, like a custom title, tags and category.
|
||||
|
||||
We also encourage providing a version. This must be a
|
||||
[semver](https://semver.org/) version string ("$MAJOR.$MINOR.$PATCH"). The UI
|
||||
will let users know if their workflow is using a mismatched version of the node.
|
||||
|
||||
```python
|
||||
@invocation("resize", title="My Resizer", tags=["resize", "image"], category="My Invocations")
|
||||
@invocation("resize", title="My Resizer", tags=["resize", "image"], category="My Invocations", version="1.0.0")
|
||||
class ResizeInvocation(BaseInvocation):
|
||||
"""Resizes an image"""
|
||||
|
||||
@ -279,8 +283,6 @@ take a look a at our [contributing nodes overview](contributingNodes).
|
||||
|
||||
## Advanced
|
||||
|
||||
-->
|
||||
|
||||
### Custom Output Types
|
||||
|
||||
Like with custom inputs, sometimes you might find yourself needing custom
|
||||
|
@ -22,12 +22,26 @@ To use a community node graph, download the the `.json` node graph file and load
|
||||

|
||||
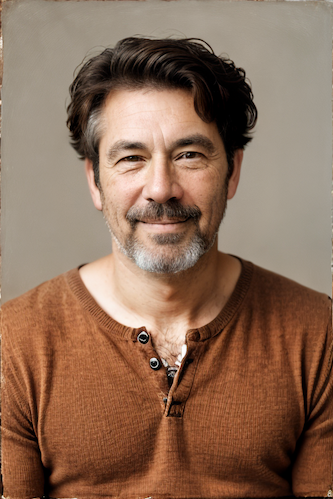
|
||||
|
||||
--------------------------------
|
||||
### Ideal Size
|
||||
|
||||
**Description:** This node calculates an ideal image size for a first pass of a multi-pass upscaling. The aim is to avoid duplication that results from choosing a size larger than the model is capable of.
|
||||
|
||||
**Node Link:** https://github.com/JPPhoto/ideal-size-node
|
||||
|
||||
--------------------------------
|
||||
### Film Grain
|
||||
|
||||
**Description:** This node adds a film grain effect to the input image based on the weights, seeds, and blur radii parameters. It works with RGB input images only.
|
||||
|
||||
**Node Link:** https://github.com/JPPhoto/film-grain-node
|
||||
|
||||
--------------------------------
|
||||
### Image Picker
|
||||
|
||||
**Description:** This InvokeAI node takes in a collection of images and randomly chooses one. This can be useful when you have a number of poses to choose from for a ControlNet node, or a number of input images for another purpose.
|
||||
|
||||
**Node Link:** https://github.com/JPPhoto/image-picker-node
|
||||
|
||||
--------------------------------
|
||||
### Retroize
|
||||
@ -95,6 +109,91 @@ a Text-Generation-Webui instance (might work remotely too, but I never tried it)
|
||||
|
||||
This node works best with SDXL models, especially as the style can be described independantly of the LLM's output.
|
||||
|
||||
--------------------------------
|
||||
### Depth Map from Wavefront OBJ
|
||||
|
||||
**Description:** Render depth maps from Wavefront .obj files (triangulated) using this simple 3D renderer utilizing numpy and matplotlib to compute and color the scene. There are simple parameters to change the FOV, camera position, and model orientation.
|
||||
|
||||
To be imported, an .obj must use triangulated meshes, so make sure to enable that option if exporting from a 3D modeling program. This renderer makes each triangle a solid color based on its average depth, so it will cause anomalies if your .obj has large triangles. In Blender, the Remesh modifier can be helpful to subdivide a mesh into small pieces that work well given these limitations.
|
||||
|
||||
**Node Link:** https://github.com/dwringer/depth-from-obj-node
|
||||
|
||||
**Example Usage:**
|
||||
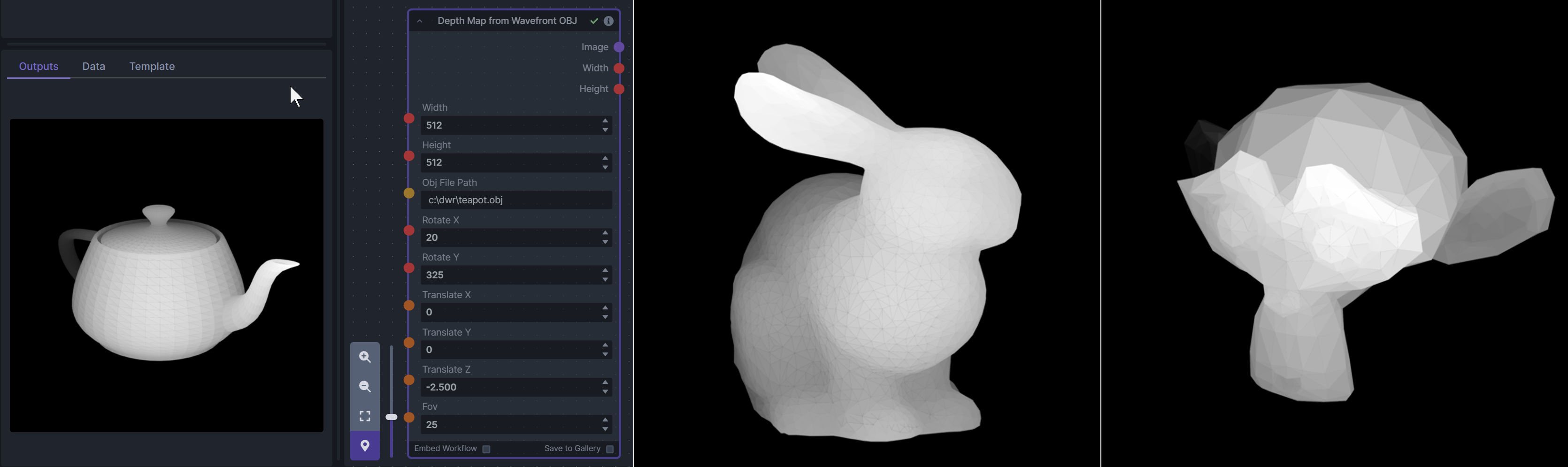
|
||||
|
||||
--------------------------------
|
||||
### Enhance Image (simple adjustments)
|
||||
|
||||
**Description:** Boost or reduce color saturation, contrast, brightness, sharpness, or invert colors of any image at any stage with this simple wrapper for pillow [PIL]'s ImageEnhance module.
|
||||
|
||||
Color inversion is toggled with a simple switch, while each of the four enhancer modes are activated by entering a value other than 1 in each corresponding input field. Values less than 1 will reduce the corresponding property, while values greater than 1 will enhance it.
|
||||
|
||||
**Node Link:** https://github.com/dwringer/image-enhance-node
|
||||
|
||||
**Example Usage:**
|
||||
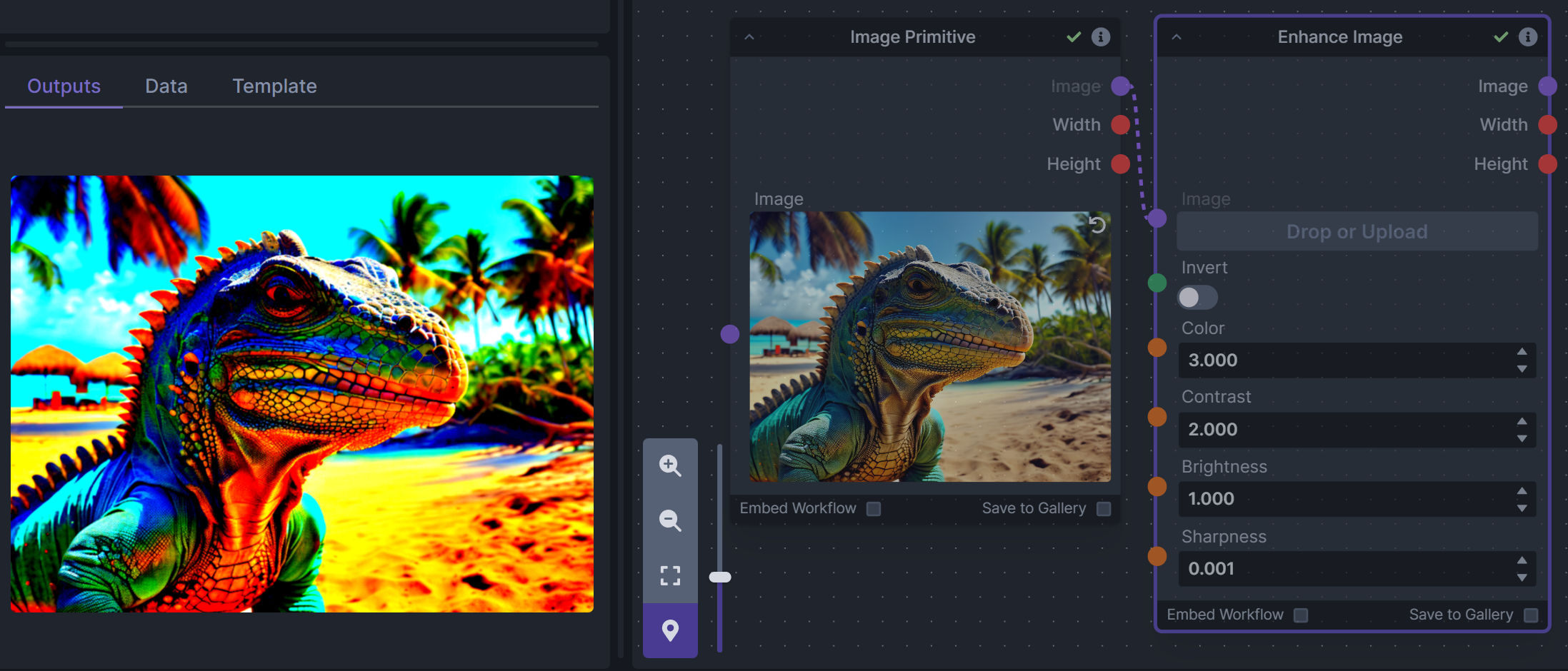
|
||||
|
||||
--------------------------------
|
||||
### Generative Grammar-Based Prompt Nodes
|
||||
|
||||
**Description:** This set of 3 nodes generates prompts from simple user-defined grammar rules (loaded from custom files - examples provided below). The prompts are made by recursively expanding a special template string, replacing nonterminal "parts-of-speech" until no more nonterminal terms remain in the string.
|
||||
|
||||
This includes 3 Nodes:
|
||||
- *Lookup Table from File* - loads a YAML file "prompt" section (or of a whole folder of YAML's) into a JSON-ified dictionary (Lookups output)
|
||||
- *Lookups Entry from Prompt* - places a single entry in a new Lookups output under the specified heading
|
||||
- *Prompt from Lookup Table* - uses a Collection of Lookups as grammar rules from which to randomly generate prompts.
|
||||
|
||||
**Node Link:** https://github.com/dwringer/generative-grammar-prompt-nodes
|
||||
|
||||
**Example Usage:**
|
||||
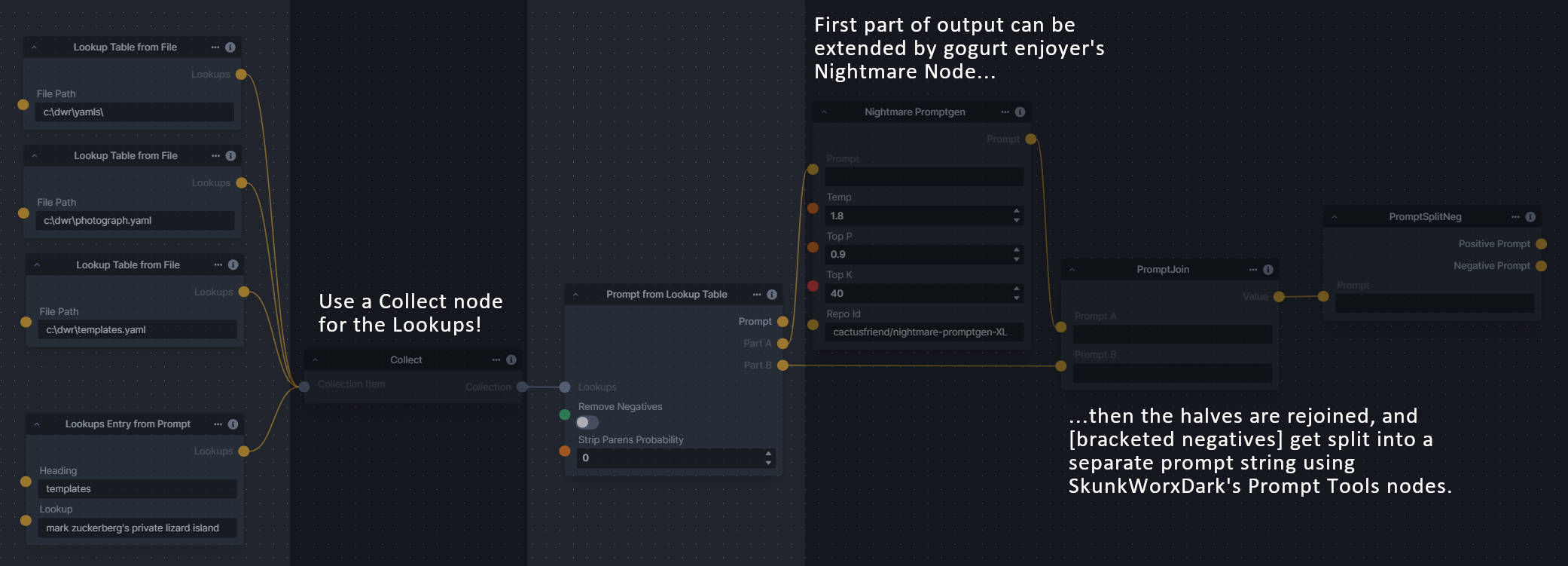
|
||||
|
||||
--------------------------------
|
||||
### Image and Mask Composition Pack
|
||||
|
||||
**Description:** This is a pack of nodes for composing masks and images, including a simple text mask creator and both image and latent offset nodes. The offsets wrap around, so these can be used in conjunction with the Seamless node to progressively generate centered on different parts of the seamless tiling.
|
||||
|
||||
This includes 4 Nodes:
|
||||
- *Text Mask (simple 2D)* - create and position a white on black (or black on white) line of text using any font locally available to Invoke.
|
||||
- *Image Compositor* - Take a subject from an image with a flat backdrop and layer it on another image using a chroma key or flood select background removal.
|
||||
- *Offset Latents* - Offset a latents tensor in the vertical and/or horizontal dimensions, wrapping it around.
|
||||
- *Offset Image* - Offset an image in the vertical and/or horizontal dimensions, wrapping it around.
|
||||
|
||||
**Node Link:** https://github.com/dwringer/composition-nodes
|
||||
|
||||
**Example Usage:**
|
||||
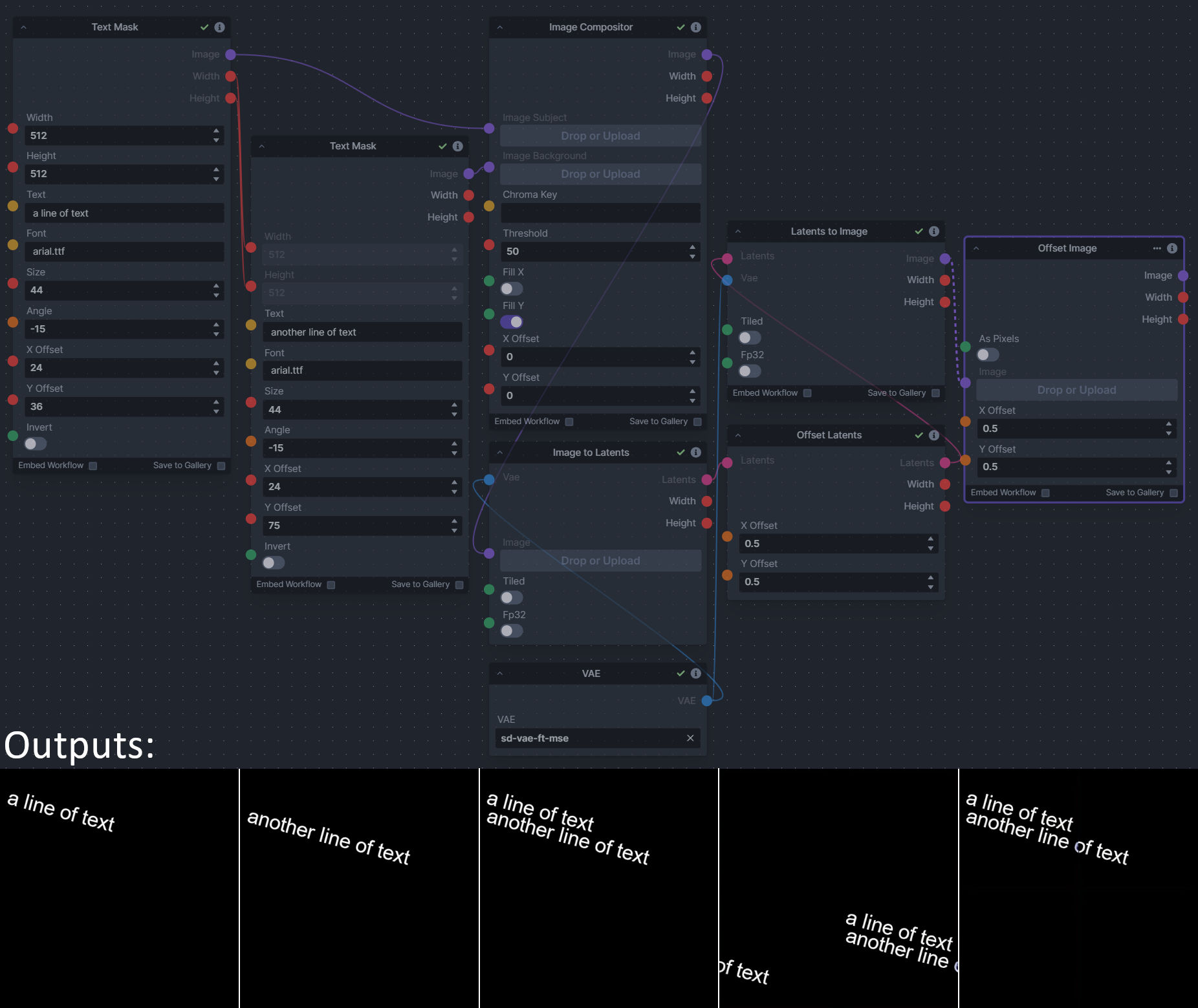
|
||||
|
||||
--------------------------------
|
||||
### Size Stepper Nodes
|
||||
|
||||
**Description:** This is a set of nodes for calculating the necessary size increments for doing upscaling workflows. Use the *Final Size & Orientation* node to enter your full size dimensions and orientation (portrait/landscape/random), then plug that and your initial generation dimensions into the *Ideal Size Stepper* and get 1, 2, or 3 intermediate pairs of dimensions for upscaling. Note this does not output the initial size or full size dimensions: the 1, 2, or 3 outputs of this node are only the intermediate sizes.
|
||||
|
||||
A third node is included, *Random Switch (Integers)*, which is just a generic version of Final Size with no orientation selection.
|
||||
|
||||
**Node Link:** https://github.com/dwringer/size-stepper-nodes
|
||||
|
||||
**Example Usage:**
|
||||
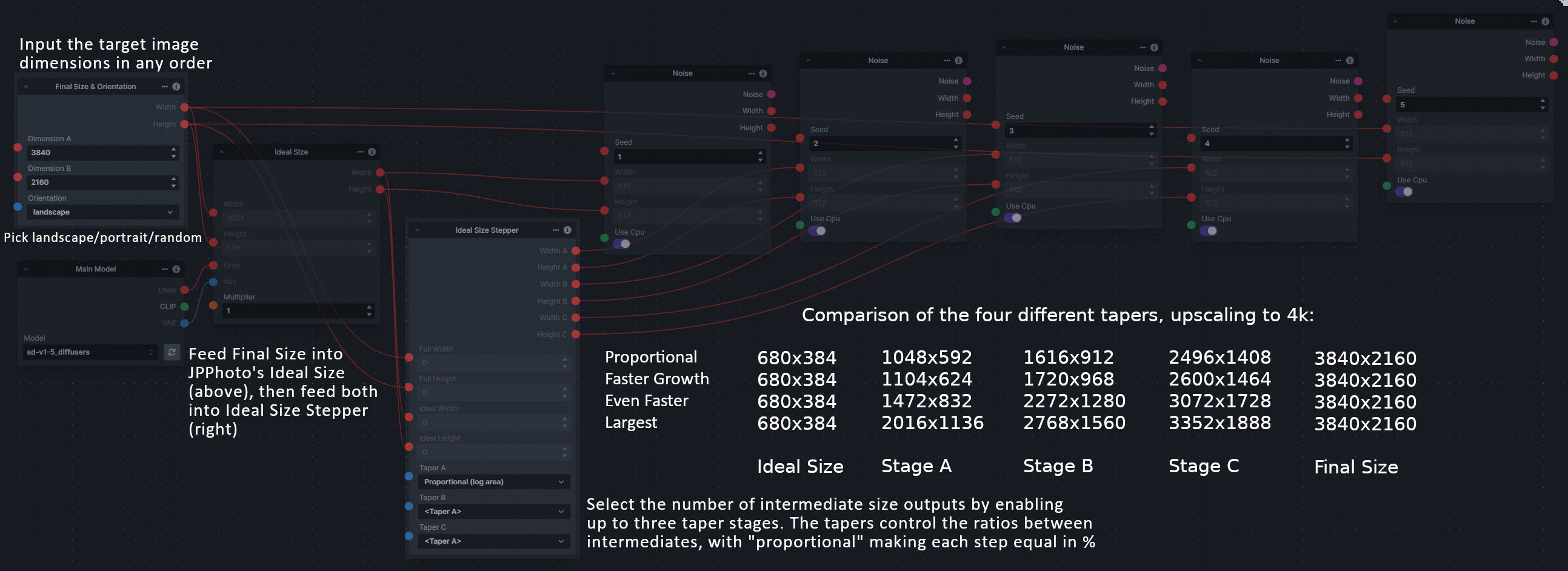
|
||||
|
||||
--------------------------------
|
||||
|
||||
### Text font to Image
|
||||
|
||||
**Description:** text font to text image node for InvokeAI, download a font to use (or if in font cache uses it from there), the text is always resized to the image size, but can control that with padding, optional 2nd line
|
||||
|
||||
**Node Link:** https://github.com/mickr777/textfontimage
|
||||
|
||||
**Output Examples**
|
||||
|
||||

|
||||
|
||||
Results after using the depth controlnet
|
||||
|
||||

|
||||
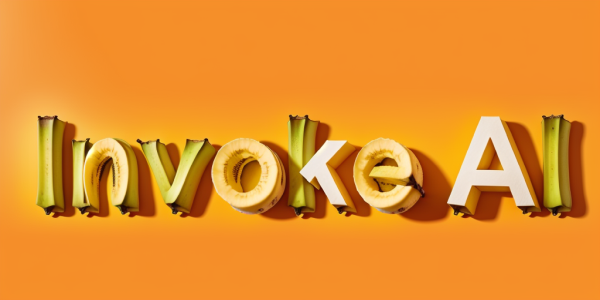
|
||||

|
||||
|
||||
--------------------------------
|
||||
|
||||
### Example Node Template
|
||||
|
@ -35,13 +35,13 @@ The table below contains a list of the default nodes shipped with InvokeAI and t
|
||||
|Inverse Lerp Image | Inverse linear interpolation of all pixels of an image|
|
||||
|Image Primitive | An image primitive value|
|
||||
|Lerp Image | Linear interpolation of all pixels of an image|
|
||||
|Image Luminosity Adjustment | Adjusts the Luminosity (Value) of an image.|
|
||||
|Offset Image Channel | Add to or subtract from an image color channel by a uniform value.|
|
||||
|Multiply Image Channel | Multiply or Invert an image color channel by a scalar value.|
|
||||
|Multiply Images | Multiplies two images together using `PIL.ImageChops.multiply()`.|
|
||||
|Blur NSFW Image | Add blur to NSFW-flagged images|
|
||||
|Paste Image | Pastes an image into another image.|
|
||||
|ImageProcessor | Base class for invocations that preprocess images for ControlNet|
|
||||
|Resize Image | Resizes an image to specific dimensions|
|
||||
|Image Saturation Adjustment | Adjusts the Saturation of an image.|
|
||||
|Scale Image | Scales an image by a factor|
|
||||
|Image to Latents | Encodes an image into latents.|
|
||||
|Add Invisible Watermark | Add an invisible watermark to an image|
|
||||
|
@ -1,19 +1,19 @@
|
||||
import typing
|
||||
from enum import Enum
|
||||
from pathlib import Path
|
||||
|
||||
from fastapi import Body
|
||||
from fastapi.routing import APIRouter
|
||||
from pathlib import Path
|
||||
from pydantic import BaseModel, Field
|
||||
|
||||
from invokeai.app.invocations.upscale import ESRGAN_MODELS
|
||||
from invokeai.backend.image_util.invisible_watermark import InvisibleWatermark
|
||||
from invokeai.backend.image_util.patchmatch import PatchMatch
|
||||
from invokeai.backend.image_util.safety_checker import SafetyChecker
|
||||
from invokeai.backend.image_util.invisible_watermark import InvisibleWatermark
|
||||
from invokeai.app.invocations.upscale import ESRGAN_MODELS
|
||||
|
||||
from invokeai.backend.util.logging import logging
|
||||
from invokeai.version import __version__
|
||||
|
||||
from ..dependencies import ApiDependencies
|
||||
from invokeai.backend.util.logging import logging
|
||||
|
||||
|
||||
class LogLevel(int, Enum):
|
||||
@ -55,7 +55,7 @@ async def get_version() -> AppVersion:
|
||||
|
||||
@app_router.get("/config", operation_id="get_config", status_code=200, response_model=AppConfig)
|
||||
async def get_config() -> AppConfig:
|
||||
infill_methods = ["tile", "lama"]
|
||||
infill_methods = ["tile", "lama", "cv2"]
|
||||
if PatchMatch.patchmatch_available():
|
||||
infill_methods.append("patchmatch")
|
||||
|
||||
|
@ -26,11 +26,16 @@ from typing import (
|
||||
from pydantic import BaseModel, Field, validator
|
||||
from pydantic.fields import Undefined, ModelField
|
||||
from pydantic.typing import NoArgAnyCallable
|
||||
import semver
|
||||
|
||||
if TYPE_CHECKING:
|
||||
from ..services.invocation_services import InvocationServices
|
||||
|
||||
|
||||
class InvalidVersionError(ValueError):
|
||||
pass
|
||||
|
||||
|
||||
class FieldDescriptions:
|
||||
denoising_start = "When to start denoising, expressed a percentage of total steps"
|
||||
denoising_end = "When to stop denoising, expressed a percentage of total steps"
|
||||
@ -105,24 +110,39 @@ class UIType(str, Enum):
|
||||
"""
|
||||
|
||||
# region Primitives
|
||||
Integer = "integer"
|
||||
Float = "float"
|
||||
Boolean = "boolean"
|
||||
String = "string"
|
||||
Array = "array"
|
||||
Image = "ImageField"
|
||||
Latents = "LatentsField"
|
||||
Color = "ColorField"
|
||||
Conditioning = "ConditioningField"
|
||||
Control = "ControlField"
|
||||
Color = "ColorField"
|
||||
ImageCollection = "ImageCollection"
|
||||
ConditioningCollection = "ConditioningCollection"
|
||||
ColorCollection = "ColorCollection"
|
||||
LatentsCollection = "LatentsCollection"
|
||||
IntegerCollection = "IntegerCollection"
|
||||
FloatCollection = "FloatCollection"
|
||||
StringCollection = "StringCollection"
|
||||
Float = "float"
|
||||
Image = "ImageField"
|
||||
Integer = "integer"
|
||||
Latents = "LatentsField"
|
||||
String = "string"
|
||||
# endregion
|
||||
|
||||
# region Collection Primitives
|
||||
BooleanCollection = "BooleanCollection"
|
||||
ColorCollection = "ColorCollection"
|
||||
ConditioningCollection = "ConditioningCollection"
|
||||
ControlCollection = "ControlCollection"
|
||||
FloatCollection = "FloatCollection"
|
||||
ImageCollection = "ImageCollection"
|
||||
IntegerCollection = "IntegerCollection"
|
||||
LatentsCollection = "LatentsCollection"
|
||||
StringCollection = "StringCollection"
|
||||
# endregion
|
||||
|
||||
# region Polymorphic Primitives
|
||||
BooleanPolymorphic = "BooleanPolymorphic"
|
||||
ColorPolymorphic = "ColorPolymorphic"
|
||||
ConditioningPolymorphic = "ConditioningPolymorphic"
|
||||
ControlPolymorphic = "ControlPolymorphic"
|
||||
FloatPolymorphic = "FloatPolymorphic"
|
||||
ImagePolymorphic = "ImagePolymorphic"
|
||||
IntegerPolymorphic = "IntegerPolymorphic"
|
||||
LatentsPolymorphic = "LatentsPolymorphic"
|
||||
StringPolymorphic = "StringPolymorphic"
|
||||
# endregion
|
||||
|
||||
# region Models
|
||||
@ -176,6 +196,7 @@ class _InputField(BaseModel):
|
||||
ui_type: Optional[UIType]
|
||||
ui_component: Optional[UIComponent]
|
||||
ui_order: Optional[int]
|
||||
item_default: Optional[Any]
|
||||
|
||||
|
||||
class _OutputField(BaseModel):
|
||||
@ -223,6 +244,7 @@ def InputField(
|
||||
ui_component: Optional[UIComponent] = None,
|
||||
ui_hidden: bool = False,
|
||||
ui_order: Optional[int] = None,
|
||||
item_default: Optional[Any] = None,
|
||||
**kwargs: Any,
|
||||
) -> Any:
|
||||
"""
|
||||
@ -249,6 +271,11 @@ def InputField(
|
||||
For this case, you could provide `UIComponent.Textarea`.
|
||||
|
||||
: param bool ui_hidden: [False] Specifies whether or not this field should be hidden in the UI.
|
||||
|
||||
: param int ui_order: [None] Specifies the order in which this field should be rendered in the UI. \
|
||||
|
||||
: param bool item_default: [None] Specifies the default item value, if this is a collection input. \
|
||||
Ignored for non-collection fields..
|
||||
"""
|
||||
return Field(
|
||||
*args,
|
||||
@ -282,6 +309,7 @@ def InputField(
|
||||
ui_component=ui_component,
|
||||
ui_hidden=ui_hidden,
|
||||
ui_order=ui_order,
|
||||
item_default=item_default,
|
||||
**kwargs,
|
||||
)
|
||||
|
||||
@ -332,6 +360,8 @@ def OutputField(
|
||||
`UIType.SDXLMainModelField` to indicate that the field is an SDXL main model field.
|
||||
|
||||
: param bool ui_hidden: [False] Specifies whether or not this field should be hidden in the UI. \
|
||||
|
||||
: param int ui_order: [None] Specifies the order in which this field should be rendered in the UI. \
|
||||
"""
|
||||
return Field(
|
||||
*args,
|
||||
@ -376,6 +406,9 @@ class UIConfigBase(BaseModel):
|
||||
tags: Optional[list[str]] = Field(default_factory=None, description="The node's tags")
|
||||
title: Optional[str] = Field(default=None, description="The node's display name")
|
||||
category: Optional[str] = Field(default=None, description="The node's category")
|
||||
version: Optional[str] = Field(
|
||||
default=None, description='The node\'s version. Should be a valid semver string e.g. "1.0.0" or "3.8.13".'
|
||||
)
|
||||
|
||||
|
||||
class InvocationContext:
|
||||
@ -474,6 +507,8 @@ class BaseInvocation(ABC, BaseModel):
|
||||
schema["tags"] = uiconfig.tags
|
||||
if uiconfig and hasattr(uiconfig, "category"):
|
||||
schema["category"] = uiconfig.category
|
||||
if uiconfig and hasattr(uiconfig, "version"):
|
||||
schema["version"] = uiconfig.version
|
||||
if "required" not in schema or not isinstance(schema["required"], list):
|
||||
schema["required"] = list()
|
||||
schema["required"].extend(["type", "id"])
|
||||
@ -542,7 +577,11 @@ GenericBaseInvocation = TypeVar("GenericBaseInvocation", bound=BaseInvocation)
|
||||
|
||||
|
||||
def invocation(
|
||||
invocation_type: str, title: Optional[str] = None, tags: Optional[list[str]] = None, category: Optional[str] = None
|
||||
invocation_type: str,
|
||||
title: Optional[str] = None,
|
||||
tags: Optional[list[str]] = None,
|
||||
category: Optional[str] = None,
|
||||
version: Optional[str] = None,
|
||||
) -> Callable[[Type[GenericBaseInvocation]], Type[GenericBaseInvocation]]:
|
||||
"""
|
||||
Adds metadata to an invocation.
|
||||
@ -569,6 +608,12 @@ def invocation(
|
||||
cls.UIConfig.tags = tags
|
||||
if category is not None:
|
||||
cls.UIConfig.category = category
|
||||
if version is not None:
|
||||
try:
|
||||
semver.Version.parse(version)
|
||||
except ValueError as e:
|
||||
raise InvalidVersionError(f'Invalid version string for node "{invocation_type}": "{version}"') from e
|
||||
cls.UIConfig.version = version
|
||||
|
||||
# Add the invocation type to the pydantic model of the invocation
|
||||
invocation_type_annotation = Literal[invocation_type] # type: ignore
|
||||
@ -580,8 +625,9 @@ def invocation(
|
||||
config=cls.__config__,
|
||||
)
|
||||
cls.__fields__.update({"type": invocation_type_field})
|
||||
cls.__annotations__.update({"type": invocation_type_annotation})
|
||||
|
||||
# to support 3.9, 3.10 and 3.11, as described in https://docs.python.org/3/howto/annotations.html
|
||||
if annotations := cls.__dict__.get("__annotations__", None):
|
||||
annotations.update({"type": invocation_type_annotation})
|
||||
return cls
|
||||
|
||||
return wrapper
|
||||
@ -615,7 +661,10 @@ def invocation_output(
|
||||
config=cls.__config__,
|
||||
)
|
||||
cls.__fields__.update({"type": output_type_field})
|
||||
cls.__annotations__.update({"type": output_type_annotation})
|
||||
|
||||
# to support 3.9, 3.10 and 3.11, as described in https://docs.python.org/3/howto/annotations.html
|
||||
if annotations := cls.__dict__.get("__annotations__", None):
|
||||
annotations.update({"type": output_type_annotation})
|
||||
|
||||
return cls
|
||||
|
||||
|
@ -10,7 +10,9 @@ from invokeai.app.util.misc import SEED_MAX, get_random_seed
|
||||
from .baseinvocation import BaseInvocation, InputField, InvocationContext, invocation
|
||||
|
||||
|
||||
@invocation("range", title="Integer Range", tags=["collection", "integer", "range"], category="collections")
|
||||
@invocation(
|
||||
"range", title="Integer Range", tags=["collection", "integer", "range"], category="collections", version="1.0.0"
|
||||
)
|
||||
class RangeInvocation(BaseInvocation):
|
||||
"""Creates a range of numbers from start to stop with step"""
|
||||
|
||||
@ -33,6 +35,7 @@ class RangeInvocation(BaseInvocation):
|
||||
title="Integer Range of Size",
|
||||
tags=["collection", "integer", "size", "range"],
|
||||
category="collections",
|
||||
version="1.0.0",
|
||||
)
|
||||
class RangeOfSizeInvocation(BaseInvocation):
|
||||
"""Creates a range from start to start + size with step"""
|
||||
@ -50,6 +53,7 @@ class RangeOfSizeInvocation(BaseInvocation):
|
||||
title="Random Range",
|
||||
tags=["range", "integer", "random", "collection"],
|
||||
category="collections",
|
||||
version="1.0.0",
|
||||
)
|
||||
class RandomRangeInvocation(BaseInvocation):
|
||||
"""Creates a collection of random numbers"""
|
||||
|
@ -44,7 +44,7 @@ class ConditioningFieldData:
|
||||
# PerpNeg = "perp_neg"
|
||||
|
||||
|
||||
@invocation("compel", title="Prompt", tags=["prompt", "compel"], category="conditioning")
|
||||
@invocation("compel", title="Prompt", tags=["prompt", "compel"], category="conditioning", version="1.0.0")
|
||||
class CompelInvocation(BaseInvocation):
|
||||
"""Parse prompt using compel package to conditioning."""
|
||||
|
||||
@ -267,6 +267,7 @@ class SDXLPromptInvocationBase:
|
||||
title="SDXL Prompt",
|
||||
tags=["sdxl", "compel", "prompt"],
|
||||
category="conditioning",
|
||||
version="1.0.0",
|
||||
)
|
||||
class SDXLCompelPromptInvocation(BaseInvocation, SDXLPromptInvocationBase):
|
||||
"""Parse prompt using compel package to conditioning."""
|
||||
@ -351,6 +352,7 @@ class SDXLCompelPromptInvocation(BaseInvocation, SDXLPromptInvocationBase):
|
||||
title="SDXL Refiner Prompt",
|
||||
tags=["sdxl", "compel", "prompt"],
|
||||
category="conditioning",
|
||||
version="1.0.0",
|
||||
)
|
||||
class SDXLRefinerCompelPromptInvocation(BaseInvocation, SDXLPromptInvocationBase):
|
||||
"""Parse prompt using compel package to conditioning."""
|
||||
@ -403,7 +405,7 @@ class ClipSkipInvocationOutput(BaseInvocationOutput):
|
||||
clip: ClipField = OutputField(default=None, description=FieldDescriptions.clip, title="CLIP")
|
||||
|
||||
|
||||
@invocation("clip_skip", title="CLIP Skip", tags=["clipskip", "clip", "skip"], category="conditioning")
|
||||
@invocation("clip_skip", title="CLIP Skip", tags=["clipskip", "clip", "skip"], category="conditioning", version="1.0.0")
|
||||
class ClipSkipInvocation(BaseInvocation):
|
||||
"""Skip layers in clip text_encoder model."""
|
||||
|
||||
|
@ -95,14 +95,12 @@ class ControlOutput(BaseInvocationOutput):
|
||||
control: ControlField = OutputField(description=FieldDescriptions.control)
|
||||
|
||||
|
||||
@invocation("controlnet", title="ControlNet", tags=["controlnet"], category="controlnet")
|
||||
@invocation("controlnet", title="ControlNet", tags=["controlnet"], category="controlnet", version="1.0.0")
|
||||
class ControlNetInvocation(BaseInvocation):
|
||||
"""Collects ControlNet info to pass to other nodes"""
|
||||
|
||||
image: ImageField = InputField(description="The control image")
|
||||
control_model: ControlNetModelField = InputField(
|
||||
default="lllyasviel/sd-controlnet-canny", description=FieldDescriptions.controlnet_model, input=Input.Direct
|
||||
)
|
||||
control_model: ControlNetModelField = InputField(description=FieldDescriptions.controlnet_model, input=Input.Direct)
|
||||
control_weight: Union[float, List[float]] = InputField(
|
||||
default=1.0, description="The weight given to the ControlNet", ui_type=UIType.Float
|
||||
)
|
||||
@ -129,7 +127,9 @@ class ControlNetInvocation(BaseInvocation):
|
||||
)
|
||||
|
||||
|
||||
@invocation("image_processor", title="Base Image Processor", tags=["controlnet"], category="controlnet")
|
||||
@invocation(
|
||||
"image_processor", title="Base Image Processor", tags=["controlnet"], category="controlnet", version="1.0.0"
|
||||
)
|
||||
class ImageProcessorInvocation(BaseInvocation):
|
||||
"""Base class for invocations that preprocess images for ControlNet"""
|
||||
|
||||
@ -173,6 +173,7 @@ class ImageProcessorInvocation(BaseInvocation):
|
||||
title="Canny Processor",
|
||||
tags=["controlnet", "canny"],
|
||||
category="controlnet",
|
||||
version="1.0.0",
|
||||
)
|
||||
class CannyImageProcessorInvocation(ImageProcessorInvocation):
|
||||
"""Canny edge detection for ControlNet"""
|
||||
@ -195,6 +196,7 @@ class CannyImageProcessorInvocation(ImageProcessorInvocation):
|
||||
title="HED (softedge) Processor",
|
||||
tags=["controlnet", "hed", "softedge"],
|
||||
category="controlnet",
|
||||
version="1.0.0",
|
||||
)
|
||||
class HedImageProcessorInvocation(ImageProcessorInvocation):
|
||||
"""Applies HED edge detection to image"""
|
||||
@ -223,6 +225,7 @@ class HedImageProcessorInvocation(ImageProcessorInvocation):
|
||||
title="Lineart Processor",
|
||||
tags=["controlnet", "lineart"],
|
||||
category="controlnet",
|
||||
version="1.0.0",
|
||||
)
|
||||
class LineartImageProcessorInvocation(ImageProcessorInvocation):
|
||||
"""Applies line art processing to image"""
|
||||
@ -244,6 +247,7 @@ class LineartImageProcessorInvocation(ImageProcessorInvocation):
|
||||
title="Lineart Anime Processor",
|
||||
tags=["controlnet", "lineart", "anime"],
|
||||
category="controlnet",
|
||||
version="1.0.0",
|
||||
)
|
||||
class LineartAnimeImageProcessorInvocation(ImageProcessorInvocation):
|
||||
"""Applies line art anime processing to image"""
|
||||
@ -266,6 +270,7 @@ class LineartAnimeImageProcessorInvocation(ImageProcessorInvocation):
|
||||
title="Openpose Processor",
|
||||
tags=["controlnet", "openpose", "pose"],
|
||||
category="controlnet",
|
||||
version="1.0.0",
|
||||
)
|
||||
class OpenposeImageProcessorInvocation(ImageProcessorInvocation):
|
||||
"""Applies Openpose processing to image"""
|
||||
@ -290,6 +295,7 @@ class OpenposeImageProcessorInvocation(ImageProcessorInvocation):
|
||||
title="Midas Depth Processor",
|
||||
tags=["controlnet", "midas"],
|
||||
category="controlnet",
|
||||
version="1.0.0",
|
||||
)
|
||||
class MidasDepthImageProcessorInvocation(ImageProcessorInvocation):
|
||||
"""Applies Midas depth processing to image"""
|
||||
@ -316,6 +322,7 @@ class MidasDepthImageProcessorInvocation(ImageProcessorInvocation):
|
||||
title="Normal BAE Processor",
|
||||
tags=["controlnet"],
|
||||
category="controlnet",
|
||||
version="1.0.0",
|
||||
)
|
||||
class NormalbaeImageProcessorInvocation(ImageProcessorInvocation):
|
||||
"""Applies NormalBae processing to image"""
|
||||
@ -331,7 +338,9 @@ class NormalbaeImageProcessorInvocation(ImageProcessorInvocation):
|
||||
return processed_image
|
||||
|
||||
|
||||
@invocation("mlsd_image_processor", title="MLSD Processor", tags=["controlnet", "mlsd"], category="controlnet")
|
||||
@invocation(
|
||||
"mlsd_image_processor", title="MLSD Processor", tags=["controlnet", "mlsd"], category="controlnet", version="1.0.0"
|
||||
)
|
||||
class MlsdImageProcessorInvocation(ImageProcessorInvocation):
|
||||
"""Applies MLSD processing to image"""
|
||||
|
||||
@ -352,7 +361,9 @@ class MlsdImageProcessorInvocation(ImageProcessorInvocation):
|
||||
return processed_image
|
||||
|
||||
|
||||
@invocation("pidi_image_processor", title="PIDI Processor", tags=["controlnet", "pidi"], category="controlnet")
|
||||
@invocation(
|
||||
"pidi_image_processor", title="PIDI Processor", tags=["controlnet", "pidi"], category="controlnet", version="1.0.0"
|
||||
)
|
||||
class PidiImageProcessorInvocation(ImageProcessorInvocation):
|
||||
"""Applies PIDI processing to image"""
|
||||
|
||||
@ -378,6 +389,7 @@ class PidiImageProcessorInvocation(ImageProcessorInvocation):
|
||||
title="Content Shuffle Processor",
|
||||
tags=["controlnet", "contentshuffle"],
|
||||
category="controlnet",
|
||||
version="1.0.0",
|
||||
)
|
||||
class ContentShuffleImageProcessorInvocation(ImageProcessorInvocation):
|
||||
"""Applies content shuffle processing to image"""
|
||||
@ -407,6 +419,7 @@ class ContentShuffleImageProcessorInvocation(ImageProcessorInvocation):
|
||||
title="Zoe (Depth) Processor",
|
||||
tags=["controlnet", "zoe", "depth"],
|
||||
category="controlnet",
|
||||
version="1.0.0",
|
||||
)
|
||||
class ZoeDepthImageProcessorInvocation(ImageProcessorInvocation):
|
||||
"""Applies Zoe depth processing to image"""
|
||||
@ -422,6 +435,7 @@ class ZoeDepthImageProcessorInvocation(ImageProcessorInvocation):
|
||||
title="Mediapipe Face Processor",
|
||||
tags=["controlnet", "mediapipe", "face"],
|
||||
category="controlnet",
|
||||
version="1.0.0",
|
||||
)
|
||||
class MediapipeFaceProcessorInvocation(ImageProcessorInvocation):
|
||||
"""Applies mediapipe face processing to image"""
|
||||
@ -444,6 +458,7 @@ class MediapipeFaceProcessorInvocation(ImageProcessorInvocation):
|
||||
title="Leres (Depth) Processor",
|
||||
tags=["controlnet", "leres", "depth"],
|
||||
category="controlnet",
|
||||
version="1.0.0",
|
||||
)
|
||||
class LeresImageProcessorInvocation(ImageProcessorInvocation):
|
||||
"""Applies leres processing to image"""
|
||||
@ -472,6 +487,7 @@ class LeresImageProcessorInvocation(ImageProcessorInvocation):
|
||||
title="Tile Resample Processor",
|
||||
tags=["controlnet", "tile"],
|
||||
category="controlnet",
|
||||
version="1.0.0",
|
||||
)
|
||||
class TileResamplerProcessorInvocation(ImageProcessorInvocation):
|
||||
"""Tile resampler processor"""
|
||||
@ -511,6 +527,7 @@ class TileResamplerProcessorInvocation(ImageProcessorInvocation):
|
||||
title="Segment Anything Processor",
|
||||
tags=["controlnet", "segmentanything"],
|
||||
category="controlnet",
|
||||
version="1.0.0",
|
||||
)
|
||||
class SegmentAnythingProcessorInvocation(ImageProcessorInvocation):
|
||||
"""Applies segment anything processing to image"""
|
||||
|
@ -10,12 +10,7 @@ from invokeai.app.models.image import ImageCategory, ResourceOrigin
|
||||
from .baseinvocation import BaseInvocation, InputField, InvocationContext, invocation
|
||||
|
||||
|
||||
@invocation(
|
||||
"cv_inpaint",
|
||||
title="OpenCV Inpaint",
|
||||
tags=["opencv", "inpaint"],
|
||||
category="inpaint",
|
||||
)
|
||||
@invocation("cv_inpaint", title="OpenCV Inpaint", tags=["opencv", "inpaint"], category="inpaint", version="1.0.0")
|
||||
class CvInpaintInvocation(BaseInvocation):
|
||||
"""Simple inpaint using opencv."""
|
||||
|
||||
|
@ -16,7 +16,7 @@ from ..models.image import ImageCategory, ResourceOrigin
|
||||
from .baseinvocation import BaseInvocation, FieldDescriptions, InputField, InvocationContext, invocation
|
||||
|
||||
|
||||
@invocation("show_image", title="Show Image", tags=["image"], category="image")
|
||||
@invocation("show_image", title="Show Image", tags=["image"], category="image", version="1.0.0")
|
||||
class ShowImageInvocation(BaseInvocation):
|
||||
"""Displays a provided image using the OS image viewer, and passes it forward in the pipeline."""
|
||||
|
||||
@ -36,7 +36,7 @@ class ShowImageInvocation(BaseInvocation):
|
||||
)
|
||||
|
||||
|
||||
@invocation("blank_image", title="Blank Image", tags=["image"], category="image")
|
||||
@invocation("blank_image", title="Blank Image", tags=["image"], category="image", version="1.0.0")
|
||||
class BlankImageInvocation(BaseInvocation):
|
||||
"""Creates a blank image and forwards it to the pipeline"""
|
||||
|
||||
@ -65,7 +65,7 @@ class BlankImageInvocation(BaseInvocation):
|
||||
)
|
||||
|
||||
|
||||
@invocation("img_crop", title="Crop Image", tags=["image", "crop"], category="image")
|
||||
@invocation("img_crop", title="Crop Image", tags=["image", "crop"], category="image", version="1.0.0")
|
||||
class ImageCropInvocation(BaseInvocation):
|
||||
"""Crops an image to a specified box. The box can be outside of the image."""
|
||||
|
||||
@ -98,7 +98,7 @@ class ImageCropInvocation(BaseInvocation):
|
||||
)
|
||||
|
||||
|
||||
@invocation("img_paste", title="Paste Image", tags=["image", "paste"], category="image")
|
||||
@invocation("img_paste", title="Paste Image", tags=["image", "paste"], category="image", version="1.0.0")
|
||||
class ImagePasteInvocation(BaseInvocation):
|
||||
"""Pastes an image into another image."""
|
||||
|
||||
@ -146,7 +146,7 @@ class ImagePasteInvocation(BaseInvocation):
|
||||
)
|
||||
|
||||
|
||||
@invocation("tomask", title="Mask from Alpha", tags=["image", "mask"], category="image")
|
||||
@invocation("tomask", title="Mask from Alpha", tags=["image", "mask"], category="image", version="1.0.0")
|
||||
class MaskFromAlphaInvocation(BaseInvocation):
|
||||
"""Extracts the alpha channel of an image as a mask."""
|
||||
|
||||
@ -177,7 +177,7 @@ class MaskFromAlphaInvocation(BaseInvocation):
|
||||
)
|
||||
|
||||
|
||||
@invocation("img_mul", title="Multiply Images", tags=["image", "multiply"], category="image")
|
||||
@invocation("img_mul", title="Multiply Images", tags=["image", "multiply"], category="image", version="1.0.0")
|
||||
class ImageMultiplyInvocation(BaseInvocation):
|
||||
"""Multiplies two images together using `PIL.ImageChops.multiply()`."""
|
||||
|
||||
@ -210,7 +210,7 @@ class ImageMultiplyInvocation(BaseInvocation):
|
||||
IMAGE_CHANNELS = Literal["A", "R", "G", "B"]
|
||||
|
||||
|
||||
@invocation("img_chan", title="Extract Image Channel", tags=["image", "channel"], category="image")
|
||||
@invocation("img_chan", title="Extract Image Channel", tags=["image", "channel"], category="image", version="1.0.0")
|
||||
class ImageChannelInvocation(BaseInvocation):
|
||||
"""Gets a channel from an image."""
|
||||
|
||||
@ -242,7 +242,7 @@ class ImageChannelInvocation(BaseInvocation):
|
||||
IMAGE_MODES = Literal["L", "RGB", "RGBA", "CMYK", "YCbCr", "LAB", "HSV", "I", "F"]
|
||||
|
||||
|
||||
@invocation("img_conv", title="Convert Image Mode", tags=["image", "convert"], category="image")
|
||||
@invocation("img_conv", title="Convert Image Mode", tags=["image", "convert"], category="image", version="1.0.0")
|
||||
class ImageConvertInvocation(BaseInvocation):
|
||||
"""Converts an image to a different mode."""
|
||||
|
||||
@ -271,7 +271,7 @@ class ImageConvertInvocation(BaseInvocation):
|
||||
)
|
||||
|
||||
|
||||
@invocation("img_blur", title="Blur Image", tags=["image", "blur"], category="image")
|
||||
@invocation("img_blur", title="Blur Image", tags=["image", "blur"], category="image", version="1.0.0")
|
||||
class ImageBlurInvocation(BaseInvocation):
|
||||
"""Blurs an image"""
|
||||
|
||||
@ -325,7 +325,7 @@ PIL_RESAMPLING_MAP = {
|
||||
}
|
||||
|
||||
|
||||
@invocation("img_resize", title="Resize Image", tags=["image", "resize"], category="image")
|
||||
@invocation("img_resize", title="Resize Image", tags=["image", "resize"], category="image", version="1.0.0")
|
||||
class ImageResizeInvocation(BaseInvocation):
|
||||
"""Resizes an image to specific dimensions"""
|
||||
|
||||
@ -365,7 +365,7 @@ class ImageResizeInvocation(BaseInvocation):
|
||||
)
|
||||
|
||||
|
||||
@invocation("img_scale", title="Scale Image", tags=["image", "scale"], category="image")
|
||||
@invocation("img_scale", title="Scale Image", tags=["image", "scale"], category="image", version="1.0.0")
|
||||
class ImageScaleInvocation(BaseInvocation):
|
||||
"""Scales an image by a factor"""
|
||||
|
||||
@ -406,7 +406,7 @@ class ImageScaleInvocation(BaseInvocation):
|
||||
)
|
||||
|
||||
|
||||
@invocation("img_lerp", title="Lerp Image", tags=["image", "lerp"], category="image")
|
||||
@invocation("img_lerp", title="Lerp Image", tags=["image", "lerp"], category="image", version="1.0.0")
|
||||
class ImageLerpInvocation(BaseInvocation):
|
||||
"""Linear interpolation of all pixels of an image"""
|
||||
|
||||
@ -439,7 +439,7 @@ class ImageLerpInvocation(BaseInvocation):
|
||||
)
|
||||
|
||||
|
||||
@invocation("img_ilerp", title="Inverse Lerp Image", tags=["image", "ilerp"], category="image")
|
||||
@invocation("img_ilerp", title="Inverse Lerp Image", tags=["image", "ilerp"], category="image", version="1.0.0")
|
||||
class ImageInverseLerpInvocation(BaseInvocation):
|
||||
"""Inverse linear interpolation of all pixels of an image"""
|
||||
|
||||
@ -472,7 +472,7 @@ class ImageInverseLerpInvocation(BaseInvocation):
|
||||
)
|
||||
|
||||
|
||||
@invocation("img_nsfw", title="Blur NSFW Image", tags=["image", "nsfw"], category="image")
|
||||
@invocation("img_nsfw", title="Blur NSFW Image", tags=["image", "nsfw"], category="image", version="1.0.0")
|
||||
class ImageNSFWBlurInvocation(BaseInvocation):
|
||||
"""Add blur to NSFW-flagged images"""
|
||||
|
||||
@ -517,7 +517,9 @@ class ImageNSFWBlurInvocation(BaseInvocation):
|
||||
return caution.resize((caution.width // 2, caution.height // 2))
|
||||
|
||||
|
||||
@invocation("img_watermark", title="Add Invisible Watermark", tags=["image", "watermark"], category="image")
|
||||
@invocation(
|
||||
"img_watermark", title="Add Invisible Watermark", tags=["image", "watermark"], category="image", version="1.0.0"
|
||||
)
|
||||
class ImageWatermarkInvocation(BaseInvocation):
|
||||
"""Add an invisible watermark to an image"""
|
||||
|
||||
@ -548,7 +550,7 @@ class ImageWatermarkInvocation(BaseInvocation):
|
||||
)
|
||||
|
||||
|
||||
@invocation("mask_edge", title="Mask Edge", tags=["image", "mask", "inpaint"], category="image")
|
||||
@invocation("mask_edge", title="Mask Edge", tags=["image", "mask", "inpaint"], category="image", version="1.0.0")
|
||||
class MaskEdgeInvocation(BaseInvocation):
|
||||
"""Applies an edge mask to an image"""
|
||||
|
||||
@ -561,7 +563,7 @@ class MaskEdgeInvocation(BaseInvocation):
|
||||
)
|
||||
|
||||
def invoke(self, context: InvocationContext) -> ImageOutput:
|
||||
mask = context.services.images.get_pil_image(self.image.image_name)
|
||||
mask = context.services.images.get_pil_image(self.image.image_name).convert("L")
|
||||
|
||||
npimg = numpy.asarray(mask, dtype=numpy.uint8)
|
||||
npgradient = numpy.uint8(255 * (1.0 - numpy.floor(numpy.abs(0.5 - numpy.float32(npimg) / 255.0) * 2.0)))
|
||||
@ -593,7 +595,9 @@ class MaskEdgeInvocation(BaseInvocation):
|
||||
)
|
||||
|
||||
|
||||
@invocation("mask_combine", title="Combine Masks", tags=["image", "mask", "multiply"], category="image")
|
||||
@invocation(
|
||||
"mask_combine", title="Combine Masks", tags=["image", "mask", "multiply"], category="image", version="1.0.0"
|
||||
)
|
||||
class MaskCombineInvocation(BaseInvocation):
|
||||
"""Combine two masks together by multiplying them using `PIL.ImageChops.multiply()`."""
|
||||
|
||||
@ -623,7 +627,7 @@ class MaskCombineInvocation(BaseInvocation):
|
||||
)
|
||||
|
||||
|
||||
@invocation("color_correct", title="Color Correct", tags=["image", "color"], category="image")
|
||||
@invocation("color_correct", title="Color Correct", tags=["image", "color"], category="image", version="1.0.0")
|
||||
class ColorCorrectInvocation(BaseInvocation):
|
||||
"""
|
||||
Shifts the colors of a target image to match the reference image, optionally
|
||||
@ -696,8 +700,13 @@ class ColorCorrectInvocation(BaseInvocation):
|
||||
# Blur the mask out (into init image) by specified amount
|
||||
if self.mask_blur_radius > 0:
|
||||
nm = numpy.asarray(pil_init_mask, dtype=numpy.uint8)
|
||||
inverted_nm = 255 - nm
|
||||
dilation_size = int(round(self.mask_blur_radius) + 20)
|
||||
dilating_kernel = cv2.getStructuringElement(cv2.MORPH_ELLIPSE, (dilation_size, dilation_size))
|
||||
inverted_dilated_nm = cv2.dilate(inverted_nm, dilating_kernel)
|
||||
dilated_nm = 255 - inverted_dilated_nm
|
||||
nmd = cv2.erode(
|
||||
nm,
|
||||
dilated_nm,
|
||||
kernel=numpy.ones((3, 3), dtype=numpy.uint8),
|
||||
iterations=int(self.mask_blur_radius / 2),
|
||||
)
|
||||
@ -728,7 +737,7 @@ class ColorCorrectInvocation(BaseInvocation):
|
||||
)
|
||||
|
||||
|
||||
@invocation("img_hue_adjust", title="Adjust Image Hue", tags=["image", "hue"], category="image")
|
||||
@invocation("img_hue_adjust", title="Adjust Image Hue", tags=["image", "hue"], category="image", version="1.0.0")
|
||||
class ImageHueAdjustmentInvocation(BaseInvocation):
|
||||
"""Adjusts the Hue of an image."""
|
||||
|
||||
@ -769,38 +778,95 @@ class ImageHueAdjustmentInvocation(BaseInvocation):
|
||||
)
|
||||
|
||||
|
||||
COLOR_CHANNELS = Literal[
|
||||
"Red (RGBA)",
|
||||
"Green (RGBA)",
|
||||
"Blue (RGBA)",
|
||||
"Alpha (RGBA)",
|
||||
"Cyan (CMYK)",
|
||||
"Magenta (CMYK)",
|
||||
"Yellow (CMYK)",
|
||||
"Black (CMYK)",
|
||||
"Hue (HSV)",
|
||||
"Saturation (HSV)",
|
||||
"Value (HSV)",
|
||||
"Luminosity (LAB)",
|
||||
"A (LAB)",
|
||||
"B (LAB)",
|
||||
"Y (YCbCr)",
|
||||
"Cb (YCbCr)",
|
||||
"Cr (YCbCr)",
|
||||
]
|
||||
|
||||
CHANNEL_FORMATS = {
|
||||
"Red (RGBA)": ("RGBA", 0),
|
||||
"Green (RGBA)": ("RGBA", 1),
|
||||
"Blue (RGBA)": ("RGBA", 2),
|
||||
"Alpha (RGBA)": ("RGBA", 3),
|
||||
"Cyan (CMYK)": ("CMYK", 0),
|
||||
"Magenta (CMYK)": ("CMYK", 1),
|
||||
"Yellow (CMYK)": ("CMYK", 2),
|
||||
"Black (CMYK)": ("CMYK", 3),
|
||||
"Hue (HSV)": ("HSV", 0),
|
||||
"Saturation (HSV)": ("HSV", 1),
|
||||
"Value (HSV)": ("HSV", 2),
|
||||
"Luminosity (LAB)": ("LAB", 0),
|
||||
"A (LAB)": ("LAB", 1),
|
||||
"B (LAB)": ("LAB", 2),
|
||||
"Y (YCbCr)": ("YCbCr", 0),
|
||||
"Cb (YCbCr)": ("YCbCr", 1),
|
||||
"Cr (YCbCr)": ("YCbCr", 2),
|
||||
}
|
||||
|
||||
|
||||
@invocation(
|
||||
"img_luminosity_adjust",
|
||||
title="Adjust Image Luminosity",
|
||||
tags=["image", "luminosity", "hsl"],
|
||||
"img_channel_offset",
|
||||
title="Offset Image Channel",
|
||||
tags=[
|
||||
"image",
|
||||
"offset",
|
||||
"red",
|
||||
"green",
|
||||
"blue",
|
||||
"alpha",
|
||||
"cyan",
|
||||
"magenta",
|
||||
"yellow",
|
||||
"black",
|
||||
"hue",
|
||||
"saturation",
|
||||
"luminosity",
|
||||
"value",
|
||||
],
|
||||
category="image",
|
||||
version="1.0.0",
|
||||
)
|
||||
class ImageLuminosityAdjustmentInvocation(BaseInvocation):
|
||||
"""Adjusts the Luminosity (Value) of an image."""
|
||||
class ImageChannelOffsetInvocation(BaseInvocation):
|
||||
"""Add or subtract a value from a specific color channel of an image."""
|
||||
|
||||
image: ImageField = InputField(description="The image to adjust")
|
||||
luminosity: float = InputField(
|
||||
default=1.0, ge=0, le=1, description="The factor by which to adjust the luminosity (value)"
|
||||
)
|
||||
channel: COLOR_CHANNELS = InputField(description="Which channel to adjust")
|
||||
offset: int = InputField(default=0, ge=-255, le=255, description="The amount to adjust the channel by")
|
||||
|
||||
def invoke(self, context: InvocationContext) -> ImageOutput:
|
||||
pil_image = context.services.images.get_pil_image(self.image.image_name)
|
||||
|
||||
# Convert PIL image to OpenCV format (numpy array), note color channel
|
||||
# ordering is changed from RGB to BGR
|
||||
image = numpy.array(pil_image.convert("RGB"))[:, :, ::-1]
|
||||
# extract the channel and mode from the input and reference tuple
|
||||
mode = CHANNEL_FORMATS[self.channel][0]
|
||||
channel_number = CHANNEL_FORMATS[self.channel][1]
|
||||
|
||||
# Convert image to HSV color space
|
||||
hsv_image = cv2.cvtColor(image, cv2.COLOR_BGR2HSV)
|
||||
# Convert PIL image to new format
|
||||
converted_image = numpy.array(pil_image.convert(mode)).astype(int)
|
||||
image_channel = converted_image[:, :, channel_number]
|
||||
|
||||
# Adjust the luminosity (value)
|
||||
hsv_image[:, :, 2] = numpy.clip(hsv_image[:, :, 2] * self.luminosity, 0, 255)
|
||||
# Adjust the value, clipping to 0..255
|
||||
image_channel = numpy.clip(image_channel + self.offset, 0, 255)
|
||||
|
||||
# Convert image back to BGR color space
|
||||
image = cv2.cvtColor(hsv_image, cv2.COLOR_HSV2BGR)
|
||||
# Put the channel back into the image
|
||||
converted_image[:, :, channel_number] = image_channel
|
||||
|
||||
# Convert back to PIL format and to original color mode
|
||||
pil_image = Image.fromarray(image[:, :, ::-1], "RGB").convert("RGBA")
|
||||
# Convert back to RGBA format and output
|
||||
pil_image = Image.fromarray(converted_image.astype(numpy.uint8), mode=mode).convert("RGBA")
|
||||
|
||||
image_dto = context.services.images.create(
|
||||
image=pil_image,
|
||||
@ -822,35 +888,60 @@ class ImageLuminosityAdjustmentInvocation(BaseInvocation):
|
||||
|
||||
|
||||
@invocation(
|
||||
"img_saturation_adjust",
|
||||
title="Adjust Image Saturation",
|
||||
tags=["image", "saturation", "hsl"],
|
||||
"img_channel_multiply",
|
||||
title="Multiply Image Channel",
|
||||
tags=[
|
||||
"image",
|
||||
"invert",
|
||||
"scale",
|
||||
"multiply",
|
||||
"red",
|
||||
"green",
|
||||
"blue",
|
||||
"alpha",
|
||||
"cyan",
|
||||
"magenta",
|
||||
"yellow",
|
||||
"black",
|
||||
"hue",
|
||||
"saturation",
|
||||
"luminosity",
|
||||
"value",
|
||||
],
|
||||
category="image",
|
||||
version="1.0.0",
|
||||
)
|
||||
class ImageSaturationAdjustmentInvocation(BaseInvocation):
|
||||
"""Adjusts the Saturation of an image."""
|
||||
class ImageChannelMultiplyInvocation(BaseInvocation):
|
||||
"""Scale a specific color channel of an image."""
|
||||
|
||||
image: ImageField = InputField(description="The image to adjust")
|
||||
saturation: float = InputField(default=1.0, ge=0, le=1, description="The factor by which to adjust the saturation")
|
||||
channel: COLOR_CHANNELS = InputField(description="Which channel to adjust")
|
||||
scale: float = InputField(default=1.0, ge=0.0, description="The amount to scale the channel by.")
|
||||
invert_channel: bool = InputField(default=False, description="Invert the channel after scaling")
|
||||
|
||||
def invoke(self, context: InvocationContext) -> ImageOutput:
|
||||
pil_image = context.services.images.get_pil_image(self.image.image_name)
|
||||
|
||||
# Convert PIL image to OpenCV format (numpy array), note color channel
|
||||
# ordering is changed from RGB to BGR
|
||||
image = numpy.array(pil_image.convert("RGB"))[:, :, ::-1]
|
||||
# extract the channel and mode from the input and reference tuple
|
||||
mode = CHANNEL_FORMATS[self.channel][0]
|
||||
channel_number = CHANNEL_FORMATS[self.channel][1]
|
||||
|
||||
# Convert image to HSV color space
|
||||
hsv_image = cv2.cvtColor(image, cv2.COLOR_BGR2HSV)
|
||||
# Convert PIL image to new format
|
||||
converted_image = numpy.array(pil_image.convert(mode)).astype(float)
|
||||
image_channel = converted_image[:, :, channel_number]
|
||||
|
||||
# Adjust the saturation
|
||||
hsv_image[:, :, 1] = numpy.clip(hsv_image[:, :, 1] * self.saturation, 0, 255)
|
||||
# Adjust the value, clipping to 0..255
|
||||
image_channel = numpy.clip(image_channel * self.scale, 0, 255)
|
||||
|
||||
# Convert image back to BGR color space
|
||||
image = cv2.cvtColor(hsv_image, cv2.COLOR_HSV2BGR)
|
||||
# Invert the channel if requested
|
||||
if self.invert_channel:
|
||||
image_channel = 255 - image_channel
|
||||
|
||||
# Convert back to PIL format and to original color mode
|
||||
pil_image = Image.fromarray(image[:, :, ::-1], "RGB").convert("RGBA")
|
||||
# Put the channel back into the image
|
||||
converted_image[:, :, channel_number] = image_channel
|
||||
|
||||
# Convert back to RGBA format and output
|
||||
pil_image = Image.fromarray(converted_image.astype(numpy.uint8), mode=mode).convert("RGBA")
|
||||
|
||||
image_dto = context.services.images.create(
|
||||
image=pil_image,
|
||||
|
@ -8,19 +8,17 @@ from PIL import Image, ImageOps
|
||||
|
||||
from invokeai.app.invocations.primitives import ColorField, ImageField, ImageOutput
|
||||
from invokeai.app.util.misc import SEED_MAX, get_random_seed
|
||||
from invokeai.backend.image_util.cv2_inpaint import cv2_inpaint
|
||||
from invokeai.backend.image_util.lama import LaMA
|
||||
from invokeai.backend.image_util.patchmatch import PatchMatch
|
||||
|
||||
from ..models.image import ImageCategory, ResourceOrigin
|
||||
from .baseinvocation import BaseInvocation, InputField, InvocationContext, invocation
|
||||
from .image import PIL_RESAMPLING_MAP, PIL_RESAMPLING_MODES
|
||||
|
||||
|
||||
def infill_methods() -> list[str]:
|
||||
methods = [
|
||||
"tile",
|
||||
"solid",
|
||||
"lama",
|
||||
]
|
||||
methods = ["tile", "solid", "lama", "cv2"]
|
||||
if PatchMatch.patchmatch_available():
|
||||
methods.insert(0, "patchmatch")
|
||||
return methods
|
||||
@ -49,6 +47,10 @@ def infill_patchmatch(im: Image.Image) -> Image.Image:
|
||||
return im_patched
|
||||
|
||||
|
||||
def infill_cv2(im: Image.Image) -> Image.Image:
|
||||
return cv2_inpaint(im)
|
||||
|
||||
|
||||
def get_tile_images(image: np.ndarray, width=8, height=8):
|
||||
_nrows, _ncols, depth = image.shape
|
||||
_strides = image.strides
|
||||
@ -116,7 +118,7 @@ def tile_fill_missing(im: Image.Image, tile_size: int = 16, seed: Optional[int]
|
||||
return si
|
||||
|
||||
|
||||
@invocation("infill_rgba", title="Solid Color Infill", tags=["image", "inpaint"], category="inpaint")
|
||||
@invocation("infill_rgba", title="Solid Color Infill", tags=["image", "inpaint"], category="inpaint", version="1.0.0")
|
||||
class InfillColorInvocation(BaseInvocation):
|
||||
"""Infills transparent areas of an image with a solid color"""
|
||||
|
||||
@ -151,7 +153,7 @@ class InfillColorInvocation(BaseInvocation):
|
||||
)
|
||||
|
||||
|
||||
@invocation("infill_tile", title="Tile Infill", tags=["image", "inpaint"], category="inpaint")
|
||||
@invocation("infill_tile", title="Tile Infill", tags=["image", "inpaint"], category="inpaint", version="1.0.0")
|
||||
class InfillTileInvocation(BaseInvocation):
|
||||
"""Infills transparent areas of an image with tiles of the image"""
|
||||
|
||||
@ -187,20 +189,42 @@ class InfillTileInvocation(BaseInvocation):
|
||||
)
|
||||
|
||||
|
||||
@invocation("infill_patchmatch", title="PatchMatch Infill", tags=["image", "inpaint"], category="inpaint")
|
||||
@invocation(
|
||||
"infill_patchmatch", title="PatchMatch Infill", tags=["image", "inpaint"], category="inpaint", version="1.0.0"
|
||||
)
|
||||
class InfillPatchMatchInvocation(BaseInvocation):
|
||||
"""Infills transparent areas of an image using the PatchMatch algorithm"""
|
||||
|
||||
image: ImageField = InputField(description="The image to infill")
|
||||
downscale: float = InputField(default=2.0, gt=0, description="Run patchmatch on downscaled image to speedup infill")
|
||||
resample_mode: PIL_RESAMPLING_MODES = InputField(default="bicubic", description="The resampling mode")
|
||||
|
||||
def invoke(self, context: InvocationContext) -> ImageOutput:
|
||||
image = context.services.images.get_pil_image(self.image.image_name)
|
||||
image = context.services.images.get_pil_image(self.image.image_name).convert("RGBA")
|
||||
|
||||
resample_mode = PIL_RESAMPLING_MAP[self.resample_mode]
|
||||
|
||||
infill_image = image.copy()
|
||||
width = int(image.width / self.downscale)
|
||||
height = int(image.height / self.downscale)
|
||||
infill_image = infill_image.resize(
|
||||
(width, height),
|
||||
resample=resample_mode,
|
||||
)
|
||||
|
||||
if PatchMatch.patchmatch_available():
|
||||
infilled = infill_patchmatch(image.copy())
|
||||
infilled = infill_patchmatch(infill_image)
|
||||
else:
|
||||
raise ValueError("PatchMatch is not available on this system")
|
||||
|
||||
infilled = infilled.resize(
|
||||
(image.width, image.height),
|
||||
resample=resample_mode,
|
||||
)
|
||||
|
||||
infilled.paste(image, (0, 0), mask=image.split()[-1])
|
||||
# image.paste(infilled, (0, 0), mask=image.split()[-1])
|
||||
|
||||
image_dto = context.services.images.create(
|
||||
image=infilled,
|
||||
image_origin=ResourceOrigin.INTERNAL,
|
||||
@ -218,7 +242,7 @@ class InfillPatchMatchInvocation(BaseInvocation):
|
||||
)
|
||||
|
||||
|
||||
@invocation("infill_lama", title="LaMa Infill", tags=["image", "inpaint"], category="inpaint")
|
||||
@invocation("infill_lama", title="LaMa Infill", tags=["image", "inpaint"], category="inpaint", version="1.0.0")
|
||||
class LaMaInfillInvocation(BaseInvocation):
|
||||
"""Infills transparent areas of an image using the LaMa model"""
|
||||
|
||||
@ -243,3 +267,30 @@ class LaMaInfillInvocation(BaseInvocation):
|
||||
width=image_dto.width,
|
||||
height=image_dto.height,
|
||||
)
|
||||
|
||||
|
||||
@invocation("infill_cv2", title="CV2 Infill", tags=["image", "inpaint"], category="inpaint")
|
||||
class CV2InfillInvocation(BaseInvocation):
|
||||
"""Infills transparent areas of an image using OpenCV Inpainting"""
|
||||
|
||||
image: ImageField = InputField(description="The image to infill")
|
||||
|
||||
def invoke(self, context: InvocationContext) -> ImageOutput:
|
||||
image = context.services.images.get_pil_image(self.image.image_name)
|
||||
|
||||
infilled = infill_cv2(image.copy())
|
||||
|
||||
image_dto = context.services.images.create(
|
||||
image=infilled,
|
||||
image_origin=ResourceOrigin.INTERNAL,
|
||||
image_category=ImageCategory.GENERAL,
|
||||
node_id=self.id,
|
||||
session_id=context.graph_execution_state_id,
|
||||
is_intermediate=self.is_intermediate,
|
||||
)
|
||||
|
||||
return ImageOutput(
|
||||
image=ImageField(image_name=image_dto.image_name),
|
||||
width=image_dto.width,
|
||||
height=image_dto.height,
|
||||
)
|
||||
|
@ -74,7 +74,7 @@ class SchedulerOutput(BaseInvocationOutput):
|
||||
scheduler: SAMPLER_NAME_VALUES = OutputField(description=FieldDescriptions.scheduler, ui_type=UIType.Scheduler)
|
||||
|
||||
|
||||
@invocation("scheduler", title="Scheduler", tags=["scheduler"], category="latents")
|
||||
@invocation("scheduler", title="Scheduler", tags=["scheduler"], category="latents", version="1.0.0")
|
||||
class SchedulerInvocation(BaseInvocation):
|
||||
"""Selects a scheduler."""
|
||||
|
||||
@ -86,7 +86,9 @@ class SchedulerInvocation(BaseInvocation):
|
||||
return SchedulerOutput(scheduler=self.scheduler)
|
||||
|
||||
|
||||
@invocation("create_denoise_mask", title="Create Denoise Mask", tags=["mask", "denoise"], category="latents")
|
||||
@invocation(
|
||||
"create_denoise_mask", title="Create Denoise Mask", tags=["mask", "denoise"], category="latents", version="1.0.0"
|
||||
)
|
||||
class CreateDenoiseMaskInvocation(BaseInvocation):
|
||||
"""Creates mask for denoising model run."""
|
||||
|
||||
@ -186,6 +188,7 @@ def get_scheduler(
|
||||
title="Denoise Latents",
|
||||
tags=["latents", "denoise", "txt2img", "t2i", "t2l", "img2img", "i2i", "l2l"],
|
||||
category="latents",
|
||||
version="1.0.0",
|
||||
)
|
||||
class DenoiseLatentsInvocation(BaseInvocation):
|
||||
"""Denoises noisy latents to decodable images"""
|
||||
@ -208,12 +211,14 @@ class DenoiseLatentsInvocation(BaseInvocation):
|
||||
)
|
||||
unet: UNetField = InputField(description=FieldDescriptions.unet, input=Input.Connection, title="UNet", ui_order=2)
|
||||
control: Union[ControlField, list[ControlField]] = InputField(
|
||||
default=None, description=FieldDescriptions.control, input=Input.Connection, ui_order=5
|
||||
default=None,
|
||||
description=FieldDescriptions.control,
|
||||
input=Input.Connection,
|
||||
ui_order=5,
|
||||
)
|
||||
latents: Optional[LatentsField] = InputField(description=FieldDescriptions.latents, input=Input.Connection)
|
||||
denoise_mask: Optional[DenoiseMaskField] = InputField(
|
||||
default=None,
|
||||
description=FieldDescriptions.mask,
|
||||
default=None, description=FieldDescriptions.mask, input=Input.Connection, ui_order=6
|
||||
)
|
||||
|
||||
@validator("cfg_scale")
|
||||
@ -317,7 +322,7 @@ class DenoiseLatentsInvocation(BaseInvocation):
|
||||
context: InvocationContext,
|
||||
# really only need model for dtype and device
|
||||
model: StableDiffusionGeneratorPipeline,
|
||||
control_input: List[ControlField],
|
||||
control_input: Union[ControlField, List[ControlField]],
|
||||
latents_shape: List[int],
|
||||
exit_stack: ExitStack,
|
||||
do_classifier_free_guidance: bool = True,
|
||||
@ -542,7 +547,9 @@ class DenoiseLatentsInvocation(BaseInvocation):
|
||||
return build_latents_output(latents_name=name, latents=result_latents, seed=seed)
|
||||
|
||||
|
||||
@invocation("l2i", title="Latents to Image", tags=["latents", "image", "vae", "l2i"], category="latents")
|
||||
@invocation(
|
||||
"l2i", title="Latents to Image", tags=["latents", "image", "vae", "l2i"], category="latents", version="1.0.0"
|
||||
)
|
||||
class LatentsToImageInvocation(BaseInvocation):
|
||||
"""Generates an image from latents."""
|
||||
|
||||
@ -639,7 +646,7 @@ class LatentsToImageInvocation(BaseInvocation):
|
||||
LATENTS_INTERPOLATION_MODE = Literal["nearest", "linear", "bilinear", "bicubic", "trilinear", "area", "nearest-exact"]
|
||||
|
||||
|
||||
@invocation("lresize", title="Resize Latents", tags=["latents", "resize"], category="latents")
|
||||
@invocation("lresize", title="Resize Latents", tags=["latents", "resize"], category="latents", version="1.0.0")
|
||||
class ResizeLatentsInvocation(BaseInvocation):
|
||||
"""Resizes latents to explicit width/height (in pixels). Provided dimensions are floor-divided by 8."""
|
||||
|
||||
@ -683,7 +690,7 @@ class ResizeLatentsInvocation(BaseInvocation):
|
||||
return build_latents_output(latents_name=name, latents=resized_latents, seed=self.latents.seed)
|
||||
|
||||
|
||||
@invocation("lscale", title="Scale Latents", tags=["latents", "resize"], category="latents")
|
||||
@invocation("lscale", title="Scale Latents", tags=["latents", "resize"], category="latents", version="1.0.0")
|
||||
class ScaleLatentsInvocation(BaseInvocation):
|
||||
"""Scales latents by a given factor."""
|
||||
|
||||
@ -719,7 +726,9 @@ class ScaleLatentsInvocation(BaseInvocation):
|
||||
return build_latents_output(latents_name=name, latents=resized_latents, seed=self.latents.seed)
|
||||
|
||||
|
||||
@invocation("i2l", title="Image to Latents", tags=["latents", "image", "vae", "i2l"], category="latents")
|
||||
@invocation(
|
||||
"i2l", title="Image to Latents", tags=["latents", "image", "vae", "i2l"], category="latents", version="1.0.0"
|
||||
)
|
||||
class ImageToLatentsInvocation(BaseInvocation):
|
||||
"""Encodes an image into latents."""
|
||||
|
||||
@ -799,7 +808,7 @@ class ImageToLatentsInvocation(BaseInvocation):
|
||||
return build_latents_output(latents_name=name, latents=latents, seed=None)
|
||||
|
||||
|
||||
@invocation("lblend", title="Blend Latents", tags=["latents", "blend"], category="latents")
|
||||
@invocation("lblend", title="Blend Latents", tags=["latents", "blend"], category="latents", version="1.0.0")
|
||||
class BlendLatentsInvocation(BaseInvocation):
|
||||
"""Blend two latents using a given alpha. Latents must have same size."""
|
||||
|
||||
|
@ -7,7 +7,7 @@ from invokeai.app.invocations.primitives import IntegerOutput
|
||||
from .baseinvocation import BaseInvocation, FieldDescriptions, InputField, InvocationContext, invocation
|
||||
|
||||
|
||||
@invocation("add", title="Add Integers", tags=["math", "add"], category="math")
|
||||
@invocation("add", title="Add Integers", tags=["math", "add"], category="math", version="1.0.0")
|
||||
class AddInvocation(BaseInvocation):
|
||||
"""Adds two numbers"""
|
||||
|
||||
@ -18,7 +18,7 @@ class AddInvocation(BaseInvocation):
|
||||
return IntegerOutput(value=self.a + self.b)
|
||||
|
||||
|
||||
@invocation("sub", title="Subtract Integers", tags=["math", "subtract"], category="math")
|
||||
@invocation("sub", title="Subtract Integers", tags=["math", "subtract"], category="math", version="1.0.0")
|
||||
class SubtractInvocation(BaseInvocation):
|
||||
"""Subtracts two numbers"""
|
||||
|
||||
@ -29,7 +29,7 @@ class SubtractInvocation(BaseInvocation):
|
||||
return IntegerOutput(value=self.a - self.b)
|
||||
|
||||
|
||||
@invocation("mul", title="Multiply Integers", tags=["math", "multiply"], category="math")
|
||||
@invocation("mul", title="Multiply Integers", tags=["math", "multiply"], category="math", version="1.0.0")
|
||||
class MultiplyInvocation(BaseInvocation):
|
||||
"""Multiplies two numbers"""
|
||||
|
||||
@ -40,7 +40,7 @@ class MultiplyInvocation(BaseInvocation):
|
||||
return IntegerOutput(value=self.a * self.b)
|
||||
|
||||
|
||||
@invocation("div", title="Divide Integers", tags=["math", "divide"], category="math")
|
||||
@invocation("div", title="Divide Integers", tags=["math", "divide"], category="math", version="1.0.0")
|
||||
class DivideInvocation(BaseInvocation):
|
||||
"""Divides two numbers"""
|
||||
|
||||
@ -51,7 +51,7 @@ class DivideInvocation(BaseInvocation):
|
||||
return IntegerOutput(value=int(self.a / self.b))
|
||||
|
||||
|
||||
@invocation("rand_int", title="Random Integer", tags=["math", "random"], category="math")
|
||||
@invocation("rand_int", title="Random Integer", tags=["math", "random"], category="math", version="1.0.0")
|
||||
class RandomIntInvocation(BaseInvocation):
|
||||
"""Outputs a single random integer."""
|
||||
|
||||
|
@ -98,7 +98,9 @@ class MetadataAccumulatorOutput(BaseInvocationOutput):
|
||||
metadata: CoreMetadata = OutputField(description="The core metadata for the image")
|
||||
|
||||
|
||||
@invocation("metadata_accumulator", title="Metadata Accumulator", tags=["metadata"], category="metadata")
|
||||
@invocation(
|
||||
"metadata_accumulator", title="Metadata Accumulator", tags=["metadata"], category="metadata", version="1.0.0"
|
||||
)
|
||||
class MetadataAccumulatorInvocation(BaseInvocation):
|
||||
"""Outputs a Core Metadata Object"""
|
||||
|
||||
|
@ -73,7 +73,7 @@ class LoRAModelField(BaseModel):
|
||||
base_model: BaseModelType = Field(description="Base model")
|
||||
|
||||
|
||||
@invocation("main_model_loader", title="Main Model", tags=["model"], category="model")
|
||||
@invocation("main_model_loader", title="Main Model", tags=["model"], category="model", version="1.0.0")
|
||||
class MainModelLoaderInvocation(BaseInvocation):
|
||||
"""Loads a main model, outputting its submodels."""
|
||||
|
||||
@ -173,7 +173,7 @@ class LoraLoaderOutput(BaseInvocationOutput):
|
||||
clip: Optional[ClipField] = OutputField(default=None, description=FieldDescriptions.clip, title="CLIP")
|
||||
|
||||
|
||||
@invocation("lora_loader", title="LoRA", tags=["model"], category="model")
|
||||
@invocation("lora_loader", title="LoRA", tags=["model"], category="model", version="1.0.0")
|
||||
class LoraLoaderInvocation(BaseInvocation):
|
||||
"""Apply selected lora to unet and text_encoder."""
|
||||
|
||||
@ -244,7 +244,7 @@ class SDXLLoraLoaderOutput(BaseInvocationOutput):
|
||||
clip2: Optional[ClipField] = OutputField(default=None, description=FieldDescriptions.clip, title="CLIP 2")
|
||||
|
||||
|
||||
@invocation("sdxl_lora_loader", title="SDXL LoRA", tags=["lora", "model"], category="model")
|
||||
@invocation("sdxl_lora_loader", title="SDXL LoRA", tags=["lora", "model"], category="model", version="1.0.0")
|
||||
class SDXLLoraLoaderInvocation(BaseInvocation):
|
||||
"""Apply selected lora to unet and text_encoder."""
|
||||
|
||||
@ -338,7 +338,7 @@ class VaeLoaderOutput(BaseInvocationOutput):
|
||||
vae: VaeField = OutputField(description=FieldDescriptions.vae, title="VAE")
|
||||
|
||||
|
||||
@invocation("vae_loader", title="VAE", tags=["vae", "model"], category="model")
|
||||
@invocation("vae_loader", title="VAE", tags=["vae", "model"], category="model", version="1.0.0")
|
||||
class VaeLoaderInvocation(BaseInvocation):
|
||||
"""Loads a VAE model, outputting a VaeLoaderOutput"""
|
||||
|
||||
@ -376,7 +376,7 @@ class SeamlessModeOutput(BaseInvocationOutput):
|
||||
vae: Optional[VaeField] = OutputField(description=FieldDescriptions.vae, title="VAE")
|
||||
|
||||
|
||||
@invocation("seamless", title="Seamless", tags=["seamless", "model"], category="model")
|
||||
@invocation("seamless", title="Seamless", tags=["seamless", "model"], category="model", version="1.0.0")
|
||||
class SeamlessModeInvocation(BaseInvocation):
|
||||
"""Applies the seamless transformation to the Model UNet and VAE."""
|
||||
|
||||
|
@ -78,7 +78,7 @@ def build_noise_output(latents_name: str, latents: torch.Tensor, seed: int):
|
||||
)
|
||||
|
||||
|
||||
@invocation("noise", title="Noise", tags=["latents", "noise"], category="latents")
|
||||
@invocation("noise", title="Noise", tags=["latents", "noise"], category="latents", version="1.0.0")
|
||||
class NoiseInvocation(BaseInvocation):
|
||||
"""Generates latent noise."""
|
||||
|
||||
|
@ -56,7 +56,7 @@ ORT_TO_NP_TYPE = {
|
||||
PRECISION_VALUES = Literal[tuple(list(ORT_TO_NP_TYPE.keys()))]
|
||||
|
||||
|
||||
@invocation("prompt_onnx", title="ONNX Prompt (Raw)", tags=["prompt", "onnx"], category="conditioning")
|
||||
@invocation("prompt_onnx", title="ONNX Prompt (Raw)", tags=["prompt", "onnx"], category="conditioning", version="1.0.0")
|
||||
class ONNXPromptInvocation(BaseInvocation):
|
||||
prompt: str = InputField(default="", description=FieldDescriptions.raw_prompt, ui_component=UIComponent.Textarea)
|
||||
clip: ClipField = InputField(description=FieldDescriptions.clip, input=Input.Connection)
|
||||
@ -143,6 +143,7 @@ class ONNXPromptInvocation(BaseInvocation):
|
||||
title="ONNX Text to Latents",
|
||||
tags=["latents", "inference", "txt2img", "onnx"],
|
||||
category="latents",
|
||||
version="1.0.0",
|
||||
)
|
||||
class ONNXTextToLatentsInvocation(BaseInvocation):
|
||||
"""Generates latents from conditionings."""
|
||||
@ -319,6 +320,7 @@ class ONNXTextToLatentsInvocation(BaseInvocation):
|
||||
title="ONNX Latents to Image",
|
||||
tags=["latents", "image", "vae", "onnx"],
|
||||
category="image",
|
||||
version="1.0.0",
|
||||
)
|
||||
class ONNXLatentsToImageInvocation(BaseInvocation):
|
||||
"""Generates an image from latents."""
|
||||
@ -403,7 +405,7 @@ class OnnxModelField(BaseModel):
|
||||
model_type: ModelType = Field(description="Model Type")
|
||||
|
||||
|
||||
@invocation("onnx_model_loader", title="ONNX Main Model", tags=["onnx", "model"], category="model")
|
||||
@invocation("onnx_model_loader", title="ONNX Main Model", tags=["onnx", "model"], category="model", version="1.0.0")
|
||||
class OnnxModelLoaderInvocation(BaseInvocation):
|
||||
"""Loads a main model, outputting its submodels."""
|
||||
|
||||
|
@ -45,7 +45,7 @@ from invokeai.app.invocations.primitives import FloatCollectionOutput
|
||||
from .baseinvocation import BaseInvocation, InputField, InvocationContext, invocation
|
||||
|
||||
|
||||
@invocation("float_range", title="Float Range", tags=["math", "range"], category="math")
|
||||
@invocation("float_range", title="Float Range", tags=["math", "range"], category="math", version="1.0.0")
|
||||
class FloatLinearRangeInvocation(BaseInvocation):
|
||||
"""Creates a range"""
|
||||
|
||||
@ -96,7 +96,7 @@ EASING_FUNCTION_KEYS = Literal[tuple(list(EASING_FUNCTIONS_MAP.keys()))]
|
||||
|
||||
|
||||
# actually I think for now could just use CollectionOutput (which is list[Any]
|
||||
@invocation("step_param_easing", title="Step Param Easing", tags=["step", "easing"], category="step")
|
||||
@invocation("step_param_easing", title="Step Param Easing", tags=["step", "easing"], category="step", version="1.0.0")
|
||||
class StepParamEasingInvocation(BaseInvocation):
|
||||
"""Experimental per-step parameter easing for denoising steps"""
|
||||
|
||||
|
@ -14,7 +14,6 @@ from .baseinvocation import (
|
||||
InvocationContext,
|
||||
OutputField,
|
||||
UIComponent,
|
||||
UIType,
|
||||
invocation,
|
||||
invocation_output,
|
||||
)
|
||||
@ -40,10 +39,14 @@ class BooleanOutput(BaseInvocationOutput):
|
||||
class BooleanCollectionOutput(BaseInvocationOutput):
|
||||
"""Base class for nodes that output a collection of booleans"""
|
||||
|
||||
collection: list[bool] = OutputField(description="The output boolean collection", ui_type=UIType.BooleanCollection)
|
||||
collection: list[bool] = OutputField(
|
||||
description="The output boolean collection",
|
||||
)
|
||||
|
||||
|
||||
@invocation("boolean", title="Boolean Primitive", tags=["primitives", "boolean"], category="primitives")
|
||||
@invocation(
|
||||
"boolean", title="Boolean Primitive", tags=["primitives", "boolean"], category="primitives", version="1.0.0"
|
||||
)
|
||||
class BooleanInvocation(BaseInvocation):
|
||||
"""A boolean primitive value"""
|
||||
|
||||
@ -58,13 +61,12 @@ class BooleanInvocation(BaseInvocation):
|
||||
title="Boolean Collection Primitive",
|
||||
tags=["primitives", "boolean", "collection"],
|
||||
category="primitives",
|
||||
version="1.0.0",
|
||||
)
|
||||
class BooleanCollectionInvocation(BaseInvocation):
|
||||
"""A collection of boolean primitive values"""
|
||||
|
||||
collection: list[bool] = InputField(
|
||||
default_factory=list, description="The collection of boolean values", ui_type=UIType.BooleanCollection
|
||||
)
|
||||
collection: list[bool] = InputField(default_factory=list, description="The collection of boolean values")
|
||||
|
||||
def invoke(self, context: InvocationContext) -> BooleanCollectionOutput:
|
||||
return BooleanCollectionOutput(collection=self.collection)
|
||||
@ -86,10 +88,14 @@ class IntegerOutput(BaseInvocationOutput):
|
||||
class IntegerCollectionOutput(BaseInvocationOutput):
|
||||
"""Base class for nodes that output a collection of integers"""
|
||||
|
||||
collection: list[int] = OutputField(description="The int collection", ui_type=UIType.IntegerCollection)
|
||||
collection: list[int] = OutputField(
|
||||
description="The int collection",
|
||||
)
|
||||
|
||||
|
||||
@invocation("integer", title="Integer Primitive", tags=["primitives", "integer"], category="primitives")
|
||||
@invocation(
|
||||
"integer", title="Integer Primitive", tags=["primitives", "integer"], category="primitives", version="1.0.0"
|
||||
)
|
||||
class IntegerInvocation(BaseInvocation):
|
||||
"""An integer primitive value"""
|
||||
|
||||
@ -104,13 +110,12 @@ class IntegerInvocation(BaseInvocation):
|
||||
title="Integer Collection Primitive",
|
||||
tags=["primitives", "integer", "collection"],
|
||||
category="primitives",
|
||||
version="1.0.0",
|
||||
)
|
||||
class IntegerCollectionInvocation(BaseInvocation):
|
||||
"""A collection of integer primitive values"""
|
||||
|
||||
collection: list[int] = InputField(
|
||||
default_factory=list, description="The collection of integer values", ui_type=UIType.IntegerCollection
|
||||
)
|
||||
collection: list[int] = InputField(default_factory=list, description="The collection of integer values")
|
||||
|
||||
def invoke(self, context: InvocationContext) -> IntegerCollectionOutput:
|
||||
return IntegerCollectionOutput(collection=self.collection)
|
||||
@ -132,10 +137,12 @@ class FloatOutput(BaseInvocationOutput):
|
||||
class FloatCollectionOutput(BaseInvocationOutput):
|
||||
"""Base class for nodes that output a collection of floats"""
|
||||
|
||||
collection: list[float] = OutputField(description="The float collection", ui_type=UIType.FloatCollection)
|
||||
collection: list[float] = OutputField(
|
||||
description="The float collection",
|
||||
)
|
||||
|
||||
|
||||
@invocation("float", title="Float Primitive", tags=["primitives", "float"], category="primitives")
|
||||
@invocation("float", title="Float Primitive", tags=["primitives", "float"], category="primitives", version="1.0.0")
|
||||
class FloatInvocation(BaseInvocation):
|
||||
"""A float primitive value"""
|
||||
|
||||
@ -150,13 +157,12 @@ class FloatInvocation(BaseInvocation):
|
||||
title="Float Collection Primitive",
|
||||
tags=["primitives", "float", "collection"],
|
||||
category="primitives",
|
||||
version="1.0.0",
|
||||
)
|
||||
class FloatCollectionInvocation(BaseInvocation):
|
||||
"""A collection of float primitive values"""
|
||||
|
||||
collection: list[float] = InputField(
|
||||
default_factory=list, description="The collection of float values", ui_type=UIType.FloatCollection
|
||||
)
|
||||
collection: list[float] = InputField(default_factory=list, description="The collection of float values")
|
||||
|
||||
def invoke(self, context: InvocationContext) -> FloatCollectionOutput:
|
||||
return FloatCollectionOutput(collection=self.collection)
|
||||
@ -178,10 +184,12 @@ class StringOutput(BaseInvocationOutput):
|
||||
class StringCollectionOutput(BaseInvocationOutput):
|
||||
"""Base class for nodes that output a collection of strings"""
|
||||
|
||||
collection: list[str] = OutputField(description="The output strings", ui_type=UIType.StringCollection)
|
||||
collection: list[str] = OutputField(
|
||||
description="The output strings",
|
||||
)
|
||||
|
||||
|
||||
@invocation("string", title="String Primitive", tags=["primitives", "string"], category="primitives")
|
||||
@invocation("string", title="String Primitive", tags=["primitives", "string"], category="primitives", version="1.0.0")
|
||||
class StringInvocation(BaseInvocation):
|
||||
"""A string primitive value"""
|
||||
|
||||
@ -196,13 +204,12 @@ class StringInvocation(BaseInvocation):
|
||||
title="String Collection Primitive",
|
||||
tags=["primitives", "string", "collection"],
|
||||
category="primitives",
|
||||
version="1.0.0",
|
||||
)
|
||||
class StringCollectionInvocation(BaseInvocation):
|
||||
"""A collection of string primitive values"""
|
||||
|
||||
collection: list[str] = InputField(
|
||||
default_factory=list, description="The collection of string values", ui_type=UIType.StringCollection
|
||||
)
|
||||
collection: list[str] = InputField(default_factory=list, description="The collection of string values")
|
||||
|
||||
def invoke(self, context: InvocationContext) -> StringCollectionOutput:
|
||||
return StringCollectionOutput(collection=self.collection)
|
||||
@ -232,10 +239,12 @@ class ImageOutput(BaseInvocationOutput):
|
||||
class ImageCollectionOutput(BaseInvocationOutput):
|
||||
"""Base class for nodes that output a collection of images"""
|
||||
|
||||
collection: list[ImageField] = OutputField(description="The output images", ui_type=UIType.ImageCollection)
|
||||
collection: list[ImageField] = OutputField(
|
||||
description="The output images",
|
||||
)
|
||||
|
||||
|
||||
@invocation("image", title="Image Primitive", tags=["primitives", "image"], category="primitives")
|
||||
@invocation("image", title="Image Primitive", tags=["primitives", "image"], category="primitives", version="1.0.0")
|
||||
class ImageInvocation(BaseInvocation):
|
||||
"""An image primitive value"""
|
||||
|
||||
@ -256,13 +265,12 @@ class ImageInvocation(BaseInvocation):
|
||||
title="Image Collection Primitive",
|
||||
tags=["primitives", "image", "collection"],
|
||||
category="primitives",
|
||||
version="1.0.0",
|
||||
)
|
||||
class ImageCollectionInvocation(BaseInvocation):
|
||||
"""A collection of image primitive values"""
|
||||
|
||||
collection: list[ImageField] = InputField(
|
||||
default_factory=list, description="The collection of image values", ui_type=UIType.ImageCollection
|
||||
)
|
||||
collection: list[ImageField] = InputField(description="The collection of image values")
|
||||
|
||||
def invoke(self, context: InvocationContext) -> ImageCollectionOutput:
|
||||
return ImageCollectionOutput(collection=self.collection)
|
||||
@ -316,11 +324,12 @@ class LatentsCollectionOutput(BaseInvocationOutput):
|
||||
|
||||
collection: list[LatentsField] = OutputField(
|
||||
description=FieldDescriptions.latents,
|
||||
ui_type=UIType.LatentsCollection,
|
||||
)
|
||||
|
||||
|
||||
@invocation("latents", title="Latents Primitive", tags=["primitives", "latents"], category="primitives")
|
||||
@invocation(
|
||||
"latents", title="Latents Primitive", tags=["primitives", "latents"], category="primitives", version="1.0.0"
|
||||
)
|
||||
class LatentsInvocation(BaseInvocation):
|
||||
"""A latents tensor primitive value"""
|
||||
|
||||
@ -337,12 +346,13 @@ class LatentsInvocation(BaseInvocation):
|
||||
title="Latents Collection Primitive",
|
||||
tags=["primitives", "latents", "collection"],
|
||||
category="primitives",
|
||||
version="1.0.0",
|
||||
)
|
||||
class LatentsCollectionInvocation(BaseInvocation):
|
||||
"""A collection of latents tensor primitive values"""
|
||||
|
||||
collection: list[LatentsField] = InputField(
|
||||
description="The collection of latents tensors", ui_type=UIType.LatentsCollection
|
||||
description="The collection of latents tensors",
|
||||
)
|
||||
|
||||
def invoke(self, context: InvocationContext) -> LatentsCollectionOutput:
|
||||
@ -385,10 +395,12 @@ class ColorOutput(BaseInvocationOutput):
|
||||
class ColorCollectionOutput(BaseInvocationOutput):
|
||||
"""Base class for nodes that output a collection of colors"""
|
||||
|
||||
collection: list[ColorField] = OutputField(description="The output colors", ui_type=UIType.ColorCollection)
|
||||
collection: list[ColorField] = OutputField(
|
||||
description="The output colors",
|
||||
)
|
||||
|
||||
|
||||
@invocation("color", title="Color Primitive", tags=["primitives", "color"], category="primitives")
|
||||
@invocation("color", title="Color Primitive", tags=["primitives", "color"], category="primitives", version="1.0.0")
|
||||
class ColorInvocation(BaseInvocation):
|
||||
"""A color primitive value"""
|
||||
|
||||
@ -422,7 +434,6 @@ class ConditioningCollectionOutput(BaseInvocationOutput):
|
||||
|
||||
collection: list[ConditioningField] = OutputField(
|
||||
description="The output conditioning tensors",
|
||||
ui_type=UIType.ConditioningCollection,
|
||||
)
|
||||
|
||||
|
||||
@ -431,6 +442,7 @@ class ConditioningCollectionOutput(BaseInvocationOutput):
|
||||
title="Conditioning Primitive",
|
||||
tags=["primitives", "conditioning"],
|
||||
category="primitives",
|
||||
version="1.0.0",
|
||||
)
|
||||
class ConditioningInvocation(BaseInvocation):
|
||||
"""A conditioning tensor primitive value"""
|
||||
@ -446,6 +458,7 @@ class ConditioningInvocation(BaseInvocation):
|
||||
title="Conditioning Collection Primitive",
|
||||
tags=["primitives", "conditioning", "collection"],
|
||||
category="primitives",
|
||||
version="1.0.0",
|
||||
)
|
||||
class ConditioningCollectionInvocation(BaseInvocation):
|
||||
"""A collection of conditioning tensor primitive values"""
|
||||
@ -453,7 +466,6 @@ class ConditioningCollectionInvocation(BaseInvocation):
|
||||
collection: list[ConditioningField] = InputField(
|
||||
default_factory=list,
|
||||
description="The collection of conditioning tensors",
|
||||
ui_type=UIType.ConditioningCollection,
|
||||
)
|
||||
|
||||
def invoke(self, context: InvocationContext) -> ConditioningCollectionOutput:
|
||||
|
@ -10,7 +10,7 @@ from invokeai.app.invocations.primitives import StringCollectionOutput
|
||||
from .baseinvocation import BaseInvocation, InputField, InvocationContext, UIComponent, invocation
|
||||
|
||||
|
||||
@invocation("dynamic_prompt", title="Dynamic Prompt", tags=["prompt", "collection"], category="prompt")
|
||||
@invocation("dynamic_prompt", title="Dynamic Prompt", tags=["prompt", "collection"], category="prompt", version="1.0.0")
|
||||
class DynamicPromptInvocation(BaseInvocation):
|
||||
"""Parses a prompt using adieyal/dynamicprompts' random or combinatorial generator"""
|
||||
|
||||
@ -29,7 +29,7 @@ class DynamicPromptInvocation(BaseInvocation):
|
||||
return StringCollectionOutput(collection=prompts)
|
||||
|
||||
|
||||
@invocation("prompt_from_file", title="Prompts from File", tags=["prompt", "file"], category="prompt")
|
||||
@invocation("prompt_from_file", title="Prompts from File", tags=["prompt", "file"], category="prompt", version="1.0.0")
|
||||
class PromptsFromFileInvocation(BaseInvocation):
|
||||
"""Loads prompts from a text file"""
|
||||
|
||||
|
@ -33,7 +33,7 @@ class SDXLRefinerModelLoaderOutput(BaseInvocationOutput):
|
||||
vae: VaeField = OutputField(description=FieldDescriptions.vae, title="VAE")
|
||||
|
||||
|
||||
@invocation("sdxl_model_loader", title="SDXL Main Model", tags=["model", "sdxl"], category="model")
|
||||
@invocation("sdxl_model_loader", title="SDXL Main Model", tags=["model", "sdxl"], category="model", version="1.0.0")
|
||||
class SDXLModelLoaderInvocation(BaseInvocation):
|
||||
"""Loads an sdxl base model, outputting its submodels."""
|
||||
|
||||
@ -119,6 +119,7 @@ class SDXLModelLoaderInvocation(BaseInvocation):
|
||||
title="SDXL Refiner Model",
|
||||
tags=["model", "sdxl", "refiner"],
|
||||
category="model",
|
||||
version="1.0.0",
|
||||
)
|
||||
class SDXLRefinerModelLoaderInvocation(BaseInvocation):
|
||||
"""Loads an sdxl refiner model, outputting its submodels."""
|
||||
|
@ -23,7 +23,7 @@ ESRGAN_MODELS = Literal[
|
||||
]
|
||||
|
||||
|
||||
@invocation("esrgan", title="Upscale (RealESRGAN)", tags=["esrgan", "upscale"], category="esrgan")
|
||||
@invocation("esrgan", title="Upscale (RealESRGAN)", tags=["esrgan", "upscale"], category="esrgan", version="1.0.0")
|
||||
class ESRGANInvocation(BaseInvocation):
|
||||
"""Upscales an image using RealESRGAN."""
|
||||
|
||||
|
@ -112,6 +112,10 @@ def are_connection_types_compatible(from_type: Any, to_type: Any) -> bool:
|
||||
if to_type in get_args(from_type):
|
||||
return True
|
||||
|
||||
# allow int -> float, pydantic will cast for us
|
||||
if from_type is int and to_type is float:
|
||||
return True
|
||||
|
||||
# if not issubclass(from_type, to_type):
|
||||
if not is_union_subtype(from_type, to_type):
|
||||
return False
|
||||
|
20
invokeai/backend/image_util/cv2_inpaint.py
Normal file
20
invokeai/backend/image_util/cv2_inpaint.py
Normal file
@ -0,0 +1,20 @@
|
||||
import cv2
|
||||
import numpy as np
|
||||
from PIL import Image
|
||||
|
||||
|
||||
def cv2_inpaint(image: Image.Image) -> Image.Image:
|
||||
# Prepare Image
|
||||
image_array = np.array(image.convert("RGB"))
|
||||
image_cv = cv2.cvtColor(image_array, cv2.COLOR_RGB2BGR)
|
||||
|
||||
# Prepare Mask From Alpha Channel
|
||||
mask = image.split()[3].convert("RGB")
|
||||
mask_array = np.array(mask)
|
||||
mask_cv = cv2.cvtColor(mask_array, cv2.COLOR_BGR2GRAY)
|
||||
mask_inv = cv2.bitwise_not(mask_cv)
|
||||
|
||||
# Inpaint Image
|
||||
inpainted_result = cv2.inpaint(image_cv, mask_inv, 3, cv2.INPAINT_TELEA)
|
||||
inpainted_image = Image.fromarray(cv2.cvtColor(inpainted_result, cv2.COLOR_BGR2RGB))
|
||||
return inpainted_image
|
@ -5,6 +5,7 @@ import numpy as np
|
||||
import torch
|
||||
from PIL import Image
|
||||
|
||||
import invokeai.backend.util.logging as logger
|
||||
from invokeai.app.services.config import get_invokeai_config
|
||||
from invokeai.backend.util.devices import choose_torch_device
|
||||
|
||||
@ -19,7 +20,7 @@ def norm_img(np_img):
|
||||
|
||||
def load_jit_model(url_or_path, device):
|
||||
model_path = url_or_path
|
||||
print(f"Loading model from: {model_path}")
|
||||
logger.info(f"Loading model from: {model_path}")
|
||||
model = torch.jit.load(model_path, map_location="cpu").to(device)
|
||||
model.eval()
|
||||
return model
|
||||
@ -52,5 +53,6 @@ class LaMA:
|
||||
|
||||
del model
|
||||
gc.collect()
|
||||
torch.cuda.empty_cache()
|
||||
|
||||
return infilled_image
|
||||
|
@ -290,9 +290,20 @@ def download_realesrgan():
|
||||
download_with_progress_bar(model["url"], config.models_path / model["dest"], model["description"])
|
||||
|
||||
|
||||
# ---------------------------------------------
|
||||
def download_lama():
|
||||
logger.info("Installing lama infill model")
|
||||
download_with_progress_bar(
|
||||
"https://github.com/Sanster/models/releases/download/add_big_lama/big-lama.pt",
|
||||
config.models_path / "core/misc/lama/lama.pt",
|
||||
"lama infill model",
|
||||
)
|
||||
|
||||
|
||||
# ---------------------------------------------
|
||||
def download_support_models():
|
||||
download_realesrgan()
|
||||
download_lama()
|
||||
download_conversion_models()
|
||||
|
||||
|
||||
|
@ -1,6 +0,0 @@
|
||||
from ldm.modules.image_degradation.bsrgan import ( # noqa: F401
|
||||
degradation_bsrgan_variant as degradation_fn_bsr,
|
||||
)
|
||||
from ldm.modules.image_degradation.bsrgan_light import ( # noqa: F401
|
||||
degradation_bsrgan_variant as degradation_fn_bsr_light,
|
||||
)
|
@ -1,794 +0,0 @@
|
||||
# -*- coding: utf-8 -*-
|
||||
"""
|
||||
# --------------------------------------------
|
||||
# Super-Resolution
|
||||
# --------------------------------------------
|
||||
#
|
||||
# Kai Zhang (cskaizhang@gmail.com)
|
||||
# https://github.com/cszn
|
||||
# From 2019/03--2021/08
|
||||
# --------------------------------------------
|
||||
"""
|
||||
|
||||
import random
|
||||
from functools import partial
|
||||
|
||||
import albumentations
|
||||
import cv2
|
||||
import ldm.modules.image_degradation.utils_image as util
|
||||
import numpy as np
|
||||
import scipy
|
||||
import scipy.stats as ss
|
||||
import torch
|
||||
from scipy import ndimage
|
||||
from scipy.interpolate import interp2d
|
||||
from scipy.linalg import orth
|
||||
|
||||
|
||||
def modcrop_np(img, sf):
|
||||
"""
|
||||
Args:
|
||||
img: numpy image, WxH or WxHxC
|
||||
sf: scale factor
|
||||
Return:
|
||||
cropped image
|
||||
"""
|
||||
w, h = img.shape[:2]
|
||||
im = np.copy(img)
|
||||
return im[: w - w % sf, : h - h % sf, ...]
|
||||
|
||||
|
||||
"""
|
||||
# --------------------------------------------
|
||||
# anisotropic Gaussian kernels
|
||||
# --------------------------------------------
|
||||
"""
|
||||
|
||||
|
||||
def analytic_kernel(k):
|
||||
"""Calculate the X4 kernel from the X2 kernel (for proof see appendix in paper)"""
|
||||
k_size = k.shape[0]
|
||||
# Calculate the big kernels size
|
||||
big_k = np.zeros((3 * k_size - 2, 3 * k_size - 2))
|
||||
# Loop over the small kernel to fill the big one
|
||||
for r in range(k_size):
|
||||
for c in range(k_size):
|
||||
big_k[2 * r : 2 * r + k_size, 2 * c : 2 * c + k_size] += k[r, c] * k
|
||||
# Crop the edges of the big kernel to ignore very small values and increase run time of SR
|
||||
crop = k_size // 2
|
||||
cropped_big_k = big_k[crop:-crop, crop:-crop]
|
||||
# Normalize to 1
|
||||
return cropped_big_k / cropped_big_k.sum()
|
||||
|
||||
|
||||
def anisotropic_Gaussian(ksize=15, theta=np.pi, l1=6, l2=6):
|
||||
"""generate an anisotropic Gaussian kernel
|
||||
Args:
|
||||
ksize : e.g., 15, kernel size
|
||||
theta : [0, pi], rotation angle range
|
||||
l1 : [0.1,50], scaling of eigenvalues
|
||||
l2 : [0.1,l1], scaling of eigenvalues
|
||||
If l1 = l2, will get an isotropic Gaussian kernel.
|
||||
Returns:
|
||||
k : kernel
|
||||
"""
|
||||
|
||||
v = np.dot(
|
||||
np.array([[np.cos(theta), -np.sin(theta)], [np.sin(theta), np.cos(theta)]]),
|
||||
np.array([1.0, 0.0]),
|
||||
)
|
||||
V = np.array([[v[0], v[1]], [v[1], -v[0]]])
|
||||
D = np.array([[l1, 0], [0, l2]])
|
||||
Sigma = np.dot(np.dot(V, D), np.linalg.inv(V))
|
||||
k = gm_blur_kernel(mean=[0, 0], cov=Sigma, size=ksize)
|
||||
|
||||
return k
|
||||
|
||||
|
||||
def gm_blur_kernel(mean, cov, size=15):
|
||||
center = size / 2.0 + 0.5
|
||||
k = np.zeros([size, size])
|
||||
for y in range(size):
|
||||
for x in range(size):
|
||||
cy = y - center + 1
|
||||
cx = x - center + 1
|
||||
k[y, x] = ss.multivariate_normal.pdf([cx, cy], mean=mean, cov=cov)
|
||||
|
||||
k = k / np.sum(k)
|
||||
return k
|
||||
|
||||
|
||||
def shift_pixel(x, sf, upper_left=True):
|
||||
"""shift pixel for super-resolution with different scale factors
|
||||
Args:
|
||||
x: WxHxC or WxH
|
||||
sf: scale factor
|
||||
upper_left: shift direction
|
||||
"""
|
||||
h, w = x.shape[:2]
|
||||
shift = (sf - 1) * 0.5
|
||||
xv, yv = np.arange(0, w, 1.0), np.arange(0, h, 1.0)
|
||||
if upper_left:
|
||||
x1 = xv + shift
|
||||
y1 = yv + shift
|
||||
else:
|
||||
x1 = xv - shift
|
||||
y1 = yv - shift
|
||||
|
||||
x1 = np.clip(x1, 0, w - 1)
|
||||
y1 = np.clip(y1, 0, h - 1)
|
||||
|
||||
if x.ndim == 2:
|
||||
x = interp2d(xv, yv, x)(x1, y1)
|
||||
if x.ndim == 3:
|
||||
for i in range(x.shape[-1]):
|
||||
x[:, :, i] = interp2d(xv, yv, x[:, :, i])(x1, y1)
|
||||
|
||||
return x
|
||||
|
||||
|
||||
def blur(x, k):
|
||||
"""
|
||||
x: image, NxcxHxW
|
||||
k: kernel, Nx1xhxw
|
||||
"""
|
||||
n, c = x.shape[:2]
|
||||
p1, p2 = (k.shape[-2] - 1) // 2, (k.shape[-1] - 1) // 2
|
||||
x = torch.nn.functional.pad(x, pad=(p1, p2, p1, p2), mode="replicate")
|
||||
k = k.repeat(1, c, 1, 1)
|
||||
k = k.view(-1, 1, k.shape[2], k.shape[3])
|
||||
x = x.view(1, -1, x.shape[2], x.shape[3])
|
||||
x = torch.nn.functional.conv2d(x, k, bias=None, stride=1, padding=0, groups=n * c)
|
||||
x = x.view(n, c, x.shape[2], x.shape[3])
|
||||
|
||||
return x
|
||||
|
||||
|
||||
def gen_kernel(
|
||||
k_size=np.array([15, 15]),
|
||||
scale_factor=np.array([4, 4]),
|
||||
min_var=0.6,
|
||||
max_var=10.0,
|
||||
noise_level=0,
|
||||
):
|
||||
""" "
|
||||
# modified version of https://github.com/assafshocher/BlindSR_dataset_generator
|
||||
# Kai Zhang
|
||||
# min_var = 0.175 * sf # variance of the gaussian kernel will be sampled between min_var and max_var
|
||||
# max_var = 2.5 * sf
|
||||
"""
|
||||
# Set random eigen-vals (lambdas) and angle (theta) for COV matrix
|
||||
lambda_1 = min_var + np.random.rand() * (max_var - min_var)
|
||||
lambda_2 = min_var + np.random.rand() * (max_var - min_var)
|
||||
theta = np.random.rand() * np.pi # random theta
|
||||
noise = -noise_level + np.random.rand(*k_size) * noise_level * 2
|
||||
|
||||
# Set COV matrix using Lambdas and Theta
|
||||
LAMBDA = np.diag([lambda_1, lambda_2])
|
||||
Q = np.array([[np.cos(theta), -np.sin(theta)], [np.sin(theta), np.cos(theta)]])
|
||||
SIGMA = Q @ LAMBDA @ Q.T
|
||||
INV_SIGMA = np.linalg.inv(SIGMA)[None, None, :, :]
|
||||
|
||||
# Set expectation position (shifting kernel for aligned image)
|
||||
MU = k_size // 2 - 0.5 * (scale_factor - 1) # - 0.5 * (scale_factor - k_size % 2)
|
||||
MU = MU[None, None, :, None]
|
||||
|
||||
# Create meshgrid for Gaussian
|
||||
[X, Y] = np.meshgrid(range(k_size[0]), range(k_size[1]))
|
||||
Z = np.stack([X, Y], 2)[:, :, :, None]
|
||||
|
||||
# Calcualte Gaussian for every pixel of the kernel
|
||||
ZZ = Z - MU
|
||||
ZZ_t = ZZ.transpose(0, 1, 3, 2)
|
||||
raw_kernel = np.exp(-0.5 * np.squeeze(ZZ_t @ INV_SIGMA @ ZZ)) * (1 + noise)
|
||||
|
||||
# shift the kernel so it will be centered
|
||||
# raw_kernel_centered = kernel_shift(raw_kernel, scale_factor)
|
||||
|
||||
# Normalize the kernel and return
|
||||
# kernel = raw_kernel_centered / np.sum(raw_kernel_centered)
|
||||
kernel = raw_kernel / np.sum(raw_kernel)
|
||||
return kernel
|
||||
|
||||
|
||||
def fspecial_gaussian(hsize, sigma):
|
||||
hsize = [hsize, hsize]
|
||||
siz = [(hsize[0] - 1.0) / 2.0, (hsize[1] - 1.0) / 2.0]
|
||||
std = sigma
|
||||
[x, y] = np.meshgrid(np.arange(-siz[1], siz[1] + 1), np.arange(-siz[0], siz[0] + 1))
|
||||
arg = -(x * x + y * y) / (2 * std * std)
|
||||
h = np.exp(arg)
|
||||
h[h < scipy.finfo(float).eps * h.max()] = 0
|
||||
sumh = h.sum()
|
||||
if sumh != 0:
|
||||
h = h / sumh
|
||||
return h
|
||||
|
||||
|
||||
def fspecial_laplacian(alpha):
|
||||
alpha = max([0, min([alpha, 1])])
|
||||
h1 = alpha / (alpha + 1)
|
||||
h2 = (1 - alpha) / (alpha + 1)
|
||||
h = [[h1, h2, h1], [h2, -4 / (alpha + 1), h2], [h1, h2, h1]]
|
||||
h = np.array(h)
|
||||
return h
|
||||
|
||||
|
||||
def fspecial(filter_type, *args, **kwargs):
|
||||
"""
|
||||
python code from:
|
||||
https://github.com/ronaldosena/imagens-medicas-2/blob/40171a6c259edec7827a6693a93955de2bd39e76/Aulas/aula_2_-_uniform_filter/matlab_fspecial.py
|
||||
"""
|
||||
if filter_type == "gaussian":
|
||||
return fspecial_gaussian(*args, **kwargs)
|
||||
if filter_type == "laplacian":
|
||||
return fspecial_laplacian(*args, **kwargs)
|
||||
|
||||
|
||||
"""
|
||||
# --------------------------------------------
|
||||
# degradation models
|
||||
# --------------------------------------------
|
||||
"""
|
||||
|
||||
|
||||
def bicubic_degradation(x, sf=3):
|
||||
"""
|
||||
Args:
|
||||
x: HxWxC image, [0, 1]
|
||||
sf: down-scale factor
|
||||
Return:
|
||||
bicubicly downsampled LR image
|
||||
"""
|
||||
x = util.imresize_np(x, scale=1 / sf)
|
||||
return x
|
||||
|
||||
|
||||
def srmd_degradation(x, k, sf=3):
|
||||
"""blur + bicubic downsampling
|
||||
Args:
|
||||
x: HxWxC image, [0, 1]
|
||||
k: hxw, double
|
||||
sf: down-scale factor
|
||||
Return:
|
||||
downsampled LR image
|
||||
Reference:
|
||||
@inproceedings{zhang2018learning,
|
||||
title={Learning a single convolutional super-resolution network for multiple degradations},
|
||||
author={Zhang, Kai and Zuo, Wangmeng and Zhang, Lei},
|
||||
booktitle={IEEE Conference on Computer Vision and Pattern Recognition},
|
||||
pages={3262--3271},
|
||||
year={2018}
|
||||
}
|
||||
"""
|
||||
x = ndimage.filters.convolve(x, np.expand_dims(k, axis=2), mode="wrap") # 'nearest' | 'mirror'
|
||||
x = bicubic_degradation(x, sf=sf)
|
||||
return x
|
||||
|
||||
|
||||
def dpsr_degradation(x, k, sf=3):
|
||||
"""bicubic downsampling + blur
|
||||
Args:
|
||||
x: HxWxC image, [0, 1]
|
||||
k: hxw, double
|
||||
sf: down-scale factor
|
||||
Return:
|
||||
downsampled LR image
|
||||
Reference:
|
||||
@inproceedings{zhang2019deep,
|
||||
title={Deep Plug-and-Play Super-Resolution for Arbitrary Blur Kernels},
|
||||
author={Zhang, Kai and Zuo, Wangmeng and Zhang, Lei},
|
||||
booktitle={IEEE Conference on Computer Vision and Pattern Recognition},
|
||||
pages={1671--1681},
|
||||
year={2019}
|
||||
}
|
||||
"""
|
||||
x = bicubic_degradation(x, sf=sf)
|
||||
x = ndimage.filters.convolve(x, np.expand_dims(k, axis=2), mode="wrap")
|
||||
return x
|
||||
|
||||
|
||||
def classical_degradation(x, k, sf=3):
|
||||
"""blur + downsampling
|
||||
Args:
|
||||
x: HxWxC image, [0, 1]/[0, 255]
|
||||
k: hxw, double
|
||||
sf: down-scale factor
|
||||
Return:
|
||||
downsampled LR image
|
||||
"""
|
||||
x = ndimage.filters.convolve(x, np.expand_dims(k, axis=2), mode="wrap")
|
||||
# x = filters.correlate(x, np.expand_dims(np.flip(k), axis=2))
|
||||
st = 0
|
||||
return x[st::sf, st::sf, ...]
|
||||
|
||||
|
||||
def add_sharpening(img, weight=0.5, radius=50, threshold=10):
|
||||
"""USM sharpening. borrowed from real-ESRGAN
|
||||
Input image: I; Blurry image: B.
|
||||
1. K = I + weight * (I - B)
|
||||
2. Mask = 1 if abs(I - B) > threshold, else: 0
|
||||
3. Blur mask:
|
||||
4. Out = Mask * K + (1 - Mask) * I
|
||||
Args:
|
||||
img (Numpy array): Input image, HWC, BGR; float32, [0, 1].
|
||||
weight (float): Sharp weight. Default: 1.
|
||||
radius (float): Kernel size of Gaussian blur. Default: 50.
|
||||
threshold (int):
|
||||
"""
|
||||
if radius % 2 == 0:
|
||||
radius += 1
|
||||
blur = cv2.GaussianBlur(img, (radius, radius), 0)
|
||||
residual = img - blur
|
||||
mask = np.abs(residual) * 255 > threshold
|
||||
mask = mask.astype("float32")
|
||||
soft_mask = cv2.GaussianBlur(mask, (radius, radius), 0)
|
||||
|
||||
K = img + weight * residual
|
||||
K = np.clip(K, 0, 1)
|
||||
return soft_mask * K + (1 - soft_mask) * img
|
||||
|
||||
|
||||
def add_blur(img, sf=4):
|
||||
wd2 = 4.0 + sf
|
||||
wd = 2.0 + 0.2 * sf
|
||||
if random.random() < 0.5:
|
||||
l1 = wd2 * random.random()
|
||||
l2 = wd2 * random.random()
|
||||
k = anisotropic_Gaussian(
|
||||
ksize=2 * random.randint(2, 11) + 3,
|
||||
theta=random.random() * np.pi,
|
||||
l1=l1,
|
||||
l2=l2,
|
||||
)
|
||||
else:
|
||||
k = fspecial("gaussian", 2 * random.randint(2, 11) + 3, wd * random.random())
|
||||
img = ndimage.filters.convolve(img, np.expand_dims(k, axis=2), mode="mirror")
|
||||
|
||||
return img
|
||||
|
||||
|
||||
def add_resize(img, sf=4):
|
||||
rnum = np.random.rand()
|
||||
if rnum > 0.8: # up
|
||||
sf1 = random.uniform(1, 2)
|
||||
elif rnum < 0.7: # down
|
||||
sf1 = random.uniform(0.5 / sf, 1)
|
||||
else:
|
||||
sf1 = 1.0
|
||||
img = cv2.resize(
|
||||
img,
|
||||
(int(sf1 * img.shape[1]), int(sf1 * img.shape[0])),
|
||||
interpolation=random.choice([1, 2, 3]),
|
||||
)
|
||||
img = np.clip(img, 0.0, 1.0)
|
||||
|
||||
return img
|
||||
|
||||
|
||||
# def add_Gaussian_noise(img, noise_level1=2, noise_level2=25):
|
||||
# noise_level = random.randint(noise_level1, noise_level2)
|
||||
# rnum = np.random.rand()
|
||||
# if rnum > 0.6: # add color Gaussian noise
|
||||
# img += np.random.normal(0, noise_level / 255.0, img.shape).astype(np.float32)
|
||||
# elif rnum < 0.4: # add grayscale Gaussian noise
|
||||
# img += np.random.normal(0, noise_level / 255.0, (*img.shape[:2], 1)).astype(np.float32)
|
||||
# else: # add noise
|
||||
# L = noise_level2 / 255.
|
||||
# D = np.diag(np.random.rand(3))
|
||||
# U = orth(np.random.rand(3, 3))
|
||||
# conv = np.dot(np.dot(np.transpose(U), D), U)
|
||||
# img += np.random.multivariate_normal([0, 0, 0], np.abs(L ** 2 * conv), img.shape[:2]).astype(np.float32)
|
||||
# img = np.clip(img, 0.0, 1.0)
|
||||
# return img
|
||||
|
||||
|
||||
def add_Gaussian_noise(img, noise_level1=2, noise_level2=25):
|
||||
noise_level = random.randint(noise_level1, noise_level2)
|
||||
rnum = np.random.rand()
|
||||
if rnum > 0.6: # add color Gaussian noise
|
||||
img = img + np.random.normal(0, noise_level / 255.0, img.shape).astype(np.float32)
|
||||
elif rnum < 0.4: # add grayscale Gaussian noise
|
||||
img = img + np.random.normal(0, noise_level / 255.0, (*img.shape[:2], 1)).astype(np.float32)
|
||||
else: # add noise
|
||||
L = noise_level2 / 255.0
|
||||
D = np.diag(np.random.rand(3))
|
||||
U = orth(np.random.rand(3, 3))
|
||||
conv = np.dot(np.dot(np.transpose(U), D), U)
|
||||
img = img + np.random.multivariate_normal([0, 0, 0], np.abs(L**2 * conv), img.shape[:2]).astype(np.float32)
|
||||
img = np.clip(img, 0.0, 1.0)
|
||||
return img
|
||||
|
||||
|
||||
def add_speckle_noise(img, noise_level1=2, noise_level2=25):
|
||||
noise_level = random.randint(noise_level1, noise_level2)
|
||||
img = np.clip(img, 0.0, 1.0)
|
||||
rnum = random.random()
|
||||
if rnum > 0.6:
|
||||
img += img * np.random.normal(0, noise_level / 255.0, img.shape).astype(np.float32)
|
||||
elif rnum < 0.4:
|
||||
img += img * np.random.normal(0, noise_level / 255.0, (*img.shape[:2], 1)).astype(np.float32)
|
||||
else:
|
||||
L = noise_level2 / 255.0
|
||||
D = np.diag(np.random.rand(3))
|
||||
U = orth(np.random.rand(3, 3))
|
||||
conv = np.dot(np.dot(np.transpose(U), D), U)
|
||||
img += img * np.random.multivariate_normal([0, 0, 0], np.abs(L**2 * conv), img.shape[:2]).astype(np.float32)
|
||||
img = np.clip(img, 0.0, 1.0)
|
||||
return img
|
||||
|
||||
|
||||
def add_Poisson_noise(img):
|
||||
img = np.clip((img * 255.0).round(), 0, 255) / 255.0
|
||||
vals = 10 ** (2 * random.random() + 2.0) # [2, 4]
|
||||
if random.random() < 0.5:
|
||||
img = np.random.poisson(img * vals).astype(np.float32) / vals
|
||||
else:
|
||||
img_gray = np.dot(img[..., :3], [0.299, 0.587, 0.114])
|
||||
img_gray = np.clip((img_gray * 255.0).round(), 0, 255) / 255.0
|
||||
noise_gray = np.random.poisson(img_gray * vals).astype(np.float32) / vals - img_gray
|
||||
img += noise_gray[:, :, np.newaxis]
|
||||
img = np.clip(img, 0.0, 1.0)
|
||||
return img
|
||||
|
||||
|
||||
def add_JPEG_noise(img):
|
||||
quality_factor = random.randint(30, 95)
|
||||
img = cv2.cvtColor(util.single2uint(img), cv2.COLOR_RGB2BGR)
|
||||
result, encimg = cv2.imencode(".jpg", img, [int(cv2.IMWRITE_JPEG_QUALITY), quality_factor])
|
||||
img = cv2.imdecode(encimg, 1)
|
||||
img = cv2.cvtColor(util.uint2single(img), cv2.COLOR_BGR2RGB)
|
||||
return img
|
||||
|
||||
|
||||
def random_crop(lq, hq, sf=4, lq_patchsize=64):
|
||||
h, w = lq.shape[:2]
|
||||
rnd_h = random.randint(0, h - lq_patchsize)
|
||||
rnd_w = random.randint(0, w - lq_patchsize)
|
||||
lq = lq[rnd_h : rnd_h + lq_patchsize, rnd_w : rnd_w + lq_patchsize, :]
|
||||
|
||||
rnd_h_H, rnd_w_H = int(rnd_h * sf), int(rnd_w * sf)
|
||||
hq = hq[
|
||||
rnd_h_H : rnd_h_H + lq_patchsize * sf,
|
||||
rnd_w_H : rnd_w_H + lq_patchsize * sf,
|
||||
:,
|
||||
]
|
||||
return lq, hq
|
||||
|
||||
|
||||
def degradation_bsrgan(img, sf=4, lq_patchsize=72, isp_model=None):
|
||||
"""
|
||||
This is the degradation model of BSRGAN from the paper
|
||||
"Designing a Practical Degradation Model for Deep Blind Image Super-Resolution"
|
||||
----------
|
||||
img: HXWXC, [0, 1], its size should be large than (lq_patchsizexsf)x(lq_patchsizexsf)
|
||||
sf: scale factor
|
||||
isp_model: camera ISP model
|
||||
Returns
|
||||
-------
|
||||
img: low-quality patch, size: lq_patchsizeXlq_patchsizeXC, range: [0, 1]
|
||||
hq: corresponding high-quality patch, size: (lq_patchsizexsf)X(lq_patchsizexsf)XC, range: [0, 1]
|
||||
"""
|
||||
isp_prob, jpeg_prob, scale2_prob = 0.25, 0.9, 0.25
|
||||
sf_ori = sf
|
||||
|
||||
h1, w1 = img.shape[:2]
|
||||
img = img.copy()[: w1 - w1 % sf, : h1 - h1 % sf, ...] # mod crop
|
||||
h, w = img.shape[:2]
|
||||
|
||||
if h < lq_patchsize * sf or w < lq_patchsize * sf:
|
||||
raise ValueError(f"img size ({h1}X{w1}) is too small!")
|
||||
|
||||
hq = img.copy()
|
||||
|
||||
if sf == 4 and random.random() < scale2_prob: # downsample1
|
||||
if np.random.rand() < 0.5:
|
||||
img = cv2.resize(
|
||||
img,
|
||||
(int(1 / 2 * img.shape[1]), int(1 / 2 * img.shape[0])),
|
||||
interpolation=random.choice([1, 2, 3]),
|
||||
)
|
||||
else:
|
||||
img = util.imresize_np(img, 1 / 2, True)
|
||||
img = np.clip(img, 0.0, 1.0)
|
||||
sf = 2
|
||||
|
||||
shuffle_order = random.sample(range(7), 7)
|
||||
idx1, idx2 = shuffle_order.index(2), shuffle_order.index(3)
|
||||
if idx1 > idx2: # keep downsample3 last
|
||||
shuffle_order[idx1], shuffle_order[idx2] = (
|
||||
shuffle_order[idx2],
|
||||
shuffle_order[idx1],
|
||||
)
|
||||
|
||||
for i in shuffle_order:
|
||||
if i == 0:
|
||||
img = add_blur(img, sf=sf)
|
||||
|
||||
elif i == 1:
|
||||
img = add_blur(img, sf=sf)
|
||||
|
||||
elif i == 2:
|
||||
a, b = img.shape[1], img.shape[0]
|
||||
# downsample2
|
||||
if random.random() < 0.75:
|
||||
sf1 = random.uniform(1, 2 * sf)
|
||||
img = cv2.resize(
|
||||
img,
|
||||
(int(1 / sf1 * img.shape[1]), int(1 / sf1 * img.shape[0])),
|
||||
interpolation=random.choice([1, 2, 3]),
|
||||
)
|
||||
else:
|
||||
k = fspecial("gaussian", 25, random.uniform(0.1, 0.6 * sf))
|
||||
k_shifted = shift_pixel(k, sf)
|
||||
k_shifted = k_shifted / k_shifted.sum() # blur with shifted kernel
|
||||
img = ndimage.filters.convolve(img, np.expand_dims(k_shifted, axis=2), mode="mirror")
|
||||
img = img[0::sf, 0::sf, ...] # nearest downsampling
|
||||
img = np.clip(img, 0.0, 1.0)
|
||||
|
||||
elif i == 3:
|
||||
# downsample3
|
||||
img = cv2.resize(
|
||||
img,
|
||||
(int(1 / sf * a), int(1 / sf * b)),
|
||||
interpolation=random.choice([1, 2, 3]),
|
||||
)
|
||||
img = np.clip(img, 0.0, 1.0)
|
||||
|
||||
elif i == 4:
|
||||
# add Gaussian noise
|
||||
img = add_Gaussian_noise(img, noise_level1=2, noise_level2=25)
|
||||
|
||||
elif i == 5:
|
||||
# add JPEG noise
|
||||
if random.random() < jpeg_prob:
|
||||
img = add_JPEG_noise(img)
|
||||
|
||||
elif i == 6:
|
||||
# add processed camera sensor noise
|
||||
if random.random() < isp_prob and isp_model is not None:
|
||||
with torch.no_grad():
|
||||
img, hq = isp_model.forward(img.copy(), hq)
|
||||
|
||||
# add final JPEG compression noise
|
||||
img = add_JPEG_noise(img)
|
||||
|
||||
# random crop
|
||||
img, hq = random_crop(img, hq, sf_ori, lq_patchsize)
|
||||
|
||||
return img, hq
|
||||
|
||||
|
||||
# todo no isp_model?
|
||||
def degradation_bsrgan_variant(image, sf=4, isp_model=None):
|
||||
"""
|
||||
This is the degradation model of BSRGAN from the paper
|
||||
"Designing a Practical Degradation Model for Deep Blind Image Super-Resolution"
|
||||
----------
|
||||
sf: scale factor
|
||||
isp_model: camera ISP model
|
||||
Returns
|
||||
-------
|
||||
img: low-quality patch, size: lq_patchsizeXlq_patchsizeXC, range: [0, 1]
|
||||
hq: corresponding high-quality patch, size: (lq_patchsizexsf)X(lq_patchsizexsf)XC, range: [0, 1]
|
||||
"""
|
||||
image = util.uint2single(image)
|
||||
jpeg_prob, scale2_prob = 0.9, 0.25
|
||||
# isp_prob = 0.25 # uncomment with `if i== 6` block below
|
||||
# sf_ori = sf # uncomment with `if i== 6` block below
|
||||
|
||||
h1, w1 = image.shape[:2]
|
||||
image = image.copy()[: w1 - w1 % sf, : h1 - h1 % sf, ...] # mod crop
|
||||
h, w = image.shape[:2]
|
||||
|
||||
# hq = image.copy() # uncomment with `if i== 6` block below
|
||||
|
||||
if sf == 4 and random.random() < scale2_prob: # downsample1
|
||||
if np.random.rand() < 0.5:
|
||||
image = cv2.resize(
|
||||
image,
|
||||
(int(1 / 2 * image.shape[1]), int(1 / 2 * image.shape[0])),
|
||||
interpolation=random.choice([1, 2, 3]),
|
||||
)
|
||||
else:
|
||||
image = util.imresize_np(image, 1 / 2, True)
|
||||
image = np.clip(image, 0.0, 1.0)
|
||||
sf = 2
|
||||
|
||||
shuffle_order = random.sample(range(7), 7)
|
||||
idx1, idx2 = shuffle_order.index(2), shuffle_order.index(3)
|
||||
if idx1 > idx2: # keep downsample3 last
|
||||
shuffle_order[idx1], shuffle_order[idx2] = (
|
||||
shuffle_order[idx2],
|
||||
shuffle_order[idx1],
|
||||
)
|
||||
|
||||
for i in shuffle_order:
|
||||
if i == 0:
|
||||
image = add_blur(image, sf=sf)
|
||||
|
||||
elif i == 1:
|
||||
image = add_blur(image, sf=sf)
|
||||
|
||||
elif i == 2:
|
||||
a, b = image.shape[1], image.shape[0]
|
||||
# downsample2
|
||||
if random.random() < 0.75:
|
||||
sf1 = random.uniform(1, 2 * sf)
|
||||
image = cv2.resize(
|
||||
image,
|
||||
(
|
||||
int(1 / sf1 * image.shape[1]),
|
||||
int(1 / sf1 * image.shape[0]),
|
||||
),
|
||||
interpolation=random.choice([1, 2, 3]),
|
||||
)
|
||||
else:
|
||||
k = fspecial("gaussian", 25, random.uniform(0.1, 0.6 * sf))
|
||||
k_shifted = shift_pixel(k, sf)
|
||||
k_shifted = k_shifted / k_shifted.sum() # blur with shifted kernel
|
||||
image = ndimage.filters.convolve(image, np.expand_dims(k_shifted, axis=2), mode="mirror")
|
||||
image = image[0::sf, 0::sf, ...] # nearest downsampling
|
||||
image = np.clip(image, 0.0, 1.0)
|
||||
|
||||
elif i == 3:
|
||||
# downsample3
|
||||
image = cv2.resize(
|
||||
image,
|
||||
(int(1 / sf * a), int(1 / sf * b)),
|
||||
interpolation=random.choice([1, 2, 3]),
|
||||
)
|
||||
image = np.clip(image, 0.0, 1.0)
|
||||
|
||||
elif i == 4:
|
||||
# add Gaussian noise
|
||||
image = add_Gaussian_noise(image, noise_level1=2, noise_level2=25)
|
||||
|
||||
elif i == 5:
|
||||
# add JPEG noise
|
||||
if random.random() < jpeg_prob:
|
||||
image = add_JPEG_noise(image)
|
||||
|
||||
# elif i == 6:
|
||||
# # add processed camera sensor noise
|
||||
# if random.random() < isp_prob and isp_model is not None:
|
||||
# with torch.no_grad():
|
||||
# img, hq = isp_model.forward(img.copy(), hq)
|
||||
|
||||
# add final JPEG compression noise
|
||||
image = add_JPEG_noise(image)
|
||||
image = util.single2uint(image)
|
||||
example = {"image": image}
|
||||
return example
|
||||
|
||||
|
||||
# TODO incase there is a pickle error one needs to replace a += x with a = a + x in add_speckle_noise etc...
|
||||
def degradation_bsrgan_plus(
|
||||
img,
|
||||
sf=4,
|
||||
shuffle_prob=0.5,
|
||||
use_sharp=True,
|
||||
lq_patchsize=64,
|
||||
isp_model=None,
|
||||
):
|
||||
"""
|
||||
This is an extended degradation model by combining
|
||||
the degradation models of BSRGAN and Real-ESRGAN
|
||||
----------
|
||||
img: HXWXC, [0, 1], its size should be large than (lq_patchsizexsf)x(lq_patchsizexsf)
|
||||
sf: scale factor
|
||||
use_shuffle: the degradation shuffle
|
||||
use_sharp: sharpening the img
|
||||
Returns
|
||||
-------
|
||||
img: low-quality patch, size: lq_patchsizeXlq_patchsizeXC, range: [0, 1]
|
||||
hq: corresponding high-quality patch, size: (lq_patchsizexsf)X(lq_patchsizexsf)XC, range: [0, 1]
|
||||
"""
|
||||
|
||||
h1, w1 = img.shape[:2]
|
||||
img = img.copy()[: w1 - w1 % sf, : h1 - h1 % sf, ...] # mod crop
|
||||
h, w = img.shape[:2]
|
||||
|
||||
if h < lq_patchsize * sf or w < lq_patchsize * sf:
|
||||
raise ValueError(f"img size ({h1}X{w1}) is too small!")
|
||||
|
||||
if use_sharp:
|
||||
img = add_sharpening(img)
|
||||
hq = img.copy()
|
||||
|
||||
if random.random() < shuffle_prob:
|
||||
shuffle_order = random.sample(range(13), 13)
|
||||
else:
|
||||
shuffle_order = list(range(13))
|
||||
# local shuffle for noise, JPEG is always the last one
|
||||
shuffle_order[2:6] = random.sample(shuffle_order[2:6], len(range(2, 6)))
|
||||
shuffle_order[9:13] = random.sample(shuffle_order[9:13], len(range(9, 13)))
|
||||
|
||||
poisson_prob, speckle_prob, isp_prob = 0.1, 0.1, 0.1
|
||||
|
||||
for i in shuffle_order:
|
||||
if i == 0:
|
||||
img = add_blur(img, sf=sf)
|
||||
elif i == 1:
|
||||
img = add_resize(img, sf=sf)
|
||||
elif i == 2:
|
||||
img = add_Gaussian_noise(img, noise_level1=2, noise_level2=25)
|
||||
elif i == 3:
|
||||
if random.random() < poisson_prob:
|
||||
img = add_Poisson_noise(img)
|
||||
elif i == 4:
|
||||
if random.random() < speckle_prob:
|
||||
img = add_speckle_noise(img)
|
||||
elif i == 5:
|
||||
if random.random() < isp_prob and isp_model is not None:
|
||||
with torch.no_grad():
|
||||
img, hq = isp_model.forward(img.copy(), hq)
|
||||
elif i == 6:
|
||||
img = add_JPEG_noise(img)
|
||||
elif i == 7:
|
||||
img = add_blur(img, sf=sf)
|
||||
elif i == 8:
|
||||
img = add_resize(img, sf=sf)
|
||||
elif i == 9:
|
||||
img = add_Gaussian_noise(img, noise_level1=2, noise_level2=25)
|
||||
elif i == 10:
|
||||
if random.random() < poisson_prob:
|
||||
img = add_Poisson_noise(img)
|
||||
elif i == 11:
|
||||
if random.random() < speckle_prob:
|
||||
img = add_speckle_noise(img)
|
||||
elif i == 12:
|
||||
if random.random() < isp_prob and isp_model is not None:
|
||||
with torch.no_grad():
|
||||
img, hq = isp_model.forward(img.copy(), hq)
|
||||
else:
|
||||
print("check the shuffle!")
|
||||
|
||||
# resize to desired size
|
||||
img = cv2.resize(
|
||||
img,
|
||||
(int(1 / sf * hq.shape[1]), int(1 / sf * hq.shape[0])),
|
||||
interpolation=random.choice([1, 2, 3]),
|
||||
)
|
||||
|
||||
# add final JPEG compression noise
|
||||
img = add_JPEG_noise(img)
|
||||
|
||||
# random crop
|
||||
img, hq = random_crop(img, hq, sf, lq_patchsize)
|
||||
|
||||
return img, hq
|
||||
|
||||
|
||||
if __name__ == "__main__":
|
||||
print("hey")
|
||||
img = util.imread_uint("utils/test.png", 3)
|
||||
print(img)
|
||||
img = util.uint2single(img)
|
||||
print(img)
|
||||
img = img[:448, :448]
|
||||
h = img.shape[0] // 4
|
||||
print("resizing to", h)
|
||||
sf = 4
|
||||
deg_fn = partial(degradation_bsrgan_variant, sf=sf)
|
||||
for i in range(20):
|
||||
print(i)
|
||||
img_lq = deg_fn(img)
|
||||
print(img_lq)
|
||||
img_lq_bicubic = albumentations.SmallestMaxSize(max_size=h, interpolation=cv2.INTER_CUBIC)(image=img)["image"]
|
||||
print(img_lq.shape)
|
||||
print("bicubic", img_lq_bicubic.shape)
|
||||
# print(img_hq.shape)
|
||||
lq_nearest = cv2.resize(
|
||||
util.single2uint(img_lq),
|
||||
(int(sf * img_lq.shape[1]), int(sf * img_lq.shape[0])),
|
||||
interpolation=0,
|
||||
)
|
||||
lq_bicubic_nearest = cv2.resize(
|
||||
util.single2uint(img_lq_bicubic),
|
||||
(int(sf * img_lq.shape[1]), int(sf * img_lq.shape[0])),
|
||||
interpolation=0,
|
||||
)
|
||||
# img_concat = np.concatenate([lq_bicubic_nearest, lq_nearest, util.single2uint(img_hq)], axis=1)
|
||||
img_concat = np.concatenate([lq_bicubic_nearest, lq_nearest], axis=1)
|
||||
util.imsave(img_concat, str(i) + ".png")
|
@ -1,704 +0,0 @@
|
||||
# -*- coding: utf-8 -*-
|
||||
import random
|
||||
from functools import partial
|
||||
|
||||
import albumentations
|
||||
import cv2
|
||||
import ldm.modules.image_degradation.utils_image as util
|
||||
import numpy as np
|
||||
import scipy
|
||||
import scipy.stats as ss
|
||||
import torch
|
||||
from scipy import ndimage
|
||||
from scipy.interpolate import interp2d
|
||||
from scipy.linalg import orth
|
||||
|
||||
"""
|
||||
# --------------------------------------------
|
||||
# Super-Resolution
|
||||
# --------------------------------------------
|
||||
#
|
||||
# Kai Zhang (cskaizhang@gmail.com)
|
||||
# https://github.com/cszn
|
||||
# From 2019/03--2021/08
|
||||
# --------------------------------------------
|
||||
"""
|
||||
|
||||
|
||||
def modcrop_np(img, sf):
|
||||
"""
|
||||
Args:
|
||||
img: numpy image, WxH or WxHxC
|
||||
sf: scale factor
|
||||
Return:
|
||||
cropped image
|
||||
"""
|
||||
w, h = img.shape[:2]
|
||||
im = np.copy(img)
|
||||
return im[: w - w % sf, : h - h % sf, ...]
|
||||
|
||||
|
||||
"""
|
||||
# --------------------------------------------
|
||||
# anisotropic Gaussian kernels
|
||||
# --------------------------------------------
|
||||
"""
|
||||
|
||||
|
||||
def analytic_kernel(k):
|
||||
"""Calculate the X4 kernel from the X2 kernel (for proof see appendix in paper)"""
|
||||
k_size = k.shape[0]
|
||||
# Calculate the big kernels size
|
||||
big_k = np.zeros((3 * k_size - 2, 3 * k_size - 2))
|
||||
# Loop over the small kernel to fill the big one
|
||||
for r in range(k_size):
|
||||
for c in range(k_size):
|
||||
big_k[2 * r : 2 * r + k_size, 2 * c : 2 * c + k_size] += k[r, c] * k
|
||||
# Crop the edges of the big kernel to ignore very small values and increase run time of SR
|
||||
crop = k_size // 2
|
||||
cropped_big_k = big_k[crop:-crop, crop:-crop]
|
||||
# Normalize to 1
|
||||
return cropped_big_k / cropped_big_k.sum()
|
||||
|
||||
|
||||
def anisotropic_Gaussian(ksize=15, theta=np.pi, l1=6, l2=6):
|
||||
"""generate an anisotropic Gaussian kernel
|
||||
Args:
|
||||
ksize : e.g., 15, kernel size
|
||||
theta : [0, pi], rotation angle range
|
||||
l1 : [0.1,50], scaling of eigenvalues
|
||||
l2 : [0.1,l1], scaling of eigenvalues
|
||||
If l1 = l2, will get an isotropic Gaussian kernel.
|
||||
Returns:
|
||||
k : kernel
|
||||
"""
|
||||
|
||||
v = np.dot(
|
||||
np.array([[np.cos(theta), -np.sin(theta)], [np.sin(theta), np.cos(theta)]]),
|
||||
np.array([1.0, 0.0]),
|
||||
)
|
||||
V = np.array([[v[0], v[1]], [v[1], -v[0]]])
|
||||
D = np.array([[l1, 0], [0, l2]])
|
||||
Sigma = np.dot(np.dot(V, D), np.linalg.inv(V))
|
||||
k = gm_blur_kernel(mean=[0, 0], cov=Sigma, size=ksize)
|
||||
|
||||
return k
|
||||
|
||||
|
||||
def gm_blur_kernel(mean, cov, size=15):
|
||||
center = size / 2.0 + 0.5
|
||||
k = np.zeros([size, size])
|
||||
for y in range(size):
|
||||
for x in range(size):
|
||||
cy = y - center + 1
|
||||
cx = x - center + 1
|
||||
k[y, x] = ss.multivariate_normal.pdf([cx, cy], mean=mean, cov=cov)
|
||||
|
||||
k = k / np.sum(k)
|
||||
return k
|
||||
|
||||
|
||||
def shift_pixel(x, sf, upper_left=True):
|
||||
"""shift pixel for super-resolution with different scale factors
|
||||
Args:
|
||||
x: WxHxC or WxH
|
||||
sf: scale factor
|
||||
upper_left: shift direction
|
||||
"""
|
||||
h, w = x.shape[:2]
|
||||
shift = (sf - 1) * 0.5
|
||||
xv, yv = np.arange(0, w, 1.0), np.arange(0, h, 1.0)
|
||||
if upper_left:
|
||||
x1 = xv + shift
|
||||
y1 = yv + shift
|
||||
else:
|
||||
x1 = xv - shift
|
||||
y1 = yv - shift
|
||||
|
||||
x1 = np.clip(x1, 0, w - 1)
|
||||
y1 = np.clip(y1, 0, h - 1)
|
||||
|
||||
if x.ndim == 2:
|
||||
x = interp2d(xv, yv, x)(x1, y1)
|
||||
if x.ndim == 3:
|
||||
for i in range(x.shape[-1]):
|
||||
x[:, :, i] = interp2d(xv, yv, x[:, :, i])(x1, y1)
|
||||
|
||||
return x
|
||||
|
||||
|
||||
def blur(x, k):
|
||||
"""
|
||||
x: image, NxcxHxW
|
||||
k: kernel, Nx1xhxw
|
||||
"""
|
||||
n, c = x.shape[:2]
|
||||
p1, p2 = (k.shape[-2] - 1) // 2, (k.shape[-1] - 1) // 2
|
||||
x = torch.nn.functional.pad(x, pad=(p1, p2, p1, p2), mode="replicate")
|
||||
k = k.repeat(1, c, 1, 1)
|
||||
k = k.view(-1, 1, k.shape[2], k.shape[3])
|
||||
x = x.view(1, -1, x.shape[2], x.shape[3])
|
||||
x = torch.nn.functional.conv2d(x, k, bias=None, stride=1, padding=0, groups=n * c)
|
||||
x = x.view(n, c, x.shape[2], x.shape[3])
|
||||
|
||||
return x
|
||||
|
||||
|
||||
def gen_kernel(
|
||||
k_size=np.array([15, 15]),
|
||||
scale_factor=np.array([4, 4]),
|
||||
min_var=0.6,
|
||||
max_var=10.0,
|
||||
noise_level=0,
|
||||
):
|
||||
""" "
|
||||
# modified version of https://github.com/assafshocher/BlindSR_dataset_generator
|
||||
# Kai Zhang
|
||||
# min_var = 0.175 * sf # variance of the gaussian kernel will be sampled between min_var and max_var
|
||||
# max_var = 2.5 * sf
|
||||
"""
|
||||
# Set random eigen-vals (lambdas) and angle (theta) for COV matrix
|
||||
lambda_1 = min_var + np.random.rand() * (max_var - min_var)
|
||||
lambda_2 = min_var + np.random.rand() * (max_var - min_var)
|
||||
theta = np.random.rand() * np.pi # random theta
|
||||
noise = -noise_level + np.random.rand(*k_size) * noise_level * 2
|
||||
|
||||
# Set COV matrix using Lambdas and Theta
|
||||
LAMBDA = np.diag([lambda_1, lambda_2])
|
||||
Q = np.array([[np.cos(theta), -np.sin(theta)], [np.sin(theta), np.cos(theta)]])
|
||||
SIGMA = Q @ LAMBDA @ Q.T
|
||||
INV_SIGMA = np.linalg.inv(SIGMA)[None, None, :, :]
|
||||
|
||||
# Set expectation position (shifting kernel for aligned image)
|
||||
MU = k_size // 2 - 0.5 * (scale_factor - 1) # - 0.5 * (scale_factor - k_size % 2)
|
||||
MU = MU[None, None, :, None]
|
||||
|
||||
# Create meshgrid for Gaussian
|
||||
[X, Y] = np.meshgrid(range(k_size[0]), range(k_size[1]))
|
||||
Z = np.stack([X, Y], 2)[:, :, :, None]
|
||||
|
||||
# Calcualte Gaussian for every pixel of the kernel
|
||||
ZZ = Z - MU
|
||||
ZZ_t = ZZ.transpose(0, 1, 3, 2)
|
||||
raw_kernel = np.exp(-0.5 * np.squeeze(ZZ_t @ INV_SIGMA @ ZZ)) * (1 + noise)
|
||||
|
||||
# shift the kernel so it will be centered
|
||||
# raw_kernel_centered = kernel_shift(raw_kernel, scale_factor)
|
||||
|
||||
# Normalize the kernel and return
|
||||
# kernel = raw_kernel_centered / np.sum(raw_kernel_centered)
|
||||
kernel = raw_kernel / np.sum(raw_kernel)
|
||||
return kernel
|
||||
|
||||
|
||||
def fspecial_gaussian(hsize, sigma):
|
||||
hsize = [hsize, hsize]
|
||||
siz = [(hsize[0] - 1.0) / 2.0, (hsize[1] - 1.0) / 2.0]
|
||||
std = sigma
|
||||
[x, y] = np.meshgrid(np.arange(-siz[1], siz[1] + 1), np.arange(-siz[0], siz[0] + 1))
|
||||
arg = -(x * x + y * y) / (2 * std * std)
|
||||
h = np.exp(arg)
|
||||
h[h < scipy.finfo(float).eps * h.max()] = 0
|
||||
sumh = h.sum()
|
||||
if sumh != 0:
|
||||
h = h / sumh
|
||||
return h
|
||||
|
||||
|
||||
def fspecial_laplacian(alpha):
|
||||
alpha = max([0, min([alpha, 1])])
|
||||
h1 = alpha / (alpha + 1)
|
||||
h2 = (1 - alpha) / (alpha + 1)
|
||||
h = [[h1, h2, h1], [h2, -4 / (alpha + 1), h2], [h1, h2, h1]]
|
||||
h = np.array(h)
|
||||
return h
|
||||
|
||||
|
||||
def fspecial(filter_type, *args, **kwargs):
|
||||
"""
|
||||
python code from:
|
||||
https://github.com/ronaldosena/imagens-medicas-2/blob/40171a6c259edec7827a6693a93955de2bd39e76/Aulas/aula_2_-_uniform_filter/matlab_fspecial.py
|
||||
"""
|
||||
if filter_type == "gaussian":
|
||||
return fspecial_gaussian(*args, **kwargs)
|
||||
if filter_type == "laplacian":
|
||||
return fspecial_laplacian(*args, **kwargs)
|
||||
|
||||
|
||||
"""
|
||||
# --------------------------------------------
|
||||
# degradation models
|
||||
# --------------------------------------------
|
||||
"""
|
||||
|
||||
|
||||
def bicubic_degradation(x, sf=3):
|
||||
"""
|
||||
Args:
|
||||
x: HxWxC image, [0, 1]
|
||||
sf: down-scale factor
|
||||
Return:
|
||||
bicubicly downsampled LR image
|
||||
"""
|
||||
x = util.imresize_np(x, scale=1 / sf)
|
||||
return x
|
||||
|
||||
|
||||
def srmd_degradation(x, k, sf=3):
|
||||
"""blur + bicubic downsampling
|
||||
Args:
|
||||
x: HxWxC image, [0, 1]
|
||||
k: hxw, double
|
||||
sf: down-scale factor
|
||||
Return:
|
||||
downsampled LR image
|
||||
Reference:
|
||||
@inproceedings{zhang2018learning,
|
||||
title={Learning a single convolutional super-resolution network for multiple degradations},
|
||||
author={Zhang, Kai and Zuo, Wangmeng and Zhang, Lei},
|
||||
booktitle={IEEE Conference on Computer Vision and Pattern Recognition},
|
||||
pages={3262--3271},
|
||||
year={2018}
|
||||
}
|
||||
"""
|
||||
x = ndimage.filters.convolve(x, np.expand_dims(k, axis=2), mode="wrap") # 'nearest' | 'mirror'
|
||||
x = bicubic_degradation(x, sf=sf)
|
||||
return x
|
||||
|
||||
|
||||
def dpsr_degradation(x, k, sf=3):
|
||||
"""bicubic downsampling + blur
|
||||
Args:
|
||||
x: HxWxC image, [0, 1]
|
||||
k: hxw, double
|
||||
sf: down-scale factor
|
||||
Return:
|
||||
downsampled LR image
|
||||
Reference:
|
||||
@inproceedings{zhang2019deep,
|
||||
title={Deep Plug-and-Play Super-Resolution for Arbitrary Blur Kernels},
|
||||
author={Zhang, Kai and Zuo, Wangmeng and Zhang, Lei},
|
||||
booktitle={IEEE Conference on Computer Vision and Pattern Recognition},
|
||||
pages={1671--1681},
|
||||
year={2019}
|
||||
}
|
||||
"""
|
||||
x = bicubic_degradation(x, sf=sf)
|
||||
x = ndimage.filters.convolve(x, np.expand_dims(k, axis=2), mode="wrap")
|
||||
return x
|
||||
|
||||
|
||||
def classical_degradation(x, k, sf=3):
|
||||
"""blur + downsampling
|
||||
Args:
|
||||
x: HxWxC image, [0, 1]/[0, 255]
|
||||
k: hxw, double
|
||||
sf: down-scale factor
|
||||
Return:
|
||||
downsampled LR image
|
||||
"""
|
||||
x = ndimage.filters.convolve(x, np.expand_dims(k, axis=2), mode="wrap")
|
||||
# x = filters.correlate(x, np.expand_dims(np.flip(k), axis=2))
|
||||
st = 0
|
||||
return x[st::sf, st::sf, ...]
|
||||
|
||||
|
||||
def add_sharpening(img, weight=0.5, radius=50, threshold=10):
|
||||
"""USM sharpening. borrowed from real-ESRGAN
|
||||
Input image: I; Blurry image: B.
|
||||
1. K = I + weight * (I - B)
|
||||
2. Mask = 1 if abs(I - B) > threshold, else: 0
|
||||
3. Blur mask:
|
||||
4. Out = Mask * K + (1 - Mask) * I
|
||||
Args:
|
||||
img (Numpy array): Input image, HWC, BGR; float32, [0, 1].
|
||||
weight (float): Sharp weight. Default: 1.
|
||||
radius (float): Kernel size of Gaussian blur. Default: 50.
|
||||
threshold (int):
|
||||
"""
|
||||
if radius % 2 == 0:
|
||||
radius += 1
|
||||
blur = cv2.GaussianBlur(img, (radius, radius), 0)
|
||||
residual = img - blur
|
||||
mask = np.abs(residual) * 255 > threshold
|
||||
mask = mask.astype("float32")
|
||||
soft_mask = cv2.GaussianBlur(mask, (radius, radius), 0)
|
||||
|
||||
K = img + weight * residual
|
||||
K = np.clip(K, 0, 1)
|
||||
return soft_mask * K + (1 - soft_mask) * img
|
||||
|
||||
|
||||
def add_blur(img, sf=4):
|
||||
wd2 = 4.0 + sf
|
||||
wd = 2.0 + 0.2 * sf
|
||||
|
||||
wd2 = wd2 / 4
|
||||
wd = wd / 4
|
||||
|
||||
if random.random() < 0.5:
|
||||
l1 = wd2 * random.random()
|
||||
l2 = wd2 * random.random()
|
||||
k = anisotropic_Gaussian(
|
||||
ksize=random.randint(2, 11) + 3,
|
||||
theta=random.random() * np.pi,
|
||||
l1=l1,
|
||||
l2=l2,
|
||||
)
|
||||
else:
|
||||
k = fspecial("gaussian", random.randint(2, 4) + 3, wd * random.random())
|
||||
img = ndimage.filters.convolve(img, np.expand_dims(k, axis=2), mode="mirror")
|
||||
|
||||
return img
|
||||
|
||||
|
||||
def add_resize(img, sf=4):
|
||||
rnum = np.random.rand()
|
||||
if rnum > 0.8: # up
|
||||
sf1 = random.uniform(1, 2)
|
||||
elif rnum < 0.7: # down
|
||||
sf1 = random.uniform(0.5 / sf, 1)
|
||||
else:
|
||||
sf1 = 1.0
|
||||
img = cv2.resize(
|
||||
img,
|
||||
(int(sf1 * img.shape[1]), int(sf1 * img.shape[0])),
|
||||
interpolation=random.choice([1, 2, 3]),
|
||||
)
|
||||
img = np.clip(img, 0.0, 1.0)
|
||||
|
||||
return img
|
||||
|
||||
|
||||
# def add_Gaussian_noise(img, noise_level1=2, noise_level2=25):
|
||||
# noise_level = random.randint(noise_level1, noise_level2)
|
||||
# rnum = np.random.rand()
|
||||
# if rnum > 0.6: # add color Gaussian noise
|
||||
# img += np.random.normal(0, noise_level / 255.0, img.shape).astype(np.float32)
|
||||
# elif rnum < 0.4: # add grayscale Gaussian noise
|
||||
# img += np.random.normal(0, noise_level / 255.0, (*img.shape[:2], 1)).astype(np.float32)
|
||||
# else: # add noise
|
||||
# L = noise_level2 / 255.
|
||||
# D = np.diag(np.random.rand(3))
|
||||
# U = orth(np.random.rand(3, 3))
|
||||
# conv = np.dot(np.dot(np.transpose(U), D), U)
|
||||
# img += np.random.multivariate_normal([0, 0, 0], np.abs(L ** 2 * conv), img.shape[:2]).astype(np.float32)
|
||||
# img = np.clip(img, 0.0, 1.0)
|
||||
# return img
|
||||
|
||||
|
||||
def add_Gaussian_noise(img, noise_level1=2, noise_level2=25):
|
||||
noise_level = random.randint(noise_level1, noise_level2)
|
||||
rnum = np.random.rand()
|
||||
if rnum > 0.6: # add color Gaussian noise
|
||||
img = img + np.random.normal(0, noise_level / 255.0, img.shape).astype(np.float32)
|
||||
elif rnum < 0.4: # add grayscale Gaussian noise
|
||||
img = img + np.random.normal(0, noise_level / 255.0, (*img.shape[:2], 1)).astype(np.float32)
|
||||
else: # add noise
|
||||
L = noise_level2 / 255.0
|
||||
D = np.diag(np.random.rand(3))
|
||||
U = orth(np.random.rand(3, 3))
|
||||
conv = np.dot(np.dot(np.transpose(U), D), U)
|
||||
img = img + np.random.multivariate_normal([0, 0, 0], np.abs(L**2 * conv), img.shape[:2]).astype(np.float32)
|
||||
img = np.clip(img, 0.0, 1.0)
|
||||
return img
|
||||
|
||||
|
||||
def add_speckle_noise(img, noise_level1=2, noise_level2=25):
|
||||
noise_level = random.randint(noise_level1, noise_level2)
|
||||
img = np.clip(img, 0.0, 1.0)
|
||||
rnum = random.random()
|
||||
if rnum > 0.6:
|
||||
img += img * np.random.normal(0, noise_level / 255.0, img.shape).astype(np.float32)
|
||||
elif rnum < 0.4:
|
||||
img += img * np.random.normal(0, noise_level / 255.0, (*img.shape[:2], 1)).astype(np.float32)
|
||||
else:
|
||||
L = noise_level2 / 255.0
|
||||
D = np.diag(np.random.rand(3))
|
||||
U = orth(np.random.rand(3, 3))
|
||||
conv = np.dot(np.dot(np.transpose(U), D), U)
|
||||
img += img * np.random.multivariate_normal([0, 0, 0], np.abs(L**2 * conv), img.shape[:2]).astype(np.float32)
|
||||
img = np.clip(img, 0.0, 1.0)
|
||||
return img
|
||||
|
||||
|
||||
def add_Poisson_noise(img):
|
||||
img = np.clip((img * 255.0).round(), 0, 255) / 255.0
|
||||
vals = 10 ** (2 * random.random() + 2.0) # [2, 4]
|
||||
if random.random() < 0.5:
|
||||
img = np.random.poisson(img * vals).astype(np.float32) / vals
|
||||
else:
|
||||
img_gray = np.dot(img[..., :3], [0.299, 0.587, 0.114])
|
||||
img_gray = np.clip((img_gray * 255.0).round(), 0, 255) / 255.0
|
||||
noise_gray = np.random.poisson(img_gray * vals).astype(np.float32) / vals - img_gray
|
||||
img += noise_gray[:, :, np.newaxis]
|
||||
img = np.clip(img, 0.0, 1.0)
|
||||
return img
|
||||
|
||||
|
||||
def add_JPEG_noise(img):
|
||||
quality_factor = random.randint(80, 95)
|
||||
img = cv2.cvtColor(util.single2uint(img), cv2.COLOR_RGB2BGR)
|
||||
result, encimg = cv2.imencode(".jpg", img, [int(cv2.IMWRITE_JPEG_QUALITY), quality_factor])
|
||||
img = cv2.imdecode(encimg, 1)
|
||||
img = cv2.cvtColor(util.uint2single(img), cv2.COLOR_BGR2RGB)
|
||||
return img
|
||||
|
||||
|
||||
def random_crop(lq, hq, sf=4, lq_patchsize=64):
|
||||
h, w = lq.shape[:2]
|
||||
rnd_h = random.randint(0, h - lq_patchsize)
|
||||
rnd_w = random.randint(0, w - lq_patchsize)
|
||||
lq = lq[rnd_h : rnd_h + lq_patchsize, rnd_w : rnd_w + lq_patchsize, :]
|
||||
|
||||
rnd_h_H, rnd_w_H = int(rnd_h * sf), int(rnd_w * sf)
|
||||
hq = hq[
|
||||
rnd_h_H : rnd_h_H + lq_patchsize * sf,
|
||||
rnd_w_H : rnd_w_H + lq_patchsize * sf,
|
||||
:,
|
||||
]
|
||||
return lq, hq
|
||||
|
||||
|
||||
def degradation_bsrgan(img, sf=4, lq_patchsize=72, isp_model=None):
|
||||
"""
|
||||
This is the degradation model of BSRGAN from the paper
|
||||
"Designing a Practical Degradation Model for Deep Blind Image Super-Resolution"
|
||||
----------
|
||||
img: HXWXC, [0, 1], its size should be large than (lq_patchsizexsf)x(lq_patchsizexsf)
|
||||
sf: scale factor
|
||||
isp_model: camera ISP model
|
||||
Returns
|
||||
-------
|
||||
img: low-quality patch, size: lq_patchsizeXlq_patchsizeXC, range: [0, 1]
|
||||
hq: corresponding high-quality patch, size: (lq_patchsizexsf)X(lq_patchsizexsf)XC, range: [0, 1]
|
||||
"""
|
||||
isp_prob, jpeg_prob, scale2_prob = 0.25, 0.9, 0.25
|
||||
sf_ori = sf
|
||||
|
||||
h1, w1 = img.shape[:2]
|
||||
img = img.copy()[: w1 - w1 % sf, : h1 - h1 % sf, ...] # mod crop
|
||||
h, w = img.shape[:2]
|
||||
|
||||
if h < lq_patchsize * sf or w < lq_patchsize * sf:
|
||||
raise ValueError(f"img size ({h1}X{w1}) is too small!")
|
||||
|
||||
hq = img.copy()
|
||||
|
||||
if sf == 4 and random.random() < scale2_prob: # downsample1
|
||||
if np.random.rand() < 0.5:
|
||||
img = cv2.resize(
|
||||
img,
|
||||
(int(1 / 2 * img.shape[1]), int(1 / 2 * img.shape[0])),
|
||||
interpolation=random.choice([1, 2, 3]),
|
||||
)
|
||||
else:
|
||||
img = util.imresize_np(img, 1 / 2, True)
|
||||
img = np.clip(img, 0.0, 1.0)
|
||||
sf = 2
|
||||
|
||||
shuffle_order = random.sample(range(7), 7)
|
||||
idx1, idx2 = shuffle_order.index(2), shuffle_order.index(3)
|
||||
if idx1 > idx2: # keep downsample3 last
|
||||
shuffle_order[idx1], shuffle_order[idx2] = (
|
||||
shuffle_order[idx2],
|
||||
shuffle_order[idx1],
|
||||
)
|
||||
|
||||
for i in shuffle_order:
|
||||
if i == 0:
|
||||
img = add_blur(img, sf=sf)
|
||||
|
||||
elif i == 1:
|
||||
img = add_blur(img, sf=sf)
|
||||
|
||||
elif i == 2:
|
||||
a, b = img.shape[1], img.shape[0]
|
||||
# downsample2
|
||||
if random.random() < 0.75:
|
||||
sf1 = random.uniform(1, 2 * sf)
|
||||
img = cv2.resize(
|
||||
img,
|
||||
(int(1 / sf1 * img.shape[1]), int(1 / sf1 * img.shape[0])),
|
||||
interpolation=random.choice([1, 2, 3]),
|
||||
)
|
||||
else:
|
||||
k = fspecial("gaussian", 25, random.uniform(0.1, 0.6 * sf))
|
||||
k_shifted = shift_pixel(k, sf)
|
||||
k_shifted = k_shifted / k_shifted.sum() # blur with shifted kernel
|
||||
img = ndimage.filters.convolve(img, np.expand_dims(k_shifted, axis=2), mode="mirror")
|
||||
img = img[0::sf, 0::sf, ...] # nearest downsampling
|
||||
img = np.clip(img, 0.0, 1.0)
|
||||
|
||||
elif i == 3:
|
||||
# downsample3
|
||||
img = cv2.resize(
|
||||
img,
|
||||
(int(1 / sf * a), int(1 / sf * b)),
|
||||
interpolation=random.choice([1, 2, 3]),
|
||||
)
|
||||
img = np.clip(img, 0.0, 1.0)
|
||||
|
||||
elif i == 4:
|
||||
# add Gaussian noise
|
||||
img = add_Gaussian_noise(img, noise_level1=2, noise_level2=8)
|
||||
|
||||
elif i == 5:
|
||||
# add JPEG noise
|
||||
if random.random() < jpeg_prob:
|
||||
img = add_JPEG_noise(img)
|
||||
|
||||
elif i == 6:
|
||||
# add processed camera sensor noise
|
||||
if random.random() < isp_prob and isp_model is not None:
|
||||
with torch.no_grad():
|
||||
img, hq = isp_model.forward(img.copy(), hq)
|
||||
|
||||
# add final JPEG compression noise
|
||||
img = add_JPEG_noise(img)
|
||||
|
||||
# random crop
|
||||
img, hq = random_crop(img, hq, sf_ori, lq_patchsize)
|
||||
|
||||
return img, hq
|
||||
|
||||
|
||||
# todo no isp_model?
|
||||
def degradation_bsrgan_variant(image, sf=4, isp_model=None):
|
||||
"""
|
||||
This is the degradation model of BSRGAN from the paper
|
||||
"Designing a Practical Degradation Model for Deep Blind Image Super-Resolution"
|
||||
----------
|
||||
sf: scale factor
|
||||
isp_model: camera ISP model
|
||||
Returns
|
||||
-------
|
||||
img: low-quality patch, size: lq_patchsizeXlq_patchsizeXC, range: [0, 1]
|
||||
hq: corresponding high-quality patch, size: (lq_patchsizexsf)X(lq_patchsizexsf)XC, range: [0, 1]
|
||||
"""
|
||||
image = util.uint2single(image)
|
||||
jpeg_prob, scale2_prob = 0.9, 0.25
|
||||
# isp_prob = 0.25 # uncomment with `if i== 6` block below
|
||||
# sf_ori = sf # uncomment with `if i== 6` block below
|
||||
|
||||
h1, w1 = image.shape[:2]
|
||||
image = image.copy()[: w1 - w1 % sf, : h1 - h1 % sf, ...] # mod crop
|
||||
h, w = image.shape[:2]
|
||||
|
||||
# hq = image.copy() # uncomment with `if i== 6` block below
|
||||
|
||||
if sf == 4 and random.random() < scale2_prob: # downsample1
|
||||
if np.random.rand() < 0.5:
|
||||
image = cv2.resize(
|
||||
image,
|
||||
(int(1 / 2 * image.shape[1]), int(1 / 2 * image.shape[0])),
|
||||
interpolation=random.choice([1, 2, 3]),
|
||||
)
|
||||
else:
|
||||
image = util.imresize_np(image, 1 / 2, True)
|
||||
image = np.clip(image, 0.0, 1.0)
|
||||
sf = 2
|
||||
|
||||
shuffle_order = random.sample(range(7), 7)
|
||||
idx1, idx2 = shuffle_order.index(2), shuffle_order.index(3)
|
||||
if idx1 > idx2: # keep downsample3 last
|
||||
shuffle_order[idx1], shuffle_order[idx2] = (
|
||||
shuffle_order[idx2],
|
||||
shuffle_order[idx1],
|
||||
)
|
||||
|
||||
for i in shuffle_order:
|
||||
if i == 0:
|
||||
image = add_blur(image, sf=sf)
|
||||
|
||||
# elif i == 1:
|
||||
# image = add_blur(image, sf=sf)
|
||||
|
||||
if i == 0:
|
||||
pass
|
||||
|
||||
elif i == 2:
|
||||
a, b = image.shape[1], image.shape[0]
|
||||
# downsample2
|
||||
if random.random() < 0.8:
|
||||
sf1 = random.uniform(1, 2 * sf)
|
||||
image = cv2.resize(
|
||||
image,
|
||||
(
|
||||
int(1 / sf1 * image.shape[1]),
|
||||
int(1 / sf1 * image.shape[0]),
|
||||
),
|
||||
interpolation=random.choice([1, 2, 3]),
|
||||
)
|
||||
else:
|
||||
k = fspecial("gaussian", 25, random.uniform(0.1, 0.6 * sf))
|
||||
k_shifted = shift_pixel(k, sf)
|
||||
k_shifted = k_shifted / k_shifted.sum() # blur with shifted kernel
|
||||
image = ndimage.filters.convolve(image, np.expand_dims(k_shifted, axis=2), mode="mirror")
|
||||
image = image[0::sf, 0::sf, ...] # nearest downsampling
|
||||
|
||||
image = np.clip(image, 0.0, 1.0)
|
||||
|
||||
elif i == 3:
|
||||
# downsample3
|
||||
image = cv2.resize(
|
||||
image,
|
||||
(int(1 / sf * a), int(1 / sf * b)),
|
||||
interpolation=random.choice([1, 2, 3]),
|
||||
)
|
||||
image = np.clip(image, 0.0, 1.0)
|
||||
|
||||
elif i == 4:
|
||||
# add Gaussian noise
|
||||
image = add_Gaussian_noise(image, noise_level1=1, noise_level2=2)
|
||||
|
||||
elif i == 5:
|
||||
# add JPEG noise
|
||||
if random.random() < jpeg_prob:
|
||||
image = add_JPEG_noise(image)
|
||||
#
|
||||
# elif i == 6:
|
||||
# # add processed camera sensor noise
|
||||
# if random.random() < isp_prob and isp_model is not None:
|
||||
# with torch.no_grad():
|
||||
# img, hq = isp_model.forward(img.copy(), hq)
|
||||
|
||||
# add final JPEG compression noise
|
||||
image = add_JPEG_noise(image)
|
||||
image = util.single2uint(image)
|
||||
example = {"image": image}
|
||||
return example
|
||||
|
||||
|
||||
if __name__ == "__main__":
|
||||
print("hey")
|
||||
img = util.imread_uint("utils/test.png", 3)
|
||||
img = img[:448, :448]
|
||||
h = img.shape[0] // 4
|
||||
print("resizing to", h)
|
||||
sf = 4
|
||||
deg_fn = partial(degradation_bsrgan_variant, sf=sf)
|
||||
for i in range(20):
|
||||
print(i)
|
||||
img_hq = img
|
||||
img_lq = deg_fn(img)["image"]
|
||||
img_hq, img_lq = util.uint2single(img_hq), util.uint2single(img_lq)
|
||||
print(img_lq)
|
||||
img_lq_bicubic = albumentations.SmallestMaxSize(max_size=h, interpolation=cv2.INTER_CUBIC)(image=img_hq)[
|
||||
"image"
|
||||
]
|
||||
print(img_lq.shape)
|
||||
print("bicubic", img_lq_bicubic.shape)
|
||||
print(img_hq.shape)
|
||||
lq_nearest = cv2.resize(
|
||||
util.single2uint(img_lq),
|
||||
(int(sf * img_lq.shape[1]), int(sf * img_lq.shape[0])),
|
||||
interpolation=0,
|
||||
)
|
||||
lq_bicubic_nearest = cv2.resize(
|
||||
util.single2uint(img_lq_bicubic),
|
||||
(int(sf * img_lq.shape[1]), int(sf * img_lq.shape[0])),
|
||||
interpolation=0,
|
||||
)
|
||||
img_concat = np.concatenate([lq_bicubic_nearest, lq_nearest, util.single2uint(img_hq)], axis=1)
|
||||
util.imsave(img_concat, str(i) + ".png")
|
Binary file not shown.
Before Width: | Height: | Size: 431 KiB |
@ -1,968 +0,0 @@
|
||||
import math
|
||||
import os
|
||||
import random
|
||||
from datetime import datetime
|
||||
|
||||
import cv2
|
||||
import numpy as np
|
||||
import torch
|
||||
from torchvision.utils import make_grid
|
||||
|
||||
import invokeai.backend.util.logging as logger
|
||||
|
||||
os.environ["KMP_DUPLICATE_LIB_OK"] = "TRUE"
|
||||
|
||||
|
||||
"""
|
||||
# --------------------------------------------
|
||||
# Kai Zhang (github: https://github.com/cszn)
|
||||
# 03/Mar/2019
|
||||
# --------------------------------------------
|
||||
# https://github.com/twhui/SRGAN-pyTorch
|
||||
# https://github.com/xinntao/BasicSR
|
||||
# --------------------------------------------
|
||||
"""
|
||||
|
||||
|
||||
IMG_EXTENSIONS = [
|
||||
".jpg",
|
||||
".JPG",
|
||||
".jpeg",
|
||||
".JPEG",
|
||||
".png",
|
||||
".PNG",
|
||||
".ppm",
|
||||
".PPM",
|
||||
".bmp",
|
||||
".BMP",
|
||||
".tif",
|
||||
]
|
||||
|
||||
|
||||
def is_image_file(filename):
|
||||
return any(filename.endswith(extension) for extension in IMG_EXTENSIONS)
|
||||
|
||||
|
||||
def get_timestamp():
|
||||
return datetime.now().strftime("%y%m%d-%H%M%S")
|
||||
|
||||
|
||||
def imshow(x, title=None, cbar=False, figsize=None):
|
||||
import matplotlib.pyplot as plt
|
||||
|
||||
plt.figure(figsize=figsize)
|
||||
plt.imshow(np.squeeze(x), interpolation="nearest", cmap="gray")
|
||||
if title:
|
||||
plt.title(title)
|
||||
if cbar:
|
||||
plt.colorbar()
|
||||
plt.show()
|
||||
|
||||
|
||||
def surf(Z, cmap="rainbow", figsize=None):
|
||||
import matplotlib.pyplot as plt
|
||||
|
||||
plt.figure(figsize=figsize)
|
||||
ax3 = plt.axes(projection="3d")
|
||||
|
||||
w, h = Z.shape[:2]
|
||||
xx = np.arange(0, w, 1)
|
||||
yy = np.arange(0, h, 1)
|
||||
X, Y = np.meshgrid(xx, yy)
|
||||
ax3.plot_surface(X, Y, Z, cmap=cmap)
|
||||
# ax3.contour(X,Y,Z, zdim='z',offset=-2,cmap=cmap)
|
||||
plt.show()
|
||||
|
||||
|
||||
"""
|
||||
# --------------------------------------------
|
||||
# get image pathes
|
||||
# --------------------------------------------
|
||||
"""
|
||||
|
||||
|
||||
def get_image_paths(dataroot):
|
||||
paths = None # return None if dataroot is None
|
||||
if dataroot is not None:
|
||||
paths = sorted(_get_paths_from_images(dataroot))
|
||||
return paths
|
||||
|
||||
|
||||
def _get_paths_from_images(path):
|
||||
assert os.path.isdir(path), "{:s} is not a valid directory".format(path)
|
||||
images = []
|
||||
for dirpath, _, fnames in sorted(os.walk(path, followlinks=True)):
|
||||
for fname in sorted(fnames):
|
||||
if is_image_file(fname):
|
||||
img_path = os.path.join(dirpath, fname)
|
||||
images.append(img_path)
|
||||
assert images, "{:s} has no valid image file".format(path)
|
||||
return images
|
||||
|
||||
|
||||
"""
|
||||
# --------------------------------------------
|
||||
# split large images into small images
|
||||
# --------------------------------------------
|
||||
"""
|
||||
|
||||
|
||||
def patches_from_image(img, p_size=512, p_overlap=64, p_max=800):
|
||||
w, h = img.shape[:2]
|
||||
patches = []
|
||||
if w > p_max and h > p_max:
|
||||
w1 = list(np.arange(0, w - p_size, p_size - p_overlap, dtype=np.int))
|
||||
h1 = list(np.arange(0, h - p_size, p_size - p_overlap, dtype=np.int))
|
||||
w1.append(w - p_size)
|
||||
h1.append(h - p_size)
|
||||
# print(w1)
|
||||
# print(h1)
|
||||
for i in w1:
|
||||
for j in h1:
|
||||
patches.append(img[i : i + p_size, j : j + p_size, :])
|
||||
else:
|
||||
patches.append(img)
|
||||
|
||||
return patches
|
||||
|
||||
|
||||
def imssave(imgs, img_path):
|
||||
"""
|
||||
imgs: list, N images of size WxHxC
|
||||
"""
|
||||
img_name, ext = os.path.splitext(os.path.basename(img_path))
|
||||
|
||||
for i, img in enumerate(imgs):
|
||||
if img.ndim == 3:
|
||||
img = img[:, :, [2, 1, 0]]
|
||||
new_path = os.path.join(
|
||||
os.path.dirname(img_path),
|
||||
img_name + str("_s{:04d}".format(i)) + ".png",
|
||||
)
|
||||
cv2.imwrite(new_path, img)
|
||||
|
||||
|
||||
def split_imageset(
|
||||
original_dataroot,
|
||||
taget_dataroot,
|
||||
n_channels=3,
|
||||
p_size=800,
|
||||
p_overlap=96,
|
||||
p_max=1000,
|
||||
):
|
||||
"""
|
||||
split the large images from original_dataroot into small overlapped images with size (p_size)x(p_size),
|
||||
and save them into taget_dataroot; only the images with larger size than (p_max)x(p_max)
|
||||
will be splitted.
|
||||
Args:
|
||||
original_dataroot:
|
||||
taget_dataroot:
|
||||
p_size: size of small images
|
||||
p_overlap: patch size in training is a good choice
|
||||
p_max: images with smaller size than (p_max)x(p_max) keep unchanged.
|
||||
"""
|
||||
paths = get_image_paths(original_dataroot)
|
||||
for img_path in paths:
|
||||
# img_name, ext = os.path.splitext(os.path.basename(img_path))
|
||||
img = imread_uint(img_path, n_channels=n_channels)
|
||||
patches = patches_from_image(img, p_size, p_overlap, p_max)
|
||||
imssave(patches, os.path.join(taget_dataroot, os.path.basename(img_path)))
|
||||
# if original_dataroot == taget_dataroot:
|
||||
# del img_path
|
||||
|
||||
|
||||
"""
|
||||
# --------------------------------------------
|
||||
# makedir
|
||||
# --------------------------------------------
|
||||
"""
|
||||
|
||||
|
||||
def mkdir(path):
|
||||
if not os.path.exists(path):
|
||||
os.makedirs(path)
|
||||
|
||||
|
||||
def mkdirs(paths):
|
||||
if isinstance(paths, str):
|
||||
mkdir(paths)
|
||||
else:
|
||||
for path in paths:
|
||||
mkdir(path)
|
||||
|
||||
|
||||
def mkdir_and_rename(path):
|
||||
if os.path.exists(path):
|
||||
new_name = path + "_archived_" + get_timestamp()
|
||||
logger.error("Path already exists. Rename it to [{:s}]".format(new_name))
|
||||
os.replace(path, new_name)
|
||||
os.makedirs(path)
|
||||
|
||||
|
||||
"""
|
||||
# --------------------------------------------
|
||||
# read image from path
|
||||
# opencv is fast, but read BGR numpy image
|
||||
# --------------------------------------------
|
||||
"""
|
||||
|
||||
|
||||
# --------------------------------------------
|
||||
# get uint8 image of size HxWxn_channles (RGB)
|
||||
# --------------------------------------------
|
||||
def imread_uint(path, n_channels=3):
|
||||
# input: path
|
||||
# output: HxWx3(RGB or GGG), or HxWx1 (G)
|
||||
if n_channels == 1:
|
||||
img = cv2.imread(path, 0) # cv2.IMREAD_GRAYSCALE
|
||||
img = np.expand_dims(img, axis=2) # HxWx1
|
||||
elif n_channels == 3:
|
||||
img = cv2.imread(path, cv2.IMREAD_UNCHANGED) # BGR or G
|
||||
if img.ndim == 2:
|
||||
img = cv2.cvtColor(img, cv2.COLOR_GRAY2RGB) # GGG
|
||||
else:
|
||||
img = cv2.cvtColor(img, cv2.COLOR_BGR2RGB) # RGB
|
||||
return img
|
||||
|
||||
|
||||
# --------------------------------------------
|
||||
# matlab's imwrite
|
||||
# --------------------------------------------
|
||||
def imsave(img, img_path):
|
||||
img = np.squeeze(img)
|
||||
if img.ndim == 3:
|
||||
img = img[:, :, [2, 1, 0]]
|
||||
cv2.imwrite(img_path, img)
|
||||
|
||||
|
||||
def imwrite(img, img_path):
|
||||
img = np.squeeze(img)
|
||||
if img.ndim == 3:
|
||||
img = img[:, :, [2, 1, 0]]
|
||||
cv2.imwrite(img_path, img)
|
||||
|
||||
|
||||
# --------------------------------------------
|
||||
# get single image of size HxWxn_channles (BGR)
|
||||
# --------------------------------------------
|
||||
def read_img(path):
|
||||
# read image by cv2
|
||||
# return: Numpy float32, HWC, BGR, [0,1]
|
||||
img = cv2.imread(path, cv2.IMREAD_UNCHANGED) # cv2.IMREAD_GRAYSCALE
|
||||
img = img.astype(np.float32) / 255.0
|
||||
if img.ndim == 2:
|
||||
img = np.expand_dims(img, axis=2)
|
||||
# some images have 4 channels
|
||||
if img.shape[2] > 3:
|
||||
img = img[:, :, :3]
|
||||
return img
|
||||
|
||||
|
||||
"""
|
||||
# --------------------------------------------
|
||||
# image format conversion
|
||||
# --------------------------------------------
|
||||
# numpy(single) <---> numpy(unit)
|
||||
# numpy(single) <---> tensor
|
||||
# numpy(unit) <---> tensor
|
||||
# --------------------------------------------
|
||||
"""
|
||||
|
||||
|
||||
# --------------------------------------------
|
||||
# numpy(single) [0, 1] <---> numpy(unit)
|
||||
# --------------------------------------------
|
||||
|
||||
|
||||
def uint2single(img):
|
||||
return np.float32(img / 255.0)
|
||||
|
||||
|
||||
def single2uint(img):
|
||||
return np.uint8((img.clip(0, 1) * 255.0).round())
|
||||
|
||||
|
||||
def uint162single(img):
|
||||
return np.float32(img / 65535.0)
|
||||
|
||||
|
||||
def single2uint16(img):
|
||||
return np.uint16((img.clip(0, 1) * 65535.0).round())
|
||||
|
||||
|
||||
# --------------------------------------------
|
||||
# numpy(unit) (HxWxC or HxW) <---> tensor
|
||||
# --------------------------------------------
|
||||
|
||||
|
||||
# convert uint to 4-dimensional torch tensor
|
||||
def uint2tensor4(img):
|
||||
if img.ndim == 2:
|
||||
img = np.expand_dims(img, axis=2)
|
||||
return torch.from_numpy(np.ascontiguousarray(img)).permute(2, 0, 1).float().div(255.0).unsqueeze(0)
|
||||
|
||||
|
||||
# convert uint to 3-dimensional torch tensor
|
||||
def uint2tensor3(img):
|
||||
if img.ndim == 2:
|
||||
img = np.expand_dims(img, axis=2)
|
||||
return torch.from_numpy(np.ascontiguousarray(img)).permute(2, 0, 1).float().div(255.0)
|
||||
|
||||
|
||||
# convert 2/3/4-dimensional torch tensor to uint
|
||||
def tensor2uint(img):
|
||||
img = img.data.squeeze().float().clamp_(0, 1).cpu().numpy()
|
||||
if img.ndim == 3:
|
||||
img = np.transpose(img, (1, 2, 0))
|
||||
return np.uint8((img * 255.0).round())
|
||||
|
||||
|
||||
# --------------------------------------------
|
||||
# numpy(single) (HxWxC) <---> tensor
|
||||
# --------------------------------------------
|
||||
|
||||
|
||||
# convert single (HxWxC) to 3-dimensional torch tensor
|
||||
def single2tensor3(img):
|
||||
return torch.from_numpy(np.ascontiguousarray(img)).permute(2, 0, 1).float()
|
||||
|
||||
|
||||
# convert single (HxWxC) to 4-dimensional torch tensor
|
||||
def single2tensor4(img):
|
||||
return torch.from_numpy(np.ascontiguousarray(img)).permute(2, 0, 1).float().unsqueeze(0)
|
||||
|
||||
|
||||
# convert torch tensor to single
|
||||
def tensor2single(img):
|
||||
img = img.data.squeeze().float().cpu().numpy()
|
||||
if img.ndim == 3:
|
||||
img = np.transpose(img, (1, 2, 0))
|
||||
|
||||
return img
|
||||
|
||||
|
||||
# convert torch tensor to single
|
||||
def tensor2single3(img):
|
||||
img = img.data.squeeze().float().cpu().numpy()
|
||||
if img.ndim == 3:
|
||||
img = np.transpose(img, (1, 2, 0))
|
||||
elif img.ndim == 2:
|
||||
img = np.expand_dims(img, axis=2)
|
||||
return img
|
||||
|
||||
|
||||
def single2tensor5(img):
|
||||
return torch.from_numpy(np.ascontiguousarray(img)).permute(2, 0, 1, 3).float().unsqueeze(0)
|
||||
|
||||
|
||||
def single32tensor5(img):
|
||||
return torch.from_numpy(np.ascontiguousarray(img)).float().unsqueeze(0).unsqueeze(0)
|
||||
|
||||
|
||||
def single42tensor4(img):
|
||||
return torch.from_numpy(np.ascontiguousarray(img)).permute(2, 0, 1, 3).float()
|
||||
|
||||
|
||||
# from skimage.io import imread, imsave
|
||||
def tensor2img(tensor, out_type=np.uint8, min_max=(0, 1)):
|
||||
"""
|
||||
Converts a torch Tensor into an image Numpy array of BGR channel order
|
||||
Input: 4D(B,(3/1),H,W), 3D(C,H,W), or 2D(H,W), any range, RGB channel order
|
||||
Output: 3D(H,W,C) or 2D(H,W), [0,255], np.uint8 (default)
|
||||
"""
|
||||
tensor = tensor.squeeze().float().cpu().clamp_(*min_max) # squeeze first, then clamp
|
||||
tensor = (tensor - min_max[0]) / (min_max[1] - min_max[0]) # to range [0,1]
|
||||
n_dim = tensor.dim()
|
||||
if n_dim == 4:
|
||||
n_img = len(tensor)
|
||||
img_np = make_grid(tensor, nrow=int(math.sqrt(n_img)), normalize=False).numpy()
|
||||
img_np = np.transpose(img_np[[2, 1, 0], :, :], (1, 2, 0)) # HWC, BGR
|
||||
elif n_dim == 3:
|
||||
img_np = tensor.numpy()
|
||||
img_np = np.transpose(img_np[[2, 1, 0], :, :], (1, 2, 0)) # HWC, BGR
|
||||
elif n_dim == 2:
|
||||
img_np = tensor.numpy()
|
||||
else:
|
||||
raise TypeError("Only support 4D, 3D and 2D tensor. But received with dimension: {:d}".format(n_dim))
|
||||
if out_type == np.uint8:
|
||||
img_np = (img_np * 255.0).round()
|
||||
# Important. Unlike matlab, numpy.unit8() WILL NOT round by default.
|
||||
return img_np.astype(out_type)
|
||||
|
||||
|
||||
"""
|
||||
# --------------------------------------------
|
||||
# Augmentation, flipe and/or rotate
|
||||
# --------------------------------------------
|
||||
# The following two are enough.
|
||||
# (1) augmet_img: numpy image of WxHxC or WxH
|
||||
# (2) augment_img_tensor4: tensor image 1xCxWxH
|
||||
# --------------------------------------------
|
||||
"""
|
||||
|
||||
|
||||
def augment_img(img, mode=0):
|
||||
"""Kai Zhang (github: https://github.com/cszn)"""
|
||||
if mode == 0:
|
||||
return img
|
||||
elif mode == 1:
|
||||
return np.flipud(np.rot90(img))
|
||||
elif mode == 2:
|
||||
return np.flipud(img)
|
||||
elif mode == 3:
|
||||
return np.rot90(img, k=3)
|
||||
elif mode == 4:
|
||||
return np.flipud(np.rot90(img, k=2))
|
||||
elif mode == 5:
|
||||
return np.rot90(img)
|
||||
elif mode == 6:
|
||||
return np.rot90(img, k=2)
|
||||
elif mode == 7:
|
||||
return np.flipud(np.rot90(img, k=3))
|
||||
|
||||
|
||||
def augment_img_tensor4(img, mode=0):
|
||||
"""Kai Zhang (github: https://github.com/cszn)"""
|
||||
if mode == 0:
|
||||
return img
|
||||
elif mode == 1:
|
||||
return img.rot90(1, [2, 3]).flip([2])
|
||||
elif mode == 2:
|
||||
return img.flip([2])
|
||||
elif mode == 3:
|
||||
return img.rot90(3, [2, 3])
|
||||
elif mode == 4:
|
||||
return img.rot90(2, [2, 3]).flip([2])
|
||||
elif mode == 5:
|
||||
return img.rot90(1, [2, 3])
|
||||
elif mode == 6:
|
||||
return img.rot90(2, [2, 3])
|
||||
elif mode == 7:
|
||||
return img.rot90(3, [2, 3]).flip([2])
|
||||
|
||||
|
||||
def augment_img_tensor(img, mode=0):
|
||||
"""Kai Zhang (github: https://github.com/cszn)"""
|
||||
img_size = img.size()
|
||||
img_np = img.data.cpu().numpy()
|
||||
if len(img_size) == 3:
|
||||
img_np = np.transpose(img_np, (1, 2, 0))
|
||||
elif len(img_size) == 4:
|
||||
img_np = np.transpose(img_np, (2, 3, 1, 0))
|
||||
img_np = augment_img(img_np, mode=mode)
|
||||
img_tensor = torch.from_numpy(np.ascontiguousarray(img_np))
|
||||
if len(img_size) == 3:
|
||||
img_tensor = img_tensor.permute(2, 0, 1)
|
||||
elif len(img_size) == 4:
|
||||
img_tensor = img_tensor.permute(3, 2, 0, 1)
|
||||
|
||||
return img_tensor.type_as(img)
|
||||
|
||||
|
||||
def augment_img_np3(img, mode=0):
|
||||
if mode == 0:
|
||||
return img
|
||||
elif mode == 1:
|
||||
return img.transpose(1, 0, 2)
|
||||
elif mode == 2:
|
||||
return img[::-1, :, :]
|
||||
elif mode == 3:
|
||||
img = img[::-1, :, :]
|
||||
img = img.transpose(1, 0, 2)
|
||||
return img
|
||||
elif mode == 4:
|
||||
return img[:, ::-1, :]
|
||||
elif mode == 5:
|
||||
img = img[:, ::-1, :]
|
||||
img = img.transpose(1, 0, 2)
|
||||
return img
|
||||
elif mode == 6:
|
||||
img = img[:, ::-1, :]
|
||||
img = img[::-1, :, :]
|
||||
return img
|
||||
elif mode == 7:
|
||||
img = img[:, ::-1, :]
|
||||
img = img[::-1, :, :]
|
||||
img = img.transpose(1, 0, 2)
|
||||
return img
|
||||
|
||||
|
||||
def augment_imgs(img_list, hflip=True, rot=True):
|
||||
# horizontal flip OR rotate
|
||||
hflip = hflip and random.random() < 0.5
|
||||
vflip = rot and random.random() < 0.5
|
||||
rot90 = rot and random.random() < 0.5
|
||||
|
||||
def _augment(img):
|
||||
if hflip:
|
||||
img = img[:, ::-1, :]
|
||||
if vflip:
|
||||
img = img[::-1, :, :]
|
||||
if rot90:
|
||||
img = img.transpose(1, 0, 2)
|
||||
return img
|
||||
|
||||
return [_augment(img) for img in img_list]
|
||||
|
||||
|
||||
"""
|
||||
# --------------------------------------------
|
||||
# modcrop and shave
|
||||
# --------------------------------------------
|
||||
"""
|
||||
|
||||
|
||||
def modcrop(img_in, scale):
|
||||
# img_in: Numpy, HWC or HW
|
||||
img = np.copy(img_in)
|
||||
if img.ndim == 2:
|
||||
H, W = img.shape
|
||||
H_r, W_r = H % scale, W % scale
|
||||
img = img[: H - H_r, : W - W_r]
|
||||
elif img.ndim == 3:
|
||||
H, W, C = img.shape
|
||||
H_r, W_r = H % scale, W % scale
|
||||
img = img[: H - H_r, : W - W_r, :]
|
||||
else:
|
||||
raise ValueError("Wrong img ndim: [{:d}].".format(img.ndim))
|
||||
return img
|
||||
|
||||
|
||||
def shave(img_in, border=0):
|
||||
# img_in: Numpy, HWC or HW
|
||||
img = np.copy(img_in)
|
||||
h, w = img.shape[:2]
|
||||
img = img[border : h - border, border : w - border]
|
||||
return img
|
||||
|
||||
|
||||
"""
|
||||
# --------------------------------------------
|
||||
# image processing process on numpy image
|
||||
# channel_convert(in_c, tar_type, img_list):
|
||||
# rgb2ycbcr(img, only_y=True):
|
||||
# bgr2ycbcr(img, only_y=True):
|
||||
# ycbcr2rgb(img):
|
||||
# --------------------------------------------
|
||||
"""
|
||||
|
||||
|
||||
def rgb2ycbcr(img, only_y=True):
|
||||
"""same as matlab rgb2ycbcr
|
||||
only_y: only return Y channel
|
||||
Input:
|
||||
uint8, [0, 255]
|
||||
float, [0, 1]
|
||||
"""
|
||||
in_img_type = img.dtype
|
||||
img.astype(np.float32)
|
||||
if in_img_type != np.uint8:
|
||||
img *= 255.0
|
||||
# convert
|
||||
if only_y:
|
||||
rlt = np.dot(img, [65.481, 128.553, 24.966]) / 255.0 + 16.0
|
||||
else:
|
||||
rlt = np.matmul(
|
||||
img,
|
||||
[
|
||||
[65.481, -37.797, 112.0],
|
||||
[128.553, -74.203, -93.786],
|
||||
[24.966, 112.0, -18.214],
|
||||
],
|
||||
) / 255.0 + [16, 128, 128]
|
||||
if in_img_type == np.uint8:
|
||||
rlt = rlt.round()
|
||||
else:
|
||||
rlt /= 255.0
|
||||
return rlt.astype(in_img_type)
|
||||
|
||||
|
||||
def ycbcr2rgb(img):
|
||||
"""same as matlab ycbcr2rgb
|
||||
Input:
|
||||
uint8, [0, 255]
|
||||
float, [0, 1]
|
||||
"""
|
||||
in_img_type = img.dtype
|
||||
img.astype(np.float32)
|
||||
if in_img_type != np.uint8:
|
||||
img *= 255.0
|
||||
# convert
|
||||
rlt = np.matmul(
|
||||
img,
|
||||
[
|
||||
[0.00456621, 0.00456621, 0.00456621],
|
||||
[0, -0.00153632, 0.00791071],
|
||||
[0.00625893, -0.00318811, 0],
|
||||
],
|
||||
) * 255.0 + [-222.921, 135.576, -276.836]
|
||||
if in_img_type == np.uint8:
|
||||
rlt = rlt.round()
|
||||
else:
|
||||
rlt /= 255.0
|
||||
return rlt.astype(in_img_type)
|
||||
|
||||
|
||||
def bgr2ycbcr(img, only_y=True):
|
||||
"""bgr version of rgb2ycbcr
|
||||
only_y: only return Y channel
|
||||
Input:
|
||||
uint8, [0, 255]
|
||||
float, [0, 1]
|
||||
"""
|
||||
in_img_type = img.dtype
|
||||
img.astype(np.float32)
|
||||
if in_img_type != np.uint8:
|
||||
img *= 255.0
|
||||
# convert
|
||||
if only_y:
|
||||
rlt = np.dot(img, [24.966, 128.553, 65.481]) / 255.0 + 16.0
|
||||
else:
|
||||
rlt = np.matmul(
|
||||
img,
|
||||
[
|
||||
[24.966, 112.0, -18.214],
|
||||
[128.553, -74.203, -93.786],
|
||||
[65.481, -37.797, 112.0],
|
||||
],
|
||||
) / 255.0 + [16, 128, 128]
|
||||
if in_img_type == np.uint8:
|
||||
rlt = rlt.round()
|
||||
else:
|
||||
rlt /= 255.0
|
||||
return rlt.astype(in_img_type)
|
||||
|
||||
|
||||
def channel_convert(in_c, tar_type, img_list):
|
||||
# conversion among BGR, gray and y
|
||||
if in_c == 3 and tar_type == "gray": # BGR to gray
|
||||
gray_list = [cv2.cvtColor(img, cv2.COLOR_BGR2GRAY) for img in img_list]
|
||||
return [np.expand_dims(img, axis=2) for img in gray_list]
|
||||
elif in_c == 3 and tar_type == "y": # BGR to y
|
||||
y_list = [bgr2ycbcr(img, only_y=True) for img in img_list]
|
||||
return [np.expand_dims(img, axis=2) for img in y_list]
|
||||
elif in_c == 1 and tar_type == "RGB": # gray/y to BGR
|
||||
return [cv2.cvtColor(img, cv2.COLOR_GRAY2BGR) for img in img_list]
|
||||
else:
|
||||
return img_list
|
||||
|
||||
|
||||
"""
|
||||
# --------------------------------------------
|
||||
# metric, PSNR and SSIM
|
||||
# --------------------------------------------
|
||||
"""
|
||||
|
||||
|
||||
# --------------------------------------------
|
||||
# PSNR
|
||||
# --------------------------------------------
|
||||
def calculate_psnr(img1, img2, border=0):
|
||||
# img1 and img2 have range [0, 255]
|
||||
# img1 = img1.squeeze()
|
||||
# img2 = img2.squeeze()
|
||||
if not img1.shape == img2.shape:
|
||||
raise ValueError("Input images must have the same dimensions.")
|
||||
h, w = img1.shape[:2]
|
||||
img1 = img1[border : h - border, border : w - border]
|
||||
img2 = img2[border : h - border, border : w - border]
|
||||
|
||||
img1 = img1.astype(np.float64)
|
||||
img2 = img2.astype(np.float64)
|
||||
mse = np.mean((img1 - img2) ** 2)
|
||||
if mse == 0:
|
||||
return float("inf")
|
||||
return 20 * math.log10(255.0 / math.sqrt(mse))
|
||||
|
||||
|
||||
# --------------------------------------------
|
||||
# SSIM
|
||||
# --------------------------------------------
|
||||
def calculate_ssim(img1, img2, border=0):
|
||||
"""calculate SSIM
|
||||
the same outputs as MATLAB's
|
||||
img1, img2: [0, 255]
|
||||
"""
|
||||
# img1 = img1.squeeze()
|
||||
# img2 = img2.squeeze()
|
||||
if not img1.shape == img2.shape:
|
||||
raise ValueError("Input images must have the same dimensions.")
|
||||
h, w = img1.shape[:2]
|
||||
img1 = img1[border : h - border, border : w - border]
|
||||
img2 = img2[border : h - border, border : w - border]
|
||||
|
||||
if img1.ndim == 2:
|
||||
return ssim(img1, img2)
|
||||
elif img1.ndim == 3:
|
||||
if img1.shape[2] == 3:
|
||||
ssims = []
|
||||
for i in range(3):
|
||||
ssims.append(ssim(img1[:, :, i], img2[:, :, i]))
|
||||
return np.array(ssims).mean()
|
||||
elif img1.shape[2] == 1:
|
||||
return ssim(np.squeeze(img1), np.squeeze(img2))
|
||||
else:
|
||||
raise ValueError("Wrong input image dimensions.")
|
||||
|
||||
|
||||
def ssim(img1, img2):
|
||||
C1 = (0.01 * 255) ** 2
|
||||
C2 = (0.03 * 255) ** 2
|
||||
|
||||
img1 = img1.astype(np.float64)
|
||||
img2 = img2.astype(np.float64)
|
||||
kernel = cv2.getGaussianKernel(11, 1.5)
|
||||
window = np.outer(kernel, kernel.transpose())
|
||||
|
||||
mu1 = cv2.filter2D(img1, -1, window)[5:-5, 5:-5] # valid
|
||||
mu2 = cv2.filter2D(img2, -1, window)[5:-5, 5:-5]
|
||||
mu1_sq = mu1**2
|
||||
mu2_sq = mu2**2
|
||||
mu1_mu2 = mu1 * mu2
|
||||
sigma1_sq = cv2.filter2D(img1**2, -1, window)[5:-5, 5:-5] - mu1_sq
|
||||
sigma2_sq = cv2.filter2D(img2**2, -1, window)[5:-5, 5:-5] - mu2_sq
|
||||
sigma12 = cv2.filter2D(img1 * img2, -1, window)[5:-5, 5:-5] - mu1_mu2
|
||||
|
||||
ssim_map = ((2 * mu1_mu2 + C1) * (2 * sigma12 + C2)) / ((mu1_sq + mu2_sq + C1) * (sigma1_sq + sigma2_sq + C2))
|
||||
return ssim_map.mean()
|
||||
|
||||
|
||||
"""
|
||||
# --------------------------------------------
|
||||
# matlab's bicubic imresize (numpy and torch) [0, 1]
|
||||
# --------------------------------------------
|
||||
"""
|
||||
|
||||
|
||||
# matlab 'imresize' function, now only support 'bicubic'
|
||||
def cubic(x):
|
||||
absx = torch.abs(x)
|
||||
absx2 = absx**2
|
||||
absx3 = absx**3
|
||||
return (1.5 * absx3 - 2.5 * absx2 + 1) * ((absx <= 1).type_as(absx)) + (
|
||||
-0.5 * absx3 + 2.5 * absx2 - 4 * absx + 2
|
||||
) * (((absx > 1) * (absx <= 2)).type_as(absx))
|
||||
|
||||
|
||||
def calculate_weights_indices(in_length, out_length, scale, kernel, kernel_width, antialiasing):
|
||||
if (scale < 1) and (antialiasing):
|
||||
# Use a modified kernel to simultaneously interpolate and antialias- larger kernel width
|
||||
kernel_width = kernel_width / scale
|
||||
|
||||
# Output-space coordinates
|
||||
x = torch.linspace(1, out_length, out_length)
|
||||
|
||||
# Input-space coordinates. Calculate the inverse mapping such that 0.5
|
||||
# in output space maps to 0.5 in input space, and 0.5+scale in output
|
||||
# space maps to 1.5 in input space.
|
||||
u = x / scale + 0.5 * (1 - 1 / scale)
|
||||
|
||||
# What is the left-most pixel that can be involved in the computation?
|
||||
left = torch.floor(u - kernel_width / 2)
|
||||
|
||||
# What is the maximum number of pixels that can be involved in the
|
||||
# computation? Note: it's OK to use an extra pixel here; if the
|
||||
# corresponding weights are all zero, it will be eliminated at the end
|
||||
# of this function.
|
||||
P = math.ceil(kernel_width) + 2
|
||||
|
||||
# The indices of the input pixels involved in computing the k-th output
|
||||
# pixel are in row k of the indices matrix.
|
||||
indices = left.view(out_length, 1).expand(out_length, P) + torch.linspace(0, P - 1, P).view(1, P).expand(
|
||||
out_length, P
|
||||
)
|
||||
|
||||
# The weights used to compute the k-th output pixel are in row k of the
|
||||
# weights matrix.
|
||||
distance_to_center = u.view(out_length, 1).expand(out_length, P) - indices
|
||||
# apply cubic kernel
|
||||
if (scale < 1) and (antialiasing):
|
||||
weights = scale * cubic(distance_to_center * scale)
|
||||
else:
|
||||
weights = cubic(distance_to_center)
|
||||
# Normalize the weights matrix so that each row sums to 1.
|
||||
weights_sum = torch.sum(weights, 1).view(out_length, 1)
|
||||
weights = weights / weights_sum.expand(out_length, P)
|
||||
|
||||
# If a column in weights is all zero, get rid of it. only consider the first and last column.
|
||||
weights_zero_tmp = torch.sum((weights == 0), 0)
|
||||
if not math.isclose(weights_zero_tmp[0], 0, rel_tol=1e-6):
|
||||
indices = indices.narrow(1, 1, P - 2)
|
||||
weights = weights.narrow(1, 1, P - 2)
|
||||
if not math.isclose(weights_zero_tmp[-1], 0, rel_tol=1e-6):
|
||||
indices = indices.narrow(1, 0, P - 2)
|
||||
weights = weights.narrow(1, 0, P - 2)
|
||||
weights = weights.contiguous()
|
||||
indices = indices.contiguous()
|
||||
sym_len_s = -indices.min() + 1
|
||||
sym_len_e = indices.max() - in_length
|
||||
indices = indices + sym_len_s - 1
|
||||
return weights, indices, int(sym_len_s), int(sym_len_e)
|
||||
|
||||
|
||||
# --------------------------------------------
|
||||
# imresize for tensor image [0, 1]
|
||||
# --------------------------------------------
|
||||
def imresize(img, scale, antialiasing=True):
|
||||
# Now the scale should be the same for H and W
|
||||
# input: img: pytorch tensor, CHW or HW [0,1]
|
||||
# output: CHW or HW [0,1] w/o round
|
||||
need_squeeze = True if img.dim() == 2 else False
|
||||
if need_squeeze:
|
||||
img.unsqueeze_(0)
|
||||
in_C, in_H, in_W = img.size()
|
||||
out_C, out_H, out_W = (
|
||||
in_C,
|
||||
math.ceil(in_H * scale),
|
||||
math.ceil(in_W * scale),
|
||||
)
|
||||
kernel_width = 4
|
||||
kernel = "cubic"
|
||||
|
||||
# Return the desired dimension order for performing the resize. The
|
||||
# strategy is to perform the resize first along the dimension with the
|
||||
# smallest scale factor.
|
||||
# Now we do not support this.
|
||||
|
||||
# get weights and indices
|
||||
weights_H, indices_H, sym_len_Hs, sym_len_He = calculate_weights_indices(
|
||||
in_H, out_H, scale, kernel, kernel_width, antialiasing
|
||||
)
|
||||
weights_W, indices_W, sym_len_Ws, sym_len_We = calculate_weights_indices(
|
||||
in_W, out_W, scale, kernel, kernel_width, antialiasing
|
||||
)
|
||||
# process H dimension
|
||||
# symmetric copying
|
||||
img_aug = torch.FloatTensor(in_C, in_H + sym_len_Hs + sym_len_He, in_W)
|
||||
img_aug.narrow(1, sym_len_Hs, in_H).copy_(img)
|
||||
|
||||
sym_patch = img[:, :sym_len_Hs, :]
|
||||
inv_idx = torch.arange(sym_patch.size(1) - 1, -1, -1).long()
|
||||
sym_patch_inv = sym_patch.index_select(1, inv_idx)
|
||||
img_aug.narrow(1, 0, sym_len_Hs).copy_(sym_patch_inv)
|
||||
|
||||
sym_patch = img[:, -sym_len_He:, :]
|
||||
inv_idx = torch.arange(sym_patch.size(1) - 1, -1, -1).long()
|
||||
sym_patch_inv = sym_patch.index_select(1, inv_idx)
|
||||
img_aug.narrow(1, sym_len_Hs + in_H, sym_len_He).copy_(sym_patch_inv)
|
||||
|
||||
out_1 = torch.FloatTensor(in_C, out_H, in_W)
|
||||
kernel_width = weights_H.size(1)
|
||||
for i in range(out_H):
|
||||
idx = int(indices_H[i][0])
|
||||
for j in range(out_C):
|
||||
out_1[j, i, :] = img_aug[j, idx : idx + kernel_width, :].transpose(0, 1).mv(weights_H[i])
|
||||
|
||||
# process W dimension
|
||||
# symmetric copying
|
||||
out_1_aug = torch.FloatTensor(in_C, out_H, in_W + sym_len_Ws + sym_len_We)
|
||||
out_1_aug.narrow(2, sym_len_Ws, in_W).copy_(out_1)
|
||||
|
||||
sym_patch = out_1[:, :, :sym_len_Ws]
|
||||
inv_idx = torch.arange(sym_patch.size(2) - 1, -1, -1).long()
|
||||
sym_patch_inv = sym_patch.index_select(2, inv_idx)
|
||||
out_1_aug.narrow(2, 0, sym_len_Ws).copy_(sym_patch_inv)
|
||||
|
||||
sym_patch = out_1[:, :, -sym_len_We:]
|
||||
inv_idx = torch.arange(sym_patch.size(2) - 1, -1, -1).long()
|
||||
sym_patch_inv = sym_patch.index_select(2, inv_idx)
|
||||
out_1_aug.narrow(2, sym_len_Ws + in_W, sym_len_We).copy_(sym_patch_inv)
|
||||
|
||||
out_2 = torch.FloatTensor(in_C, out_H, out_W)
|
||||
kernel_width = weights_W.size(1)
|
||||
for i in range(out_W):
|
||||
idx = int(indices_W[i][0])
|
||||
for j in range(out_C):
|
||||
out_2[j, :, i] = out_1_aug[j, :, idx : idx + kernel_width].mv(weights_W[i])
|
||||
if need_squeeze:
|
||||
out_2.squeeze_()
|
||||
return out_2
|
||||
|
||||
|
||||
# --------------------------------------------
|
||||
# imresize for numpy image [0, 1]
|
||||
# --------------------------------------------
|
||||
def imresize_np(img, scale, antialiasing=True):
|
||||
# Now the scale should be the same for H and W
|
||||
# input: img: Numpy, HWC or HW [0,1]
|
||||
# output: HWC or HW [0,1] w/o round
|
||||
img = torch.from_numpy(img)
|
||||
need_squeeze = True if img.dim() == 2 else False
|
||||
if need_squeeze:
|
||||
img.unsqueeze_(2)
|
||||
|
||||
in_H, in_W, in_C = img.size()
|
||||
out_C, out_H, out_W = (
|
||||
in_C,
|
||||
math.ceil(in_H * scale),
|
||||
math.ceil(in_W * scale),
|
||||
)
|
||||
kernel_width = 4
|
||||
kernel = "cubic"
|
||||
|
||||
# Return the desired dimension order for performing the resize. The
|
||||
# strategy is to perform the resize first along the dimension with the
|
||||
# smallest scale factor.
|
||||
# Now we do not support this.
|
||||
|
||||
# get weights and indices
|
||||
weights_H, indices_H, sym_len_Hs, sym_len_He = calculate_weights_indices(
|
||||
in_H, out_H, scale, kernel, kernel_width, antialiasing
|
||||
)
|
||||
weights_W, indices_W, sym_len_Ws, sym_len_We = calculate_weights_indices(
|
||||
in_W, out_W, scale, kernel, kernel_width, antialiasing
|
||||
)
|
||||
# process H dimension
|
||||
# symmetric copying
|
||||
img_aug = torch.FloatTensor(in_H + sym_len_Hs + sym_len_He, in_W, in_C)
|
||||
img_aug.narrow(0, sym_len_Hs, in_H).copy_(img)
|
||||
|
||||
sym_patch = img[:sym_len_Hs, :, :]
|
||||
inv_idx = torch.arange(sym_patch.size(0) - 1, -1, -1).long()
|
||||
sym_patch_inv = sym_patch.index_select(0, inv_idx)
|
||||
img_aug.narrow(0, 0, sym_len_Hs).copy_(sym_patch_inv)
|
||||
|
||||
sym_patch = img[-sym_len_He:, :, :]
|
||||
inv_idx = torch.arange(sym_patch.size(0) - 1, -1, -1).long()
|
||||
sym_patch_inv = sym_patch.index_select(0, inv_idx)
|
||||
img_aug.narrow(0, sym_len_Hs + in_H, sym_len_He).copy_(sym_patch_inv)
|
||||
|
||||
out_1 = torch.FloatTensor(out_H, in_W, in_C)
|
||||
kernel_width = weights_H.size(1)
|
||||
for i in range(out_H):
|
||||
idx = int(indices_H[i][0])
|
||||
for j in range(out_C):
|
||||
out_1[i, :, j] = img_aug[idx : idx + kernel_width, :, j].transpose(0, 1).mv(weights_H[i])
|
||||
|
||||
# process W dimension
|
||||
# symmetric copying
|
||||
out_1_aug = torch.FloatTensor(out_H, in_W + sym_len_Ws + sym_len_We, in_C)
|
||||
out_1_aug.narrow(1, sym_len_Ws, in_W).copy_(out_1)
|
||||
|
||||
sym_patch = out_1[:, :sym_len_Ws, :]
|
||||
inv_idx = torch.arange(sym_patch.size(1) - 1, -1, -1).long()
|
||||
sym_patch_inv = sym_patch.index_select(1, inv_idx)
|
||||
out_1_aug.narrow(1, 0, sym_len_Ws).copy_(sym_patch_inv)
|
||||
|
||||
sym_patch = out_1[:, -sym_len_We:, :]
|
||||
inv_idx = torch.arange(sym_patch.size(1) - 1, -1, -1).long()
|
||||
sym_patch_inv = sym_patch.index_select(1, inv_idx)
|
||||
out_1_aug.narrow(1, sym_len_Ws + in_W, sym_len_We).copy_(sym_patch_inv)
|
||||
|
||||
out_2 = torch.FloatTensor(out_H, out_W, in_C)
|
||||
kernel_width = weights_W.size(1)
|
||||
for i in range(out_W):
|
||||
idx = int(indices_W[i][0])
|
||||
for j in range(out_C):
|
||||
out_2[:, i, j] = out_1_aug[:, idx : idx + kernel_width, j].mv(weights_W[i])
|
||||
if need_squeeze:
|
||||
out_2.squeeze_()
|
||||
|
||||
return out_2.numpy()
|
||||
|
||||
|
||||
if __name__ == "__main__":
|
||||
print("---")
|
||||
# img = imread_uint('test.bmp', 3)
|
||||
# img = uint2single(img)
|
||||
# img_bicubic = imresize_np(img, 1/4)
|
@ -10,7 +10,6 @@ from .devices import ( # noqa: F401
|
||||
normalize_device,
|
||||
torch_dtype,
|
||||
)
|
||||
from .log import write_log # noqa: F401
|
||||
from .util import ( # noqa: F401
|
||||
ask_user,
|
||||
download_with_resume,
|
||||
|
@ -75,6 +75,7 @@
|
||||
"@reduxjs/toolkit": "^1.9.5",
|
||||
"@roarr/browser-log-writer": "^1.1.5",
|
||||
"@stevebel/png": "^1.5.1",
|
||||
"compare-versions": "^6.1.0",
|
||||
"dateformat": "^5.0.3",
|
||||
"formik": "^2.4.3",
|
||||
"framer-motion": "^10.16.1",
|
||||
|
@ -511,6 +511,7 @@
|
||||
"maskBlur": "Blur",
|
||||
"maskBlurMethod": "Blur Method",
|
||||
"coherencePassHeader": "Coherence Pass",
|
||||
"coherenceMode": "Mode",
|
||||
"coherenceSteps": "Steps",
|
||||
"coherenceStrength": "Strength",
|
||||
"seamLowThreshold": "Low",
|
||||
@ -520,6 +521,7 @@
|
||||
"scaledHeight": "Scaled H",
|
||||
"infillMethod": "Infill Method",
|
||||
"tileSize": "Tile Size",
|
||||
"patchmatchDownScaleSize": "Downscale",
|
||||
"boundingBoxHeader": "Bounding Box",
|
||||
"seamCorrectionHeader": "Seam Correction",
|
||||
"infillScalingHeader": "Infill and Scaling",
|
||||
|
@ -84,6 +84,7 @@ import { addUserInvokedCanvasListener } from './listeners/userInvokedCanvas';
|
||||
import { addUserInvokedImageToImageListener } from './listeners/userInvokedImageToImage';
|
||||
import { addUserInvokedNodesListener } from './listeners/userInvokedNodes';
|
||||
import { addUserInvokedTextToImageListener } from './listeners/userInvokedTextToImage';
|
||||
import { addWorkflowLoadedListener } from './listeners/workflowLoaded';
|
||||
|
||||
export const listenerMiddleware = createListenerMiddleware();
|
||||
|
||||
@ -202,6 +203,9 @@ addBoardIdSelectedListener();
|
||||
// Node schemas
|
||||
addReceivedOpenAPISchemaListener();
|
||||
|
||||
// Workflows
|
||||
addWorkflowLoadedListener();
|
||||
|
||||
// DND
|
||||
addImageDroppedListener();
|
||||
|
||||
|
@ -0,0 +1,55 @@
|
||||
import { logger } from 'app/logging/logger';
|
||||
import { workflowLoadRequested } from 'features/nodes/store/actions';
|
||||
import { workflowLoaded } from 'features/nodes/store/nodesSlice';
|
||||
import { $flow } from 'features/nodes/store/reactFlowInstance';
|
||||
import { validateWorkflow } from 'features/nodes/util/validateWorkflow';
|
||||
import { addToast } from 'features/system/store/systemSlice';
|
||||
import { makeToast } from 'features/system/util/makeToast';
|
||||
import { setActiveTab } from 'features/ui/store/uiSlice';
|
||||
import { startAppListening } from '..';
|
||||
|
||||
export const addWorkflowLoadedListener = () => {
|
||||
startAppListening({
|
||||
actionCreator: workflowLoadRequested,
|
||||
effect: (action, { dispatch, getState }) => {
|
||||
const log = logger('nodes');
|
||||
const workflow = action.payload;
|
||||
const nodeTemplates = getState().nodes.nodeTemplates;
|
||||
|
||||
const { workflow: validatedWorkflow, errors } = validateWorkflow(
|
||||
workflow,
|
||||
nodeTemplates
|
||||
);
|
||||
|
||||
dispatch(workflowLoaded(validatedWorkflow));
|
||||
|
||||
if (!errors.length) {
|
||||
dispatch(
|
||||
addToast(
|
||||
makeToast({
|
||||
title: 'Workflow Loaded',
|
||||
status: 'success',
|
||||
})
|
||||
)
|
||||
);
|
||||
} else {
|
||||
dispatch(
|
||||
addToast(
|
||||
makeToast({
|
||||
title: 'Workflow Loaded with Warnings',
|
||||
status: 'warning',
|
||||
})
|
||||
)
|
||||
);
|
||||
errors.forEach(({ message, ...rest }) => {
|
||||
log.warn(rest, message);
|
||||
});
|
||||
}
|
||||
|
||||
dispatch(setActiveTab('nodes'));
|
||||
requestAnimationFrame(() => {
|
||||
$flow.get()?.fitView();
|
||||
});
|
||||
},
|
||||
});
|
||||
};
|
@ -31,48 +31,54 @@ const selector = createSelector(
|
||||
reasons.push('No initial image selected');
|
||||
}
|
||||
|
||||
if (activeTabName === 'nodes' && nodes.shouldValidateGraph) {
|
||||
if (!nodes.nodes.length) {
|
||||
reasons.push('No nodes in graph');
|
||||
}
|
||||
|
||||
nodes.nodes.forEach((node) => {
|
||||
if (!isInvocationNode(node)) {
|
||||
return;
|
||||
if (activeTabName === 'nodes') {
|
||||
if (nodes.shouldValidateGraph) {
|
||||
if (!nodes.nodes.length) {
|
||||
reasons.push('No nodes in graph');
|
||||
}
|
||||
|
||||
const nodeTemplate = nodes.nodeTemplates[node.data.type];
|
||||
|
||||
if (!nodeTemplate) {
|
||||
// Node type not found
|
||||
reasons.push('Missing node template');
|
||||
return;
|
||||
}
|
||||
|
||||
const connectedEdges = getConnectedEdges([node], nodes.edges);
|
||||
|
||||
forEach(node.data.inputs, (field) => {
|
||||
const fieldTemplate = nodeTemplate.inputs[field.name];
|
||||
const hasConnection = connectedEdges.some(
|
||||
(edge) =>
|
||||
edge.target === node.id && edge.targetHandle === field.name
|
||||
);
|
||||
|
||||
if (!fieldTemplate) {
|
||||
reasons.push('Missing field template');
|
||||
nodes.nodes.forEach((node) => {
|
||||
if (!isInvocationNode(node)) {
|
||||
return;
|
||||
}
|
||||
|
||||
if (fieldTemplate.required && !field.value && !hasConnection) {
|
||||
reasons.push(
|
||||
`${node.data.label || nodeTemplate.title} -> ${
|
||||
field.label || fieldTemplate.title
|
||||
} missing input`
|
||||
const nodeTemplate = nodes.nodeTemplates[node.data.type];
|
||||
|
||||
if (!nodeTemplate) {
|
||||
// Node type not found
|
||||
reasons.push('Missing node template');
|
||||
return;
|
||||
}
|
||||
|
||||
const connectedEdges = getConnectedEdges([node], nodes.edges);
|
||||
|
||||
forEach(node.data.inputs, (field) => {
|
||||
const fieldTemplate = nodeTemplate.inputs[field.name];
|
||||
const hasConnection = connectedEdges.some(
|
||||
(edge) =>
|
||||
edge.target === node.id && edge.targetHandle === field.name
|
||||
);
|
||||
return;
|
||||
}
|
||||
|
||||
if (!fieldTemplate) {
|
||||
reasons.push('Missing field template');
|
||||
return;
|
||||
}
|
||||
|
||||
if (
|
||||
fieldTemplate.required &&
|
||||
field.value === undefined &&
|
||||
!hasConnection
|
||||
) {
|
||||
reasons.push(
|
||||
`${node.data.label || nodeTemplate.title} -> ${
|
||||
field.label || fieldTemplate.title
|
||||
} missing input`
|
||||
);
|
||||
return;
|
||||
}
|
||||
});
|
||||
});
|
||||
});
|
||||
}
|
||||
} else {
|
||||
if (!model) {
|
||||
reasons.push('No model selected');
|
||||
|
@ -1,2 +1,2 @@
|
||||
export const colorTokenToCssVar = (colorToken: string) =>
|
||||
`var(--invokeai-colors-${colorToken.split('.').join('-')}`;
|
||||
`var(--invokeai-colors-${colorToken.split('.').join('-')})`;
|
||||
|
@ -118,7 +118,11 @@ const IAICanvasToolChooserOptions = () => {
|
||||
useHotkeys(
|
||||
['BracketLeft'],
|
||||
() => {
|
||||
dispatch(setBrushSize(Math.max(brushSize - 5, 5)));
|
||||
if (brushSize - 5 <= 5) {
|
||||
dispatch(setBrushSize(Math.max(brushSize - 1, 1)));
|
||||
} else {
|
||||
dispatch(setBrushSize(Math.max(brushSize - 5, 1)));
|
||||
}
|
||||
},
|
||||
{
|
||||
enabled: () => !isStaging,
|
||||
|
@ -17,16 +17,13 @@ import { useAppDispatch, useAppSelector } from 'app/store/storeHooks';
|
||||
import IAIIconButton from 'common/components/IAIIconButton';
|
||||
import { DeleteImageButton } from 'features/deleteImageModal/components/DeleteImageButton';
|
||||
import { imagesToDeleteSelected } from 'features/deleteImageModal/store/slice';
|
||||
import { workflowLoaded } from 'features/nodes/store/nodesSlice';
|
||||
import { workflowLoadRequested } from 'features/nodes/store/actions';
|
||||
import ParamUpscalePopover from 'features/parameters/components/Parameters/Upscale/ParamUpscaleSettings';
|
||||
import { useRecallParameters } from 'features/parameters/hooks/useRecallParameters';
|
||||
import { initialImageSelected } from 'features/parameters/store/actions';
|
||||
import { useFeatureStatus } from 'features/system/hooks/useFeatureStatus';
|
||||
import { addToast } from 'features/system/store/systemSlice';
|
||||
import { makeToast } from 'features/system/util/makeToast';
|
||||
import { activeTabNameSelector } from 'features/ui/store/uiSelectors';
|
||||
import {
|
||||
setActiveTab,
|
||||
setShouldShowImageDetails,
|
||||
setShouldShowProgressInViewer,
|
||||
} from 'features/ui/store/uiSlice';
|
||||
@ -124,16 +121,7 @@ const CurrentImageButtons = (props: CurrentImageButtonsProps) => {
|
||||
if (!workflow) {
|
||||
return;
|
||||
}
|
||||
dispatch(workflowLoaded(workflow));
|
||||
dispatch(setActiveTab('nodes'));
|
||||
dispatch(
|
||||
addToast(
|
||||
makeToast({
|
||||
title: 'Workflow Loaded',
|
||||
status: 'success',
|
||||
})
|
||||
)
|
||||
);
|
||||
dispatch(workflowLoadRequested(workflow));
|
||||
}, [dispatch, workflow]);
|
||||
|
||||
const handleClickUseAllParameters = useCallback(() => {
|
||||
|
@ -7,12 +7,9 @@ import {
|
||||
isModalOpenChanged,
|
||||
} from 'features/changeBoardModal/store/slice';
|
||||
import { imagesToDeleteSelected } from 'features/deleteImageModal/store/slice';
|
||||
import { workflowLoaded } from 'features/nodes/store/nodesSlice';
|
||||
import { useRecallParameters } from 'features/parameters/hooks/useRecallParameters';
|
||||
import { initialImageSelected } from 'features/parameters/store/actions';
|
||||
import { useFeatureStatus } from 'features/system/hooks/useFeatureStatus';
|
||||
import { addToast } from 'features/system/store/systemSlice';
|
||||
import { makeToast } from 'features/system/util/makeToast';
|
||||
import { useCopyImageToClipboard } from 'features/ui/hooks/useCopyImageToClipboard';
|
||||
import { setActiveTab } from 'features/ui/store/uiSlice';
|
||||
import { memo, useCallback } from 'react';
|
||||
@ -36,6 +33,7 @@ import {
|
||||
} from 'services/api/endpoints/images';
|
||||
import { ImageDTO } from 'services/api/types';
|
||||
import { sentImageToCanvas, sentImageToImg2Img } from '../../store/actions';
|
||||
import { workflowLoadRequested } from 'features/nodes/store/actions';
|
||||
|
||||
type SingleSelectionMenuItemsProps = {
|
||||
imageDTO: ImageDTO;
|
||||
@ -102,16 +100,7 @@ const SingleSelectionMenuItems = (props: SingleSelectionMenuItemsProps) => {
|
||||
if (!workflow) {
|
||||
return;
|
||||
}
|
||||
dispatch(workflowLoaded(workflow));
|
||||
dispatch(setActiveTab('nodes'));
|
||||
dispatch(
|
||||
addToast(
|
||||
makeToast({
|
||||
title: 'Workflow Loaded',
|
||||
status: 'success',
|
||||
})
|
||||
)
|
||||
);
|
||||
dispatch(workflowLoadRequested(workflow));
|
||||
}, [dispatch, workflow]);
|
||||
|
||||
const handleSendToImageToImage = useCallback(() => {
|
||||
|
@ -3,6 +3,7 @@ import { createSelector } from '@reduxjs/toolkit';
|
||||
import { stateSelector } from 'app/store/store';
|
||||
import { useAppDispatch, useAppSelector } from 'app/store/storeHooks';
|
||||
import { defaultSelectorOptions } from 'app/store/util/defaultMemoizeOptions';
|
||||
import { $flow } from 'features/nodes/store/reactFlowInstance';
|
||||
import { contextMenusClosed } from 'features/ui/store/uiSlice';
|
||||
import { useCallback } from 'react';
|
||||
import { useHotkeys } from 'react-hotkeys-hook';
|
||||
@ -13,6 +14,7 @@ import {
|
||||
OnConnectStart,
|
||||
OnEdgesChange,
|
||||
OnEdgesDelete,
|
||||
OnInit,
|
||||
OnMoveEnd,
|
||||
OnNodesChange,
|
||||
OnNodesDelete,
|
||||
@ -147,6 +149,11 @@ export const Flow = () => {
|
||||
dispatch(contextMenusClosed());
|
||||
}, [dispatch]);
|
||||
|
||||
const onInit: OnInit = useCallback((flow) => {
|
||||
$flow.set(flow);
|
||||
flow.fitView();
|
||||
}, []);
|
||||
|
||||
useHotkeys(['Ctrl+c', 'Meta+c'], (e) => {
|
||||
e.preventDefault();
|
||||
dispatch(selectionCopied());
|
||||
@ -170,6 +177,7 @@ export const Flow = () => {
|
||||
edgeTypes={edgeTypes}
|
||||
nodes={nodes}
|
||||
edges={edges}
|
||||
onInit={onInit}
|
||||
onNodesChange={onNodesChange}
|
||||
onEdgesChange={onEdgesChange}
|
||||
onEdgesDelete={onEdgesDelete}
|
||||
|
@ -12,6 +12,7 @@ import {
|
||||
Tooltip,
|
||||
useDisclosure,
|
||||
} from '@chakra-ui/react';
|
||||
import { compare } from 'compare-versions';
|
||||
import { useNodeData } from 'features/nodes/hooks/useNodeData';
|
||||
import { useNodeLabel } from 'features/nodes/hooks/useNodeLabel';
|
||||
import { useNodeTemplate } from 'features/nodes/hooks/useNodeTemplate';
|
||||
@ -20,6 +21,7 @@ import { isInvocationNodeData } from 'features/nodes/types/types';
|
||||
import { memo, useMemo } from 'react';
|
||||
import { FaInfoCircle } from 'react-icons/fa';
|
||||
import NotesTextarea from './NotesTextarea';
|
||||
import { useDoNodeVersionsMatch } from 'features/nodes/hooks/useDoNodeVersionsMatch';
|
||||
|
||||
interface Props {
|
||||
nodeId: string;
|
||||
@ -29,6 +31,7 @@ const InvocationNodeNotes = ({ nodeId }: Props) => {
|
||||
const { isOpen, onOpen, onClose } = useDisclosure();
|
||||
const label = useNodeLabel(nodeId);
|
||||
const title = useNodeTemplateTitle(nodeId);
|
||||
const doVersionsMatch = useDoNodeVersionsMatch(nodeId);
|
||||
|
||||
return (
|
||||
<>
|
||||
@ -50,7 +53,11 @@ const InvocationNodeNotes = ({ nodeId }: Props) => {
|
||||
>
|
||||
<Icon
|
||||
as={FaInfoCircle}
|
||||
sx={{ boxSize: 4, w: 8, color: 'base.400' }}
|
||||
sx={{
|
||||
boxSize: 4,
|
||||
w: 8,
|
||||
color: doVersionsMatch ? 'base.400' : 'error.400',
|
||||
}}
|
||||
/>
|
||||
</Flex>
|
||||
</Tooltip>
|
||||
@ -92,16 +99,59 @@ const TooltipContent = memo(({ nodeId }: { nodeId: string }) => {
|
||||
return 'Unknown Node';
|
||||
}, [data, nodeTemplate]);
|
||||
|
||||
const versionComponent = useMemo(() => {
|
||||
if (!isInvocationNodeData(data) || !nodeTemplate) {
|
||||
return null;
|
||||
}
|
||||
|
||||
if (!data.version) {
|
||||
return (
|
||||
<Text as="span" sx={{ color: 'error.500' }}>
|
||||
Version unknown
|
||||
</Text>
|
||||
);
|
||||
}
|
||||
|
||||
if (!nodeTemplate.version) {
|
||||
return (
|
||||
<Text as="span" sx={{ color: 'error.500' }}>
|
||||
Version {data.version} (unknown template)
|
||||
</Text>
|
||||
);
|
||||
}
|
||||
|
||||
if (compare(data.version, nodeTemplate.version, '<')) {
|
||||
return (
|
||||
<Text as="span" sx={{ color: 'error.500' }}>
|
||||
Version {data.version} (update node)
|
||||
</Text>
|
||||
);
|
||||
}
|
||||
|
||||
if (compare(data.version, nodeTemplate.version, '>')) {
|
||||
return (
|
||||
<Text as="span" sx={{ color: 'error.500' }}>
|
||||
Version {data.version} (update app)
|
||||
</Text>
|
||||
);
|
||||
}
|
||||
|
||||
return <Text as="span">Version {data.version}</Text>;
|
||||
}, [data, nodeTemplate]);
|
||||
|
||||
if (!isInvocationNodeData(data)) {
|
||||
return <Text sx={{ fontWeight: 600 }}>Unknown Node</Text>;
|
||||
}
|
||||
|
||||
return (
|
||||
<Flex sx={{ flexDir: 'column' }}>
|
||||
<Text sx={{ fontWeight: 600 }}>{title}</Text>
|
||||
<Text as="span" sx={{ fontWeight: 600 }}>
|
||||
{title}
|
||||
</Text>
|
||||
<Text sx={{ opacity: 0.7, fontStyle: 'oblique 5deg' }}>
|
||||
{nodeTemplate?.description}
|
||||
</Text>
|
||||
{versionComponent}
|
||||
{data?.notes && <Text>{data.notes}</Text>}
|
||||
</Flex>
|
||||
);
|
||||
|
@ -1,8 +1,11 @@
|
||||
import { Tooltip } from '@chakra-ui/react';
|
||||
import { colorTokenToCssVar } from 'common/util/colorTokenToCssVar';
|
||||
import {
|
||||
COLLECTION_TYPES,
|
||||
FIELDS,
|
||||
HANDLE_TOOLTIP_OPEN_DELAY,
|
||||
MODEL_TYPES,
|
||||
POLYMORPHIC_TYPES,
|
||||
} from 'features/nodes/types/constants';
|
||||
import {
|
||||
InputFieldTemplate,
|
||||
@ -18,6 +21,7 @@ export const handleBaseStyles: CSSProperties = {
|
||||
borderWidth: 0,
|
||||
zIndex: 1,
|
||||
};
|
||||
``;
|
||||
|
||||
export const inputHandleStyles: CSSProperties = {
|
||||
left: '-1rem',
|
||||
@ -44,15 +48,25 @@ const FieldHandle = (props: FieldHandleProps) => {
|
||||
connectionError,
|
||||
} = props;
|
||||
const { name, type } = fieldTemplate;
|
||||
const { color, title } = FIELDS[type];
|
||||
const { color: typeColor, title } = FIELDS[type];
|
||||
|
||||
const styles: CSSProperties = useMemo(() => {
|
||||
const isCollectionType = COLLECTION_TYPES.includes(type);
|
||||
const isPolymorphicType = POLYMORPHIC_TYPES.includes(type);
|
||||
const isModelType = MODEL_TYPES.includes(type);
|
||||
const color = colorTokenToCssVar(typeColor);
|
||||
const s: CSSProperties = {
|
||||
backgroundColor: colorTokenToCssVar(color),
|
||||
backgroundColor:
|
||||
isCollectionType || isPolymorphicType
|
||||
? 'var(--invokeai-colors-base-900)'
|
||||
: color,
|
||||
position: 'absolute',
|
||||
width: '1rem',
|
||||
height: '1rem',
|
||||
borderWidth: 0,
|
||||
borderWidth: isCollectionType || isPolymorphicType ? 4 : 0,
|
||||
borderStyle: 'solid',
|
||||
borderColor: color,
|
||||
borderRadius: isModelType ? 4 : '100%',
|
||||
zIndex: 1,
|
||||
};
|
||||
|
||||
@ -78,11 +92,12 @@ const FieldHandle = (props: FieldHandleProps) => {
|
||||
|
||||
return s;
|
||||
}, [
|
||||
color,
|
||||
connectionError,
|
||||
handleType,
|
||||
isConnectionInProgress,
|
||||
isConnectionStartField,
|
||||
type,
|
||||
typeColor,
|
||||
]);
|
||||
|
||||
const tooltip = useMemo(() => {
|
||||
|
@ -75,6 +75,7 @@ const InputField = ({ nodeId, fieldName }: Props) => {
|
||||
sx={{
|
||||
display: 'flex',
|
||||
alignItems: 'center',
|
||||
h: 'full',
|
||||
mb: 0,
|
||||
px: 1,
|
||||
gap: 2,
|
||||
|
@ -3,18 +3,10 @@ import { useFieldData } from 'features/nodes/hooks/useFieldData';
|
||||
import { useFieldTemplate } from 'features/nodes/hooks/useFieldTemplate';
|
||||
import { memo } from 'react';
|
||||
import BooleanInputField from './inputs/BooleanInputField';
|
||||
import ClipInputField from './inputs/ClipInputField';
|
||||
import CollectionInputField from './inputs/CollectionInputField';
|
||||
import CollectionItemInputField from './inputs/CollectionItemInputField';
|
||||
import ColorInputField from './inputs/ColorInputField';
|
||||
import ConditioningInputField from './inputs/ConditioningInputField';
|
||||
import ControlInputField from './inputs/ControlInputField';
|
||||
import ControlNetModelInputField from './inputs/ControlNetModelInputField';
|
||||
import DenoiseMaskInputField from './inputs/DenoiseMaskInputField';
|
||||
import EnumInputField from './inputs/EnumInputField';
|
||||
import ImageCollectionInputField from './inputs/ImageCollectionInputField';
|
||||
import ImageInputField from './inputs/ImageInputField';
|
||||
import LatentsInputField from './inputs/LatentsInputField';
|
||||
import LoRAModelInputField from './inputs/LoRAModelInputField';
|
||||
import MainModelInputField from './inputs/MainModelInputField';
|
||||
import NumberInputField from './inputs/NumberInputField';
|
||||
@ -22,8 +14,6 @@ import RefinerModelInputField from './inputs/RefinerModelInputField';
|
||||
import SDXLMainModelInputField from './inputs/SDXLMainModelInputField';
|
||||
import SchedulerInputField from './inputs/SchedulerInputField';
|
||||
import StringInputField from './inputs/StringInputField';
|
||||
import UnetInputField from './inputs/UnetInputField';
|
||||
import VaeInputField from './inputs/VaeInputField';
|
||||
import VaeModelInputField from './inputs/VaeModelInputField';
|
||||
|
||||
type InputFieldProps = {
|
||||
@ -31,7 +21,6 @@ type InputFieldProps = {
|
||||
fieldName: string;
|
||||
};
|
||||
|
||||
// build an individual input element based on the schema
|
||||
const InputFieldRenderer = ({ nodeId, fieldName }: InputFieldProps) => {
|
||||
const field = useFieldData(nodeId, fieldName);
|
||||
const fieldTemplate = useFieldTemplate(nodeId, fieldName, 'input');
|
||||
@ -93,88 +82,6 @@ const InputFieldRenderer = ({ nodeId, fieldName }: InputFieldProps) => {
|
||||
);
|
||||
}
|
||||
|
||||
if (
|
||||
field?.type === 'LatentsField' &&
|
||||
fieldTemplate?.type === 'LatentsField'
|
||||
) {
|
||||
return (
|
||||
<LatentsInputField
|
||||
nodeId={nodeId}
|
||||
field={field}
|
||||
fieldTemplate={fieldTemplate}
|
||||
/>
|
||||
);
|
||||
}
|
||||
|
||||
if (
|
||||
field?.type === 'DenoiseMaskField' &&
|
||||
fieldTemplate?.type === 'DenoiseMaskField'
|
||||
) {
|
||||
return (
|
||||
<DenoiseMaskInputField
|
||||
nodeId={nodeId}
|
||||
field={field}
|
||||
fieldTemplate={fieldTemplate}
|
||||
/>
|
||||
);
|
||||
}
|
||||
|
||||
if (
|
||||
field?.type === 'ConditioningField' &&
|
||||
fieldTemplate?.type === 'ConditioningField'
|
||||
) {
|
||||
return (
|
||||
<ConditioningInputField
|
||||
nodeId={nodeId}
|
||||
field={field}
|
||||
fieldTemplate={fieldTemplate}
|
||||
/>
|
||||
);
|
||||
}
|
||||
|
||||
if (field?.type === 'UNetField' && fieldTemplate?.type === 'UNetField') {
|
||||
return (
|
||||
<UnetInputField
|
||||
nodeId={nodeId}
|
||||
field={field}
|
||||
fieldTemplate={fieldTemplate}
|
||||
/>
|
||||
);
|
||||
}
|
||||
|
||||
if (field?.type === 'ClipField' && fieldTemplate?.type === 'ClipField') {
|
||||
return (
|
||||
<ClipInputField
|
||||
nodeId={nodeId}
|
||||
field={field}
|
||||
fieldTemplate={fieldTemplate}
|
||||
/>
|
||||
);
|
||||
}
|
||||
|
||||
if (field?.type === 'VaeField' && fieldTemplate?.type === 'VaeField') {
|
||||
return (
|
||||
<VaeInputField
|
||||
nodeId={nodeId}
|
||||
field={field}
|
||||
fieldTemplate={fieldTemplate}
|
||||
/>
|
||||
);
|
||||
}
|
||||
|
||||
if (
|
||||
field?.type === 'ControlField' &&
|
||||
fieldTemplate?.type === 'ControlField'
|
||||
) {
|
||||
return (
|
||||
<ControlInputField
|
||||
nodeId={nodeId}
|
||||
field={field}
|
||||
fieldTemplate={fieldTemplate}
|
||||
/>
|
||||
);
|
||||
}
|
||||
|
||||
if (
|
||||
field?.type === 'MainModelField' &&
|
||||
fieldTemplate?.type === 'MainModelField'
|
||||
@ -240,29 +147,6 @@ const InputFieldRenderer = ({ nodeId, fieldName }: InputFieldProps) => {
|
||||
);
|
||||
}
|
||||
|
||||
if (field?.type === 'Collection' && fieldTemplate?.type === 'Collection') {
|
||||
return (
|
||||
<CollectionInputField
|
||||
nodeId={nodeId}
|
||||
field={field}
|
||||
fieldTemplate={fieldTemplate}
|
||||
/>
|
||||
);
|
||||
}
|
||||
|
||||
if (
|
||||
field?.type === 'CollectionItem' &&
|
||||
fieldTemplate?.type === 'CollectionItem'
|
||||
) {
|
||||
return (
|
||||
<CollectionItemInputField
|
||||
nodeId={nodeId}
|
||||
field={field}
|
||||
fieldTemplate={fieldTemplate}
|
||||
/>
|
||||
);
|
||||
}
|
||||
|
||||
if (field?.type === 'ColorField' && fieldTemplate?.type === 'ColorField') {
|
||||
return (
|
||||
<ColorInputField
|
||||
@ -273,19 +157,6 @@ const InputFieldRenderer = ({ nodeId, fieldName }: InputFieldProps) => {
|
||||
);
|
||||
}
|
||||
|
||||
if (
|
||||
field?.type === 'ImageCollection' &&
|
||||
fieldTemplate?.type === 'ImageCollection'
|
||||
) {
|
||||
return (
|
||||
<ImageCollectionInputField
|
||||
nodeId={nodeId}
|
||||
field={field}
|
||||
fieldTemplate={fieldTemplate}
|
||||
/>
|
||||
);
|
||||
}
|
||||
|
||||
if (
|
||||
field?.type === 'SDXLMainModelField' &&
|
||||
fieldTemplate?.type === 'SDXLMainModelField'
|
||||
@ -309,6 +180,11 @@ const InputFieldRenderer = ({ nodeId, fieldName }: InputFieldProps) => {
|
||||
);
|
||||
}
|
||||
|
||||
if (field && fieldTemplate) {
|
||||
// Fallback for when there is no component for the type
|
||||
return null;
|
||||
}
|
||||
|
||||
return (
|
||||
<Box p={1}>
|
||||
<Text
|
||||
|
@ -1,12 +1,17 @@
|
||||
import {
|
||||
ControlInputFieldTemplate,
|
||||
ControlInputFieldValue,
|
||||
ControlPolymorphicInputFieldTemplate,
|
||||
ControlPolymorphicInputFieldValue,
|
||||
FieldComponentProps,
|
||||
} from 'features/nodes/types/types';
|
||||
import { memo } from 'react';
|
||||
|
||||
const ControlInputFieldComponent = (
|
||||
_props: FieldComponentProps<ControlInputFieldValue, ControlInputFieldTemplate>
|
||||
_props: FieldComponentProps<
|
||||
ControlInputFieldValue | ControlPolymorphicInputFieldValue,
|
||||
ControlInputFieldTemplate | ControlPolymorphicInputFieldTemplate
|
||||
>
|
||||
) => {
|
||||
return null;
|
||||
};
|
||||
|
@ -9,9 +9,9 @@ import {
|
||||
} from 'features/dnd/types';
|
||||
import { fieldImageValueChanged } from 'features/nodes/store/nodesSlice';
|
||||
import {
|
||||
FieldComponentProps,
|
||||
ImageInputFieldTemplate,
|
||||
ImageInputFieldValue,
|
||||
FieldComponentProps,
|
||||
} from 'features/nodes/types/types';
|
||||
import { memo, useCallback, useMemo } from 'react';
|
||||
import { FaUndo } from 'react-icons/fa';
|
||||
|
@ -2,11 +2,16 @@ import {
|
||||
LatentsInputFieldTemplate,
|
||||
LatentsInputFieldValue,
|
||||
FieldComponentProps,
|
||||
LatentsPolymorphicInputFieldValue,
|
||||
LatentsPolymorphicInputFieldTemplate,
|
||||
} from 'features/nodes/types/types';
|
||||
import { memo } from 'react';
|
||||
|
||||
const LatentsInputFieldComponent = (
|
||||
_props: FieldComponentProps<LatentsInputFieldValue, LatentsInputFieldTemplate>
|
||||
_props: FieldComponentProps<
|
||||
LatentsInputFieldValue | LatentsPolymorphicInputFieldValue,
|
||||
LatentsInputFieldTemplate | LatentsPolymorphicInputFieldTemplate
|
||||
>
|
||||
) => {
|
||||
return null;
|
||||
};
|
||||
|
@ -9,11 +9,11 @@ import { useAppDispatch } from 'app/store/storeHooks';
|
||||
import { numberStringRegex } from 'common/components/IAINumberInput';
|
||||
import { fieldNumberValueChanged } from 'features/nodes/store/nodesSlice';
|
||||
import {
|
||||
FieldComponentProps,
|
||||
FloatInputFieldTemplate,
|
||||
FloatInputFieldValue,
|
||||
IntegerInputFieldTemplate,
|
||||
IntegerInputFieldValue,
|
||||
FieldComponentProps,
|
||||
} from 'features/nodes/types/types';
|
||||
import { memo, useEffect, useMemo, useState } from 'react';
|
||||
|
||||
|
@ -9,13 +9,20 @@ import { stateSelector } from 'app/store/store';
|
||||
import { useAppDispatch, useAppSelector } from 'app/store/storeHooks';
|
||||
import NodeSelectionOverlay from 'common/components/NodeSelectionOverlay';
|
||||
import { useMouseOverNode } from 'features/nodes/hooks/useMouseOverNode';
|
||||
import { nodeExclusivelySelected } from 'features/nodes/store/nodesSlice';
|
||||
import {
|
||||
DRAG_HANDLE_CLASSNAME,
|
||||
NODE_WIDTH,
|
||||
} from 'features/nodes/types/constants';
|
||||
import { NodeStatus } from 'features/nodes/types/types';
|
||||
import { contextMenusClosed } from 'features/ui/store/uiSlice';
|
||||
import { PropsWithChildren, memo, useCallback, useMemo } from 'react';
|
||||
import {
|
||||
MouseEvent,
|
||||
PropsWithChildren,
|
||||
memo,
|
||||
useCallback,
|
||||
useMemo,
|
||||
} from 'react';
|
||||
|
||||
type NodeWrapperProps = PropsWithChildren & {
|
||||
nodeId: string;
|
||||
@ -57,9 +64,15 @@ const NodeWrapper = (props: NodeWrapperProps) => {
|
||||
|
||||
const opacity = useAppSelector((state) => state.nodes.nodeOpacity);
|
||||
|
||||
const handleClick = useCallback(() => {
|
||||
dispatch(contextMenusClosed());
|
||||
}, [dispatch]);
|
||||
const handleClick = useCallback(
|
||||
(e: MouseEvent<HTMLDivElement>) => {
|
||||
if (!e.ctrlKey && !e.altKey && !e.metaKey && !e.shiftKey) {
|
||||
dispatch(nodeExclusivelySelected(nodeId));
|
||||
}
|
||||
dispatch(contextMenusClosed());
|
||||
},
|
||||
[dispatch, nodeId]
|
||||
);
|
||||
|
||||
return (
|
||||
<Box
|
||||
|
@ -138,13 +138,14 @@ export const useBuildNodeData = () => {
|
||||
data: {
|
||||
id: nodeId,
|
||||
type,
|
||||
inputs,
|
||||
outputs,
|
||||
isOpen: true,
|
||||
version: template.version,
|
||||
label: '',
|
||||
notes: '',
|
||||
isOpen: true,
|
||||
embedWorkflow: false,
|
||||
isIntermediate: true,
|
||||
inputs,
|
||||
outputs,
|
||||
},
|
||||
};
|
||||
|
||||
|
@ -0,0 +1,33 @@
|
||||
import { createSelector } from '@reduxjs/toolkit';
|
||||
import { stateSelector } from 'app/store/store';
|
||||
import { useAppSelector } from 'app/store/storeHooks';
|
||||
import { defaultSelectorOptions } from 'app/store/util/defaultMemoizeOptions';
|
||||
import { compareVersions } from 'compare-versions';
|
||||
import { useMemo } from 'react';
|
||||
import { isInvocationNode } from '../types/types';
|
||||
|
||||
export const useDoNodeVersionsMatch = (nodeId: string) => {
|
||||
const selector = useMemo(
|
||||
() =>
|
||||
createSelector(
|
||||
stateSelector,
|
||||
({ nodes }) => {
|
||||
const node = nodes.nodes.find((node) => node.id === nodeId);
|
||||
if (!isInvocationNode(node)) {
|
||||
return false;
|
||||
}
|
||||
const nodeTemplate = nodes.nodeTemplates[node?.data.type ?? ''];
|
||||
if (!nodeTemplate?.version || !node.data?.version) {
|
||||
return false;
|
||||
}
|
||||
return compareVersions(nodeTemplate.version, node.data.version) === 0;
|
||||
},
|
||||
defaultSelectorOptions
|
||||
),
|
||||
[nodeId]
|
||||
);
|
||||
|
||||
const nodeTemplate = useAppSelector(selector);
|
||||
|
||||
return nodeTemplate;
|
||||
};
|
@ -15,7 +15,7 @@ export const useDoesInputHaveValue = (nodeId: string, fieldName: string) => {
|
||||
if (!isInvocationNode(node)) {
|
||||
return;
|
||||
}
|
||||
return Boolean(node?.data.inputs[fieldName]?.value);
|
||||
return node?.data.inputs[fieldName]?.value !== undefined;
|
||||
},
|
||||
defaultSelectorOptions
|
||||
),
|
||||
|
@ -3,9 +3,19 @@ import graphlib from '@dagrejs/graphlib';
|
||||
import { useAppSelector } from 'app/store/storeHooks';
|
||||
import { useCallback } from 'react';
|
||||
import { Connection, Edge, Node, useReactFlow } from 'reactflow';
|
||||
import { COLLECTION_TYPES } from '../types/constants';
|
||||
import {
|
||||
COLLECTION_MAP,
|
||||
COLLECTION_TYPES,
|
||||
POLYMORPHIC_TO_SINGLE_MAP,
|
||||
POLYMORPHIC_TYPES,
|
||||
} from '../types/constants';
|
||||
import { InvocationNodeData } from '../types/types';
|
||||
|
||||
/**
|
||||
* NOTE: The logic here must be duplicated in `invokeai/frontend/web/src/features/nodes/store/util/makeIsConnectionValidSelector.ts`
|
||||
* TODO: Figure out how to do this without duplicating all the logic
|
||||
*/
|
||||
|
||||
export const useIsValidConnection = () => {
|
||||
const flow = useReactFlow();
|
||||
const shouldValidateGraph = useAppSelector(
|
||||
@ -42,6 +52,19 @@ export const useIsValidConnection = () => {
|
||||
return false;
|
||||
}
|
||||
|
||||
if (
|
||||
edges
|
||||
.filter((edge) => {
|
||||
return edge.target === target && edge.targetHandle === targetHandle;
|
||||
})
|
||||
.find((edge) => {
|
||||
edge.source === source && edge.sourceHandle === sourceHandle;
|
||||
})
|
||||
) {
|
||||
// We already have a connection from this source to this target
|
||||
return false;
|
||||
}
|
||||
|
||||
// Connection is invalid if target already has a connection
|
||||
if (
|
||||
edges.find((edge) => {
|
||||
@ -53,21 +76,62 @@ export const useIsValidConnection = () => {
|
||||
return false;
|
||||
}
|
||||
|
||||
// Connection types must be the same for a connection
|
||||
if (
|
||||
sourceType !== targetType &&
|
||||
sourceType !== 'CollectionItem' &&
|
||||
targetType !== 'CollectionItem'
|
||||
) {
|
||||
if (
|
||||
!(
|
||||
COLLECTION_TYPES.includes(targetType) &&
|
||||
COLLECTION_TYPES.includes(sourceType)
|
||||
)
|
||||
) {
|
||||
return false;
|
||||
}
|
||||
/**
|
||||
* Connection types must be the same for a connection, with exceptions:
|
||||
* - CollectionItem can connect to any non-Collection
|
||||
* - Non-Collections can connect to CollectionItem
|
||||
* - Anything (non-Collections, Collections, Polymorphics) can connect to Polymorphics of the same base type
|
||||
* - Generic Collection can connect to any other Collection or Polymorphic
|
||||
* - Any Collection can connect to a Generic Collection
|
||||
*/
|
||||
|
||||
if (sourceType !== targetType) {
|
||||
const isCollectionItemToNonCollection =
|
||||
sourceType === 'CollectionItem' &&
|
||||
!COLLECTION_TYPES.includes(targetType);
|
||||
|
||||
const isNonCollectionToCollectionItem =
|
||||
targetType === 'CollectionItem' &&
|
||||
!COLLECTION_TYPES.includes(sourceType) &&
|
||||
!POLYMORPHIC_TYPES.includes(sourceType);
|
||||
|
||||
const isAnythingToPolymorphicOfSameBaseType =
|
||||
POLYMORPHIC_TYPES.includes(targetType) &&
|
||||
(() => {
|
||||
if (!POLYMORPHIC_TYPES.includes(targetType)) {
|
||||
return false;
|
||||
}
|
||||
const baseType =
|
||||
POLYMORPHIC_TO_SINGLE_MAP[
|
||||
targetType as keyof typeof POLYMORPHIC_TO_SINGLE_MAP
|
||||
];
|
||||
|
||||
const collectionType =
|
||||
COLLECTION_MAP[baseType as keyof typeof COLLECTION_MAP];
|
||||
|
||||
return sourceType === baseType || sourceType === collectionType;
|
||||
})();
|
||||
|
||||
const isGenericCollectionToAnyCollectionOrPolymorphic =
|
||||
sourceType === 'Collection' &&
|
||||
(COLLECTION_TYPES.includes(targetType) ||
|
||||
POLYMORPHIC_TYPES.includes(targetType));
|
||||
|
||||
const isCollectionToGenericCollection =
|
||||
targetType === 'Collection' && COLLECTION_TYPES.includes(sourceType);
|
||||
|
||||
const isIntToFloat = sourceType === 'integer' && targetType === 'float';
|
||||
|
||||
return (
|
||||
isCollectionItemToNonCollection ||
|
||||
isNonCollectionToCollectionItem ||
|
||||
isAnythingToPolymorphicOfSameBaseType ||
|
||||
isGenericCollectionToAnyCollectionOrPolymorphic ||
|
||||
isCollectionToGenericCollection ||
|
||||
isIntToFloat
|
||||
);
|
||||
}
|
||||
|
||||
// Graphs much be acyclic (no loops!)
|
||||
return getIsGraphAcyclic(source, target, nodes, edges);
|
||||
},
|
||||
|
@ -2,13 +2,13 @@ import { ListItem, Text, UnorderedList } from '@chakra-ui/react';
|
||||
import { useLogger } from 'app/logging/useLogger';
|
||||
import { useAppDispatch } from 'app/store/storeHooks';
|
||||
import { parseify } from 'common/util/serialize';
|
||||
import { workflowLoaded } from 'features/nodes/store/nodesSlice';
|
||||
import { zValidatedWorkflow } from 'features/nodes/types/types';
|
||||
import { zWorkflow } from 'features/nodes/types/types';
|
||||
import { addToast } from 'features/system/store/systemSlice';
|
||||
import { makeToast } from 'features/system/util/makeToast';
|
||||
import { memo, useCallback } from 'react';
|
||||
import { ZodError } from 'zod';
|
||||
import { fromZodError, fromZodIssue } from 'zod-validation-error';
|
||||
import { workflowLoadRequested } from '../store/actions';
|
||||
|
||||
export const useLoadWorkflowFromFile = () => {
|
||||
const dispatch = useAppDispatch();
|
||||
@ -24,7 +24,7 @@ export const useLoadWorkflowFromFile = () => {
|
||||
|
||||
try {
|
||||
const parsedJSON = JSON.parse(String(rawJSON));
|
||||
const result = zValidatedWorkflow.safeParse(parsedJSON);
|
||||
const result = zWorkflow.safeParse(parsedJSON);
|
||||
|
||||
if (!result.success) {
|
||||
const { message } = fromZodError(result.error, {
|
||||
@ -45,32 +45,8 @@ export const useLoadWorkflowFromFile = () => {
|
||||
reader.abort();
|
||||
return;
|
||||
}
|
||||
dispatch(workflowLoaded(result.data.workflow));
|
||||
|
||||
if (!result.data.warnings.length) {
|
||||
dispatch(
|
||||
addToast(
|
||||
makeToast({
|
||||
title: 'Workflow Loaded',
|
||||
status: 'success',
|
||||
})
|
||||
)
|
||||
);
|
||||
reader.abort();
|
||||
return;
|
||||
}
|
||||
|
||||
dispatch(
|
||||
addToast(
|
||||
makeToast({
|
||||
title: 'Workflow Loaded with Warnings',
|
||||
status: 'warning',
|
||||
})
|
||||
)
|
||||
);
|
||||
result.data.warnings.forEach(({ message, ...rest }) => {
|
||||
logger.warn(rest, message);
|
||||
});
|
||||
dispatch(workflowLoadRequested(result.data));
|
||||
|
||||
reader.abort();
|
||||
} catch {
|
||||
|
@ -1,5 +1,6 @@
|
||||
import { createAction, isAnyOf } from '@reduxjs/toolkit';
|
||||
import { Graph } from 'services/api/types';
|
||||
import { Workflow } from '../types/types';
|
||||
|
||||
export const textToImageGraphBuilt = createAction<Graph>(
|
||||
'nodes/textToImageGraphBuilt'
|
||||
@ -16,3 +17,7 @@ export const isAnyGraphBuilt = isAnyOf(
|
||||
canvasGraphBuilt,
|
||||
nodesGraphBuilt
|
||||
);
|
||||
|
||||
export const workflowLoadRequested = createAction<Workflow>(
|
||||
'nodes/workflowLoadRequested'
|
||||
);
|
||||
|
@ -443,6 +443,17 @@ const nodesSlice = createSlice({
|
||||
}
|
||||
node.data.notes = notes;
|
||||
},
|
||||
nodeExclusivelySelected: (state, action: PayloadAction<string>) => {
|
||||
const nodeId = action.payload;
|
||||
state.nodes = applyNodeChanges(
|
||||
state.nodes.map((n) => ({
|
||||
id: n.id,
|
||||
type: 'select',
|
||||
selected: n.id === nodeId ? true : false,
|
||||
})),
|
||||
state.nodes
|
||||
);
|
||||
},
|
||||
selectedNodesChanged: (state, action: PayloadAction<string[]>) => {
|
||||
state.selectedNodes = action.payload;
|
||||
},
|
||||
@ -892,6 +903,7 @@ export const {
|
||||
nodeEmbedWorkflowChanged,
|
||||
nodeIsIntermediateChanged,
|
||||
mouseOverNodeChanged,
|
||||
nodeExclusivelySelected,
|
||||
} = nodesSlice.actions;
|
||||
|
||||
export default nodesSlice.reducer;
|
||||
|
@ -0,0 +1,4 @@
|
||||
import { atom } from 'nanostores';
|
||||
import { ReactFlowInstance } from 'reactflow';
|
||||
|
||||
export const $flow = atom<ReactFlowInstance | null>(null);
|
@ -1,10 +1,20 @@
|
||||
import { createSelector } from '@reduxjs/toolkit';
|
||||
import { stateSelector } from 'app/store/store';
|
||||
import { getIsGraphAcyclic } from 'features/nodes/hooks/useIsValidConnection';
|
||||
import { COLLECTION_TYPES } from 'features/nodes/types/constants';
|
||||
import {
|
||||
COLLECTION_MAP,
|
||||
COLLECTION_TYPES,
|
||||
POLYMORPHIC_TO_SINGLE_MAP,
|
||||
POLYMORPHIC_TYPES,
|
||||
} from 'features/nodes/types/constants';
|
||||
import { FieldType } from 'features/nodes/types/types';
|
||||
import { HandleType } from 'reactflow';
|
||||
|
||||
/**
|
||||
* NOTE: The logic here must be duplicated in `invokeai/frontend/web/src/features/nodes/hooks/useIsValidConnection.ts`
|
||||
* TODO: Figure out how to do this without duplicating all the logic
|
||||
*/
|
||||
|
||||
export const makeConnectionErrorSelector = (
|
||||
nodeId: string,
|
||||
fieldName: string,
|
||||
@ -19,11 +29,6 @@ export const makeConnectionErrorSelector = (
|
||||
const { currentConnectionFieldType, connectionStartParams, nodes, edges } =
|
||||
state.nodes;
|
||||
|
||||
if (!state.nodes.shouldValidateGraph) {
|
||||
// manual override!
|
||||
return null;
|
||||
}
|
||||
|
||||
if (!connectionStartParams || !currentConnectionFieldType) {
|
||||
return 'No connection in progress';
|
||||
}
|
||||
@ -38,9 +43,9 @@ export const makeConnectionErrorSelector = (
|
||||
return 'No connection data';
|
||||
}
|
||||
|
||||
const targetFieldType =
|
||||
const targetType =
|
||||
handleType === 'target' ? fieldType : currentConnectionFieldType;
|
||||
const sourceFieldType =
|
||||
const sourceType =
|
||||
handleType === 'source' ? fieldType : currentConnectionFieldType;
|
||||
|
||||
if (nodeId === connectionNodeId) {
|
||||
@ -55,30 +60,73 @@ export const makeConnectionErrorSelector = (
|
||||
}
|
||||
|
||||
if (
|
||||
fieldType !== currentConnectionFieldType &&
|
||||
fieldType !== 'CollectionItem' &&
|
||||
currentConnectionFieldType !== 'CollectionItem'
|
||||
) {
|
||||
if (
|
||||
!(
|
||||
COLLECTION_TYPES.includes(targetFieldType) &&
|
||||
COLLECTION_TYPES.includes(sourceFieldType)
|
||||
)
|
||||
) {
|
||||
// except for collection items, field types must match
|
||||
return 'Field types must match';
|
||||
}
|
||||
}
|
||||
|
||||
if (
|
||||
handleType === 'target' &&
|
||||
edges.find((edge) => {
|
||||
return edge.target === nodeId && edge.targetHandle === fieldName;
|
||||
}) &&
|
||||
// except CollectionItem inputs can have multiples
|
||||
targetFieldType !== 'CollectionItem'
|
||||
targetType !== 'CollectionItem'
|
||||
) {
|
||||
return 'Inputs may only have one connection';
|
||||
return 'Input may only have one connection';
|
||||
}
|
||||
|
||||
/**
|
||||
* Connection types must be the same for a connection, with exceptions:
|
||||
* - CollectionItem can connect to any non-Collection
|
||||
* - Non-Collections can connect to CollectionItem
|
||||
* - Anything (non-Collections, Collections, Polymorphics) can connect to Polymorphics of the same base type
|
||||
* - Generic Collection can connect to any other Collection or Polymorphic
|
||||
* - Any Collection can connect to a Generic Collection
|
||||
*/
|
||||
|
||||
if (sourceType !== targetType) {
|
||||
const isCollectionItemToNonCollection =
|
||||
sourceType === 'CollectionItem' &&
|
||||
!COLLECTION_TYPES.includes(targetType);
|
||||
|
||||
const isNonCollectionToCollectionItem =
|
||||
targetType === 'CollectionItem' &&
|
||||
!COLLECTION_TYPES.includes(sourceType) &&
|
||||
!POLYMORPHIC_TYPES.includes(sourceType);
|
||||
|
||||
const isAnythingToPolymorphicOfSameBaseType =
|
||||
POLYMORPHIC_TYPES.includes(targetType) &&
|
||||
(() => {
|
||||
if (!POLYMORPHIC_TYPES.includes(targetType)) {
|
||||
return false;
|
||||
}
|
||||
const baseType =
|
||||
POLYMORPHIC_TO_SINGLE_MAP[
|
||||
targetType as keyof typeof POLYMORPHIC_TO_SINGLE_MAP
|
||||
];
|
||||
|
||||
const collectionType =
|
||||
COLLECTION_MAP[baseType as keyof typeof COLLECTION_MAP];
|
||||
|
||||
return sourceType === baseType || sourceType === collectionType;
|
||||
})();
|
||||
|
||||
const isGenericCollectionToAnyCollectionOrPolymorphic =
|
||||
sourceType === 'Collection' &&
|
||||
(COLLECTION_TYPES.includes(targetType) ||
|
||||
POLYMORPHIC_TYPES.includes(targetType));
|
||||
|
||||
const isCollectionToGenericCollection =
|
||||
targetType === 'Collection' && COLLECTION_TYPES.includes(sourceType);
|
||||
|
||||
const isIntToFloat = sourceType === 'integer' && targetType === 'float';
|
||||
|
||||
if (
|
||||
!(
|
||||
isCollectionItemToNonCollection ||
|
||||
isNonCollectionToCollectionItem ||
|
||||
isAnythingToPolymorphicOfSameBaseType ||
|
||||
isGenericCollectionToAnyCollectionOrPolymorphic ||
|
||||
isCollectionToGenericCollection ||
|
||||
isIntToFloat
|
||||
)
|
||||
) {
|
||||
return 'Field types must match';
|
||||
}
|
||||
}
|
||||
|
||||
const isGraphAcyclic = getIsGraphAcyclic(
|
||||
|
@ -17,176 +17,297 @@ export const KIND_MAP = {
|
||||
export const COLLECTION_TYPES: FieldType[] = [
|
||||
'Collection',
|
||||
'IntegerCollection',
|
||||
'BooleanCollection',
|
||||
'FloatCollection',
|
||||
'StringCollection',
|
||||
'BooleanCollection',
|
||||
'ImageCollection',
|
||||
'LatentsCollection',
|
||||
'ConditioningCollection',
|
||||
'ControlCollection',
|
||||
'ColorCollection',
|
||||
];
|
||||
|
||||
export const POLYMORPHIC_TYPES = [
|
||||
'IntegerPolymorphic',
|
||||
'BooleanPolymorphic',
|
||||
'FloatPolymorphic',
|
||||
'StringPolymorphic',
|
||||
'ImagePolymorphic',
|
||||
'LatentsPolymorphic',
|
||||
'ConditioningPolymorphic',
|
||||
'ControlPolymorphic',
|
||||
'ColorPolymorphic',
|
||||
];
|
||||
|
||||
export const MODEL_TYPES = [
|
||||
'ControlNetModelField',
|
||||
'LoRAModelField',
|
||||
'MainModelField',
|
||||
'ONNXModelField',
|
||||
'SDXLMainModelField',
|
||||
'SDXLRefinerModelField',
|
||||
'VaeModelField',
|
||||
'UNetField',
|
||||
'VaeField',
|
||||
'ClipField',
|
||||
];
|
||||
|
||||
export const COLLECTION_MAP = {
|
||||
integer: 'IntegerCollection',
|
||||
boolean: 'BooleanCollection',
|
||||
number: 'FloatCollection',
|
||||
float: 'FloatCollection',
|
||||
string: 'StringCollection',
|
||||
ImageField: 'ImageCollection',
|
||||
LatentsField: 'LatentsCollection',
|
||||
ConditioningField: 'ConditioningCollection',
|
||||
ControlField: 'ControlCollection',
|
||||
ColorField: 'ColorCollection',
|
||||
};
|
||||
export const isCollectionItemType = (
|
||||
itemType: string | undefined
|
||||
): itemType is keyof typeof COLLECTION_MAP =>
|
||||
Boolean(itemType && itemType in COLLECTION_MAP);
|
||||
|
||||
export const SINGLE_TO_POLYMORPHIC_MAP = {
|
||||
integer: 'IntegerPolymorphic',
|
||||
boolean: 'BooleanPolymorphic',
|
||||
number: 'FloatPolymorphic',
|
||||
float: 'FloatPolymorphic',
|
||||
string: 'StringPolymorphic',
|
||||
ImageField: 'ImagePolymorphic',
|
||||
LatentsField: 'LatentsPolymorphic',
|
||||
ConditioningField: 'ConditioningPolymorphic',
|
||||
ControlField: 'ControlPolymorphic',
|
||||
ColorField: 'ColorPolymorphic',
|
||||
};
|
||||
|
||||
export const POLYMORPHIC_TO_SINGLE_MAP = {
|
||||
IntegerPolymorphic: 'integer',
|
||||
BooleanPolymorphic: 'boolean',
|
||||
FloatPolymorphic: 'float',
|
||||
StringPolymorphic: 'string',
|
||||
ImagePolymorphic: 'ImageField',
|
||||
LatentsPolymorphic: 'LatentsField',
|
||||
ConditioningPolymorphic: 'ConditioningField',
|
||||
ControlPolymorphic: 'ControlField',
|
||||
ColorPolymorphic: 'ColorField',
|
||||
};
|
||||
|
||||
export const isPolymorphicItemType = (
|
||||
itemType: string | undefined
|
||||
): itemType is keyof typeof SINGLE_TO_POLYMORPHIC_MAP =>
|
||||
Boolean(itemType && itemType in SINGLE_TO_POLYMORPHIC_MAP);
|
||||
|
||||
export const FIELDS: Record<FieldType, FieldUIConfig> = {
|
||||
integer: {
|
||||
title: 'Integer',
|
||||
description: 'Integers are whole numbers, without a decimal point.',
|
||||
color: 'red.500',
|
||||
},
|
||||
float: {
|
||||
title: 'Float',
|
||||
description: 'Floats are numbers with a decimal point.',
|
||||
color: 'orange.500',
|
||||
},
|
||||
string: {
|
||||
title: 'String',
|
||||
description: 'Strings are text.',
|
||||
color: 'yellow.500',
|
||||
},
|
||||
boolean: {
|
||||
title: 'Boolean',
|
||||
color: 'green.500',
|
||||
description: 'Booleans are true or false.',
|
||||
title: 'Boolean',
|
||||
},
|
||||
enum: {
|
||||
title: 'Enum',
|
||||
description: 'Enums are values that may be one of a number of options.',
|
||||
color: 'blue.500',
|
||||
BooleanCollection: {
|
||||
color: 'green.500',
|
||||
description: 'A collection of booleans.',
|
||||
title: 'Boolean Collection',
|
||||
},
|
||||
array: {
|
||||
title: 'Array',
|
||||
description: 'Enums are values that may be one of a number of options.',
|
||||
color: 'base.500',
|
||||
},
|
||||
ImageField: {
|
||||
title: 'Image',
|
||||
description: 'Images may be passed between nodes.',
|
||||
color: 'purple.500',
|
||||
},
|
||||
DenoiseMaskField: {
|
||||
title: 'Denoise Mask',
|
||||
description: 'Denoise Mask may be passed between nodes',
|
||||
color: 'base.500',
|
||||
},
|
||||
LatentsField: {
|
||||
title: 'Latents',
|
||||
description: 'Latents may be passed between nodes.',
|
||||
color: 'pink.500',
|
||||
},
|
||||
LatentsCollection: {
|
||||
title: 'Latents Collection',
|
||||
description: 'Latents may be passed between nodes.',
|
||||
color: 'pink.500',
|
||||
},
|
||||
ConditioningField: {
|
||||
color: 'cyan.500',
|
||||
title: 'Conditioning',
|
||||
description: 'Conditioning may be passed between nodes.',
|
||||
},
|
||||
ConditioningCollection: {
|
||||
color: 'cyan.500',
|
||||
title: 'Conditioning Collection',
|
||||
description: 'Conditioning may be passed between nodes.',
|
||||
},
|
||||
ImageCollection: {
|
||||
title: 'Image Collection',
|
||||
description: 'A collection of images.',
|
||||
color: 'base.300',
|
||||
},
|
||||
UNetField: {
|
||||
color: 'red.500',
|
||||
title: 'UNet',
|
||||
description: 'UNet submodel.',
|
||||
BooleanPolymorphic: {
|
||||
color: 'green.500',
|
||||
description: 'A collection of booleans.',
|
||||
title: 'Boolean Polymorphic',
|
||||
},
|
||||
ClipField: {
|
||||
color: 'green.500',
|
||||
title: 'Clip',
|
||||
description: 'Tokenizer and text_encoder submodels.',
|
||||
},
|
||||
VaeField: {
|
||||
color: 'blue.500',
|
||||
title: 'Vae',
|
||||
description: 'Vae submodel.',
|
||||
},
|
||||
ControlField: {
|
||||
color: 'cyan.500',
|
||||
title: 'Control',
|
||||
description: 'Control info passed between nodes.',
|
||||
},
|
||||
MainModelField: {
|
||||
color: 'teal.500',
|
||||
title: 'Model',
|
||||
description: 'TODO',
|
||||
},
|
||||
SDXLRefinerModelField: {
|
||||
color: 'teal.500',
|
||||
title: 'Refiner Model',
|
||||
description: 'TODO',
|
||||
},
|
||||
VaeModelField: {
|
||||
color: 'teal.500',
|
||||
title: 'VAE',
|
||||
description: 'TODO',
|
||||
},
|
||||
LoRAModelField: {
|
||||
color: 'teal.500',
|
||||
title: 'LoRA',
|
||||
description: 'TODO',
|
||||
},
|
||||
ControlNetModelField: {
|
||||
color: 'teal.500',
|
||||
title: 'ControlNet',
|
||||
description: 'TODO',
|
||||
},
|
||||
Scheduler: {
|
||||
color: 'base.500',
|
||||
title: 'Scheduler',
|
||||
description: 'TODO',
|
||||
title: 'Clip',
|
||||
},
|
||||
Collection: {
|
||||
color: 'base.500',
|
||||
title: 'Collection',
|
||||
description: 'TODO',
|
||||
title: 'Collection',
|
||||
},
|
||||
CollectionItem: {
|
||||
color: 'base.500',
|
||||
title: 'Collection Item',
|
||||
description: 'TODO',
|
||||
title: 'Collection Item',
|
||||
},
|
||||
ColorCollection: {
|
||||
color: 'pink.300',
|
||||
description: 'A collection of colors.',
|
||||
title: 'Color Collection',
|
||||
},
|
||||
ColorField: {
|
||||
title: 'Color',
|
||||
color: 'pink.300',
|
||||
description: 'A RGBA color.',
|
||||
color: 'base.500',
|
||||
title: 'Color',
|
||||
},
|
||||
BooleanCollection: {
|
||||
title: 'Boolean Collection',
|
||||
description: 'A collection of booleans.',
|
||||
color: 'green.500',
|
||||
ColorPolymorphic: {
|
||||
color: 'pink.300',
|
||||
description: 'A collection of colors.',
|
||||
title: 'Color Polymorphic',
|
||||
},
|
||||
IntegerCollection: {
|
||||
title: 'Integer Collection',
|
||||
description: 'A collection of integers.',
|
||||
color: 'red.500',
|
||||
ConditioningCollection: {
|
||||
color: 'cyan.500',
|
||||
description: 'Conditioning may be passed between nodes.',
|
||||
title: 'Conditioning Collection',
|
||||
},
|
||||
ConditioningField: {
|
||||
color: 'cyan.500',
|
||||
description: 'Conditioning may be passed between nodes.',
|
||||
title: 'Conditioning',
|
||||
},
|
||||
ConditioningPolymorphic: {
|
||||
color: 'cyan.500',
|
||||
description: 'Conditioning may be passed between nodes.',
|
||||
title: 'Conditioning Polymorphic',
|
||||
},
|
||||
ControlCollection: {
|
||||
color: 'teal.500',
|
||||
description: 'Control info passed between nodes.',
|
||||
title: 'Control Collection',
|
||||
},
|
||||
ControlField: {
|
||||
color: 'teal.500',
|
||||
description: 'Control info passed between nodes.',
|
||||
title: 'Control',
|
||||
},
|
||||
ControlNetModelField: {
|
||||
color: 'teal.500',
|
||||
description: 'TODO',
|
||||
title: 'ControlNet',
|
||||
},
|
||||
ControlPolymorphic: {
|
||||
color: 'teal.500',
|
||||
description: 'Control info passed between nodes.',
|
||||
title: 'Control Polymorphic',
|
||||
},
|
||||
DenoiseMaskField: {
|
||||
color: 'blue.300',
|
||||
description: 'Denoise Mask may be passed between nodes',
|
||||
title: 'Denoise Mask',
|
||||
},
|
||||
enum: {
|
||||
color: 'blue.500',
|
||||
description: 'Enums are values that may be one of a number of options.',
|
||||
title: 'Enum',
|
||||
},
|
||||
float: {
|
||||
color: 'orange.500',
|
||||
description: 'Floats are numbers with a decimal point.',
|
||||
title: 'Float',
|
||||
},
|
||||
FloatCollection: {
|
||||
color: 'orange.500',
|
||||
title: 'Float Collection',
|
||||
description: 'A collection of floats.',
|
||||
title: 'Float Collection',
|
||||
},
|
||||
ColorCollection: {
|
||||
color: 'base.500',
|
||||
title: 'Color Collection',
|
||||
description: 'A collection of colors.',
|
||||
FloatPolymorphic: {
|
||||
color: 'orange.500',
|
||||
description: 'A collection of floats.',
|
||||
title: 'Float Polymorphic',
|
||||
},
|
||||
ImageCollection: {
|
||||
color: 'purple.500',
|
||||
description: 'A collection of images.',
|
||||
title: 'Image Collection',
|
||||
},
|
||||
ImageField: {
|
||||
color: 'purple.500',
|
||||
description: 'Images may be passed between nodes.',
|
||||
title: 'Image',
|
||||
},
|
||||
ImagePolymorphic: {
|
||||
color: 'purple.500',
|
||||
description: 'A collection of images.',
|
||||
title: 'Image Polymorphic',
|
||||
},
|
||||
integer: {
|
||||
color: 'red.500',
|
||||
description: 'Integers are whole numbers, without a decimal point.',
|
||||
title: 'Integer',
|
||||
},
|
||||
IntegerCollection: {
|
||||
color: 'red.500',
|
||||
description: 'A collection of integers.',
|
||||
title: 'Integer Collection',
|
||||
},
|
||||
IntegerPolymorphic: {
|
||||
color: 'red.500',
|
||||
description: 'A collection of integers.',
|
||||
title: 'Integer Polymorphic',
|
||||
},
|
||||
LatentsCollection: {
|
||||
color: 'pink.500',
|
||||
description: 'Latents may be passed between nodes.',
|
||||
title: 'Latents Collection',
|
||||
},
|
||||
LatentsField: {
|
||||
color: 'pink.500',
|
||||
description: 'Latents may be passed between nodes.',
|
||||
title: 'Latents',
|
||||
},
|
||||
LatentsPolymorphic: {
|
||||
color: 'pink.500',
|
||||
description: 'Latents may be passed between nodes.',
|
||||
title: 'Latents Polymorphic',
|
||||
},
|
||||
LoRAModelField: {
|
||||
color: 'teal.500',
|
||||
description: 'TODO',
|
||||
title: 'LoRA',
|
||||
},
|
||||
MainModelField: {
|
||||
color: 'teal.500',
|
||||
description: 'TODO',
|
||||
title: 'Model',
|
||||
},
|
||||
ONNXModelField: {
|
||||
color: 'base.500',
|
||||
title: 'ONNX Model',
|
||||
color: 'teal.500',
|
||||
description: 'ONNX model field.',
|
||||
title: 'ONNX Model',
|
||||
},
|
||||
Scheduler: {
|
||||
color: 'base.500',
|
||||
description: 'TODO',
|
||||
title: 'Scheduler',
|
||||
},
|
||||
SDXLMainModelField: {
|
||||
color: 'base.500',
|
||||
title: 'SDXL Model',
|
||||
color: 'teal.500',
|
||||
description: 'SDXL model field.',
|
||||
title: 'SDXL Model',
|
||||
},
|
||||
SDXLRefinerModelField: {
|
||||
color: 'teal.500',
|
||||
description: 'TODO',
|
||||
title: 'Refiner Model',
|
||||
},
|
||||
string: {
|
||||
color: 'yellow.500',
|
||||
description: 'Strings are text.',
|
||||
title: 'String',
|
||||
},
|
||||
StringCollection: {
|
||||
color: 'yellow.500',
|
||||
title: 'String Collection',
|
||||
description: 'A collection of strings.',
|
||||
title: 'String Collection',
|
||||
},
|
||||
StringPolymorphic: {
|
||||
color: 'yellow.500',
|
||||
description: 'A collection of strings.',
|
||||
title: 'String Polymorphic',
|
||||
},
|
||||
UNetField: {
|
||||
color: 'red.500',
|
||||
description: 'UNet submodel.',
|
||||
title: 'UNet',
|
||||
},
|
||||
VaeField: {
|
||||
color: 'blue.500',
|
||||
description: 'Vae submodel.',
|
||||
title: 'Vae',
|
||||
},
|
||||
VaeModelField: {
|
||||
color: 'teal.500',
|
||||
description: 'TODO',
|
||||
title: 'VAE',
|
||||
},
|
||||
};
|
||||
|
@ -11,7 +11,7 @@ import { keyBy } from 'lodash-es';
|
||||
import { OpenAPIV3 } from 'openapi-types';
|
||||
import { RgbaColor } from 'react-colorful';
|
||||
import { Node } from 'reactflow';
|
||||
import { Graph, ImageDTO, _InputField, _OutputField } from 'services/api/types';
|
||||
import { Graph, _InputField, _OutputField } from 'services/api/types';
|
||||
import {
|
||||
AnyInvocationType,
|
||||
AnyResult,
|
||||
@ -52,6 +52,10 @@ export type InvocationTemplate = {
|
||||
* The type of this node's output
|
||||
*/
|
||||
outputType: string; // TODO: generate a union of output types
|
||||
/**
|
||||
* The invocation's version.
|
||||
*/
|
||||
version?: string;
|
||||
};
|
||||
|
||||
export type FieldUIConfig = {
|
||||
@ -62,50 +66,48 @@ export type FieldUIConfig = {
|
||||
|
||||
// TODO: Get this from the OpenAPI schema? may be tricky...
|
||||
export const zFieldType = z.enum([
|
||||
// region Primitives
|
||||
'integer',
|
||||
'float',
|
||||
'boolean',
|
||||
'string',
|
||||
'array',
|
||||
'ImageField',
|
||||
'DenoiseMaskField',
|
||||
'LatentsField',
|
||||
'ConditioningField',
|
||||
'ControlField',
|
||||
'ColorField',
|
||||
'ImageCollection',
|
||||
'ConditioningCollection',
|
||||
'ColorCollection',
|
||||
'LatentsCollection',
|
||||
'IntegerCollection',
|
||||
'FloatCollection',
|
||||
'StringCollection',
|
||||
'BooleanCollection',
|
||||
// endregion
|
||||
|
||||
// region Models
|
||||
'MainModelField',
|
||||
'SDXLMainModelField',
|
||||
'SDXLRefinerModelField',
|
||||
'ONNXModelField',
|
||||
'VaeModelField',
|
||||
'LoRAModelField',
|
||||
'ControlNetModelField',
|
||||
'UNetField',
|
||||
'VaeField',
|
||||
'BooleanPolymorphic',
|
||||
'ClipField',
|
||||
// endregion
|
||||
|
||||
// region Iterate/Collect
|
||||
'Collection',
|
||||
'CollectionItem',
|
||||
// endregion
|
||||
|
||||
// region Misc
|
||||
'ColorCollection',
|
||||
'ColorField',
|
||||
'ColorPolymorphic',
|
||||
'ConditioningCollection',
|
||||
'ConditioningField',
|
||||
'ConditioningPolymorphic',
|
||||
'ControlCollection',
|
||||
'ControlField',
|
||||
'ControlNetModelField',
|
||||
'ControlPolymorphic',
|
||||
'DenoiseMaskField',
|
||||
'enum',
|
||||
'float',
|
||||
'FloatCollection',
|
||||
'FloatPolymorphic',
|
||||
'ImageCollection',
|
||||
'ImageField',
|
||||
'ImagePolymorphic',
|
||||
'integer',
|
||||
'IntegerCollection',
|
||||
'IntegerPolymorphic',
|
||||
'LatentsCollection',
|
||||
'LatentsField',
|
||||
'LatentsPolymorphic',
|
||||
'LoRAModelField',
|
||||
'MainModelField',
|
||||
'ONNXModelField',
|
||||
'Scheduler',
|
||||
// endregion
|
||||
'SDXLMainModelField',
|
||||
'SDXLRefinerModelField',
|
||||
'string',
|
||||
'StringCollection',
|
||||
'StringPolymorphic',
|
||||
'UNetField',
|
||||
'VaeField',
|
||||
'VaeModelField',
|
||||
]);
|
||||
|
||||
export type FieldType = z.infer<typeof zFieldType>;
|
||||
@ -122,38 +124,6 @@ export const isFieldType = (value: unknown): value is FieldType =>
|
||||
zFieldType.safeParse(value).success ||
|
||||
zReservedFieldType.safeParse(value).success;
|
||||
|
||||
/**
|
||||
* An input field template is generated on each page load from the OpenAPI schema.
|
||||
*
|
||||
* The template provides the field type and other field metadata (e.g. title, description,
|
||||
* maximum length, pattern to match, etc).
|
||||
*/
|
||||
export type InputFieldTemplate =
|
||||
| IntegerInputFieldTemplate
|
||||
| FloatInputFieldTemplate
|
||||
| StringInputFieldTemplate
|
||||
| BooleanInputFieldTemplate
|
||||
| ImageInputFieldTemplate
|
||||
| DenoiseMaskInputFieldTemplate
|
||||
| LatentsInputFieldTemplate
|
||||
| ConditioningInputFieldTemplate
|
||||
| UNetInputFieldTemplate
|
||||
| ClipInputFieldTemplate
|
||||
| VaeInputFieldTemplate
|
||||
| ControlInputFieldTemplate
|
||||
| EnumInputFieldTemplate
|
||||
| MainModelInputFieldTemplate
|
||||
| SDXLMainModelInputFieldTemplate
|
||||
| SDXLRefinerModelInputFieldTemplate
|
||||
| VaeModelInputFieldTemplate
|
||||
| LoRAModelInputFieldTemplate
|
||||
| ControlNetModelInputFieldTemplate
|
||||
| CollectionInputFieldTemplate
|
||||
| CollectionItemInputFieldTemplate
|
||||
| ColorInputFieldTemplate
|
||||
| ImageCollectionInputFieldTemplate
|
||||
| SchedulerInputFieldTemplate;
|
||||
|
||||
/**
|
||||
* Indicates the kind of input(s) this field may have.
|
||||
*/
|
||||
@ -232,24 +202,88 @@ export const zIntegerInputFieldValue = zInputFieldValueBase.extend({
|
||||
});
|
||||
export type IntegerInputFieldValue = z.infer<typeof zIntegerInputFieldValue>;
|
||||
|
||||
export const zIntegerCollectionInputFieldValue = zInputFieldValueBase.extend({
|
||||
type: z.literal('IntegerCollection'),
|
||||
value: z.array(z.number().int()).optional(),
|
||||
});
|
||||
export type IntegerCollectionInputFieldValue = z.infer<
|
||||
typeof zIntegerCollectionInputFieldValue
|
||||
>;
|
||||
|
||||
export const zIntegerPolymorphicInputFieldValue = zInputFieldValueBase.extend({
|
||||
type: z.literal('IntegerPolymorphic'),
|
||||
value: z.union([z.number().int(), z.array(z.number().int())]).optional(),
|
||||
});
|
||||
export type IntegerPolymorphicInputFieldValue = z.infer<
|
||||
typeof zIntegerPolymorphicInputFieldValue
|
||||
>;
|
||||
|
||||
export const zFloatInputFieldValue = zInputFieldValueBase.extend({
|
||||
type: z.literal('float'),
|
||||
value: z.number().optional(),
|
||||
});
|
||||
export type FloatInputFieldValue = z.infer<typeof zFloatInputFieldValue>;
|
||||
|
||||
export const zFloatCollectionInputFieldValue = zInputFieldValueBase.extend({
|
||||
type: z.literal('FloatCollection'),
|
||||
value: z.array(z.number()).optional(),
|
||||
});
|
||||
export type FloatCollectionInputFieldValue = z.infer<
|
||||
typeof zFloatCollectionInputFieldValue
|
||||
>;
|
||||
|
||||
export const zFloatPolymorphicInputFieldValue = zInputFieldValueBase.extend({
|
||||
type: z.literal('FloatPolymorphic'),
|
||||
value: z.union([z.number(), z.array(z.number())]).optional(),
|
||||
});
|
||||
export type FloatPolymorphicInputFieldValue = z.infer<
|
||||
typeof zFloatPolymorphicInputFieldValue
|
||||
>;
|
||||
|
||||
export const zStringInputFieldValue = zInputFieldValueBase.extend({
|
||||
type: z.literal('string'),
|
||||
value: z.string().optional(),
|
||||
});
|
||||
export type StringInputFieldValue = z.infer<typeof zStringInputFieldValue>;
|
||||
|
||||
export const zStringCollectionInputFieldValue = zInputFieldValueBase.extend({
|
||||
type: z.literal('StringCollection'),
|
||||
value: z.array(z.string()).optional(),
|
||||
});
|
||||
export type StringCollectionInputFieldValue = z.infer<
|
||||
typeof zStringCollectionInputFieldValue
|
||||
>;
|
||||
|
||||
export const zStringPolymorphicInputFieldValue = zInputFieldValueBase.extend({
|
||||
type: z.literal('StringPolymorphic'),
|
||||
value: z.union([z.string(), z.array(z.string())]).optional(),
|
||||
});
|
||||
export type StringPolymorphicInputFieldValue = z.infer<
|
||||
typeof zStringPolymorphicInputFieldValue
|
||||
>;
|
||||
|
||||
export const zBooleanInputFieldValue = zInputFieldValueBase.extend({
|
||||
type: z.literal('boolean'),
|
||||
value: z.boolean().optional(),
|
||||
});
|
||||
export type BooleanInputFieldValue = z.infer<typeof zBooleanInputFieldValue>;
|
||||
|
||||
export const zBooleanCollectionInputFieldValue = zInputFieldValueBase.extend({
|
||||
type: z.literal('BooleanCollection'),
|
||||
value: z.array(z.boolean()).optional(),
|
||||
});
|
||||
export type BooleanCollectionInputFieldValue = z.infer<
|
||||
typeof zBooleanCollectionInputFieldValue
|
||||
>;
|
||||
|
||||
export const zBooleanPolymorphicInputFieldValue = zInputFieldValueBase.extend({
|
||||
type: z.literal('BooleanPolymorphic'),
|
||||
value: z.union([z.boolean(), z.array(z.boolean())]).optional(),
|
||||
});
|
||||
export type BooleanPolymorphicInputFieldValue = z.infer<
|
||||
typeof zBooleanPolymorphicInputFieldValue
|
||||
>;
|
||||
|
||||
export const zEnumInputFieldValue = zInputFieldValueBase.extend({
|
||||
type: z.literal('enum'),
|
||||
value: z.union([z.string(), z.number()]).optional(),
|
||||
@ -262,6 +296,22 @@ export const zLatentsInputFieldValue = zInputFieldValueBase.extend({
|
||||
});
|
||||
export type LatentsInputFieldValue = z.infer<typeof zLatentsInputFieldValue>;
|
||||
|
||||
export const zLatentsCollectionInputFieldValue = zInputFieldValueBase.extend({
|
||||
type: z.literal('LatentsCollection'),
|
||||
value: z.array(zLatentsField).optional(),
|
||||
});
|
||||
export type LatentsCollectionInputFieldValue = z.infer<
|
||||
typeof zLatentsCollectionInputFieldValue
|
||||
>;
|
||||
|
||||
export const zLatentsPolymorphicInputFieldValue = zInputFieldValueBase.extend({
|
||||
type: z.literal('LatentsPolymorphic'),
|
||||
value: z.union([zLatentsField, z.array(zLatentsField)]).optional(),
|
||||
});
|
||||
export type LatentsPolymorphicInputFieldValue = z.infer<
|
||||
typeof zLatentsPolymorphicInputFieldValue
|
||||
>;
|
||||
|
||||
export const zDenoiseMaskInputFieldValue = zInputFieldValueBase.extend({
|
||||
type: z.literal('DenoiseMaskField'),
|
||||
value: zDenoiseMaskField.optional(),
|
||||
@ -278,6 +328,26 @@ export type ConditioningInputFieldValue = z.infer<
|
||||
typeof zConditioningInputFieldValue
|
||||
>;
|
||||
|
||||
export const zConditioningCollectionInputFieldValue =
|
||||
zInputFieldValueBase.extend({
|
||||
type: z.literal('ConditioningCollection'),
|
||||
value: z.array(zConditioningField).optional(),
|
||||
});
|
||||
export type ConditioningCollectionInputFieldValue = z.infer<
|
||||
typeof zConditioningCollectionInputFieldValue
|
||||
>;
|
||||
|
||||
export const zConditioningPolymorphicInputFieldValue =
|
||||
zInputFieldValueBase.extend({
|
||||
type: z.literal('ConditioningPolymorphic'),
|
||||
value: z
|
||||
.union([zConditioningField, z.array(zConditioningField)])
|
||||
.optional(),
|
||||
});
|
||||
export type ConditioningPolymorphicInputFieldValue = z.infer<
|
||||
typeof zConditioningPolymorphicInputFieldValue
|
||||
>;
|
||||
|
||||
export const zControlNetModel = zModelIdentifier;
|
||||
export type ControlNetModel = z.infer<typeof zControlNetModel>;
|
||||
|
||||
@ -302,6 +372,22 @@ export const zControlInputFieldValue = zInputFieldValueBase.extend({
|
||||
});
|
||||
export type ControlInputFieldValue = z.infer<typeof zControlInputFieldValue>;
|
||||
|
||||
export const zControlPolymorphicInputFieldValue = zInputFieldValueBase.extend({
|
||||
type: z.literal('ControlPolymorphic'),
|
||||
value: z.union([zControlField, z.array(zControlField)]).optional(),
|
||||
});
|
||||
export type ControlPolymorphicInputFieldValue = z.infer<
|
||||
typeof zControlPolymorphicInputFieldValue
|
||||
>;
|
||||
|
||||
export const zControlCollectionInputFieldValue = zInputFieldValueBase.extend({
|
||||
type: z.literal('ControlCollection'),
|
||||
value: z.array(zControlField).optional(),
|
||||
});
|
||||
export type ControlCollectionInputFieldValue = z.infer<
|
||||
typeof zControlCollectionInputFieldValue
|
||||
>;
|
||||
|
||||
export const zModelType = z.enum([
|
||||
'onnx',
|
||||
'main',
|
||||
@ -381,6 +467,14 @@ export const zImageInputFieldValue = zInputFieldValueBase.extend({
|
||||
});
|
||||
export type ImageInputFieldValue = z.infer<typeof zImageInputFieldValue>;
|
||||
|
||||
export const zImagePolymorphicInputFieldValue = zInputFieldValueBase.extend({
|
||||
type: z.literal('ImagePolymorphic'),
|
||||
value: z.union([zImageField, z.array(zImageField)]).optional(),
|
||||
});
|
||||
export type ImagePolymorphicInputFieldValue = z.infer<
|
||||
typeof zImagePolymorphicInputFieldValue
|
||||
>;
|
||||
|
||||
export const zImageCollectionInputFieldValue = zInputFieldValueBase.extend({
|
||||
type: z.literal('ImageCollection'),
|
||||
value: z.array(zImageField).optional(),
|
||||
@ -473,6 +567,22 @@ export const zColorInputFieldValue = zInputFieldValueBase.extend({
|
||||
});
|
||||
export type ColorInputFieldValue = z.infer<typeof zColorInputFieldValue>;
|
||||
|
||||
export const zColorCollectionInputFieldValue = zInputFieldValueBase.extend({
|
||||
type: z.literal('ColorCollection'),
|
||||
value: z.array(zColorField).optional(),
|
||||
});
|
||||
export type ColorCollectionInputFieldValue = z.infer<
|
||||
typeof zColorCollectionInputFieldValue
|
||||
>;
|
||||
|
||||
export const zColorPolymorphicInputFieldValue = zInputFieldValueBase.extend({
|
||||
type: z.literal('ColorPolymorphic'),
|
||||
value: z.union([zColorField, z.array(zColorField)]).optional(),
|
||||
});
|
||||
export type ColorPolymorphicInputFieldValue = z.infer<
|
||||
typeof zColorPolymorphicInputFieldValue
|
||||
>;
|
||||
|
||||
export const zSchedulerInputFieldValue = zInputFieldValueBase.extend({
|
||||
type: z.literal('Scheduler'),
|
||||
value: zScheduler.optional(),
|
||||
@ -482,30 +592,47 @@ export type SchedulerInputFieldValue = z.infer<
|
||||
>;
|
||||
|
||||
export const zInputFieldValue = z.discriminatedUnion('type', [
|
||||
zIntegerInputFieldValue,
|
||||
zFloatInputFieldValue,
|
||||
zStringInputFieldValue,
|
||||
zBooleanCollectionInputFieldValue,
|
||||
zBooleanInputFieldValue,
|
||||
zImageInputFieldValue,
|
||||
zLatentsInputFieldValue,
|
||||
zDenoiseMaskInputFieldValue,
|
||||
zConditioningInputFieldValue,
|
||||
zUNetInputFieldValue,
|
||||
zBooleanPolymorphicInputFieldValue,
|
||||
zClipInputFieldValue,
|
||||
zVaeInputFieldValue,
|
||||
zControlInputFieldValue,
|
||||
zEnumInputFieldValue,
|
||||
zMainModelInputFieldValue,
|
||||
zSDXLMainModelInputFieldValue,
|
||||
zSDXLRefinerModelInputFieldValue,
|
||||
zVaeModelInputFieldValue,
|
||||
zLoRAModelInputFieldValue,
|
||||
zControlNetModelInputFieldValue,
|
||||
zCollectionInputFieldValue,
|
||||
zCollectionItemInputFieldValue,
|
||||
zColorInputFieldValue,
|
||||
zColorCollectionInputFieldValue,
|
||||
zColorPolymorphicInputFieldValue,
|
||||
zConditioningInputFieldValue,
|
||||
zConditioningCollectionInputFieldValue,
|
||||
zConditioningPolymorphicInputFieldValue,
|
||||
zControlInputFieldValue,
|
||||
zControlNetModelInputFieldValue,
|
||||
zControlCollectionInputFieldValue,
|
||||
zControlPolymorphicInputFieldValue,
|
||||
zDenoiseMaskInputFieldValue,
|
||||
zEnumInputFieldValue,
|
||||
zFloatCollectionInputFieldValue,
|
||||
zFloatInputFieldValue,
|
||||
zFloatPolymorphicInputFieldValue,
|
||||
zImageCollectionInputFieldValue,
|
||||
zImagePolymorphicInputFieldValue,
|
||||
zImageInputFieldValue,
|
||||
zIntegerCollectionInputFieldValue,
|
||||
zIntegerPolymorphicInputFieldValue,
|
||||
zIntegerInputFieldValue,
|
||||
zLatentsInputFieldValue,
|
||||
zLatentsCollectionInputFieldValue,
|
||||
zLatentsPolymorphicInputFieldValue,
|
||||
zLoRAModelInputFieldValue,
|
||||
zMainModelInputFieldValue,
|
||||
zSchedulerInputFieldValue,
|
||||
zSDXLMainModelInputFieldValue,
|
||||
zSDXLRefinerModelInputFieldValue,
|
||||
zStringCollectionInputFieldValue,
|
||||
zStringPolymorphicInputFieldValue,
|
||||
zStringInputFieldValue,
|
||||
zUNetInputFieldValue,
|
||||
zVaeInputFieldValue,
|
||||
zVaeModelInputFieldValue,
|
||||
]);
|
||||
|
||||
export type InputFieldValue = z.infer<typeof zInputFieldValue>;
|
||||
@ -514,7 +641,6 @@ export type InputFieldTemplateBase = {
|
||||
name: string;
|
||||
title: string;
|
||||
description: string;
|
||||
type: FieldType;
|
||||
required: boolean;
|
||||
fieldKind: 'input';
|
||||
} & _InputField;
|
||||
@ -529,6 +655,19 @@ export type IntegerInputFieldTemplate = InputFieldTemplateBase & {
|
||||
exclusiveMinimum?: boolean;
|
||||
};
|
||||
|
||||
export type IntegerCollectionInputFieldTemplate = InputFieldTemplateBase & {
|
||||
type: 'IntegerCollection';
|
||||
default: number[];
|
||||
item_default?: number;
|
||||
};
|
||||
|
||||
export type IntegerPolymorphicInputFieldTemplate = Omit<
|
||||
IntegerInputFieldTemplate,
|
||||
'type'
|
||||
> & {
|
||||
type: 'IntegerPolymorphic';
|
||||
};
|
||||
|
||||
export type FloatInputFieldTemplate = InputFieldTemplateBase & {
|
||||
type: 'float';
|
||||
default: number;
|
||||
@ -539,6 +678,19 @@ export type FloatInputFieldTemplate = InputFieldTemplateBase & {
|
||||
exclusiveMinimum?: boolean;
|
||||
};
|
||||
|
||||
export type FloatCollectionInputFieldTemplate = InputFieldTemplateBase & {
|
||||
type: 'FloatCollection';
|
||||
default: number[];
|
||||
item_default?: number;
|
||||
};
|
||||
|
||||
export type FloatPolymorphicInputFieldTemplate = Omit<
|
||||
FloatInputFieldTemplate,
|
||||
'type'
|
||||
> & {
|
||||
type: 'FloatPolymorphic';
|
||||
};
|
||||
|
||||
export type StringInputFieldTemplate = InputFieldTemplateBase & {
|
||||
type: 'string';
|
||||
default: string;
|
||||
@ -547,19 +699,53 @@ export type StringInputFieldTemplate = InputFieldTemplateBase & {
|
||||
pattern?: string;
|
||||
};
|
||||
|
||||
export type StringCollectionInputFieldTemplate = InputFieldTemplateBase & {
|
||||
type: 'StringCollection';
|
||||
default: string[];
|
||||
item_default?: string;
|
||||
};
|
||||
|
||||
export type StringPolymorphicInputFieldTemplate = Omit<
|
||||
StringInputFieldTemplate,
|
||||
'type'
|
||||
> & {
|
||||
type: 'StringPolymorphic';
|
||||
};
|
||||
|
||||
export type BooleanInputFieldTemplate = InputFieldTemplateBase & {
|
||||
default: boolean;
|
||||
type: 'boolean';
|
||||
};
|
||||
|
||||
export type BooleanCollectionInputFieldTemplate = InputFieldTemplateBase & {
|
||||
type: 'BooleanCollection';
|
||||
default: boolean[];
|
||||
item_default?: boolean;
|
||||
};
|
||||
|
||||
export type BooleanPolymorphicInputFieldTemplate = Omit<
|
||||
BooleanInputFieldTemplate,
|
||||
'type'
|
||||
> & {
|
||||
type: 'BooleanPolymorphic';
|
||||
};
|
||||
|
||||
export type ImageInputFieldTemplate = InputFieldTemplateBase & {
|
||||
default: ImageDTO;
|
||||
default: ImageField;
|
||||
type: 'ImageField';
|
||||
};
|
||||
|
||||
export type ImageCollectionInputFieldTemplate = InputFieldTemplateBase & {
|
||||
default: ImageField[];
|
||||
type: 'ImageCollection';
|
||||
item_default?: ImageField;
|
||||
};
|
||||
|
||||
export type ImagePolymorphicInputFieldTemplate = Omit<
|
||||
ImageInputFieldTemplate,
|
||||
'type'
|
||||
> & {
|
||||
type: 'ImagePolymorphic';
|
||||
};
|
||||
|
||||
export type DenoiseMaskInputFieldTemplate = InputFieldTemplateBase & {
|
||||
@ -568,15 +754,40 @@ export type DenoiseMaskInputFieldTemplate = InputFieldTemplateBase & {
|
||||
};
|
||||
|
||||
export type LatentsInputFieldTemplate = InputFieldTemplateBase & {
|
||||
default: string;
|
||||
default: LatentsField;
|
||||
type: 'LatentsField';
|
||||
};
|
||||
|
||||
export type LatentsCollectionInputFieldTemplate = InputFieldTemplateBase & {
|
||||
default: LatentsField[];
|
||||
type: 'LatentsCollection';
|
||||
item_default?: LatentsField;
|
||||
};
|
||||
|
||||
export type LatentsPolymorphicInputFieldTemplate = InputFieldTemplateBase & {
|
||||
default: LatentsField;
|
||||
type: 'LatentsPolymorphic';
|
||||
};
|
||||
|
||||
export type ConditioningInputFieldTemplate = InputFieldTemplateBase & {
|
||||
default: undefined;
|
||||
type: 'ConditioningField';
|
||||
};
|
||||
|
||||
export type ConditioningCollectionInputFieldTemplate =
|
||||
InputFieldTemplateBase & {
|
||||
default: ConditioningField[];
|
||||
type: 'ConditioningCollection';
|
||||
item_default?: ConditioningField;
|
||||
};
|
||||
|
||||
export type ConditioningPolymorphicInputFieldTemplate = Omit<
|
||||
ConditioningInputFieldTemplate,
|
||||
'type'
|
||||
> & {
|
||||
type: 'ConditioningPolymorphic';
|
||||
};
|
||||
|
||||
export type UNetInputFieldTemplate = InputFieldTemplateBase & {
|
||||
default: undefined;
|
||||
type: 'UNetField';
|
||||
@ -597,6 +808,19 @@ export type ControlInputFieldTemplate = InputFieldTemplateBase & {
|
||||
type: 'ControlField';
|
||||
};
|
||||
|
||||
export type ControlCollectionInputFieldTemplate = InputFieldTemplateBase & {
|
||||
default: undefined;
|
||||
type: 'ControlCollection';
|
||||
item_default?: ControlField;
|
||||
};
|
||||
|
||||
export type ControlPolymorphicInputFieldTemplate = Omit<
|
||||
ControlInputFieldTemplate,
|
||||
'type'
|
||||
> & {
|
||||
type: 'ControlPolymorphic';
|
||||
};
|
||||
|
||||
export type EnumInputFieldTemplate = InputFieldTemplateBase & {
|
||||
default: string | number;
|
||||
type: 'enum';
|
||||
@ -649,6 +873,18 @@ export type ColorInputFieldTemplate = InputFieldTemplateBase & {
|
||||
type: 'ColorField';
|
||||
};
|
||||
|
||||
export type ColorPolymorphicInputFieldTemplate = Omit<
|
||||
ColorInputFieldTemplate,
|
||||
'type'
|
||||
> & {
|
||||
type: 'ColorPolymorphic';
|
||||
};
|
||||
|
||||
export type ColorCollectionInputFieldTemplate = InputFieldTemplateBase & {
|
||||
default: [];
|
||||
type: 'ColorCollection';
|
||||
};
|
||||
|
||||
export type SchedulerInputFieldTemplate = InputFieldTemplateBase & {
|
||||
default: SchedulerParam;
|
||||
type: 'Scheduler';
|
||||
@ -659,6 +895,55 @@ export type WorkflowInputFieldTemplate = InputFieldTemplateBase & {
|
||||
type: 'WorkflowField';
|
||||
};
|
||||
|
||||
/**
|
||||
* An input field template is generated on each page load from the OpenAPI schema.
|
||||
*
|
||||
* The template provides the field type and other field metadata (e.g. title, description,
|
||||
* maximum length, pattern to match, etc).
|
||||
*/
|
||||
export type InputFieldTemplate =
|
||||
| BooleanCollectionInputFieldTemplate
|
||||
| BooleanPolymorphicInputFieldTemplate
|
||||
| BooleanInputFieldTemplate
|
||||
| ClipInputFieldTemplate
|
||||
| CollectionInputFieldTemplate
|
||||
| CollectionItemInputFieldTemplate
|
||||
| ColorInputFieldTemplate
|
||||
| ColorCollectionInputFieldTemplate
|
||||
| ColorPolymorphicInputFieldTemplate
|
||||
| ConditioningInputFieldTemplate
|
||||
| ConditioningCollectionInputFieldTemplate
|
||||
| ConditioningPolymorphicInputFieldTemplate
|
||||
| ControlInputFieldTemplate
|
||||
| ControlCollectionInputFieldTemplate
|
||||
| ControlNetModelInputFieldTemplate
|
||||
| ControlPolymorphicInputFieldTemplate
|
||||
| DenoiseMaskInputFieldTemplate
|
||||
| EnumInputFieldTemplate
|
||||
| FloatCollectionInputFieldTemplate
|
||||
| FloatInputFieldTemplate
|
||||
| FloatPolymorphicInputFieldTemplate
|
||||
| ImageCollectionInputFieldTemplate
|
||||
| ImagePolymorphicInputFieldTemplate
|
||||
| ImageInputFieldTemplate
|
||||
| IntegerCollectionInputFieldTemplate
|
||||
| IntegerPolymorphicInputFieldTemplate
|
||||
| IntegerInputFieldTemplate
|
||||
| LatentsInputFieldTemplate
|
||||
| LatentsCollectionInputFieldTemplate
|
||||
| LatentsPolymorphicInputFieldTemplate
|
||||
| LoRAModelInputFieldTemplate
|
||||
| MainModelInputFieldTemplate
|
||||
| SchedulerInputFieldTemplate
|
||||
| SDXLMainModelInputFieldTemplate
|
||||
| SDXLRefinerModelInputFieldTemplate
|
||||
| StringCollectionInputFieldTemplate
|
||||
| StringPolymorphicInputFieldTemplate
|
||||
| StringInputFieldTemplate
|
||||
| UNetInputFieldTemplate
|
||||
| VaeInputFieldTemplate
|
||||
| VaeModelInputFieldTemplate;
|
||||
|
||||
export const isInputFieldValue = (
|
||||
field?: InputFieldValue | OutputFieldValue
|
||||
): field is InputFieldValue => Boolean(field && field.fieldKind === 'input');
|
||||
@ -681,6 +966,7 @@ export type InvocationSchemaExtra = {
|
||||
title: string;
|
||||
category?: string;
|
||||
tags?: string[];
|
||||
version?: string;
|
||||
properties: Omit<
|
||||
NonNullable<OpenAPIV3.SchemaObject['properties']> &
|
||||
(_InputField | _OutputField),
|
||||
@ -731,8 +1017,22 @@ export type InvocationSchemaObject = (
|
||||
) & { class: 'invocation' };
|
||||
|
||||
export const isSchemaObject = (
|
||||
obj: OpenAPIV3.ReferenceObject | OpenAPIV3.SchemaObject
|
||||
): obj is OpenAPIV3.SchemaObject => !('$ref' in obj);
|
||||
obj: OpenAPIV3.ReferenceObject | OpenAPIV3.SchemaObject | undefined
|
||||
): obj is OpenAPIV3.SchemaObject => Boolean(obj && !('$ref' in obj));
|
||||
|
||||
export const isArraySchemaObject = (
|
||||
obj: OpenAPIV3.ReferenceObject | OpenAPIV3.SchemaObject | undefined
|
||||
): obj is OpenAPIV3.ArraySchemaObject =>
|
||||
Boolean(obj && !('$ref' in obj) && obj.type === 'array');
|
||||
|
||||
export const isNonArraySchemaObject = (
|
||||
obj: OpenAPIV3.ReferenceObject | OpenAPIV3.SchemaObject | undefined
|
||||
): obj is OpenAPIV3.NonArraySchemaObject =>
|
||||
Boolean(obj && !('$ref' in obj) && obj.type !== 'array');
|
||||
|
||||
export const isRefObject = (
|
||||
obj: OpenAPIV3.ReferenceObject | OpenAPIV3.SchemaObject | undefined
|
||||
): obj is OpenAPIV3.ReferenceObject => Boolean(obj && '$ref' in obj);
|
||||
|
||||
export const isInvocationSchemaObject = (
|
||||
obj:
|
||||
@ -800,6 +1100,29 @@ export const zCoreMetadata = z
|
||||
|
||||
export type CoreMetadata = z.infer<typeof zCoreMetadata>;
|
||||
|
||||
export const zSemVer = z.string().refine((val) => {
|
||||
const [major, minor, patch] = val.split('.');
|
||||
return (
|
||||
major !== undefined &&
|
||||
Number.isInteger(Number(major)) &&
|
||||
minor !== undefined &&
|
||||
Number.isInteger(Number(minor)) &&
|
||||
patch !== undefined &&
|
||||
Number.isInteger(Number(patch))
|
||||
);
|
||||
});
|
||||
|
||||
export const zParsedSemver = zSemVer.transform((val) => {
|
||||
const [major, minor, patch] = val.split('.');
|
||||
return {
|
||||
major: Number(major),
|
||||
minor: Number(minor),
|
||||
patch: Number(patch),
|
||||
};
|
||||
});
|
||||
|
||||
export type SemVer = z.infer<typeof zSemVer>;
|
||||
|
||||
export const zInvocationNodeData = z.object({
|
||||
id: z.string().trim().min(1),
|
||||
// no easy way to build this dynamically, and we don't want to anyways, because this will be used
|
||||
@ -812,6 +1135,7 @@ export const zInvocationNodeData = z.object({
|
||||
notes: z.string(),
|
||||
embedWorkflow: z.boolean(),
|
||||
isIntermediate: z.boolean(),
|
||||
version: zSemVer.optional(),
|
||||
});
|
||||
|
||||
// Massage this to get better type safety while developing
|
||||
@ -900,20 +1224,6 @@ export const zFieldIdentifier = z.object({
|
||||
|
||||
export type FieldIdentifier = z.infer<typeof zFieldIdentifier>;
|
||||
|
||||
export const zSemVer = z.string().refine((val) => {
|
||||
const [major, minor, patch] = val.split('.');
|
||||
return (
|
||||
major !== undefined &&
|
||||
minor !== undefined &&
|
||||
patch !== undefined &&
|
||||
Number.isInteger(Number(major)) &&
|
||||
Number.isInteger(Number(minor)) &&
|
||||
Number.isInteger(Number(patch))
|
||||
);
|
||||
});
|
||||
|
||||
export type SemVer = z.infer<typeof zSemVer>;
|
||||
|
||||
export type WorkflowWarning = {
|
||||
message: string;
|
||||
issues: string[];
|
||||
|
@ -1,5 +1,14 @@
|
||||
import { isBoolean, isInteger, isNumber, isString } from 'lodash-es';
|
||||
import { OpenAPIV3 } from 'openapi-types';
|
||||
import {
|
||||
COLLECTION_MAP,
|
||||
POLYMORPHIC_TYPES,
|
||||
SINGLE_TO_POLYMORPHIC_MAP,
|
||||
isCollectionItemType,
|
||||
isPolymorphicItemType,
|
||||
} from '../types/constants';
|
||||
import {
|
||||
BooleanCollectionInputFieldTemplate,
|
||||
BooleanInputFieldTemplate,
|
||||
ClipInputFieldTemplate,
|
||||
CollectionInputFieldTemplate,
|
||||
@ -11,10 +20,13 @@ import {
|
||||
DenoiseMaskInputFieldTemplate,
|
||||
EnumInputFieldTemplate,
|
||||
FieldType,
|
||||
FloatCollectionInputFieldTemplate,
|
||||
FloatPolymorphicInputFieldTemplate,
|
||||
FloatInputFieldTemplate,
|
||||
ImageCollectionInputFieldTemplate,
|
||||
ImageInputFieldTemplate,
|
||||
InputFieldTemplateBase,
|
||||
IntegerCollectionInputFieldTemplate,
|
||||
IntegerInputFieldTemplate,
|
||||
InvocationFieldSchema,
|
||||
InvocationSchemaObject,
|
||||
@ -24,11 +36,32 @@ import {
|
||||
SDXLMainModelInputFieldTemplate,
|
||||
SDXLRefinerModelInputFieldTemplate,
|
||||
SchedulerInputFieldTemplate,
|
||||
StringCollectionInputFieldTemplate,
|
||||
StringInputFieldTemplate,
|
||||
UNetInputFieldTemplate,
|
||||
VaeInputFieldTemplate,
|
||||
VaeModelInputFieldTemplate,
|
||||
isArraySchemaObject,
|
||||
isNonArraySchemaObject,
|
||||
isRefObject,
|
||||
isSchemaObject,
|
||||
ControlPolymorphicInputFieldTemplate,
|
||||
ColorPolymorphicInputFieldTemplate,
|
||||
ColorCollectionInputFieldTemplate,
|
||||
IntegerPolymorphicInputFieldTemplate,
|
||||
StringPolymorphicInputFieldTemplate,
|
||||
BooleanPolymorphicInputFieldTemplate,
|
||||
ImagePolymorphicInputFieldTemplate,
|
||||
LatentsPolymorphicInputFieldTemplate,
|
||||
LatentsCollectionInputFieldTemplate,
|
||||
ConditioningPolymorphicInputFieldTemplate,
|
||||
ConditioningCollectionInputFieldTemplate,
|
||||
ControlCollectionInputFieldTemplate,
|
||||
ImageField,
|
||||
LatentsField,
|
||||
ConditioningField,
|
||||
} from '../types/types';
|
||||
import { ControlField } from 'services/api/types';
|
||||
|
||||
export type BaseFieldProperties = 'name' | 'title' | 'description';
|
||||
|
||||
@ -45,15 +78,8 @@ export type BuildInputFieldArg = {
|
||||
* @example
|
||||
* refObjectToFieldType({ "$ref": "#/components/schemas/ImageField" }) --> 'ImageField'
|
||||
*/
|
||||
export const refObjectToFieldType = (
|
||||
refObject: OpenAPIV3.ReferenceObject
|
||||
): FieldType => {
|
||||
const name = refObject.$ref.split('/').slice(-1)[0];
|
||||
if (!name) {
|
||||
throw `Unknown field type: ${name}`;
|
||||
}
|
||||
return name as FieldType;
|
||||
};
|
||||
export const refObjectToSchemaName = (refObject: OpenAPIV3.ReferenceObject) =>
|
||||
refObject.$ref.split('/').slice(-1)[0];
|
||||
|
||||
const buildIntegerInputFieldTemplate = ({
|
||||
schemaObject,
|
||||
@ -88,6 +114,57 @@ const buildIntegerInputFieldTemplate = ({
|
||||
return template;
|
||||
};
|
||||
|
||||
const buildIntegerPolymorphicInputFieldTemplate = ({
|
||||
schemaObject,
|
||||
baseField,
|
||||
}: BuildInputFieldArg): IntegerPolymorphicInputFieldTemplate => {
|
||||
const template: IntegerPolymorphicInputFieldTemplate = {
|
||||
...baseField,
|
||||
type: 'IntegerPolymorphic',
|
||||
default: schemaObject.default ?? 0,
|
||||
};
|
||||
|
||||
if (schemaObject.multipleOf !== undefined) {
|
||||
template.multipleOf = schemaObject.multipleOf;
|
||||
}
|
||||
|
||||
if (schemaObject.maximum !== undefined) {
|
||||
template.maximum = schemaObject.maximum;
|
||||
}
|
||||
|
||||
if (schemaObject.exclusiveMaximum !== undefined) {
|
||||
template.exclusiveMaximum = schemaObject.exclusiveMaximum;
|
||||
}
|
||||
|
||||
if (schemaObject.minimum !== undefined) {
|
||||
template.minimum = schemaObject.minimum;
|
||||
}
|
||||
|
||||
if (schemaObject.exclusiveMinimum !== undefined) {
|
||||
template.exclusiveMinimum = schemaObject.exclusiveMinimum;
|
||||
}
|
||||
|
||||
return template;
|
||||
};
|
||||
|
||||
const buildIntegerCollectionInputFieldTemplate = ({
|
||||
schemaObject,
|
||||
baseField,
|
||||
}: BuildInputFieldArg): IntegerCollectionInputFieldTemplate => {
|
||||
const item_default =
|
||||
isNumber(schemaObject.item_default) && isInteger(schemaObject.item_default)
|
||||
? schemaObject.item_default
|
||||
: 0;
|
||||
const template: IntegerCollectionInputFieldTemplate = {
|
||||
...baseField,
|
||||
type: 'IntegerCollection',
|
||||
default: schemaObject.default ?? [],
|
||||
item_default,
|
||||
};
|
||||
|
||||
return template;
|
||||
};
|
||||
|
||||
const buildFloatInputFieldTemplate = ({
|
||||
schemaObject,
|
||||
baseField,
|
||||
@ -121,6 +198,54 @@ const buildFloatInputFieldTemplate = ({
|
||||
return template;
|
||||
};
|
||||
|
||||
const buildFloatPolymorphicInputFieldTemplate = ({
|
||||
schemaObject,
|
||||
baseField,
|
||||
}: BuildInputFieldArg): FloatPolymorphicInputFieldTemplate => {
|
||||
const template: FloatPolymorphicInputFieldTemplate = {
|
||||
...baseField,
|
||||
type: 'FloatPolymorphic',
|
||||
default: schemaObject.default ?? 0,
|
||||
};
|
||||
if (schemaObject.multipleOf !== undefined) {
|
||||
template.multipleOf = schemaObject.multipleOf;
|
||||
}
|
||||
|
||||
if (schemaObject.maximum !== undefined) {
|
||||
template.maximum = schemaObject.maximum;
|
||||
}
|
||||
|
||||
if (schemaObject.exclusiveMaximum !== undefined) {
|
||||
template.exclusiveMaximum = schemaObject.exclusiveMaximum;
|
||||
}
|
||||
|
||||
if (schemaObject.minimum !== undefined) {
|
||||
template.minimum = schemaObject.minimum;
|
||||
}
|
||||
|
||||
if (schemaObject.exclusiveMinimum !== undefined) {
|
||||
template.exclusiveMinimum = schemaObject.exclusiveMinimum;
|
||||
}
|
||||
return template;
|
||||
};
|
||||
|
||||
const buildFloatCollectionInputFieldTemplate = ({
|
||||
schemaObject,
|
||||
baseField,
|
||||
}: BuildInputFieldArg): FloatCollectionInputFieldTemplate => {
|
||||
const item_default = isNumber(schemaObject.item_default)
|
||||
? schemaObject.item_default
|
||||
: 0;
|
||||
const template: FloatCollectionInputFieldTemplate = {
|
||||
...baseField,
|
||||
type: 'FloatCollection',
|
||||
default: schemaObject.default ?? [],
|
||||
item_default,
|
||||
};
|
||||
|
||||
return template;
|
||||
};
|
||||
|
||||
const buildStringInputFieldTemplate = ({
|
||||
schemaObject,
|
||||
baseField,
|
||||
@ -146,6 +271,48 @@ const buildStringInputFieldTemplate = ({
|
||||
return template;
|
||||
};
|
||||
|
||||
const buildStringPolymorphicInputFieldTemplate = ({
|
||||
schemaObject,
|
||||
baseField,
|
||||
}: BuildInputFieldArg): StringPolymorphicInputFieldTemplate => {
|
||||
const template: StringPolymorphicInputFieldTemplate = {
|
||||
...baseField,
|
||||
type: 'StringPolymorphic',
|
||||
default: schemaObject.default ?? '',
|
||||
};
|
||||
|
||||
if (schemaObject.minLength !== undefined) {
|
||||
template.minLength = schemaObject.minLength;
|
||||
}
|
||||
|
||||
if (schemaObject.maxLength !== undefined) {
|
||||
template.maxLength = schemaObject.maxLength;
|
||||
}
|
||||
|
||||
if (schemaObject.pattern !== undefined) {
|
||||
template.pattern = schemaObject.pattern;
|
||||
}
|
||||
|
||||
return template;
|
||||
};
|
||||
|
||||
const buildStringCollectionInputFieldTemplate = ({
|
||||
schemaObject,
|
||||
baseField,
|
||||
}: BuildInputFieldArg): StringCollectionInputFieldTemplate => {
|
||||
const item_default = isString(schemaObject.item_default)
|
||||
? schemaObject.item_default
|
||||
: '';
|
||||
const template: StringCollectionInputFieldTemplate = {
|
||||
...baseField,
|
||||
type: 'StringCollection',
|
||||
default: schemaObject.default ?? [],
|
||||
item_default,
|
||||
};
|
||||
|
||||
return template;
|
||||
};
|
||||
|
||||
const buildBooleanInputFieldTemplate = ({
|
||||
schemaObject,
|
||||
baseField,
|
||||
@ -159,6 +326,37 @@ const buildBooleanInputFieldTemplate = ({
|
||||
return template;
|
||||
};
|
||||
|
||||
const buildBooleanPolymorphicInputFieldTemplate = ({
|
||||
schemaObject,
|
||||
baseField,
|
||||
}: BuildInputFieldArg): BooleanPolymorphicInputFieldTemplate => {
|
||||
const template: BooleanPolymorphicInputFieldTemplate = {
|
||||
...baseField,
|
||||
type: 'BooleanPolymorphic',
|
||||
default: schemaObject.default ?? false,
|
||||
};
|
||||
|
||||
return template;
|
||||
};
|
||||
|
||||
const buildBooleanCollectionInputFieldTemplate = ({
|
||||
schemaObject,
|
||||
baseField,
|
||||
}: BuildInputFieldArg): BooleanCollectionInputFieldTemplate => {
|
||||
const item_default =
|
||||
schemaObject.item_default && isBoolean(schemaObject.item_default)
|
||||
? schemaObject.item_default
|
||||
: false;
|
||||
const template: BooleanCollectionInputFieldTemplate = {
|
||||
...baseField,
|
||||
type: 'BooleanCollection',
|
||||
default: schemaObject.default ?? [],
|
||||
item_default,
|
||||
};
|
||||
|
||||
return template;
|
||||
};
|
||||
|
||||
const buildMainModelInputFieldTemplate = ({
|
||||
schemaObject,
|
||||
baseField,
|
||||
@ -250,6 +448,19 @@ const buildImageInputFieldTemplate = ({
|
||||
return template;
|
||||
};
|
||||
|
||||
const buildImagePolymorphicInputFieldTemplate = ({
|
||||
schemaObject,
|
||||
baseField,
|
||||
}: BuildInputFieldArg): ImagePolymorphicInputFieldTemplate => {
|
||||
const template: ImagePolymorphicInputFieldTemplate = {
|
||||
...baseField,
|
||||
type: 'ImagePolymorphic',
|
||||
default: schemaObject.default ?? undefined,
|
||||
};
|
||||
|
||||
return template;
|
||||
};
|
||||
|
||||
const buildImageCollectionInputFieldTemplate = ({
|
||||
schemaObject,
|
||||
baseField,
|
||||
@ -257,7 +468,8 @@ const buildImageCollectionInputFieldTemplate = ({
|
||||
const template: ImageCollectionInputFieldTemplate = {
|
||||
...baseField,
|
||||
type: 'ImageCollection',
|
||||
default: schemaObject.default ?? undefined,
|
||||
default: schemaObject.default ?? [],
|
||||
item_default: (schemaObject.item_default as ImageField) ?? undefined,
|
||||
};
|
||||
|
||||
return template;
|
||||
@ -289,6 +501,33 @@ const buildLatentsInputFieldTemplate = ({
|
||||
return template;
|
||||
};
|
||||
|
||||
const buildLatentsPolymorphicInputFieldTemplate = ({
|
||||
schemaObject,
|
||||
baseField,
|
||||
}: BuildInputFieldArg): LatentsPolymorphicInputFieldTemplate => {
|
||||
const template: LatentsPolymorphicInputFieldTemplate = {
|
||||
...baseField,
|
||||
type: 'LatentsPolymorphic',
|
||||
default: schemaObject.default ?? undefined,
|
||||
};
|
||||
|
||||
return template;
|
||||
};
|
||||
|
||||
const buildLatentsCollectionInputFieldTemplate = ({
|
||||
schemaObject,
|
||||
baseField,
|
||||
}: BuildInputFieldArg): LatentsCollectionInputFieldTemplate => {
|
||||
const template: LatentsCollectionInputFieldTemplate = {
|
||||
...baseField,
|
||||
type: 'LatentsCollection',
|
||||
default: schemaObject.default ?? [],
|
||||
item_default: (schemaObject.item_default as LatentsField) ?? undefined,
|
||||
};
|
||||
|
||||
return template;
|
||||
};
|
||||
|
||||
const buildConditioningInputFieldTemplate = ({
|
||||
schemaObject,
|
||||
baseField,
|
||||
@ -302,6 +541,33 @@ const buildConditioningInputFieldTemplate = ({
|
||||
return template;
|
||||
};
|
||||
|
||||
const buildConditioningPolymorphicInputFieldTemplate = ({
|
||||
schemaObject,
|
||||
baseField,
|
||||
}: BuildInputFieldArg): ConditioningPolymorphicInputFieldTemplate => {
|
||||
const template: ConditioningPolymorphicInputFieldTemplate = {
|
||||
...baseField,
|
||||
type: 'ConditioningPolymorphic',
|
||||
default: schemaObject.default ?? undefined,
|
||||
};
|
||||
|
||||
return template;
|
||||
};
|
||||
|
||||
const buildConditioningCollectionInputFieldTemplate = ({
|
||||
schemaObject,
|
||||
baseField,
|
||||
}: BuildInputFieldArg): ConditioningCollectionInputFieldTemplate => {
|
||||
const template: ConditioningCollectionInputFieldTemplate = {
|
||||
...baseField,
|
||||
type: 'ConditioningCollection',
|
||||
default: schemaObject.default ?? [],
|
||||
item_default: (schemaObject.item_default as ConditioningField) ?? undefined,
|
||||
};
|
||||
|
||||
return template;
|
||||
};
|
||||
|
||||
const buildUNetInputFieldTemplate = ({
|
||||
schemaObject,
|
||||
baseField,
|
||||
@ -355,6 +621,33 @@ const buildControlInputFieldTemplate = ({
|
||||
return template;
|
||||
};
|
||||
|
||||
const buildControlPolymorphicInputFieldTemplate = ({
|
||||
schemaObject,
|
||||
baseField,
|
||||
}: BuildInputFieldArg): ControlPolymorphicInputFieldTemplate => {
|
||||
const template: ControlPolymorphicInputFieldTemplate = {
|
||||
...baseField,
|
||||
type: 'ControlPolymorphic',
|
||||
default: schemaObject.default ?? undefined,
|
||||
};
|
||||
|
||||
return template;
|
||||
};
|
||||
|
||||
const buildControlCollectionInputFieldTemplate = ({
|
||||
schemaObject,
|
||||
baseField,
|
||||
}: BuildInputFieldArg): ControlCollectionInputFieldTemplate => {
|
||||
const template: ControlCollectionInputFieldTemplate = {
|
||||
...baseField,
|
||||
type: 'ControlCollection',
|
||||
default: schemaObject.default ?? [],
|
||||
item_default: (schemaObject.item_default as ControlField) ?? undefined,
|
||||
};
|
||||
|
||||
return template;
|
||||
};
|
||||
|
||||
const buildEnumInputFieldTemplate = ({
|
||||
schemaObject,
|
||||
baseField,
|
||||
@ -408,6 +701,32 @@ const buildColorInputFieldTemplate = ({
|
||||
return template;
|
||||
};
|
||||
|
||||
const buildColorPolymorphicInputFieldTemplate = ({
|
||||
schemaObject,
|
||||
baseField,
|
||||
}: BuildInputFieldArg): ColorPolymorphicInputFieldTemplate => {
|
||||
const template: ColorPolymorphicInputFieldTemplate = {
|
||||
...baseField,
|
||||
type: 'ColorPolymorphic',
|
||||
default: schemaObject.default ?? { r: 127, g: 127, b: 127, a: 255 },
|
||||
};
|
||||
|
||||
return template;
|
||||
};
|
||||
|
||||
const buildColorCollectionInputFieldTemplate = ({
|
||||
schemaObject,
|
||||
baseField,
|
||||
}: BuildInputFieldArg): ColorCollectionInputFieldTemplate => {
|
||||
const template: ColorCollectionInputFieldTemplate = {
|
||||
...baseField,
|
||||
type: 'ColorCollection',
|
||||
default: schemaObject.default ?? [],
|
||||
};
|
||||
|
||||
return template;
|
||||
};
|
||||
|
||||
const buildSchedulerInputFieldTemplate = ({
|
||||
schemaObject,
|
||||
baseField,
|
||||
@ -421,45 +740,138 @@ const buildSchedulerInputFieldTemplate = ({
|
||||
return template;
|
||||
};
|
||||
|
||||
export const getFieldType = (schemaObject: InvocationFieldSchema): string => {
|
||||
let fieldType = '';
|
||||
|
||||
const { ui_type } = schemaObject;
|
||||
if (ui_type) {
|
||||
fieldType = ui_type;
|
||||
export const getFieldType = (
|
||||
schemaObject: InvocationFieldSchema
|
||||
): string | undefined => {
|
||||
if (schemaObject?.ui_type) {
|
||||
return schemaObject.ui_type;
|
||||
} else if (!schemaObject.type) {
|
||||
// console.log('refObject', schemaObject);
|
||||
// if schemaObject has no type, then it should have one of allOf, anyOf, oneOf
|
||||
|
||||
if (schemaObject.allOf) {
|
||||
fieldType = refObjectToFieldType(
|
||||
// eslint-disable-next-line @typescript-eslint/no-non-null-assertion
|
||||
schemaObject.allOf![0] as OpenAPIV3.ReferenceObject
|
||||
);
|
||||
const allOf = schemaObject.allOf;
|
||||
if (allOf && allOf[0] && isRefObject(allOf[0])) {
|
||||
return refObjectToSchemaName(allOf[0]);
|
||||
}
|
||||
} else if (schemaObject.anyOf) {
|
||||
fieldType = refObjectToFieldType(
|
||||
// eslint-disable-next-line @typescript-eslint/no-non-null-assertion
|
||||
schemaObject.anyOf![0] as OpenAPIV3.ReferenceObject
|
||||
);
|
||||
} else if (schemaObject.oneOf) {
|
||||
fieldType = refObjectToFieldType(
|
||||
// eslint-disable-next-line @typescript-eslint/no-non-null-assertion
|
||||
schemaObject.oneOf![0] as OpenAPIV3.ReferenceObject
|
||||
);
|
||||
const anyOf = schemaObject.anyOf;
|
||||
/**
|
||||
* Handle Polymorphic inputs, eg string | string[]. In OpenAPI, this is:
|
||||
* - an `anyOf` with two items
|
||||
* - one is an `ArraySchemaObject` with a single `SchemaObject or ReferenceObject` of type T in its `items`
|
||||
* - the other is a `SchemaObject` or `ReferenceObject` of type T
|
||||
*
|
||||
* Any other cases we ignore.
|
||||
*/
|
||||
|
||||
let firstType: string | undefined;
|
||||
let secondType: string | undefined;
|
||||
|
||||
if (isArraySchemaObject(anyOf[0])) {
|
||||
// first is array, second is not
|
||||
const first = anyOf[0].items;
|
||||
const second = anyOf[1];
|
||||
if (isRefObject(first) && isRefObject(second)) {
|
||||
firstType = refObjectToSchemaName(first);
|
||||
secondType = refObjectToSchemaName(second);
|
||||
} else if (
|
||||
isNonArraySchemaObject(first) &&
|
||||
isNonArraySchemaObject(second)
|
||||
) {
|
||||
firstType = first.type;
|
||||
secondType = second.type;
|
||||
}
|
||||
} else if (isArraySchemaObject(anyOf[1])) {
|
||||
// first is not array, second is
|
||||
const first = anyOf[0];
|
||||
const second = anyOf[1].items;
|
||||
if (isRefObject(first) && isRefObject(second)) {
|
||||
firstType = refObjectToSchemaName(first);
|
||||
secondType = refObjectToSchemaName(second);
|
||||
} else if (
|
||||
isNonArraySchemaObject(first) &&
|
||||
isNonArraySchemaObject(second)
|
||||
) {
|
||||
firstType = first.type;
|
||||
secondType = second.type;
|
||||
}
|
||||
}
|
||||
if (firstType === secondType && isPolymorphicItemType(firstType)) {
|
||||
return SINGLE_TO_POLYMORPHIC_MAP[firstType];
|
||||
}
|
||||
}
|
||||
} else if (schemaObject.enum) {
|
||||
fieldType = 'enum';
|
||||
return 'enum';
|
||||
} else if (schemaObject.type) {
|
||||
if (schemaObject.type === 'number') {
|
||||
// floats are "number" in OpenAPI, while ints are "integer"
|
||||
fieldType = 'float';
|
||||
// floats are "number" in OpenAPI, while ints are "integer" - we need to distinguish them
|
||||
return 'float';
|
||||
} else if (schemaObject.type === 'array') {
|
||||
const itemType = isSchemaObject(schemaObject.items)
|
||||
? schemaObject.items.type
|
||||
: refObjectToSchemaName(schemaObject.items);
|
||||
|
||||
if (isCollectionItemType(itemType)) {
|
||||
return COLLECTION_MAP[itemType];
|
||||
}
|
||||
|
||||
return;
|
||||
} else {
|
||||
fieldType = schemaObject.type;
|
||||
return schemaObject.type;
|
||||
}
|
||||
}
|
||||
|
||||
return fieldType;
|
||||
return;
|
||||
};
|
||||
|
||||
const TEMPLATE_BUILDER_MAP = {
|
||||
boolean: buildBooleanInputFieldTemplate,
|
||||
BooleanCollection: buildBooleanCollectionInputFieldTemplate,
|
||||
BooleanPolymorphic: buildBooleanPolymorphicInputFieldTemplate,
|
||||
ClipField: buildClipInputFieldTemplate,
|
||||
Collection: buildCollectionInputFieldTemplate,
|
||||
CollectionItem: buildCollectionItemInputFieldTemplate,
|
||||
ColorCollection: buildColorCollectionInputFieldTemplate,
|
||||
ColorField: buildColorInputFieldTemplate,
|
||||
ColorPolymorphic: buildColorPolymorphicInputFieldTemplate,
|
||||
ConditioningCollection: buildConditioningCollectionInputFieldTemplate,
|
||||
ConditioningField: buildConditioningInputFieldTemplate,
|
||||
ConditioningPolymorphic: buildConditioningPolymorphicInputFieldTemplate,
|
||||
ControlCollection: buildControlCollectionInputFieldTemplate,
|
||||
ControlField: buildControlInputFieldTemplate,
|
||||
ControlNetModelField: buildControlNetModelInputFieldTemplate,
|
||||
ControlPolymorphic: buildControlPolymorphicInputFieldTemplate,
|
||||
DenoiseMaskField: buildDenoiseMaskInputFieldTemplate,
|
||||
enum: buildEnumInputFieldTemplate,
|
||||
float: buildFloatInputFieldTemplate,
|
||||
FloatCollection: buildFloatCollectionInputFieldTemplate,
|
||||
FloatPolymorphic: buildFloatPolymorphicInputFieldTemplate,
|
||||
ImageCollection: buildImageCollectionInputFieldTemplate,
|
||||
ImageField: buildImageInputFieldTemplate,
|
||||
ImagePolymorphic: buildImagePolymorphicInputFieldTemplate,
|
||||
integer: buildIntegerInputFieldTemplate,
|
||||
IntegerCollection: buildIntegerCollectionInputFieldTemplate,
|
||||
IntegerPolymorphic: buildIntegerPolymorphicInputFieldTemplate,
|
||||
LatentsCollection: buildLatentsCollectionInputFieldTemplate,
|
||||
LatentsField: buildLatentsInputFieldTemplate,
|
||||
LatentsPolymorphic: buildLatentsPolymorphicInputFieldTemplate,
|
||||
LoRAModelField: buildLoRAModelInputFieldTemplate,
|
||||
MainModelField: buildMainModelInputFieldTemplate,
|
||||
Scheduler: buildSchedulerInputFieldTemplate,
|
||||
SDXLMainModelField: buildSDXLMainModelInputFieldTemplate,
|
||||
SDXLRefinerModelField: buildRefinerModelInputFieldTemplate,
|
||||
string: buildStringInputFieldTemplate,
|
||||
StringCollection: buildStringCollectionInputFieldTemplate,
|
||||
StringPolymorphic: buildStringPolymorphicInputFieldTemplate,
|
||||
UNetField: buildUNetInputFieldTemplate,
|
||||
VaeField: buildVaeInputFieldTemplate,
|
||||
VaeModelField: buildVaeModelInputFieldTemplate,
|
||||
};
|
||||
|
||||
const isTemplatedFieldType = (
|
||||
fieldType: string | undefined
|
||||
): fieldType is keyof typeof TEMPLATE_BUILDER_MAP =>
|
||||
Boolean(fieldType && fieldType in TEMPLATE_BUILDER_MAP);
|
||||
|
||||
/**
|
||||
* Builds an input field from an invocation schema property.
|
||||
* @param fieldSchema The schema object
|
||||
@ -474,7 +886,8 @@ export const buildInputFieldTemplate = (
|
||||
const { input, ui_hidden, ui_component, ui_type, ui_order } = fieldSchema;
|
||||
|
||||
const extra = {
|
||||
input,
|
||||
// TODO: Can we support polymorphic inputs in the UI?
|
||||
input: POLYMORPHIC_TYPES.includes(fieldType) ? 'connection' : input,
|
||||
ui_hidden,
|
||||
ui_component,
|
||||
ui_type,
|
||||
@ -490,146 +903,12 @@ export const buildInputFieldTemplate = (
|
||||
...extra,
|
||||
};
|
||||
|
||||
if (fieldType === 'ImageField') {
|
||||
return buildImageInputFieldTemplate({
|
||||
schemaObject: fieldSchema,
|
||||
baseField,
|
||||
});
|
||||
if (!isTemplatedFieldType(fieldType)) {
|
||||
return;
|
||||
}
|
||||
if (fieldType === 'ImageCollection') {
|
||||
return buildImageCollectionInputFieldTemplate({
|
||||
schemaObject: fieldSchema,
|
||||
baseField,
|
||||
});
|
||||
}
|
||||
if (fieldType === 'DenoiseMaskField') {
|
||||
return buildDenoiseMaskInputFieldTemplate({
|
||||
schemaObject: fieldSchema,
|
||||
baseField,
|
||||
});
|
||||
}
|
||||
if (fieldType === 'LatentsField') {
|
||||
return buildLatentsInputFieldTemplate({
|
||||
schemaObject: fieldSchema,
|
||||
baseField,
|
||||
});
|
||||
}
|
||||
if (fieldType === 'ConditioningField') {
|
||||
return buildConditioningInputFieldTemplate({
|
||||
schemaObject: fieldSchema,
|
||||
baseField,
|
||||
});
|
||||
}
|
||||
if (fieldType === 'UNetField') {
|
||||
return buildUNetInputFieldTemplate({
|
||||
schemaObject: fieldSchema,
|
||||
baseField,
|
||||
});
|
||||
}
|
||||
if (fieldType === 'ClipField') {
|
||||
return buildClipInputFieldTemplate({
|
||||
schemaObject: fieldSchema,
|
||||
baseField,
|
||||
});
|
||||
}
|
||||
if (fieldType === 'VaeField') {
|
||||
return buildVaeInputFieldTemplate({ schemaObject: fieldSchema, baseField });
|
||||
}
|
||||
if (fieldType === 'ControlField') {
|
||||
return buildControlInputFieldTemplate({
|
||||
schemaObject: fieldSchema,
|
||||
baseField,
|
||||
});
|
||||
}
|
||||
if (fieldType === 'MainModelField') {
|
||||
return buildMainModelInputFieldTemplate({
|
||||
schemaObject: fieldSchema,
|
||||
baseField,
|
||||
});
|
||||
}
|
||||
if (fieldType === 'SDXLRefinerModelField') {
|
||||
return buildRefinerModelInputFieldTemplate({
|
||||
schemaObject: fieldSchema,
|
||||
baseField,
|
||||
});
|
||||
}
|
||||
if (fieldType === 'SDXLMainModelField') {
|
||||
return buildSDXLMainModelInputFieldTemplate({
|
||||
schemaObject: fieldSchema,
|
||||
baseField,
|
||||
});
|
||||
}
|
||||
if (fieldType === 'VaeModelField') {
|
||||
return buildVaeModelInputFieldTemplate({
|
||||
schemaObject: fieldSchema,
|
||||
baseField,
|
||||
});
|
||||
}
|
||||
if (fieldType === 'LoRAModelField') {
|
||||
return buildLoRAModelInputFieldTemplate({
|
||||
schemaObject: fieldSchema,
|
||||
baseField,
|
||||
});
|
||||
}
|
||||
if (fieldType === 'ControlNetModelField') {
|
||||
return buildControlNetModelInputFieldTemplate({
|
||||
schemaObject: fieldSchema,
|
||||
baseField,
|
||||
});
|
||||
}
|
||||
if (fieldType === 'enum') {
|
||||
return buildEnumInputFieldTemplate({
|
||||
schemaObject: fieldSchema,
|
||||
baseField,
|
||||
});
|
||||
}
|
||||
if (fieldType === 'integer') {
|
||||
return buildIntegerInputFieldTemplate({
|
||||
schemaObject: fieldSchema,
|
||||
baseField,
|
||||
});
|
||||
}
|
||||
if (fieldType === 'float') {
|
||||
return buildFloatInputFieldTemplate({
|
||||
schemaObject: fieldSchema,
|
||||
baseField,
|
||||
});
|
||||
}
|
||||
if (fieldType === 'string') {
|
||||
return buildStringInputFieldTemplate({
|
||||
schemaObject: fieldSchema,
|
||||
baseField,
|
||||
});
|
||||
}
|
||||
if (fieldType === 'boolean') {
|
||||
return buildBooleanInputFieldTemplate({
|
||||
schemaObject: fieldSchema,
|
||||
baseField,
|
||||
});
|
||||
}
|
||||
if (fieldType === 'Collection') {
|
||||
return buildCollectionInputFieldTemplate({
|
||||
schemaObject: fieldSchema,
|
||||
baseField,
|
||||
});
|
||||
}
|
||||
if (fieldType === 'CollectionItem') {
|
||||
return buildCollectionItemInputFieldTemplate({
|
||||
schemaObject: fieldSchema,
|
||||
baseField,
|
||||
});
|
||||
}
|
||||
if (fieldType === 'ColorField') {
|
||||
return buildColorInputFieldTemplate({
|
||||
schemaObject: fieldSchema,
|
||||
baseField,
|
||||
});
|
||||
}
|
||||
if (fieldType === 'Scheduler') {
|
||||
return buildSchedulerInputFieldTemplate({
|
||||
schemaObject: fieldSchema,
|
||||
baseField,
|
||||
});
|
||||
}
|
||||
return;
|
||||
|
||||
return TEMPLATE_BUILDER_MAP[fieldType]({
|
||||
schemaObject: fieldSchema,
|
||||
baseField,
|
||||
});
|
||||
};
|
||||
|
@ -1,104 +1,79 @@
|
||||
import { InputFieldTemplate, InputFieldValue } from '../types/types';
|
||||
|
||||
const FIELD_VALUE_FALLBACK_MAP = {
|
||||
'enum.number': 0,
|
||||
'enum.string': '',
|
||||
boolean: false,
|
||||
BooleanCollection: [],
|
||||
BooleanPolymorphic: false,
|
||||
ClipField: undefined,
|
||||
Collection: [],
|
||||
CollectionItem: undefined,
|
||||
ColorCollection: [],
|
||||
ColorField: undefined,
|
||||
ColorPolymorphic: undefined,
|
||||
ConditioningCollection: [],
|
||||
ConditioningField: undefined,
|
||||
ConditioningPolymorphic: undefined,
|
||||
ControlCollection: [],
|
||||
ControlField: undefined,
|
||||
ControlNetModelField: undefined,
|
||||
ControlPolymorphic: undefined,
|
||||
DenoiseMaskField: undefined,
|
||||
float: 0,
|
||||
FloatCollection: [],
|
||||
FloatPolymorphic: 0,
|
||||
ImageCollection: [],
|
||||
ImageField: undefined,
|
||||
ImagePolymorphic: undefined,
|
||||
integer: 0,
|
||||
IntegerCollection: [],
|
||||
IntegerPolymorphic: 0,
|
||||
LatentsCollection: [],
|
||||
LatentsField: undefined,
|
||||
LatentsPolymorphic: undefined,
|
||||
LoRAModelField: undefined,
|
||||
MainModelField: undefined,
|
||||
ONNXModelField: undefined,
|
||||
Scheduler: 'euler',
|
||||
SDXLMainModelField: undefined,
|
||||
SDXLRefinerModelField: undefined,
|
||||
string: '',
|
||||
StringCollection: [],
|
||||
StringPolymorphic: '',
|
||||
UNetField: undefined,
|
||||
VaeField: undefined,
|
||||
VaeModelField: undefined,
|
||||
};
|
||||
|
||||
export const buildInputFieldValue = (
|
||||
id: string,
|
||||
template: InputFieldTemplate
|
||||
): InputFieldValue => {
|
||||
const fieldValue: InputFieldValue = {
|
||||
// TODO: this should be `fieldValue: InputFieldValue`, but that introduces a TS issue I couldn't
|
||||
// resolve - for some reason, it doesn't like `template.type`, which is the discriminant for both
|
||||
// `InputFieldTemplate` union. It is (type-structurally) equal to the discriminant for the
|
||||
// `InputFieldValue` union, but TS doesn't seem to like it...
|
||||
const fieldValue = {
|
||||
id,
|
||||
name: template.name,
|
||||
type: template.type,
|
||||
label: '',
|
||||
fieldKind: 'input',
|
||||
};
|
||||
|
||||
if (template.type === 'string') {
|
||||
fieldValue.value = template.default ?? '';
|
||||
}
|
||||
|
||||
if (template.type === 'integer') {
|
||||
fieldValue.value = template.default ?? 0;
|
||||
}
|
||||
|
||||
if (template.type === 'float') {
|
||||
fieldValue.value = template.default ?? 0;
|
||||
}
|
||||
|
||||
if (template.type === 'boolean') {
|
||||
fieldValue.value = template.default ?? false;
|
||||
}
|
||||
} as InputFieldValue;
|
||||
|
||||
if (template.type === 'enum') {
|
||||
if (template.enumType === 'number') {
|
||||
fieldValue.value = template.default ?? 0;
|
||||
fieldValue.value =
|
||||
template.default ?? FIELD_VALUE_FALLBACK_MAP['enum.number'];
|
||||
}
|
||||
if (template.enumType === 'string') {
|
||||
fieldValue.value = template.default ?? '';
|
||||
fieldValue.value =
|
||||
template.default ?? FIELD_VALUE_FALLBACK_MAP['enum.string'];
|
||||
}
|
||||
}
|
||||
|
||||
if (template.type === 'Collection') {
|
||||
fieldValue.value = template.default ?? 1;
|
||||
}
|
||||
|
||||
if (template.type === 'ImageField') {
|
||||
fieldValue.value = undefined;
|
||||
}
|
||||
|
||||
if (template.type === 'ImageCollection') {
|
||||
fieldValue.value = [];
|
||||
}
|
||||
|
||||
if (template.type === 'DenoiseMaskField') {
|
||||
fieldValue.value = undefined;
|
||||
}
|
||||
|
||||
if (template.type === 'LatentsField') {
|
||||
fieldValue.value = undefined;
|
||||
}
|
||||
|
||||
if (template.type === 'ConditioningField') {
|
||||
fieldValue.value = undefined;
|
||||
}
|
||||
|
||||
if (template.type === 'UNetField') {
|
||||
fieldValue.value = undefined;
|
||||
}
|
||||
|
||||
if (template.type === 'ClipField') {
|
||||
fieldValue.value = undefined;
|
||||
}
|
||||
|
||||
if (template.type === 'VaeField') {
|
||||
fieldValue.value = undefined;
|
||||
}
|
||||
|
||||
if (template.type === 'ControlField') {
|
||||
fieldValue.value = undefined;
|
||||
}
|
||||
|
||||
if (template.type === 'MainModelField') {
|
||||
fieldValue.value = undefined;
|
||||
}
|
||||
|
||||
if (template.type === 'SDXLRefinerModelField') {
|
||||
fieldValue.value = undefined;
|
||||
}
|
||||
|
||||
if (template.type === 'VaeModelField') {
|
||||
fieldValue.value = undefined;
|
||||
}
|
||||
|
||||
if (template.type === 'LoRAModelField') {
|
||||
fieldValue.value = undefined;
|
||||
}
|
||||
|
||||
if (template.type === 'ControlNetModelField') {
|
||||
fieldValue.value = undefined;
|
||||
}
|
||||
|
||||
if (template.type === 'Scheduler') {
|
||||
fieldValue.value = 'euler';
|
||||
} else {
|
||||
fieldValue.value =
|
||||
template.default ?? FIELD_VALUE_FALLBACK_MAP[template.type];
|
||||
}
|
||||
|
||||
return fieldValue;
|
||||
|
@ -10,7 +10,8 @@ import {
|
||||
CANVAS_OUTPUT,
|
||||
INPAINT_IMAGE_RESIZE_UP,
|
||||
LATENTS_TO_IMAGE,
|
||||
MASK_BLUR,
|
||||
MASK_COMBINE,
|
||||
MASK_RESIZE_UP,
|
||||
METADATA_ACCUMULATOR,
|
||||
SDXL_CANVAS_IMAGE_TO_IMAGE_GRAPH,
|
||||
SDXL_CANVAS_INPAINT_GRAPH,
|
||||
@ -46,6 +47,8 @@ export const addSDXLRefinerToGraph = (
|
||||
const { seamlessXAxis, seamlessYAxis, vaePrecision } = state.generation;
|
||||
const { boundingBoxScaleMethod } = state.canvas;
|
||||
|
||||
const fp32 = vaePrecision === 'fp32';
|
||||
|
||||
const isUsingScaledDimensions = ['auto', 'manual'].includes(
|
||||
boundingBoxScaleMethod
|
||||
);
|
||||
@ -231,7 +234,7 @@ export const addSDXLRefinerToGraph = (
|
||||
type: 'create_denoise_mask',
|
||||
id: SDXL_REFINER_INPAINT_CREATE_MASK,
|
||||
is_intermediate: true,
|
||||
fp32: vaePrecision === 'fp32' ? true : false,
|
||||
fp32,
|
||||
};
|
||||
|
||||
if (isUsingScaledDimensions) {
|
||||
@ -257,7 +260,7 @@ export const addSDXLRefinerToGraph = (
|
||||
graph.edges.push(
|
||||
{
|
||||
source: {
|
||||
node_id: MASK_BLUR,
|
||||
node_id: isUsingScaledDimensions ? MASK_RESIZE_UP : MASK_COMBINE,
|
||||
field: 'image',
|
||||
},
|
||||
destination: {
|
||||
|
@ -2,6 +2,7 @@ import { RootState } from 'app/store/store';
|
||||
import { NonNullableGraph } from 'features/nodes/types/types';
|
||||
import { MetadataAccumulatorInvocation } from 'services/api/types';
|
||||
import {
|
||||
CANVAS_COHERENCE_INPAINT_CREATE_MASK,
|
||||
CANVAS_IMAGE_TO_IMAGE_GRAPH,
|
||||
CANVAS_INPAINT_GRAPH,
|
||||
CANVAS_OUTPAINT_GRAPH,
|
||||
@ -31,7 +32,7 @@ export const addVAEToGraph = (
|
||||
graph: NonNullableGraph,
|
||||
modelLoaderNodeId: string = MAIN_MODEL_LOADER
|
||||
): void => {
|
||||
const { vae } = state.generation;
|
||||
const { vae, canvasCoherenceMode } = state.generation;
|
||||
const { boundingBoxScaleMethod } = state.canvas;
|
||||
const { shouldUseSDXLRefiner } = state.sdxl;
|
||||
|
||||
@ -146,6 +147,20 @@ export const addVAEToGraph = (
|
||||
},
|
||||
}
|
||||
);
|
||||
|
||||
// Handle Coherence Mode
|
||||
if (canvasCoherenceMode !== 'unmasked') {
|
||||
graph.edges.push({
|
||||
source: {
|
||||
node_id: isAutoVae ? modelLoaderNodeId : VAE_LOADER,
|
||||
field: isAutoVae && isOnnxModel ? 'vae_decoder' : 'vae',
|
||||
},
|
||||
destination: {
|
||||
node_id: CANVAS_COHERENCE_INPAINT_CREATE_MASK,
|
||||
field: 'vae',
|
||||
},
|
||||
});
|
||||
}
|
||||
}
|
||||
|
||||
if (shouldUseSDXLRefiner) {
|
||||
|
@ -59,6 +59,8 @@ export const buildCanvasImageToImageGraph = (
|
||||
shouldAutoSave,
|
||||
} = state.canvas;
|
||||
|
||||
const fp32 = vaePrecision === 'fp32';
|
||||
|
||||
const isUsingScaledDimensions = ['auto', 'manual'].includes(
|
||||
boundingBoxScaleMethod
|
||||
);
|
||||
@ -245,7 +247,7 @@ export const buildCanvasImageToImageGraph = (
|
||||
id: LATENTS_TO_IMAGE,
|
||||
type: 'l2i',
|
||||
is_intermediate: true,
|
||||
fp32: vaePrecision === 'fp32' ? true : false,
|
||||
fp32,
|
||||
};
|
||||
graph.nodes[CANVAS_OUTPUT] = {
|
||||
id: CANVAS_OUTPUT,
|
||||
@ -292,7 +294,7 @@ export const buildCanvasImageToImageGraph = (
|
||||
type: 'l2i',
|
||||
id: CANVAS_OUTPUT,
|
||||
is_intermediate: !shouldAutoSave,
|
||||
fp32: vaePrecision === 'fp32' ? true : false,
|
||||
fp32,
|
||||
};
|
||||
|
||||
(graph.nodes[IMAGE_TO_LATENTS] as ImageToLatentsInvocation).image =
|
||||
|
@ -6,6 +6,7 @@ import {
|
||||
ImageBlurInvocation,
|
||||
ImageDTO,
|
||||
ImageToLatentsInvocation,
|
||||
MaskEdgeInvocation,
|
||||
NoiseInvocation,
|
||||
RandomIntInvocation,
|
||||
RangeOfSizeInvocation,
|
||||
@ -18,6 +19,8 @@ import { addVAEToGraph } from './addVAEToGraph';
|
||||
import { addWatermarkerToGraph } from './addWatermarkerToGraph';
|
||||
import {
|
||||
CANVAS_COHERENCE_DENOISE_LATENTS,
|
||||
CANVAS_COHERENCE_INPAINT_CREATE_MASK,
|
||||
CANVAS_COHERENCE_MASK_EDGE,
|
||||
CANVAS_COHERENCE_NOISE,
|
||||
CANVAS_COHERENCE_NOISE_INCREMENT,
|
||||
CANVAS_INPAINT_GRAPH,
|
||||
@ -67,6 +70,7 @@ export const buildCanvasInpaintGraph = (
|
||||
shouldUseCpuNoise,
|
||||
maskBlur,
|
||||
maskBlurMethod,
|
||||
canvasCoherenceMode,
|
||||
canvasCoherenceSteps,
|
||||
canvasCoherenceStrength,
|
||||
clipSkip,
|
||||
@ -89,6 +93,12 @@ export const buildCanvasInpaintGraph = (
|
||||
shouldAutoSave,
|
||||
} = state.canvas;
|
||||
|
||||
const fp32 = vaePrecision === 'fp32';
|
||||
|
||||
const isUsingScaledDimensions = ['auto', 'manual'].includes(
|
||||
boundingBoxScaleMethod
|
||||
);
|
||||
|
||||
let modelLoaderNodeId = MAIN_MODEL_LOADER;
|
||||
|
||||
const use_cpu = shouldUseNoiseSettings
|
||||
@ -133,13 +143,7 @@ export const buildCanvasInpaintGraph = (
|
||||
type: 'i2l',
|
||||
id: INPAINT_IMAGE,
|
||||
is_intermediate: true,
|
||||
fp32: vaePrecision === 'fp32' ? true : false,
|
||||
},
|
||||
[INPAINT_CREATE_MASK]: {
|
||||
type: 'create_denoise_mask',
|
||||
id: INPAINT_CREATE_MASK,
|
||||
is_intermediate: true,
|
||||
fp32: vaePrecision === 'fp32' ? true : false,
|
||||
fp32,
|
||||
},
|
||||
[NOISE]: {
|
||||
type: 'noise',
|
||||
@ -147,6 +151,12 @@ export const buildCanvasInpaintGraph = (
|
||||
use_cpu,
|
||||
is_intermediate: true,
|
||||
},
|
||||
[INPAINT_CREATE_MASK]: {
|
||||
type: 'create_denoise_mask',
|
||||
id: INPAINT_CREATE_MASK,
|
||||
is_intermediate: true,
|
||||
fp32,
|
||||
},
|
||||
[DENOISE_LATENTS]: {
|
||||
type: 'denoise_latents',
|
||||
id: DENOISE_LATENTS,
|
||||
@ -171,7 +181,7 @@ export const buildCanvasInpaintGraph = (
|
||||
},
|
||||
[CANVAS_COHERENCE_DENOISE_LATENTS]: {
|
||||
type: 'denoise_latents',
|
||||
id: DENOISE_LATENTS,
|
||||
id: CANVAS_COHERENCE_DENOISE_LATENTS,
|
||||
is_intermediate: true,
|
||||
steps: canvasCoherenceSteps,
|
||||
cfg_scale: cfg_scale,
|
||||
@ -183,7 +193,7 @@ export const buildCanvasInpaintGraph = (
|
||||
type: 'l2i',
|
||||
id: LATENTS_TO_IMAGE,
|
||||
is_intermediate: true,
|
||||
fp32: vaePrecision === 'fp32' ? true : false,
|
||||
fp32,
|
||||
},
|
||||
[CANVAS_OUTPUT]: {
|
||||
type: 'color_correct',
|
||||
@ -418,7 +428,7 @@ export const buildCanvasInpaintGraph = (
|
||||
};
|
||||
|
||||
// Handle Scale Before Processing
|
||||
if (['auto', 'manual'].includes(boundingBoxScaleMethod)) {
|
||||
if (isUsingScaledDimensions) {
|
||||
const scaledWidth: number = scaledBoundingBoxDimensions.width;
|
||||
const scaledHeight: number = scaledBoundingBoxDimensions.height;
|
||||
|
||||
@ -581,6 +591,116 @@ export const buildCanvasInpaintGraph = (
|
||||
);
|
||||
}
|
||||
|
||||
// Handle Coherence Mode
|
||||
if (canvasCoherenceMode !== 'unmasked') {
|
||||
// Create Mask If Coherence Mode Is Not Full
|
||||
graph.nodes[CANVAS_COHERENCE_INPAINT_CREATE_MASK] = {
|
||||
type: 'create_denoise_mask',
|
||||
id: CANVAS_COHERENCE_INPAINT_CREATE_MASK,
|
||||
is_intermediate: true,
|
||||
fp32,
|
||||
};
|
||||
|
||||
// Handle Image Input For Mask Creation
|
||||
if (isUsingScaledDimensions) {
|
||||
graph.edges.push({
|
||||
source: {
|
||||
node_id: INPAINT_IMAGE_RESIZE_UP,
|
||||
field: 'image',
|
||||
},
|
||||
destination: {
|
||||
node_id: CANVAS_COHERENCE_INPAINT_CREATE_MASK,
|
||||
field: 'image',
|
||||
},
|
||||
});
|
||||
} else {
|
||||
graph.nodes[CANVAS_COHERENCE_INPAINT_CREATE_MASK] = {
|
||||
...(graph.nodes[
|
||||
CANVAS_COHERENCE_INPAINT_CREATE_MASK
|
||||
] as CreateDenoiseMaskInvocation),
|
||||
image: canvasInitImage,
|
||||
};
|
||||
}
|
||||
|
||||
// Create Mask If Coherence Mode Is Mask
|
||||
if (canvasCoherenceMode === 'mask') {
|
||||
if (isUsingScaledDimensions) {
|
||||
graph.edges.push({
|
||||
source: {
|
||||
node_id: MASK_RESIZE_UP,
|
||||
field: 'image',
|
||||
},
|
||||
destination: {
|
||||
node_id: CANVAS_COHERENCE_INPAINT_CREATE_MASK,
|
||||
field: 'mask',
|
||||
},
|
||||
});
|
||||
} else {
|
||||
graph.nodes[CANVAS_COHERENCE_INPAINT_CREATE_MASK] = {
|
||||
...(graph.nodes[
|
||||
CANVAS_COHERENCE_INPAINT_CREATE_MASK
|
||||
] as CreateDenoiseMaskInvocation),
|
||||
mask: canvasMaskImage,
|
||||
};
|
||||
}
|
||||
}
|
||||
|
||||
// Create Mask Edge If Coherence Mode Is Edge
|
||||
if (canvasCoherenceMode === 'edge') {
|
||||
graph.nodes[CANVAS_COHERENCE_MASK_EDGE] = {
|
||||
type: 'mask_edge',
|
||||
id: CANVAS_COHERENCE_MASK_EDGE,
|
||||
is_intermediate: true,
|
||||
edge_blur: maskBlur,
|
||||
edge_size: maskBlur * 2,
|
||||
low_threshold: 100,
|
||||
high_threshold: 200,
|
||||
};
|
||||
|
||||
// Handle Scaled Dimensions For Mask Edge
|
||||
if (isUsingScaledDimensions) {
|
||||
graph.edges.push({
|
||||
source: {
|
||||
node_id: MASK_RESIZE_UP,
|
||||
field: 'image',
|
||||
},
|
||||
destination: {
|
||||
node_id: CANVAS_COHERENCE_MASK_EDGE,
|
||||
field: 'image',
|
||||
},
|
||||
});
|
||||
} else {
|
||||
graph.nodes[CANVAS_COHERENCE_MASK_EDGE] = {
|
||||
...(graph.nodes[CANVAS_COHERENCE_MASK_EDGE] as MaskEdgeInvocation),
|
||||
image: canvasMaskImage,
|
||||
};
|
||||
}
|
||||
|
||||
graph.edges.push({
|
||||
source: {
|
||||
node_id: CANVAS_COHERENCE_MASK_EDGE,
|
||||
field: 'image',
|
||||
},
|
||||
destination: {
|
||||
node_id: CANVAS_COHERENCE_INPAINT_CREATE_MASK,
|
||||
field: 'mask',
|
||||
},
|
||||
});
|
||||
}
|
||||
|
||||
// Plug Denoise Mask To Coherence Denoise Latents
|
||||
graph.edges.push({
|
||||
source: {
|
||||
node_id: CANVAS_COHERENCE_INPAINT_CREATE_MASK,
|
||||
field: 'denoise_mask',
|
||||
},
|
||||
destination: {
|
||||
node_id: CANVAS_COHERENCE_DENOISE_LATENTS,
|
||||
field: 'denoise_mask',
|
||||
},
|
||||
});
|
||||
}
|
||||
|
||||
// Handle Seed
|
||||
if (shouldRandomizeSeed) {
|
||||
// Random int node to generate the starting seed
|
||||
|
@ -2,7 +2,6 @@ import { logger } from 'app/logging/logger';
|
||||
import { RootState } from 'app/store/store';
|
||||
import { NonNullableGraph } from 'features/nodes/types/types';
|
||||
import {
|
||||
ImageBlurInvocation,
|
||||
ImageDTO,
|
||||
ImageToLatentsInvocation,
|
||||
InfillPatchMatchInvocation,
|
||||
@ -19,6 +18,8 @@ import { addVAEToGraph } from './addVAEToGraph';
|
||||
import { addWatermarkerToGraph } from './addWatermarkerToGraph';
|
||||
import {
|
||||
CANVAS_COHERENCE_DENOISE_LATENTS,
|
||||
CANVAS_COHERENCE_INPAINT_CREATE_MASK,
|
||||
CANVAS_COHERENCE_MASK_EDGE,
|
||||
CANVAS_COHERENCE_NOISE,
|
||||
CANVAS_COHERENCE_NOISE_INCREMENT,
|
||||
CANVAS_OUTPAINT_GRAPH,
|
||||
@ -34,7 +35,6 @@ import {
|
||||
ITERATE,
|
||||
LATENTS_TO_IMAGE,
|
||||
MAIN_MODEL_LOADER,
|
||||
MASK_BLUR,
|
||||
MASK_COMBINE,
|
||||
MASK_FROM_ALPHA,
|
||||
MASK_RESIZE_DOWN,
|
||||
@ -71,10 +71,11 @@ export const buildCanvasOutpaintGraph = (
|
||||
shouldUseNoiseSettings,
|
||||
shouldUseCpuNoise,
|
||||
maskBlur,
|
||||
maskBlurMethod,
|
||||
canvasCoherenceMode,
|
||||
canvasCoherenceSteps,
|
||||
canvasCoherenceStrength,
|
||||
tileSize,
|
||||
infillTileSize,
|
||||
infillPatchmatchDownscaleSize,
|
||||
infillMethod,
|
||||
clipSkip,
|
||||
seamlessXAxis,
|
||||
@ -96,6 +97,12 @@ export const buildCanvasOutpaintGraph = (
|
||||
shouldAutoSave,
|
||||
} = state.canvas;
|
||||
|
||||
const fp32 = vaePrecision === 'fp32';
|
||||
|
||||
const isUsingScaledDimensions = ['auto', 'manual'].includes(
|
||||
boundingBoxScaleMethod
|
||||
);
|
||||
|
||||
let modelLoaderNodeId = MAIN_MODEL_LOADER;
|
||||
|
||||
const use_cpu = shouldUseNoiseSettings
|
||||
@ -141,18 +148,11 @@ export const buildCanvasOutpaintGraph = (
|
||||
is_intermediate: true,
|
||||
mask2: canvasMaskImage,
|
||||
},
|
||||
[MASK_BLUR]: {
|
||||
type: 'img_blur',
|
||||
id: MASK_BLUR,
|
||||
is_intermediate: true,
|
||||
radius: maskBlur,
|
||||
blur_type: maskBlurMethod,
|
||||
},
|
||||
[INPAINT_IMAGE]: {
|
||||
type: 'i2l',
|
||||
id: INPAINT_IMAGE,
|
||||
is_intermediate: true,
|
||||
fp32: vaePrecision === 'fp32' ? true : false,
|
||||
fp32,
|
||||
},
|
||||
[NOISE]: {
|
||||
type: 'noise',
|
||||
@ -164,7 +164,7 @@ export const buildCanvasOutpaintGraph = (
|
||||
type: 'create_denoise_mask',
|
||||
id: INPAINT_CREATE_MASK,
|
||||
is_intermediate: true,
|
||||
fp32: vaePrecision === 'fp32' ? true : false,
|
||||
fp32,
|
||||
},
|
||||
[DENOISE_LATENTS]: {
|
||||
type: 'denoise_latents',
|
||||
@ -202,7 +202,7 @@ export const buildCanvasOutpaintGraph = (
|
||||
type: 'l2i',
|
||||
id: LATENTS_TO_IMAGE,
|
||||
is_intermediate: true,
|
||||
fp32: vaePrecision === 'fp32' ? true : false,
|
||||
fp32,
|
||||
},
|
||||
[CANVAS_OUTPUT]: {
|
||||
type: 'color_correct',
|
||||
@ -333,7 +333,7 @@ export const buildCanvasOutpaintGraph = (
|
||||
// Create Inpaint Mask
|
||||
{
|
||||
source: {
|
||||
node_id: MASK_BLUR,
|
||||
node_id: isUsingScaledDimensions ? MASK_RESIZE_UP : MASK_COMBINE,
|
||||
field: 'image',
|
||||
},
|
||||
destination: {
|
||||
@ -443,6 +443,16 @@ export const buildCanvasOutpaintGraph = (
|
||||
field: 'latents',
|
||||
},
|
||||
},
|
||||
{
|
||||
source: {
|
||||
node_id: INPAINT_INFILL,
|
||||
field: 'image',
|
||||
},
|
||||
destination: {
|
||||
node_id: INPAINT_CREATE_MASK,
|
||||
field: 'image',
|
||||
},
|
||||
},
|
||||
// Decode the result from Inpaint
|
||||
{
|
||||
source: {
|
||||
@ -463,6 +473,7 @@ export const buildCanvasOutpaintGraph = (
|
||||
type: 'infill_patchmatch',
|
||||
id: INPAINT_INFILL,
|
||||
is_intermediate: true,
|
||||
downscale: infillPatchmatchDownscaleSize,
|
||||
};
|
||||
}
|
||||
|
||||
@ -474,17 +485,25 @@ export const buildCanvasOutpaintGraph = (
|
||||
};
|
||||
}
|
||||
|
||||
if (infillMethod === 'cv2') {
|
||||
graph.nodes[INPAINT_INFILL] = {
|
||||
type: 'infill_cv2',
|
||||
id: INPAINT_INFILL,
|
||||
is_intermediate: true,
|
||||
};
|
||||
}
|
||||
|
||||
if (infillMethod === 'tile') {
|
||||
graph.nodes[INPAINT_INFILL] = {
|
||||
type: 'infill_tile',
|
||||
id: INPAINT_INFILL,
|
||||
is_intermediate: true,
|
||||
tile_size: tileSize,
|
||||
tile_size: infillTileSize,
|
||||
};
|
||||
}
|
||||
|
||||
// Handle Scale Before Processing
|
||||
if (['auto', 'manual'].includes(boundingBoxScaleMethod)) {
|
||||
if (isUsingScaledDimensions) {
|
||||
const scaledWidth: number = scaledBoundingBoxDimensions.width;
|
||||
const scaledHeight: number = scaledBoundingBoxDimensions.height;
|
||||
|
||||
@ -546,16 +565,6 @@ export const buildCanvasOutpaintGraph = (
|
||||
field: 'image',
|
||||
},
|
||||
},
|
||||
{
|
||||
source: {
|
||||
node_id: INPAINT_INFILL,
|
||||
field: 'image',
|
||||
},
|
||||
destination: {
|
||||
node_id: INPAINT_CREATE_MASK,
|
||||
field: 'image',
|
||||
},
|
||||
},
|
||||
// Take combined mask and resize and then blur
|
||||
{
|
||||
source: {
|
||||
@ -567,16 +576,7 @@ export const buildCanvasOutpaintGraph = (
|
||||
field: 'image',
|
||||
},
|
||||
},
|
||||
{
|
||||
source: {
|
||||
node_id: MASK_RESIZE_UP,
|
||||
field: 'image',
|
||||
},
|
||||
destination: {
|
||||
node_id: MASK_BLUR,
|
||||
field: 'image',
|
||||
},
|
||||
},
|
||||
|
||||
// Resize Results Down
|
||||
{
|
||||
source: {
|
||||
@ -658,32 +658,8 @@ export const buildCanvasOutpaintGraph = (
|
||||
...(graph.nodes[INPAINT_IMAGE] as ImageToLatentsInvocation),
|
||||
image: canvasInitImage,
|
||||
};
|
||||
graph.nodes[MASK_BLUR] = {
|
||||
...(graph.nodes[MASK_BLUR] as ImageBlurInvocation),
|
||||
};
|
||||
|
||||
graph.edges.push(
|
||||
// Take combined mask and plug it to blur
|
||||
{
|
||||
source: {
|
||||
node_id: MASK_COMBINE,
|
||||
field: 'image',
|
||||
},
|
||||
destination: {
|
||||
node_id: MASK_BLUR,
|
||||
field: 'image',
|
||||
},
|
||||
},
|
||||
{
|
||||
source: {
|
||||
node_id: INPAINT_INFILL,
|
||||
field: 'image',
|
||||
},
|
||||
destination: {
|
||||
node_id: INPAINT_CREATE_MASK,
|
||||
field: 'image',
|
||||
},
|
||||
},
|
||||
// Color Correct The Inpainted Result
|
||||
{
|
||||
source: {
|
||||
@ -707,7 +683,7 @@ export const buildCanvasOutpaintGraph = (
|
||||
},
|
||||
{
|
||||
source: {
|
||||
node_id: MASK_BLUR,
|
||||
node_id: MASK_COMBINE,
|
||||
field: 'image',
|
||||
},
|
||||
destination: {
|
||||
@ -718,6 +694,115 @@ export const buildCanvasOutpaintGraph = (
|
||||
);
|
||||
}
|
||||
|
||||
// Handle Coherence Mode
|
||||
if (canvasCoherenceMode !== 'unmasked') {
|
||||
graph.nodes[CANVAS_COHERENCE_INPAINT_CREATE_MASK] = {
|
||||
type: 'create_denoise_mask',
|
||||
id: CANVAS_COHERENCE_INPAINT_CREATE_MASK,
|
||||
is_intermediate: true,
|
||||
fp32,
|
||||
};
|
||||
|
||||
// Handle Image Input For Mask Creation
|
||||
graph.edges.push({
|
||||
source: {
|
||||
node_id: INPAINT_INFILL,
|
||||
field: 'image',
|
||||
},
|
||||
destination: {
|
||||
node_id: CANVAS_COHERENCE_INPAINT_CREATE_MASK,
|
||||
field: 'image',
|
||||
},
|
||||
});
|
||||
|
||||
// Create Mask If Coherence Mode Is Mask
|
||||
if (canvasCoherenceMode === 'mask') {
|
||||
if (isUsingScaledDimensions) {
|
||||
graph.edges.push({
|
||||
source: {
|
||||
node_id: MASK_RESIZE_UP,
|
||||
field: 'image',
|
||||
},
|
||||
destination: {
|
||||
node_id: CANVAS_COHERENCE_INPAINT_CREATE_MASK,
|
||||
field: 'mask',
|
||||
},
|
||||
});
|
||||
} else {
|
||||
graph.edges.push({
|
||||
source: {
|
||||
node_id: MASK_COMBINE,
|
||||
field: 'image',
|
||||
},
|
||||
destination: {
|
||||
node_id: CANVAS_COHERENCE_INPAINT_CREATE_MASK,
|
||||
field: 'mask',
|
||||
},
|
||||
});
|
||||
}
|
||||
}
|
||||
|
||||
if (canvasCoherenceMode === 'edge') {
|
||||
graph.nodes[CANVAS_COHERENCE_MASK_EDGE] = {
|
||||
type: 'mask_edge',
|
||||
id: CANVAS_COHERENCE_MASK_EDGE,
|
||||
is_intermediate: true,
|
||||
edge_blur: maskBlur,
|
||||
edge_size: maskBlur * 2,
|
||||
low_threshold: 100,
|
||||
high_threshold: 200,
|
||||
};
|
||||
|
||||
// Handle Scaled Dimensions For Mask Edge
|
||||
if (isUsingScaledDimensions) {
|
||||
graph.edges.push({
|
||||
source: {
|
||||
node_id: MASK_RESIZE_UP,
|
||||
field: 'image',
|
||||
},
|
||||
destination: {
|
||||
node_id: CANVAS_COHERENCE_MASK_EDGE,
|
||||
field: 'image',
|
||||
},
|
||||
});
|
||||
} else {
|
||||
graph.edges.push({
|
||||
source: {
|
||||
node_id: MASK_COMBINE,
|
||||
field: 'image',
|
||||
},
|
||||
destination: {
|
||||
node_id: CANVAS_COHERENCE_MASK_EDGE,
|
||||
field: 'image',
|
||||
},
|
||||
});
|
||||
}
|
||||
|
||||
graph.edges.push({
|
||||
source: {
|
||||
node_id: CANVAS_COHERENCE_MASK_EDGE,
|
||||
field: 'image',
|
||||
},
|
||||
destination: {
|
||||
node_id: CANVAS_COHERENCE_INPAINT_CREATE_MASK,
|
||||
field: 'mask',
|
||||
},
|
||||
});
|
||||
}
|
||||
|
||||
// Plug Denoise Mask To Coherence Denoise Latents
|
||||
graph.edges.push({
|
||||
source: {
|
||||
node_id: CANVAS_COHERENCE_INPAINT_CREATE_MASK,
|
||||
field: 'denoise_mask',
|
||||
},
|
||||
destination: {
|
||||
node_id: CANVAS_COHERENCE_DENOISE_LATENTS,
|
||||
field: 'denoise_mask',
|
||||
},
|
||||
});
|
||||
}
|
||||
|
||||
// Handle Seed
|
||||
if (shouldRandomizeSeed) {
|
||||
// Random int node to generate the starting seed
|
||||
|
@ -67,6 +67,8 @@ export const buildCanvasSDXLImageToImageGraph = (
|
||||
shouldAutoSave,
|
||||
} = state.canvas;
|
||||
|
||||
const fp32 = vaePrecision === 'fp32';
|
||||
|
||||
const isUsingScaledDimensions = ['auto', 'manual'].includes(
|
||||
boundingBoxScaleMethod
|
||||
);
|
||||
@ -133,7 +135,7 @@ export const buildCanvasSDXLImageToImageGraph = (
|
||||
type: 'i2l',
|
||||
id: IMAGE_TO_LATENTS,
|
||||
is_intermediate: true,
|
||||
fp32: vaePrecision === 'fp32' ? true : false,
|
||||
fp32,
|
||||
},
|
||||
[SDXL_DENOISE_LATENTS]: {
|
||||
type: 'denoise_latents',
|
||||
@ -258,7 +260,7 @@ export const buildCanvasSDXLImageToImageGraph = (
|
||||
id: LATENTS_TO_IMAGE,
|
||||
type: 'l2i',
|
||||
is_intermediate: true,
|
||||
fp32: vaePrecision === 'fp32' ? true : false,
|
||||
fp32,
|
||||
};
|
||||
graph.nodes[CANVAS_OUTPUT] = {
|
||||
id: CANVAS_OUTPUT,
|
||||
@ -305,7 +307,7 @@ export const buildCanvasSDXLImageToImageGraph = (
|
||||
type: 'l2i',
|
||||
id: CANVAS_OUTPUT,
|
||||
is_intermediate: !shouldAutoSave,
|
||||
fp32: vaePrecision === 'fp32' ? true : false,
|
||||
fp32,
|
||||
};
|
||||
|
||||
(graph.nodes[IMAGE_TO_LATENTS] as ImageToLatentsInvocation).image =
|
||||
|
@ -6,6 +6,7 @@ import {
|
||||
ImageBlurInvocation,
|
||||
ImageDTO,
|
||||
ImageToLatentsInvocation,
|
||||
MaskEdgeInvocation,
|
||||
NoiseInvocation,
|
||||
RandomIntInvocation,
|
||||
RangeOfSizeInvocation,
|
||||
@ -19,6 +20,8 @@ import { addVAEToGraph } from './addVAEToGraph';
|
||||
import { addWatermarkerToGraph } from './addWatermarkerToGraph';
|
||||
import {
|
||||
CANVAS_COHERENCE_DENOISE_LATENTS,
|
||||
CANVAS_COHERENCE_INPAINT_CREATE_MASK,
|
||||
CANVAS_COHERENCE_MASK_EDGE,
|
||||
CANVAS_COHERENCE_NOISE,
|
||||
CANVAS_COHERENCE_NOISE_INCREMENT,
|
||||
CANVAS_OUTPUT,
|
||||
@ -68,6 +71,7 @@ export const buildCanvasSDXLInpaintGraph = (
|
||||
shouldUseCpuNoise,
|
||||
maskBlur,
|
||||
maskBlurMethod,
|
||||
canvasCoherenceMode,
|
||||
canvasCoherenceSteps,
|
||||
canvasCoherenceStrength,
|
||||
seamlessXAxis,
|
||||
@ -96,6 +100,12 @@ export const buildCanvasSDXLInpaintGraph = (
|
||||
shouldAutoSave,
|
||||
} = state.canvas;
|
||||
|
||||
const fp32 = vaePrecision === 'fp32';
|
||||
|
||||
const isUsingScaledDimensions = ['auto', 'manual'].includes(
|
||||
boundingBoxScaleMethod
|
||||
);
|
||||
|
||||
let modelLoaderNodeId = SDXL_MODEL_LOADER;
|
||||
|
||||
const use_cpu = shouldUseNoiseSettings
|
||||
@ -137,7 +147,7 @@ export const buildCanvasSDXLInpaintGraph = (
|
||||
type: 'i2l',
|
||||
id: INPAINT_IMAGE,
|
||||
is_intermediate: true,
|
||||
fp32: vaePrecision === 'fp32' ? true : false,
|
||||
fp32,
|
||||
},
|
||||
[NOISE]: {
|
||||
type: 'noise',
|
||||
@ -149,7 +159,7 @@ export const buildCanvasSDXLInpaintGraph = (
|
||||
type: 'create_denoise_mask',
|
||||
id: INPAINT_CREATE_MASK,
|
||||
is_intermediate: true,
|
||||
fp32: vaePrecision === 'fp32' ? true : false,
|
||||
fp32,
|
||||
},
|
||||
[SDXL_DENOISE_LATENTS]: {
|
||||
type: 'denoise_latents',
|
||||
@ -177,7 +187,7 @@ export const buildCanvasSDXLInpaintGraph = (
|
||||
},
|
||||
[CANVAS_COHERENCE_DENOISE_LATENTS]: {
|
||||
type: 'denoise_latents',
|
||||
id: SDXL_DENOISE_LATENTS,
|
||||
id: CANVAS_COHERENCE_DENOISE_LATENTS,
|
||||
is_intermediate: true,
|
||||
steps: canvasCoherenceSteps,
|
||||
cfg_scale: cfg_scale,
|
||||
@ -189,7 +199,7 @@ export const buildCanvasSDXLInpaintGraph = (
|
||||
type: 'l2i',
|
||||
id: LATENTS_TO_IMAGE,
|
||||
is_intermediate: true,
|
||||
fp32: vaePrecision === 'fp32' ? true : false,
|
||||
fp32,
|
||||
},
|
||||
[CANVAS_OUTPUT]: {
|
||||
type: 'color_correct',
|
||||
@ -433,7 +443,7 @@ export const buildCanvasSDXLInpaintGraph = (
|
||||
};
|
||||
|
||||
// Handle Scale Before Processing
|
||||
if (['auto', 'manual'].includes(boundingBoxScaleMethod)) {
|
||||
if (isUsingScaledDimensions) {
|
||||
const scaledWidth: number = scaledBoundingBoxDimensions.width;
|
||||
const scaledHeight: number = scaledBoundingBoxDimensions.height;
|
||||
|
||||
@ -596,6 +606,116 @@ export const buildCanvasSDXLInpaintGraph = (
|
||||
);
|
||||
}
|
||||
|
||||
// Handle Coherence Mode
|
||||
if (canvasCoherenceMode !== 'unmasked') {
|
||||
// Create Mask If Coherence Mode Is Not Full
|
||||
graph.nodes[CANVAS_COHERENCE_INPAINT_CREATE_MASK] = {
|
||||
type: 'create_denoise_mask',
|
||||
id: CANVAS_COHERENCE_INPAINT_CREATE_MASK,
|
||||
is_intermediate: true,
|
||||
fp32,
|
||||
};
|
||||
|
||||
// Handle Image Input For Mask Creation
|
||||
if (isUsingScaledDimensions) {
|
||||
graph.edges.push({
|
||||
source: {
|
||||
node_id: INPAINT_IMAGE_RESIZE_UP,
|
||||
field: 'image',
|
||||
},
|
||||
destination: {
|
||||
node_id: CANVAS_COHERENCE_INPAINT_CREATE_MASK,
|
||||
field: 'image',
|
||||
},
|
||||
});
|
||||
} else {
|
||||
graph.nodes[CANVAS_COHERENCE_INPAINT_CREATE_MASK] = {
|
||||
...(graph.nodes[
|
||||
CANVAS_COHERENCE_INPAINT_CREATE_MASK
|
||||
] as CreateDenoiseMaskInvocation),
|
||||
image: canvasInitImage,
|
||||
};
|
||||
}
|
||||
|
||||
// Create Mask If Coherence Mode Is Mask
|
||||
if (canvasCoherenceMode === 'mask') {
|
||||
if (isUsingScaledDimensions) {
|
||||
graph.edges.push({
|
||||
source: {
|
||||
node_id: MASK_RESIZE_UP,
|
||||
field: 'image',
|
||||
},
|
||||
destination: {
|
||||
node_id: CANVAS_COHERENCE_INPAINT_CREATE_MASK,
|
||||
field: 'mask',
|
||||
},
|
||||
});
|
||||
} else {
|
||||
graph.nodes[CANVAS_COHERENCE_INPAINT_CREATE_MASK] = {
|
||||
...(graph.nodes[
|
||||
CANVAS_COHERENCE_INPAINT_CREATE_MASK
|
||||
] as CreateDenoiseMaskInvocation),
|
||||
mask: canvasMaskImage,
|
||||
};
|
||||
}
|
||||
}
|
||||
|
||||
// Create Mask Edge If Coherence Mode Is Edge
|
||||
if (canvasCoherenceMode === 'edge') {
|
||||
graph.nodes[CANVAS_COHERENCE_MASK_EDGE] = {
|
||||
type: 'mask_edge',
|
||||
id: CANVAS_COHERENCE_MASK_EDGE,
|
||||
is_intermediate: true,
|
||||
edge_blur: maskBlur,
|
||||
edge_size: maskBlur * 2,
|
||||
low_threshold: 100,
|
||||
high_threshold: 200,
|
||||
};
|
||||
|
||||
// Handle Scaled Dimensions For Mask Edge
|
||||
if (isUsingScaledDimensions) {
|
||||
graph.edges.push({
|
||||
source: {
|
||||
node_id: MASK_RESIZE_UP,
|
||||
field: 'image',
|
||||
},
|
||||
destination: {
|
||||
node_id: CANVAS_COHERENCE_MASK_EDGE,
|
||||
field: 'image',
|
||||
},
|
||||
});
|
||||
} else {
|
||||
graph.nodes[CANVAS_COHERENCE_MASK_EDGE] = {
|
||||
...(graph.nodes[CANVAS_COHERENCE_MASK_EDGE] as MaskEdgeInvocation),
|
||||
image: canvasMaskImage,
|
||||
};
|
||||
}
|
||||
|
||||
graph.edges.push({
|
||||
source: {
|
||||
node_id: CANVAS_COHERENCE_MASK_EDGE,
|
||||
field: 'image',
|
||||
},
|
||||
destination: {
|
||||
node_id: CANVAS_COHERENCE_INPAINT_CREATE_MASK,
|
||||
field: 'mask',
|
||||
},
|
||||
});
|
||||
}
|
||||
|
||||
// Plug Denoise Mask To Coherence Denoise Latents
|
||||
graph.edges.push({
|
||||
source: {
|
||||
node_id: CANVAS_COHERENCE_INPAINT_CREATE_MASK,
|
||||
field: 'denoise_mask',
|
||||
},
|
||||
destination: {
|
||||
node_id: CANVAS_COHERENCE_DENOISE_LATENTS,
|
||||
field: 'denoise_mask',
|
||||
},
|
||||
});
|
||||
}
|
||||
|
||||
// Handle Seed
|
||||
if (shouldRandomizeSeed) {
|
||||
// Random int node to generate the starting seed
|
||||
|
@ -2,7 +2,6 @@ import { logger } from 'app/logging/logger';
|
||||
import { RootState } from 'app/store/store';
|
||||
import { NonNullableGraph } from 'features/nodes/types/types';
|
||||
import {
|
||||
ImageBlurInvocation,
|
||||
ImageDTO,
|
||||
ImageToLatentsInvocation,
|
||||
InfillPatchMatchInvocation,
|
||||
@ -20,6 +19,8 @@ import { addVAEToGraph } from './addVAEToGraph';
|
||||
import { addWatermarkerToGraph } from './addWatermarkerToGraph';
|
||||
import {
|
||||
CANVAS_COHERENCE_DENOISE_LATENTS,
|
||||
CANVAS_COHERENCE_INPAINT_CREATE_MASK,
|
||||
CANVAS_COHERENCE_MASK_EDGE,
|
||||
CANVAS_COHERENCE_NOISE,
|
||||
CANVAS_COHERENCE_NOISE_INCREMENT,
|
||||
CANVAS_OUTPUT,
|
||||
@ -31,7 +32,6 @@ import {
|
||||
INPAINT_INFILL_RESIZE_DOWN,
|
||||
ITERATE,
|
||||
LATENTS_TO_IMAGE,
|
||||
MASK_BLUR,
|
||||
MASK_COMBINE,
|
||||
MASK_FROM_ALPHA,
|
||||
MASK_RESIZE_DOWN,
|
||||
@ -72,10 +72,11 @@ export const buildCanvasSDXLOutpaintGraph = (
|
||||
shouldUseNoiseSettings,
|
||||
shouldUseCpuNoise,
|
||||
maskBlur,
|
||||
maskBlurMethod,
|
||||
canvasCoherenceMode,
|
||||
canvasCoherenceSteps,
|
||||
canvasCoherenceStrength,
|
||||
tileSize,
|
||||
infillTileSize,
|
||||
infillPatchmatchDownscaleSize,
|
||||
infillMethod,
|
||||
seamlessXAxis,
|
||||
seamlessYAxis,
|
||||
@ -103,6 +104,12 @@ export const buildCanvasSDXLOutpaintGraph = (
|
||||
shouldAutoSave,
|
||||
} = state.canvas;
|
||||
|
||||
const fp32 = vaePrecision === 'fp32';
|
||||
|
||||
const isUsingScaledDimensions = ['auto', 'manual'].includes(
|
||||
boundingBoxScaleMethod
|
||||
);
|
||||
|
||||
let modelLoaderNodeId = SDXL_MODEL_LOADER;
|
||||
|
||||
const use_cpu = shouldUseNoiseSettings
|
||||
@ -145,18 +152,11 @@ export const buildCanvasSDXLOutpaintGraph = (
|
||||
is_intermediate: true,
|
||||
mask2: canvasMaskImage,
|
||||
},
|
||||
[MASK_BLUR]: {
|
||||
type: 'img_blur',
|
||||
id: MASK_BLUR,
|
||||
is_intermediate: true,
|
||||
radius: maskBlur,
|
||||
blur_type: maskBlurMethod,
|
||||
},
|
||||
[INPAINT_IMAGE]: {
|
||||
type: 'i2l',
|
||||
id: INPAINT_IMAGE,
|
||||
is_intermediate: true,
|
||||
fp32: vaePrecision === 'fp32' ? true : false,
|
||||
fp32,
|
||||
},
|
||||
[NOISE]: {
|
||||
type: 'noise',
|
||||
@ -168,7 +168,7 @@ export const buildCanvasSDXLOutpaintGraph = (
|
||||
type: 'create_denoise_mask',
|
||||
id: INPAINT_CREATE_MASK,
|
||||
is_intermediate: true,
|
||||
fp32: vaePrecision === 'fp32' ? true : false,
|
||||
fp32,
|
||||
},
|
||||
[SDXL_DENOISE_LATENTS]: {
|
||||
type: 'denoise_latents',
|
||||
@ -208,7 +208,7 @@ export const buildCanvasSDXLOutpaintGraph = (
|
||||
type: 'l2i',
|
||||
id: LATENTS_TO_IMAGE,
|
||||
is_intermediate: true,
|
||||
fp32: vaePrecision === 'fp32' ? true : false,
|
||||
fp32,
|
||||
},
|
||||
[CANVAS_OUTPUT]: {
|
||||
type: 'color_correct',
|
||||
@ -348,7 +348,7 @@ export const buildCanvasSDXLOutpaintGraph = (
|
||||
// Create Inpaint Mask
|
||||
{
|
||||
source: {
|
||||
node_id: MASK_BLUR,
|
||||
node_id: isUsingScaledDimensions ? MASK_RESIZE_UP : MASK_COMBINE,
|
||||
field: 'image',
|
||||
},
|
||||
destination: {
|
||||
@ -410,7 +410,7 @@ export const buildCanvasSDXLOutpaintGraph = (
|
||||
},
|
||||
{
|
||||
source: {
|
||||
node_id: SDXL_MODEL_LOADER,
|
||||
node_id: modelLoaderNodeId,
|
||||
field: 'unet',
|
||||
},
|
||||
destination: {
|
||||
@ -458,6 +458,16 @@ export const buildCanvasSDXLOutpaintGraph = (
|
||||
field: 'latents',
|
||||
},
|
||||
},
|
||||
{
|
||||
source: {
|
||||
node_id: INPAINT_INFILL,
|
||||
field: 'image',
|
||||
},
|
||||
destination: {
|
||||
node_id: INPAINT_CREATE_MASK,
|
||||
field: 'image',
|
||||
},
|
||||
},
|
||||
// Decode inpainted latents to image
|
||||
{
|
||||
source: {
|
||||
@ -473,12 +483,12 @@ export const buildCanvasSDXLOutpaintGraph = (
|
||||
};
|
||||
|
||||
// Add Infill Nodes
|
||||
|
||||
if (infillMethod === 'patchmatch') {
|
||||
graph.nodes[INPAINT_INFILL] = {
|
||||
type: 'infill_patchmatch',
|
||||
id: INPAINT_INFILL,
|
||||
is_intermediate: true,
|
||||
downscale: infillPatchmatchDownscaleSize,
|
||||
};
|
||||
}
|
||||
|
||||
@ -490,17 +500,25 @@ export const buildCanvasSDXLOutpaintGraph = (
|
||||
};
|
||||
}
|
||||
|
||||
if (infillMethod === 'cv2') {
|
||||
graph.nodes[INPAINT_INFILL] = {
|
||||
type: 'infill_cv2',
|
||||
id: INPAINT_INFILL,
|
||||
is_intermediate: true,
|
||||
};
|
||||
}
|
||||
|
||||
if (infillMethod === 'tile') {
|
||||
graph.nodes[INPAINT_INFILL] = {
|
||||
type: 'infill_tile',
|
||||
id: INPAINT_INFILL,
|
||||
is_intermediate: true,
|
||||
tile_size: tileSize,
|
||||
tile_size: infillTileSize,
|
||||
};
|
||||
}
|
||||
|
||||
// Handle Scale Before Processing
|
||||
if (['auto', 'manual'].includes(boundingBoxScaleMethod)) {
|
||||
if (isUsingScaledDimensions) {
|
||||
const scaledWidth: number = scaledBoundingBoxDimensions.width;
|
||||
const scaledHeight: number = scaledBoundingBoxDimensions.height;
|
||||
|
||||
@ -562,16 +580,7 @@ export const buildCanvasSDXLOutpaintGraph = (
|
||||
field: 'image',
|
||||
},
|
||||
},
|
||||
{
|
||||
source: {
|
||||
node_id: INPAINT_INFILL,
|
||||
field: 'image',
|
||||
},
|
||||
destination: {
|
||||
node_id: INPAINT_CREATE_MASK,
|
||||
field: 'image',
|
||||
},
|
||||
},
|
||||
|
||||
// Take combined mask and resize and then blur
|
||||
{
|
||||
source: {
|
||||
@ -583,16 +592,7 @@ export const buildCanvasSDXLOutpaintGraph = (
|
||||
field: 'image',
|
||||
},
|
||||
},
|
||||
{
|
||||
source: {
|
||||
node_id: MASK_RESIZE_UP,
|
||||
field: 'image',
|
||||
},
|
||||
destination: {
|
||||
node_id: MASK_BLUR,
|
||||
field: 'image',
|
||||
},
|
||||
},
|
||||
|
||||
// Resize Results Down
|
||||
{
|
||||
source: {
|
||||
@ -674,32 +674,8 @@ export const buildCanvasSDXLOutpaintGraph = (
|
||||
...(graph.nodes[INPAINT_IMAGE] as ImageToLatentsInvocation),
|
||||
image: canvasInitImage,
|
||||
};
|
||||
graph.nodes[MASK_BLUR] = {
|
||||
...(graph.nodes[MASK_BLUR] as ImageBlurInvocation),
|
||||
};
|
||||
|
||||
graph.edges.push(
|
||||
// Take combined mask and plug it to blur
|
||||
{
|
||||
source: {
|
||||
node_id: MASK_COMBINE,
|
||||
field: 'image',
|
||||
},
|
||||
destination: {
|
||||
node_id: MASK_BLUR,
|
||||
field: 'image',
|
||||
},
|
||||
},
|
||||
{
|
||||
source: {
|
||||
node_id: INPAINT_INFILL,
|
||||
field: 'image',
|
||||
},
|
||||
destination: {
|
||||
node_id: INPAINT_CREATE_MASK,
|
||||
field: 'image',
|
||||
},
|
||||
},
|
||||
// Color Correct The Inpainted Result
|
||||
{
|
||||
source: {
|
||||
@ -723,7 +699,7 @@ export const buildCanvasSDXLOutpaintGraph = (
|
||||
},
|
||||
{
|
||||
source: {
|
||||
node_id: MASK_BLUR,
|
||||
node_id: MASK_COMBINE,
|
||||
field: 'image',
|
||||
},
|
||||
destination: {
|
||||
@ -734,7 +710,116 @@ export const buildCanvasSDXLOutpaintGraph = (
|
||||
);
|
||||
}
|
||||
|
||||
// Handle seed
|
||||
// Handle Coherence Mode
|
||||
if (canvasCoherenceMode !== 'unmasked') {
|
||||
graph.nodes[CANVAS_COHERENCE_INPAINT_CREATE_MASK] = {
|
||||
type: 'create_denoise_mask',
|
||||
id: CANVAS_COHERENCE_INPAINT_CREATE_MASK,
|
||||
is_intermediate: true,
|
||||
fp32,
|
||||
};
|
||||
|
||||
// Handle Image Input For Mask Creation
|
||||
graph.edges.push({
|
||||
source: {
|
||||
node_id: INPAINT_INFILL,
|
||||
field: 'image',
|
||||
},
|
||||
destination: {
|
||||
node_id: CANVAS_COHERENCE_INPAINT_CREATE_MASK,
|
||||
field: 'image',
|
||||
},
|
||||
});
|
||||
|
||||
// Create Mask If Coherence Mode Is Mask
|
||||
if (canvasCoherenceMode === 'mask') {
|
||||
if (isUsingScaledDimensions) {
|
||||
graph.edges.push({
|
||||
source: {
|
||||
node_id: MASK_RESIZE_UP,
|
||||
field: 'image',
|
||||
},
|
||||
destination: {
|
||||
node_id: CANVAS_COHERENCE_INPAINT_CREATE_MASK,
|
||||
field: 'mask',
|
||||
},
|
||||
});
|
||||
} else {
|
||||
graph.edges.push({
|
||||
source: {
|
||||
node_id: MASK_COMBINE,
|
||||
field: 'image',
|
||||
},
|
||||
destination: {
|
||||
node_id: CANVAS_COHERENCE_INPAINT_CREATE_MASK,
|
||||
field: 'mask',
|
||||
},
|
||||
});
|
||||
}
|
||||
}
|
||||
|
||||
if (canvasCoherenceMode === 'edge') {
|
||||
graph.nodes[CANVAS_COHERENCE_MASK_EDGE] = {
|
||||
type: 'mask_edge',
|
||||
id: CANVAS_COHERENCE_MASK_EDGE,
|
||||
is_intermediate: true,
|
||||
edge_blur: maskBlur,
|
||||
edge_size: maskBlur * 2,
|
||||
low_threshold: 100,
|
||||
high_threshold: 200,
|
||||
};
|
||||
|
||||
// Handle Scaled Dimensions For Mask Edge
|
||||
if (isUsingScaledDimensions) {
|
||||
graph.edges.push({
|
||||
source: {
|
||||
node_id: MASK_RESIZE_UP,
|
||||
field: 'image',
|
||||
},
|
||||
destination: {
|
||||
node_id: CANVAS_COHERENCE_MASK_EDGE,
|
||||
field: 'image',
|
||||
},
|
||||
});
|
||||
} else {
|
||||
graph.edges.push({
|
||||
source: {
|
||||
node_id: MASK_COMBINE,
|
||||
field: 'image',
|
||||
},
|
||||
destination: {
|
||||
node_id: CANVAS_COHERENCE_MASK_EDGE,
|
||||
field: 'image',
|
||||
},
|
||||
});
|
||||
}
|
||||
|
||||
graph.edges.push({
|
||||
source: {
|
||||
node_id: CANVAS_COHERENCE_MASK_EDGE,
|
||||
field: 'image',
|
||||
},
|
||||
destination: {
|
||||
node_id: CANVAS_COHERENCE_INPAINT_CREATE_MASK,
|
||||
field: 'mask',
|
||||
},
|
||||
});
|
||||
}
|
||||
|
||||
// Plug Denoise Mask To Coherence Denoise Latents
|
||||
graph.edges.push({
|
||||
source: {
|
||||
node_id: CANVAS_COHERENCE_INPAINT_CREATE_MASK,
|
||||
field: 'denoise_mask',
|
||||
},
|
||||
destination: {
|
||||
node_id: CANVAS_COHERENCE_DENOISE_LATENTS,
|
||||
field: 'denoise_mask',
|
||||
},
|
||||
});
|
||||
}
|
||||
|
||||
// Handle Seed
|
||||
if (shouldRandomizeSeed) {
|
||||
// Random int node to generate the starting seed
|
||||
const randomIntNode: RandomIntInvocation = {
|
||||
|
@ -61,6 +61,8 @@ export const buildCanvasSDXLTextToImageGraph = (
|
||||
shouldAutoSave,
|
||||
} = state.canvas;
|
||||
|
||||
const fp32 = vaePrecision === 'fp32';
|
||||
|
||||
const isUsingScaledDimensions = ['auto', 'manual'].includes(
|
||||
boundingBoxScaleMethod
|
||||
);
|
||||
@ -252,7 +254,7 @@ export const buildCanvasSDXLTextToImageGraph = (
|
||||
id: LATENTS_TO_IMAGE,
|
||||
type: isUsingOnnxModel ? 'l2i_onnx' : 'l2i',
|
||||
is_intermediate: true,
|
||||
fp32: vaePrecision === 'fp32' ? true : false,
|
||||
fp32,
|
||||
};
|
||||
|
||||
graph.nodes[CANVAS_OUTPUT] = {
|
||||
@ -290,7 +292,7 @@ export const buildCanvasSDXLTextToImageGraph = (
|
||||
type: isUsingOnnxModel ? 'l2i_onnx' : 'l2i',
|
||||
id: CANVAS_OUTPUT,
|
||||
is_intermediate: !shouldAutoSave,
|
||||
fp32: vaePrecision === 'fp32' ? true : false,
|
||||
fp32,
|
||||
};
|
||||
|
||||
graph.edges.push({
|
||||
|
@ -59,6 +59,8 @@ export const buildCanvasTextToImageGraph = (
|
||||
shouldAutoSave,
|
||||
} = state.canvas;
|
||||
|
||||
const fp32 = vaePrecision === 'fp32';
|
||||
|
||||
const isUsingScaledDimensions = ['auto', 'manual'].includes(
|
||||
boundingBoxScaleMethod
|
||||
);
|
||||
@ -238,7 +240,7 @@ export const buildCanvasTextToImageGraph = (
|
||||
id: LATENTS_TO_IMAGE,
|
||||
type: isUsingOnnxModel ? 'l2i_onnx' : 'l2i',
|
||||
is_intermediate: true,
|
||||
fp32: vaePrecision === 'fp32' ? true : false,
|
||||
fp32,
|
||||
};
|
||||
|
||||
graph.nodes[CANVAS_OUTPUT] = {
|
||||
@ -276,7 +278,7 @@ export const buildCanvasTextToImageGraph = (
|
||||
type: isUsingOnnxModel ? 'l2i_onnx' : 'l2i',
|
||||
id: CANVAS_OUTPUT,
|
||||
is_intermediate: !shouldAutoSave,
|
||||
fp32: vaePrecision === 'fp32' ? true : false,
|
||||
fp32,
|
||||
};
|
||||
|
||||
graph.edges.push({
|
||||
|
@ -84,6 +84,8 @@ export const buildLinearImageToImageGraph = (
|
||||
throw new Error('No model found in state');
|
||||
}
|
||||
|
||||
const fp32 = vaePrecision === 'fp32';
|
||||
|
||||
let modelLoaderNodeId = MAIN_MODEL_LOADER;
|
||||
|
||||
const use_cpu = shouldUseNoiseSettings
|
||||
@ -122,7 +124,7 @@ export const buildLinearImageToImageGraph = (
|
||||
[LATENTS_TO_IMAGE]: {
|
||||
type: 'l2i',
|
||||
id: LATENTS_TO_IMAGE,
|
||||
fp32: vaePrecision === 'fp32' ? true : false,
|
||||
fp32,
|
||||
},
|
||||
[DENOISE_LATENTS]: {
|
||||
type: 'denoise_latents',
|
||||
@ -140,7 +142,7 @@ export const buildLinearImageToImageGraph = (
|
||||
// image: {
|
||||
// image_name: initialImage.image_name,
|
||||
// },
|
||||
fp32: vaePrecision === 'fp32' ? true : false,
|
||||
fp32,
|
||||
},
|
||||
},
|
||||
edges: [
|
||||
|
@ -84,6 +84,8 @@ export const buildLinearSDXLImageToImageGraph = (
|
||||
throw new Error('No model found in state');
|
||||
}
|
||||
|
||||
const fp32 = vaePrecision === 'fp32';
|
||||
|
||||
// Model Loader ID
|
||||
let modelLoaderNodeId = SDXL_MODEL_LOADER;
|
||||
|
||||
@ -124,7 +126,7 @@ export const buildLinearSDXLImageToImageGraph = (
|
||||
[LATENTS_TO_IMAGE]: {
|
||||
type: 'l2i',
|
||||
id: LATENTS_TO_IMAGE,
|
||||
fp32: vaePrecision === 'fp32' ? true : false,
|
||||
fp32,
|
||||
},
|
||||
[SDXL_DENOISE_LATENTS]: {
|
||||
type: 'denoise_latents',
|
||||
@ -144,7 +146,7 @@ export const buildLinearSDXLImageToImageGraph = (
|
||||
// image: {
|
||||
// image_name: initialImage.image_name,
|
||||
// },
|
||||
fp32: vaePrecision === 'fp32' ? true : false,
|
||||
fp32,
|
||||
},
|
||||
},
|
||||
edges: [
|
||||
|
@ -62,6 +62,8 @@ export const buildLinearSDXLTextToImageGraph = (
|
||||
throw new Error('No model found in state');
|
||||
}
|
||||
|
||||
const fp32 = vaePrecision === 'fp32';
|
||||
|
||||
// Construct Style Prompt
|
||||
const { craftedPositiveStylePrompt, craftedNegativeStylePrompt } =
|
||||
craftSDXLStylePrompt(state, shouldConcatSDXLStylePrompt);
|
||||
@ -118,7 +120,7 @@ export const buildLinearSDXLTextToImageGraph = (
|
||||
[LATENTS_TO_IMAGE]: {
|
||||
type: 'l2i',
|
||||
id: LATENTS_TO_IMAGE,
|
||||
fp32: vaePrecision === 'fp32' ? true : false,
|
||||
fp32,
|
||||
},
|
||||
},
|
||||
edges: [
|
||||
|
@ -57,6 +57,8 @@ export const buildLinearTextToImageGraph = (
|
||||
throw new Error('No model found in state');
|
||||
}
|
||||
|
||||
const fp32 = vaePrecision === 'fp32';
|
||||
|
||||
const isUsingOnnxModel = model.model_type === 'onnx';
|
||||
|
||||
let modelLoaderNodeId = isUsingOnnxModel
|
||||
@ -139,7 +141,7 @@ export const buildLinearTextToImageGraph = (
|
||||
[LATENTS_TO_IMAGE]: {
|
||||
type: isUsingOnnxModel ? 'l2i_onnx' : 'l2i',
|
||||
id: LATENTS_TO_IMAGE,
|
||||
fp32: vaePrecision === 'fp32' ? true : false,
|
||||
fp32,
|
||||
},
|
||||
},
|
||||
edges: [
|
||||
|
@ -27,11 +27,15 @@ export const INPAINT_INFILL = 'inpaint_infill';
|
||||
export const INPAINT_INFILL_RESIZE_DOWN = 'inpaint_infill_resize_down';
|
||||
export const INPAINT_FINAL_IMAGE = 'inpaint_final_image';
|
||||
export const INPAINT_CREATE_MASK = 'inpaint_create_mask';
|
||||
export const INPAINT_MASK = 'inpaint_mask';
|
||||
export const CANVAS_COHERENCE_DENOISE_LATENTS =
|
||||
'canvas_coherence_denoise_latents';
|
||||
export const CANVAS_COHERENCE_NOISE = 'canvas_coherence_noise';
|
||||
export const CANVAS_COHERENCE_NOISE_INCREMENT =
|
||||
'canvas_coherence_noise_increment';
|
||||
export const CANVAS_COHERENCE_MASK_EDGE = 'canvas_coherence_mask_edge';
|
||||
export const CANVAS_COHERENCE_INPAINT_CREATE_MASK =
|
||||
'canvas_coherence_inpaint_create_mask';
|
||||
export const MASK_FROM_ALPHA = 'tomask';
|
||||
export const MASK_EDGE = 'mask_edge';
|
||||
export const MASK_BLUR = 'mask_blur';
|
||||
|
@ -73,6 +73,7 @@ export const parseSchema = (
|
||||
const title = schema.title.replace('Invocation', '');
|
||||
const tags = schema.tags ?? [];
|
||||
const description = schema.description ?? '';
|
||||
const version = schema.version ?? '';
|
||||
|
||||
const inputs = reduce(
|
||||
schema.properties,
|
||||
@ -225,11 +226,12 @@ export const parseSchema = (
|
||||
const invocation: InvocationTemplate = {
|
||||
title,
|
||||
type,
|
||||
version,
|
||||
tags,
|
||||
description,
|
||||
outputType,
|
||||
inputs,
|
||||
outputs,
|
||||
outputType,
|
||||
};
|
||||
|
||||
Object.assign(invocationsAccumulator, { [type]: invocation });
|
||||
|
@ -0,0 +1,96 @@
|
||||
import { compareVersions } from 'compare-versions';
|
||||
import { cloneDeep, keyBy } from 'lodash-es';
|
||||
import {
|
||||
InvocationTemplate,
|
||||
Workflow,
|
||||
WorkflowWarning,
|
||||
isWorkflowInvocationNode,
|
||||
} from '../types/types';
|
||||
import { parseify } from 'common/util/serialize';
|
||||
|
||||
export const validateWorkflow = (
|
||||
workflow: Workflow,
|
||||
nodeTemplates: Record<string, InvocationTemplate>
|
||||
) => {
|
||||
const clone = cloneDeep(workflow);
|
||||
const { nodes, edges } = clone;
|
||||
const errors: WorkflowWarning[] = [];
|
||||
const invocationNodes = nodes.filter(isWorkflowInvocationNode);
|
||||
const keyedNodes = keyBy(invocationNodes, 'id');
|
||||
nodes.forEach((node) => {
|
||||
if (!isWorkflowInvocationNode(node)) {
|
||||
return;
|
||||
}
|
||||
|
||||
const nodeTemplate = nodeTemplates[node.data.type];
|
||||
if (!nodeTemplate) {
|
||||
errors.push({
|
||||
message: `Node "${node.data.type}" skipped`,
|
||||
issues: [`Node type "${node.data.type}" does not exist`],
|
||||
data: node,
|
||||
});
|
||||
return;
|
||||
}
|
||||
|
||||
if (
|
||||
nodeTemplate.version &&
|
||||
node.data.version &&
|
||||
compareVersions(nodeTemplate.version, node.data.version) !== 0
|
||||
) {
|
||||
errors.push({
|
||||
message: `Node "${node.data.type}" has mismatched version`,
|
||||
issues: [
|
||||
`Node "${node.data.type}" v${node.data.version} may be incompatible with installed v${nodeTemplate.version}`,
|
||||
],
|
||||
data: { node, nodeTemplate: parseify(nodeTemplate) },
|
||||
});
|
||||
return;
|
||||
}
|
||||
});
|
||||
edges.forEach((edge, i) => {
|
||||
const sourceNode = keyedNodes[edge.source];
|
||||
const targetNode = keyedNodes[edge.target];
|
||||
const issues: string[] = [];
|
||||
if (!sourceNode) {
|
||||
issues.push(`Output node ${edge.source} does not exist`);
|
||||
} else if (
|
||||
edge.type === 'default' &&
|
||||
!(edge.sourceHandle in sourceNode.data.outputs)
|
||||
) {
|
||||
issues.push(
|
||||
`Output field "${edge.source}.${edge.sourceHandle}" does not exist`
|
||||
);
|
||||
}
|
||||
if (!targetNode) {
|
||||
issues.push(`Input node ${edge.target} does not exist`);
|
||||
} else if (
|
||||
edge.type === 'default' &&
|
||||
!(edge.targetHandle in targetNode.data.inputs)
|
||||
) {
|
||||
issues.push(
|
||||
`Input field "${edge.target}.${edge.targetHandle}" does not exist`
|
||||
);
|
||||
}
|
||||
if (!nodeTemplates[sourceNode?.data.type ?? '__UNKNOWN_NODE_TYPE__']) {
|
||||
issues.push(
|
||||
`Source node "${edge.source}" missing template "${sourceNode?.data.type}"`
|
||||
);
|
||||
}
|
||||
if (!nodeTemplates[targetNode?.data.type ?? '__UNKNOWN_NODE_TYPE__']) {
|
||||
issues.push(
|
||||
`Source node "${edge.target}" missing template "${targetNode?.data.type}"`
|
||||
);
|
||||
}
|
||||
if (issues.length) {
|
||||
delete edges[i];
|
||||
const src = edge.type === 'default' ? edge.sourceHandle : edge.source;
|
||||
const tgt = edge.type === 'default' ? edge.targetHandle : edge.target;
|
||||
errors.push({
|
||||
message: `Edge "${src} -> ${tgt}" skipped`,
|
||||
issues,
|
||||
data: edge,
|
||||
});
|
||||
}
|
||||
});
|
||||
return { workflow: clone, errors };
|
||||
};
|
@ -0,0 +1,42 @@
|
||||
import type { RootState } from 'app/store/store';
|
||||
import { useAppDispatch, useAppSelector } from 'app/store/storeHooks';
|
||||
import { IAISelectDataType } from 'common/components/IAIMantineSearchableSelect';
|
||||
import IAIMantineSelect from 'common/components/IAIMantineSelect';
|
||||
import { setCanvasCoherenceMode } from 'features/parameters/store/generationSlice';
|
||||
import { CanvasCoherenceModeParam } from 'features/parameters/types/parameterSchemas';
|
||||
|
||||
import { memo } from 'react';
|
||||
import { useTranslation } from 'react-i18next';
|
||||
|
||||
const coherenceModeSelectData: IAISelectDataType[] = [
|
||||
{ label: 'Unmasked', value: 'unmasked' },
|
||||
{ label: 'Mask', value: 'mask' },
|
||||
{ label: 'Mask Edge', value: 'edge' },
|
||||
];
|
||||
|
||||
const ParamCanvasCoherenceMode = () => {
|
||||
const dispatch = useAppDispatch();
|
||||
const canvasCoherenceMode = useAppSelector(
|
||||
(state: RootState) => state.generation.canvasCoherenceMode
|
||||
);
|
||||
const { t } = useTranslation();
|
||||
|
||||
const handleCoherenceModeChange = (v: string | null) => {
|
||||
if (!v) {
|
||||
return;
|
||||
}
|
||||
|
||||
dispatch(setCanvasCoherenceMode(v as CanvasCoherenceModeParam));
|
||||
};
|
||||
|
||||
return (
|
||||
<IAIMantineSelect
|
||||
label={t('parameters.coherenceMode')}
|
||||
data={coherenceModeSelectData}
|
||||
value={canvasCoherenceMode}
|
||||
onChange={handleCoherenceModeChange}
|
||||
/>
|
||||
);
|
||||
};
|
||||
|
||||
export default memo(ParamCanvasCoherenceMode);
|
@ -3,6 +3,7 @@ import IAICollapse from 'common/components/IAICollapse';
|
||||
import { memo } from 'react';
|
||||
import { useTranslation } from 'react-i18next';
|
||||
import SubParametersWrapper from '../../SubParametersWrapper';
|
||||
import ParamCanvasCoherenceMode from './CoherencePass/ParamCanvasCoherenceMode';
|
||||
import ParamCanvasCoherenceSteps from './CoherencePass/ParamCanvasCoherenceSteps';
|
||||
import ParamCanvasCoherenceStrength from './CoherencePass/ParamCanvasCoherenceStrength';
|
||||
import ParamMaskBlur from './MaskAdjustment/ParamMaskBlur';
|
||||
@ -14,15 +15,16 @@ const ParamCompositingSettingsCollapse = () => {
|
||||
return (
|
||||
<IAICollapse label={t('parameters.compositingSettingsHeader')}>
|
||||
<Flex sx={{ flexDirection: 'column', gap: 2 }}>
|
||||
<SubParametersWrapper label={t('parameters.coherencePassHeader')}>
|
||||
<ParamCanvasCoherenceMode />
|
||||
<ParamCanvasCoherenceSteps />
|
||||
<ParamCanvasCoherenceStrength />
|
||||
</SubParametersWrapper>
|
||||
<Divider />
|
||||
<SubParametersWrapper label={t('parameters.maskAdjustmentsHeader')}>
|
||||
<ParamMaskBlur />
|
||||
<ParamMaskBlurMethod />
|
||||
</SubParametersWrapper>
|
||||
<Divider />
|
||||
<SubParametersWrapper label={t('parameters.coherencePassHeader')}>
|
||||
<ParamCanvasCoherenceSteps />
|
||||
<ParamCanvasCoherenceStrength />
|
||||
</SubParametersWrapper>
|
||||
</Flex>
|
||||
</IAICollapse>
|
||||
);
|
||||
|
@ -5,7 +5,7 @@ import { useTranslation } from 'react-i18next';
|
||||
import IAICollapse from 'common/components/IAICollapse';
|
||||
import SubParametersWrapper from '../../SubParametersWrapper';
|
||||
import ParamInfillMethod from './ParamInfillMethod';
|
||||
import ParamInfillTilesize from './ParamInfillTilesize';
|
||||
import ParamInfillOptions from './ParamInfillOptions';
|
||||
import ParamScaleBeforeProcessing from './ParamScaleBeforeProcessing';
|
||||
import ParamScaledHeight from './ParamScaledHeight';
|
||||
import ParamScaledWidth from './ParamScaledWidth';
|
||||
@ -18,7 +18,7 @@ const ParamInfillCollapse = () => {
|
||||
<Flex sx={{ gap: 2, flexDirection: 'column' }}>
|
||||
<SubParametersWrapper>
|
||||
<ParamInfillMethod />
|
||||
<ParamInfillTilesize />
|
||||
<ParamInfillOptions />
|
||||
</SubParametersWrapper>
|
||||
<Divider />
|
||||
<SubParametersWrapper>
|
||||
|
@ -27,9 +27,7 @@ const ParamInfillMethod = () => {
|
||||
|
||||
const { data: appConfigData, isLoading } = useGetAppConfigQuery();
|
||||
|
||||
const infill_methods = appConfigData?.infill_methods.filter(
|
||||
(method) => method !== 'lama'
|
||||
);
|
||||
const infill_methods = appConfigData?.infill_methods;
|
||||
|
||||
const { t } = useTranslation();
|
||||
|
||||
|
@ -0,0 +1,29 @@
|
||||
import { Flex } from '@chakra-ui/react';
|
||||
import { createSelector } from '@reduxjs/toolkit';
|
||||
import { useAppSelector } from 'app/store/storeHooks';
|
||||
import { defaultSelectorOptions } from 'app/store/util/defaultMemoizeOptions';
|
||||
import { generationSelector } from 'features/parameters/store/generationSelectors';
|
||||
import ParamInfillPatchmatchDownscaleSize from './ParamInfillPatchmatchDownscaleSize';
|
||||
import ParamInfillTilesize from './ParamInfillTilesize';
|
||||
|
||||
const selector = createSelector(
|
||||
[generationSelector],
|
||||
(parameters) => {
|
||||
const { infillMethod } = parameters;
|
||||
|
||||
return {
|
||||
infillMethod,
|
||||
};
|
||||
},
|
||||
defaultSelectorOptions
|
||||
);
|
||||
|
||||
export default function ParamInfillOptions() {
|
||||
const { infillMethod } = useAppSelector(selector);
|
||||
return (
|
||||
<Flex>
|
||||
{infillMethod === 'tile' && <ParamInfillTilesize />}
|
||||
{infillMethod === 'patchmatch' && <ParamInfillPatchmatchDownscaleSize />}
|
||||
</Flex>
|
||||
);
|
||||
}
|
@ -0,0 +1,58 @@
|
||||
import { createSelector } from '@reduxjs/toolkit';
|
||||
import { useAppDispatch, useAppSelector } from 'app/store/storeHooks';
|
||||
import { defaultSelectorOptions } from 'app/store/util/defaultMemoizeOptions';
|
||||
import IAISlider from 'common/components/IAISlider';
|
||||
import { generationSelector } from 'features/parameters/store/generationSelectors';
|
||||
import { setInfillPatchmatchDownscaleSize } from 'features/parameters/store/generationSlice';
|
||||
import { memo, useCallback } from 'react';
|
||||
|
||||
import { useTranslation } from 'react-i18next';
|
||||
|
||||
const selector = createSelector(
|
||||
[generationSelector],
|
||||
(parameters) => {
|
||||
const { infillPatchmatchDownscaleSize, infillMethod } = parameters;
|
||||
|
||||
return {
|
||||
infillPatchmatchDownscaleSize,
|
||||
infillMethod,
|
||||
};
|
||||
},
|
||||
defaultSelectorOptions
|
||||
);
|
||||
|
||||
const ParamInfillPatchmatchDownscaleSize = () => {
|
||||
const dispatch = useAppDispatch();
|
||||
const { infillPatchmatchDownscaleSize, infillMethod } =
|
||||
useAppSelector(selector);
|
||||
|
||||
const { t } = useTranslation();
|
||||
|
||||
const handleChange = useCallback(
|
||||
(v: number) => {
|
||||
dispatch(setInfillPatchmatchDownscaleSize(v));
|
||||
},
|
||||
[dispatch]
|
||||
);
|
||||
|
||||
const handleReset = useCallback(() => {
|
||||
dispatch(setInfillPatchmatchDownscaleSize(2));
|
||||
}, [dispatch]);
|
||||
|
||||
return (
|
||||
<IAISlider
|
||||
isDisabled={infillMethod !== 'patchmatch'}
|
||||
label={t('parameters.patchmatchDownScaleSize')}
|
||||
min={1}
|
||||
max={10}
|
||||
value={infillPatchmatchDownscaleSize}
|
||||
onChange={handleChange}
|
||||
withInput
|
||||
withSliderMarks
|
||||
withReset
|
||||
handleReset={handleReset}
|
||||
/>
|
||||
);
|
||||
};
|
||||
|
||||
export default memo(ParamInfillPatchmatchDownscaleSize);
|
@ -3,7 +3,7 @@ import { useAppDispatch, useAppSelector } from 'app/store/storeHooks';
|
||||
import { defaultSelectorOptions } from 'app/store/util/defaultMemoizeOptions';
|
||||
import IAISlider from 'common/components/IAISlider';
|
||||
import { generationSelector } from 'features/parameters/store/generationSelectors';
|
||||
import { setTileSize } from 'features/parameters/store/generationSlice';
|
||||
import { setInfillTileSize } from 'features/parameters/store/generationSlice';
|
||||
import { memo, useCallback } from 'react';
|
||||
|
||||
import { useTranslation } from 'react-i18next';
|
||||
@ -11,10 +11,10 @@ import { useTranslation } from 'react-i18next';
|
||||
const selector = createSelector(
|
||||
[generationSelector],
|
||||
(parameters) => {
|
||||
const { tileSize, infillMethod } = parameters;
|
||||
const { infillTileSize, infillMethod } = parameters;
|
||||
|
||||
return {
|
||||
tileSize,
|
||||
infillTileSize,
|
||||
infillMethod,
|
||||
};
|
||||
},
|
||||
@ -23,19 +23,19 @@ const selector = createSelector(
|
||||
|
||||
const ParamInfillTileSize = () => {
|
||||
const dispatch = useAppDispatch();
|
||||
const { tileSize, infillMethod } = useAppSelector(selector);
|
||||
const { infillTileSize, infillMethod } = useAppSelector(selector);
|
||||
|
||||
const { t } = useTranslation();
|
||||
|
||||
const handleChange = useCallback(
|
||||
(v: number) => {
|
||||
dispatch(setTileSize(v));
|
||||
dispatch(setInfillTileSize(v));
|
||||
},
|
||||
[dispatch]
|
||||
);
|
||||
|
||||
const handleReset = useCallback(() => {
|
||||
dispatch(setTileSize(32));
|
||||
dispatch(setInfillTileSize(32));
|
||||
}, [dispatch]);
|
||||
|
||||
return (
|
||||
@ -45,7 +45,7 @@ const ParamInfillTileSize = () => {
|
||||
min={16}
|
||||
max={64}
|
||||
sliderNumberInputProps={{ max: 256 }}
|
||||
value={tileSize}
|
||||
value={infillTileSize}
|
||||
onChange={handleChange}
|
||||
withInput
|
||||
withSliderMarks
|
||||
|
@ -7,6 +7,7 @@ import { ImageDTO } from 'services/api/types';
|
||||
|
||||
import { clipSkipMap } from '../types/constants';
|
||||
import {
|
||||
CanvasCoherenceModeParam,
|
||||
CfgScaleParam,
|
||||
HeightParam,
|
||||
MainModelParam,
|
||||
@ -37,6 +38,7 @@ export interface GenerationState {
|
||||
scheduler: SchedulerParam;
|
||||
maskBlur: number;
|
||||
maskBlurMethod: MaskBlurMethodParam;
|
||||
canvasCoherenceMode: CanvasCoherenceModeParam;
|
||||
canvasCoherenceSteps: number;
|
||||
canvasCoherenceStrength: StrengthParam;
|
||||
seed: SeedParam;
|
||||
@ -47,7 +49,8 @@ export interface GenerationState {
|
||||
shouldUseNoiseSettings: boolean;
|
||||
steps: StepsParam;
|
||||
threshold: number;
|
||||
tileSize: number;
|
||||
infillTileSize: number;
|
||||
infillPatchmatchDownscaleSize: number;
|
||||
variationAmount: number;
|
||||
width: WidthParam;
|
||||
shouldUseSymmetry: boolean;
|
||||
@ -77,6 +80,7 @@ export const initialGenerationState: GenerationState = {
|
||||
scheduler: 'euler',
|
||||
maskBlur: 16,
|
||||
maskBlurMethod: 'box',
|
||||
canvasCoherenceMode: 'edge',
|
||||
canvasCoherenceSteps: 20,
|
||||
canvasCoherenceStrength: 0.3,
|
||||
seed: 0,
|
||||
@ -87,7 +91,8 @@ export const initialGenerationState: GenerationState = {
|
||||
shouldUseNoiseSettings: false,
|
||||
steps: 50,
|
||||
threshold: 0,
|
||||
tileSize: 32,
|
||||
infillTileSize: 32,
|
||||
infillPatchmatchDownscaleSize: 1,
|
||||
variationAmount: 0.1,
|
||||
width: 512,
|
||||
shouldUseSymmetry: false,
|
||||
@ -206,18 +211,30 @@ export const generationSlice = createSlice({
|
||||
setMaskBlurMethod: (state, action: PayloadAction<MaskBlurMethodParam>) => {
|
||||
state.maskBlurMethod = action.payload;
|
||||
},
|
||||
setCanvasCoherenceMode: (
|
||||
state,
|
||||
action: PayloadAction<CanvasCoherenceModeParam>
|
||||
) => {
|
||||
state.canvasCoherenceMode = action.payload;
|
||||
},
|
||||
setCanvasCoherenceSteps: (state, action: PayloadAction<number>) => {
|
||||
state.canvasCoherenceSteps = action.payload;
|
||||
},
|
||||
setCanvasCoherenceStrength: (state, action: PayloadAction<number>) => {
|
||||
state.canvasCoherenceStrength = action.payload;
|
||||
},
|
||||
setTileSize: (state, action: PayloadAction<number>) => {
|
||||
state.tileSize = action.payload;
|
||||
},
|
||||
setInfillMethod: (state, action: PayloadAction<string>) => {
|
||||
state.infillMethod = action.payload;
|
||||
},
|
||||
setInfillTileSize: (state, action: PayloadAction<number>) => {
|
||||
state.infillTileSize = action.payload;
|
||||
},
|
||||
setInfillPatchmatchDownscaleSize: (
|
||||
state,
|
||||
action: PayloadAction<number>
|
||||
) => {
|
||||
state.infillPatchmatchDownscaleSize = action.payload;
|
||||
},
|
||||
setShouldUseSymmetry: (state, action: PayloadAction<boolean>) => {
|
||||
state.shouldUseSymmetry = action.payload;
|
||||
},
|
||||
@ -323,6 +340,7 @@ export const {
|
||||
setScheduler,
|
||||
setMaskBlur,
|
||||
setMaskBlurMethod,
|
||||
setCanvasCoherenceMode,
|
||||
setCanvasCoherenceSteps,
|
||||
setCanvasCoherenceStrength,
|
||||
setSeed,
|
||||
@ -332,7 +350,8 @@ export const {
|
||||
setShouldRandomizeSeed,
|
||||
setSteps,
|
||||
setThreshold,
|
||||
setTileSize,
|
||||
setInfillTileSize,
|
||||
setInfillPatchmatchDownscaleSize,
|
||||
setVariationAmount,
|
||||
setShouldUseSymmetry,
|
||||
setHorizontalSymmetrySteps,
|
||||
|
@ -418,6 +418,22 @@ export const isValidMaskBlurMethod = (
|
||||
val: unknown
|
||||
): val is MaskBlurMethodParam => zMaskBlurMethod.safeParse(val).success;
|
||||
|
||||
/**
|
||||
* Zod schema for a Canvas Coherence Mode method parameter
|
||||
*/
|
||||
export const zCanvasCoherenceMode = z.enum(['unmasked', 'mask', 'edge']);
|
||||
/**
|
||||
* Type alias for Canvas Coherence Mode parameter, inferred from its zod schema
|
||||
*/
|
||||
export type CanvasCoherenceModeParam = z.infer<typeof zCanvasCoherenceMode>;
|
||||
/**
|
||||
* Validates/type-guards a value as a mask blur method parameter
|
||||
*/
|
||||
export const isValidCoherenceModeParam = (
|
||||
val: unknown
|
||||
): val is CanvasCoherenceModeParam =>
|
||||
zCanvasCoherenceMode.safeParse(val).success;
|
||||
|
||||
// /**
|
||||
// * Zod schema for BaseModelType
|
||||
// */
|
||||
|
@ -1,11 +1,11 @@
|
||||
import { Flex } from '@chakra-ui/react';
|
||||
import { useForm } from '@mantine/form';
|
||||
import { makeToast } from 'features/system/util/makeToast';
|
||||
import { useAppDispatch } from 'app/store/storeHooks';
|
||||
import IAIButton from 'common/components/IAIButton';
|
||||
import IAIMantineTextInput from 'common/components/IAIMantineInput';
|
||||
import IAISimpleCheckbox from 'common/components/IAISimpleCheckbox';
|
||||
import { addToast } from 'features/system/store/systemSlice';
|
||||
import { makeToast } from 'features/system/util/makeToast';
|
||||
import { useState } from 'react';
|
||||
import { useTranslation } from 'react-i18next';
|
||||
import { useAddMainModelsMutation } from 'services/api/endpoints/models';
|
||||
@ -14,6 +14,7 @@ import { setAdvancedAddScanModel } from '../../store/modelManagerSlice';
|
||||
import BaseModelSelect from '../shared/BaseModelSelect';
|
||||
import CheckpointConfigsSelect from '../shared/CheckpointConfigsSelect';
|
||||
import ModelVariantSelect from '../shared/ModelVariantSelect';
|
||||
import { getModelName } from './util';
|
||||
|
||||
type AdvancedAddCheckpointProps = {
|
||||
model_path?: string;
|
||||
@ -28,7 +29,7 @@ export default function AdvancedAddCheckpoint(
|
||||
|
||||
const advancedAddCheckpointForm = useForm<CheckpointModelConfig>({
|
||||
initialValues: {
|
||||
model_name: model_path?.split('\\').splice(-1)[0]?.split('.')[0] ?? '',
|
||||
model_name: model_path ? getModelName(model_path) : '',
|
||||
base_model: 'sd-1',
|
||||
model_type: 'main',
|
||||
path: model_path ? model_path : '',
|
||||
@ -100,6 +101,17 @@ export default function AdvancedAddCheckpoint(
|
||||
label="Model Location"
|
||||
required
|
||||
{...advancedAddCheckpointForm.getInputProps('path')}
|
||||
onBlur={(e) => {
|
||||
if (advancedAddCheckpointForm.values['model_name'] === '') {
|
||||
const modelName = getModelName(e.currentTarget.value);
|
||||
if (modelName) {
|
||||
advancedAddCheckpointForm.setFieldValue(
|
||||
'model_name',
|
||||
modelName as string
|
||||
);
|
||||
}
|
||||
}
|
||||
}}
|
||||
/>
|
||||
<IAIMantineTextInput
|
||||
label="Description"
|
||||
|
@ -1,16 +1,17 @@
|
||||
import { Flex } from '@chakra-ui/react';
|
||||
import { useForm } from '@mantine/form';
|
||||
import { makeToast } from 'features/system/util/makeToast';
|
||||
import { useAppDispatch } from 'app/store/storeHooks';
|
||||
import IAIButton from 'common/components/IAIButton';
|
||||
import IAIMantineTextInput from 'common/components/IAIMantineInput';
|
||||
import { addToast } from 'features/system/store/systemSlice';
|
||||
import { makeToast } from 'features/system/util/makeToast';
|
||||
import { useTranslation } from 'react-i18next';
|
||||
import { useAddMainModelsMutation } from 'services/api/endpoints/models';
|
||||
import { DiffusersModelConfig } from 'services/api/types';
|
||||
import { setAdvancedAddScanModel } from '../../store/modelManagerSlice';
|
||||
import BaseModelSelect from '../shared/BaseModelSelect';
|
||||
import ModelVariantSelect from '../shared/ModelVariantSelect';
|
||||
import { getModelName } from './util';
|
||||
|
||||
type AdvancedAddDiffusersProps = {
|
||||
model_path?: string;
|
||||
@ -25,7 +26,7 @@ export default function AdvancedAddDiffusers(props: AdvancedAddDiffusersProps) {
|
||||
|
||||
const advancedAddDiffusersForm = useForm<DiffusersModelConfig>({
|
||||
initialValues: {
|
||||
model_name: model_path?.split('\\').splice(-1)[0] ?? '',
|
||||
model_name: model_path ? getModelName(model_path, false) : '',
|
||||
base_model: 'sd-1',
|
||||
model_type: 'main',
|
||||
path: model_path ? model_path : '',
|
||||
@ -92,6 +93,17 @@ export default function AdvancedAddDiffusers(props: AdvancedAddDiffusersProps) {
|
||||
label="Model Location"
|
||||
placeholder="Provide the path to a local folder where your Diffusers Model is stored"
|
||||
{...advancedAddDiffusersForm.getInputProps('path')}
|
||||
onBlur={(e) => {
|
||||
if (advancedAddDiffusersForm.values['model_name'] === '') {
|
||||
const modelName = getModelName(e.currentTarget.value, false);
|
||||
if (modelName) {
|
||||
advancedAddDiffusersForm.setFieldValue(
|
||||
'model_name',
|
||||
modelName as string
|
||||
);
|
||||
}
|
||||
}
|
||||
}}
|
||||
/>
|
||||
<IAIMantineTextInput
|
||||
label="Description"
|
||||
|
@ -0,0 +1,15 @@
|
||||
export function getModelName(filepath: string, isCheckpoint: boolean = true) {
|
||||
let regex;
|
||||
if (isCheckpoint) {
|
||||
regex = new RegExp('[^\\\\/]+(?=\\.)');
|
||||
} else {
|
||||
regex = new RegExp('[^\\\\/]+(?=[\\\\/]?$)');
|
||||
}
|
||||
|
||||
const match = filepath.match(regex);
|
||||
if (match) {
|
||||
return match[0];
|
||||
} else {
|
||||
return '';
|
||||
}
|
||||
}
|
266
invokeai/frontend/web/src/services/api/schema.d.ts
vendored
266
invokeai/frontend/web/src/services/api/schema.d.ts
vendored
File diff suppressed because one or more lines are too long
@ -112,6 +112,7 @@ export type ImageScaleInvocation = s['ImageScaleInvocation'];
|
||||
export type InfillPatchMatchInvocation = s['InfillPatchMatchInvocation'];
|
||||
export type InfillTileInvocation = s['InfillTileInvocation'];
|
||||
export type CreateDenoiseMaskInvocation = s['CreateDenoiseMaskInvocation'];
|
||||
export type MaskEdgeInvocation = s['MaskEdgeInvocation'];
|
||||
export type RandomIntInvocation = s['RandomIntInvocation'];
|
||||
export type CompelInvocation = s['CompelInvocation'];
|
||||
export type DynamicPromptInvocation = s['DynamicPromptInvocation'];
|
||||
|
@ -2970,6 +2970,11 @@ commondir@^1.0.1:
|
||||
resolved "https://registry.yarnpkg.com/commondir/-/commondir-1.0.1.tgz#ddd800da0c66127393cca5950ea968a3aaf1253b"
|
||||
integrity sha512-W9pAhw0ja1Edb5GVdIF1mjZw/ASI0AlShXM83UUGe2DVr5TdAPEA1OA8m/g8zWp9x6On7gqufY+FatDbC3MDQg==
|
||||
|
||||
compare-versions@^6.1.0:
|
||||
version "6.1.0"
|
||||
resolved "https://registry.yarnpkg.com/compare-versions/-/compare-versions-6.1.0.tgz#3f2131e3ae93577df111dba133e6db876ffe127a"
|
||||
integrity sha512-LNZQXhqUvqUTotpZ00qLSaify3b4VFD588aRr8MKFw4CMUr98ytzCW5wDH5qx/DEY5kCDXcbcRuCqL0szEf2tg==
|
||||
|
||||
compute-scroll-into-view@1.0.20:
|
||||
version "1.0.20"
|
||||
resolved "https://registry.yarnpkg.com/compute-scroll-into-view/-/compute-scroll-into-view-1.0.20.tgz#1768b5522d1172754f5d0c9b02de3af6be506a43"
|
||||
|
@ -1,283 +0,0 @@
|
||||
{
|
||||
"cells": [
|
||||
{
|
||||
"cell_type": "markdown",
|
||||
"metadata": {
|
||||
"id": "ycYWcsEKc6w7"
|
||||
},
|
||||
"source": [
|
||||
"# Stable Diffusion AI Notebook (Release 2.0.0)\n",
|
||||
"\n",
|
||||
"<img src=\"https://user-images.githubusercontent.com/60411196/186547976-d9de378a-9de8-4201-9c25-c057a9c59bad.jpeg\" alt=\"stable-diffusion-ai\" width=\"170px\"/> <br>\n",
|
||||
"#### Instructions:\n",
|
||||
"1. Execute each cell in order to mount a Dream bot and create images from text. <br>\n",
|
||||
"2. Once cells 1-8 were run correctly you'll be executing a terminal in cell #9, you'll need to enter `python scripts/dream.py` command to run Dream bot.<br> \n",
|
||||
"3. After launching dream bot, you'll see: <br> `Dream > ` in terminal. <br> Insert a command, eg. `Dream > Astronaut floating in a distant galaxy`, or type `-h` for help.\n",
|
||||
"3. After completion you'll see your generated images in path `stable-diffusion/outputs/img-samples/`, you can also show last generated images in cell #10.\n",
|
||||
"4. To quit Dream bot use `q` command. <br> \n",
|
||||
"---\n",
|
||||
"<font color=\"red\">Note:</font> It takes some time to load, but after installing all dependencies you can use the bot all time you want while colab instance is up. <br>\n",
|
||||
"<font color=\"red\">Requirements:</font> For this notebook to work you need to have [Stable-Diffusion-v-1-4](https://huggingface.co/CompVis/stable-diffusion-v-1-4-original) stored in your Google Drive, it will be needed in cell #7\n",
|
||||
"##### For more details visit Github repository: [invoke-ai/InvokeAI](https://github.com/invoke-ai/InvokeAI)\n",
|
||||
"---\n"
|
||||
]
|
||||
},
|
||||
{
|
||||
"cell_type": "markdown",
|
||||
"metadata": {
|
||||
"id": "dr32VLxlnouf"
|
||||
},
|
||||
"source": [
|
||||
"## ◢ Installation"
|
||||
]
|
||||
},
|
||||
{
|
||||
"cell_type": "code",
|
||||
"execution_count": null,
|
||||
"metadata": {
|
||||
"cellView": "form",
|
||||
"id": "a2Z5Qu_o8VtQ"
|
||||
},
|
||||
"outputs": [],
|
||||
"source": [
|
||||
"# @title 1. Check current GPU assigned\n",
|
||||
"!nvidia-smi -L\n",
|
||||
"!nvidia-smi"
|
||||
]
|
||||
},
|
||||
{
|
||||
"cell_type": "code",
|
||||
"execution_count": null,
|
||||
"metadata": {
|
||||
"cellView": "form",
|
||||
"id": "vbI9ZsQHzjqF"
|
||||
},
|
||||
"outputs": [],
|
||||
"source": [
|
||||
"# @title 2. Download stable-diffusion Repository\n",
|
||||
"from os.path import exists\n",
|
||||
"\n",
|
||||
"!git clone --quiet https://github.com/invoke-ai/InvokeAI.git # Original repo\n",
|
||||
"%cd /content/InvokeAI/\n",
|
||||
"!git checkout --quiet tags/v2.0.0"
|
||||
]
|
||||
},
|
||||
{
|
||||
"cell_type": "code",
|
||||
"execution_count": null,
|
||||
"metadata": {
|
||||
"cellView": "form",
|
||||
"id": "QbXcGXYEFSNB"
|
||||
},
|
||||
"outputs": [],
|
||||
"source": [
|
||||
"# @title 3. Install dependencies\n",
|
||||
"import gc\n",
|
||||
"\n",
|
||||
"!wget https://raw.githubusercontent.com/invoke-ai/InvokeAI/development/environments-and-requirements/requirements-base.txt\n",
|
||||
"!wget https://raw.githubusercontent.com/invoke-ai/InvokeAI/development/environments-and-requirements/requirements-win-colab-cuda.txt\n",
|
||||
"!pip install colab-xterm\n",
|
||||
"!pip install -r requirements-lin-win-colab-CUDA.txt\n",
|
||||
"!pip install clean-fid torchtext\n",
|
||||
"!pip install transformers\n",
|
||||
"gc.collect()"
|
||||
]
|
||||
},
|
||||
{
|
||||
"cell_type": "code",
|
||||
"execution_count": null,
|
||||
"metadata": {
|
||||
"cellView": "form",
|
||||
"id": "8rSMhgnAttQa"
|
||||
},
|
||||
"outputs": [],
|
||||
"source": [
|
||||
"# @title 4. Restart Runtime\n",
|
||||
"exit()"
|
||||
]
|
||||
},
|
||||
{
|
||||
"cell_type": "code",
|
||||
"execution_count": null,
|
||||
"metadata": {
|
||||
"cellView": "form",
|
||||
"id": "ChIDWxLVHGGJ"
|
||||
},
|
||||
"outputs": [],
|
||||
"source": [
|
||||
"# @title 5. Load small ML models required\n",
|
||||
"import gc\n",
|
||||
"\n",
|
||||
"%cd /content/InvokeAI/\n",
|
||||
"!python scripts/preload_models.py\n",
|
||||
"gc.collect()"
|
||||
]
|
||||
},
|
||||
{
|
||||
"cell_type": "markdown",
|
||||
"metadata": {
|
||||
"id": "795x1tMoo8b1"
|
||||
},
|
||||
"source": [
|
||||
"## ◢ Configuration"
|
||||
]
|
||||
},
|
||||
{
|
||||
"cell_type": "code",
|
||||
"execution_count": null,
|
||||
"metadata": {
|
||||
"cellView": "form",
|
||||
"id": "YEWPV-sF1RDM"
|
||||
},
|
||||
"outputs": [],
|
||||
"source": [
|
||||
"# @title 6. Mount google Drive\n",
|
||||
"from google.colab import drive\n",
|
||||
"\n",
|
||||
"drive.mount(\"/content/drive\")"
|
||||
]
|
||||
},
|
||||
{
|
||||
"cell_type": "code",
|
||||
"execution_count": null,
|
||||
"metadata": {
|
||||
"cellView": "form",
|
||||
"id": "zRTJeZ461WGu"
|
||||
},
|
||||
"outputs": [],
|
||||
"source": [
|
||||
"# @title 7. Drive Path to model\n",
|
||||
"# @markdown Path should start with /content/drive/path-to-your-file <br>\n",
|
||||
"# @markdown <font color=\"red\">Note:</font> Model should be downloaded from https://huggingface.co <br>\n",
|
||||
"# @markdown Lastest release: [Stable-Diffusion-v-1-4](https://huggingface.co/CompVis/stable-diffusion-v-1-4-original)\n",
|
||||
"from os.path import exists\n",
|
||||
"\n",
|
||||
"model_path = \"\" # @param {type:\"string\"}\n",
|
||||
"if exists(model_path):\n",
|
||||
" print(\"✅ Valid directory\")\n",
|
||||
"else:\n",
|
||||
" print(\"❌ File doesn't exist\")"
|
||||
]
|
||||
},
|
||||
{
|
||||
"cell_type": "code",
|
||||
"execution_count": null,
|
||||
"metadata": {
|
||||
"cellView": "form",
|
||||
"id": "UY-NNz4I8_aG"
|
||||
},
|
||||
"outputs": [],
|
||||
"source": [
|
||||
"# @title 8. Symlink to model\n",
|
||||
"\n",
|
||||
"from os.path import exists\n",
|
||||
"import os\n",
|
||||
"\n",
|
||||
"# Folder creation if it doesn't exist\n",
|
||||
"if exists(\"/content/InvokeAI/models/ldm/stable-diffusion-v1\"):\n",
|
||||
" print(\"❗ Dir stable-diffusion-v1 already exists\")\n",
|
||||
"else:\n",
|
||||
" %mkdir /content/InvokeAI/models/ldm/stable-diffusion-v1\n",
|
||||
" print(\"✅ Dir stable-diffusion-v1 created\")\n",
|
||||
"\n",
|
||||
"# Symbolic link if it doesn't exist\n",
|
||||
"if exists(\"/content/InvokeAI/models/ldm/stable-diffusion-v1/model.ckpt\"):\n",
|
||||
" print(\"❗ Symlink already created\")\n",
|
||||
"else:\n",
|
||||
" src = model_path\n",
|
||||
" dst = \"/content/InvokeAI/models/ldm/stable-diffusion-v1/model.ckpt\"\n",
|
||||
" os.symlink(src, dst)\n",
|
||||
" print(\"✅ Symbolic link created successfully\")"
|
||||
]
|
||||
},
|
||||
{
|
||||
"cell_type": "markdown",
|
||||
"metadata": {
|
||||
"id": "Mc28N0_NrCQH"
|
||||
},
|
||||
"source": [
|
||||
"## ◢ Execution"
|
||||
]
|
||||
},
|
||||
{
|
||||
"cell_type": "code",
|
||||
"execution_count": null,
|
||||
"metadata": {
|
||||
"cellView": "form",
|
||||
"id": "ir4hCrMIuUpl"
|
||||
},
|
||||
"outputs": [],
|
||||
"source": [
|
||||
"# @title 9. Run Terminal and Execute Dream bot\n",
|
||||
"# @markdown <font color=\"blue\">Steps:</font> <br>\n",
|
||||
"# @markdown 1. Execute command `python scripts/invoke.py` to run InvokeAI.<br>\n",
|
||||
"# @markdown 2. After initialized you'll see `Dream>` line.<br>\n",
|
||||
"# @markdown 3. Example text: `Astronaut floating in a distant galaxy` <br>\n",
|
||||
"# @markdown 4. To quit Dream bot use: `q` command.<br>\n",
|
||||
"\n",
|
||||
"%load_ext colabxterm\n",
|
||||
"%xterm\n",
|
||||
"gc.collect()"
|
||||
]
|
||||
},
|
||||
{
|
||||
"cell_type": "code",
|
||||
"execution_count": null,
|
||||
"metadata": {
|
||||
"cellView": "form",
|
||||
"id": "qnLohSHmKoGk"
|
||||
},
|
||||
"outputs": [],
|
||||
"source": [
|
||||
"#@title 10. Show the last 15 generated images\n",
|
||||
"import glob\n",
|
||||
"import matplotlib.pyplot as plt\n",
|
||||
"import matplotlib.image as mpimg\n",
|
||||
"%matplotlib inline\n",
|
||||
"\n",
|
||||
"images = []\n",
|
||||
"for img_path in sorted(glob.glob('/content/InvokeAI/outputs/img-samples/*.png'), reverse=True):\n",
|
||||
" images.append(mpimg.imread(img_path))\n",
|
||||
"\n",
|
||||
"images = images[:15] \n",
|
||||
"\n",
|
||||
"plt.figure(figsize=(20,10))\n",
|
||||
"\n",
|
||||
"columns = 5\n",
|
||||
"for i, image in enumerate(images):\n",
|
||||
" ax = plt.subplot(len(images) / columns + 1, columns, i + 1)\n",
|
||||
" ax.axes.xaxis.set_visible(False)\n",
|
||||
" ax.axes.yaxis.set_visible(False)\n",
|
||||
" ax.axis('off')\n",
|
||||
" plt.imshow(image)\n",
|
||||
" gc.collect()\n",
|
||||
"\n"
|
||||
]
|
||||
}
|
||||
],
|
||||
"metadata": {
|
||||
"accelerator": "GPU",
|
||||
"colab": {
|
||||
"collapsed_sections": [],
|
||||
"private_outputs": true,
|
||||
"provenance": []
|
||||
},
|
||||
"gpuClass": "standard",
|
||||
"kernelspec": {
|
||||
"display_name": "Python 3.9.12 64-bit",
|
||||
"language": "python",
|
||||
"name": "python3"
|
||||
},
|
||||
"language_info": {
|
||||
"name": "python",
|
||||
"version": "3.9.12"
|
||||
},
|
||||
"vscode": {
|
||||
"interpreter": {
|
||||
"hash": "4e870c5c5fe42db7e2c5647ae5af656ff3391bf8c2b729cbf7fa0e16ca8cb5af"
|
||||
}
|
||||
}
|
||||
},
|
||||
"nbformat": 4,
|
||||
"nbformat_minor": 0
|
||||
}
|
@ -1,339 +0,0 @@
|
||||
from torchvision.datasets.utils import download_url
|
||||
from ldm.util import instantiate_from_config
|
||||
import torch
|
||||
import os
|
||||
|
||||
# todo ?
|
||||
from google.colab import files
|
||||
from IPython.display import Image as ipyimg
|
||||
import ipywidgets as widgets
|
||||
from PIL import Image
|
||||
from einops import rearrange, repeat
|
||||
import torchvision
|
||||
from ldm.models.diffusion.ddim import DDIMSampler
|
||||
from ldm.util import ismap
|
||||
import time
|
||||
from omegaconf import OmegaConf
|
||||
from ldm.invoke.devices import choose_torch_device
|
||||
|
||||
|
||||
def download_models(mode):
|
||||
if mode == "superresolution":
|
||||
# this is the small bsr light model
|
||||
url_conf = "https://heibox.uni-heidelberg.de/f/31a76b13ea27482981b4/?dl=1"
|
||||
url_ckpt = "https://heibox.uni-heidelberg.de/f/578df07c8fc04ffbadf3/?dl=1"
|
||||
|
||||
path_conf = "logs/diffusion/superresolution_bsr/configs/project.yaml"
|
||||
path_ckpt = "logs/diffusion/superresolution_bsr/checkpoints/last.ckpt"
|
||||
|
||||
download_url(url_conf, path_conf)
|
||||
download_url(url_ckpt, path_ckpt)
|
||||
|
||||
path_conf = path_conf + "/?dl=1" # fix it
|
||||
path_ckpt = path_ckpt + "/?dl=1" # fix it
|
||||
return path_conf, path_ckpt
|
||||
|
||||
else:
|
||||
raise NotImplementedError
|
||||
|
||||
|
||||
def load_model_from_config(config, ckpt):
|
||||
print(f"Loading model from {ckpt}")
|
||||
pl_sd = torch.load(ckpt, map_location="cpu")
|
||||
global_step = pl_sd["global_step"]
|
||||
sd = pl_sd["state_dict"]
|
||||
model = instantiate_from_config(config.model)
|
||||
m, u = model.load_state_dict(sd, strict=False)
|
||||
model.cuda()
|
||||
model.eval()
|
||||
return {"model": model}, global_step
|
||||
|
||||
|
||||
def get_model(mode):
|
||||
path_conf, path_ckpt = download_models(mode)
|
||||
config = OmegaConf.load(path_conf)
|
||||
model, step = load_model_from_config(config, path_ckpt)
|
||||
return model
|
||||
|
||||
|
||||
def get_custom_cond(mode):
|
||||
dest = "data/example_conditioning"
|
||||
|
||||
if mode == "superresolution":
|
||||
uploaded_img = files.upload()
|
||||
filename = next(iter(uploaded_img))
|
||||
name, filetype = filename.split(".") # todo assumes just one dot in name !
|
||||
os.rename(f"{filename}", f"{dest}/{mode}/custom_{name}.{filetype}")
|
||||
|
||||
elif mode == "text_conditional":
|
||||
w = widgets.Text(value="A cake with cream!", disabled=True)
|
||||
display(w) # noqa: F821
|
||||
|
||||
with open(f"{dest}/{mode}/custom_{w.value[:20]}.txt", "w") as f:
|
||||
f.write(w.value)
|
||||
|
||||
elif mode == "class_conditional":
|
||||
w = widgets.IntSlider(min=0, max=1000)
|
||||
display(w) # noqa: F821
|
||||
with open(f"{dest}/{mode}/custom.txt", "w") as f:
|
||||
f.write(w.value)
|
||||
|
||||
else:
|
||||
raise NotImplementedError(f"cond not implemented for mode{mode}")
|
||||
|
||||
|
||||
def get_cond_options(mode):
|
||||
path = "data/example_conditioning"
|
||||
path = os.path.join(path, mode)
|
||||
onlyfiles = [f for f in sorted(os.listdir(path))]
|
||||
return path, onlyfiles
|
||||
|
||||
|
||||
def select_cond_path(mode):
|
||||
path = "data/example_conditioning" # todo
|
||||
path = os.path.join(path, mode)
|
||||
onlyfiles = [f for f in sorted(os.listdir(path))]
|
||||
|
||||
selected = widgets.RadioButtons(options=onlyfiles, description="Select conditioning:", disabled=False)
|
||||
display(selected) # noqa: F821
|
||||
selected_path = os.path.join(path, selected.value)
|
||||
return selected_path
|
||||
|
||||
|
||||
def get_cond(mode, selected_path):
|
||||
example = dict()
|
||||
if mode == "superresolution":
|
||||
up_f = 4
|
||||
visualize_cond_img(selected_path)
|
||||
|
||||
c = Image.open(selected_path)
|
||||
c = torch.unsqueeze(torchvision.transforms.ToTensor()(c), 0)
|
||||
c_up = torchvision.transforms.functional.resize(c, size=[up_f * c.shape[2], up_f * c.shape[3]], antialias=True)
|
||||
c_up = rearrange(c_up, "1 c h w -> 1 h w c")
|
||||
c = rearrange(c, "1 c h w -> 1 h w c")
|
||||
c = 2.0 * c - 1.0
|
||||
|
||||
device = choose_torch_device()
|
||||
c = c.to(device)
|
||||
example["LR_image"] = c
|
||||
example["image"] = c_up
|
||||
|
||||
return example
|
||||
|
||||
|
||||
def visualize_cond_img(path):
|
||||
display(ipyimg(filename=path)) # noqa: F821
|
||||
|
||||
|
||||
def run(model, selected_path, task, custom_steps, resize_enabled=False, classifier_ckpt=None, global_step=None):
|
||||
example = get_cond(task, selected_path)
|
||||
|
||||
save_intermediate_vid = False
|
||||
n_runs = 1
|
||||
masked = False
|
||||
guider = None
|
||||
ckwargs = None
|
||||
mode = "ddim"
|
||||
ddim_use_x0_pred = False
|
||||
temperature = 1.0
|
||||
eta = 1.0
|
||||
make_progrow = True
|
||||
custom_shape = None
|
||||
|
||||
height, width = example["image"].shape[1:3]
|
||||
split_input = height >= 128 and width >= 128
|
||||
|
||||
if split_input:
|
||||
ks = 128
|
||||
stride = 64
|
||||
vqf = 4 #
|
||||
model.split_input_params = {
|
||||
"ks": (ks, ks),
|
||||
"stride": (stride, stride),
|
||||
"vqf": vqf,
|
||||
"patch_distributed_vq": True,
|
||||
"tie_braker": False,
|
||||
"clip_max_weight": 0.5,
|
||||
"clip_min_weight": 0.01,
|
||||
"clip_max_tie_weight": 0.5,
|
||||
"clip_min_tie_weight": 0.01,
|
||||
}
|
||||
else:
|
||||
if hasattr(model, "split_input_params"):
|
||||
delattr(model, "split_input_params")
|
||||
|
||||
invert_mask = False
|
||||
|
||||
x_T = None
|
||||
for n in range(n_runs):
|
||||
if custom_shape is not None:
|
||||
x_T = torch.randn(1, custom_shape[1], custom_shape[2], custom_shape[3]).to(model.device)
|
||||
x_T = repeat(x_T, "1 c h w -> b c h w", b=custom_shape[0])
|
||||
|
||||
logs = make_convolutional_sample(
|
||||
example,
|
||||
model,
|
||||
mode=mode,
|
||||
custom_steps=custom_steps,
|
||||
eta=eta,
|
||||
swap_mode=False,
|
||||
masked=masked,
|
||||
invert_mask=invert_mask,
|
||||
quantize_x0=False,
|
||||
custom_schedule=None,
|
||||
decode_interval=10,
|
||||
resize_enabled=resize_enabled,
|
||||
custom_shape=custom_shape,
|
||||
temperature=temperature,
|
||||
noise_dropout=0.0,
|
||||
corrector=guider,
|
||||
corrector_kwargs=ckwargs,
|
||||
x_T=x_T,
|
||||
save_intermediate_vid=save_intermediate_vid,
|
||||
make_progrow=make_progrow,
|
||||
ddim_use_x0_pred=ddim_use_x0_pred,
|
||||
)
|
||||
return logs
|
||||
|
||||
|
||||
@torch.no_grad()
|
||||
def convsample_ddim(
|
||||
model,
|
||||
cond,
|
||||
steps,
|
||||
shape,
|
||||
eta=1.0,
|
||||
callback=None,
|
||||
normals_sequence=None,
|
||||
mask=None,
|
||||
x0=None,
|
||||
quantize_x0=False,
|
||||
img_callback=None,
|
||||
temperature=1.0,
|
||||
noise_dropout=0.0,
|
||||
score_corrector=None,
|
||||
corrector_kwargs=None,
|
||||
x_T=None,
|
||||
log_every_t=None,
|
||||
):
|
||||
ddim = DDIMSampler(model)
|
||||
bs = shape[0] # dont know where this comes from but wayne
|
||||
shape = shape[1:] # cut batch dim
|
||||
print(f"Sampling with eta = {eta}; steps: {steps}")
|
||||
samples, intermediates = ddim.sample(
|
||||
steps,
|
||||
batch_size=bs,
|
||||
shape=shape,
|
||||
conditioning=cond,
|
||||
callback=callback,
|
||||
normals_sequence=normals_sequence,
|
||||
quantize_x0=quantize_x0,
|
||||
eta=eta,
|
||||
mask=mask,
|
||||
x0=x0,
|
||||
temperature=temperature,
|
||||
verbose=False,
|
||||
score_corrector=score_corrector,
|
||||
corrector_kwargs=corrector_kwargs,
|
||||
x_T=x_T,
|
||||
)
|
||||
|
||||
return samples, intermediates
|
||||
|
||||
|
||||
@torch.no_grad()
|
||||
def make_convolutional_sample(
|
||||
batch,
|
||||
model,
|
||||
mode="vanilla",
|
||||
custom_steps=None,
|
||||
eta=1.0,
|
||||
swap_mode=False,
|
||||
masked=False,
|
||||
invert_mask=True,
|
||||
quantize_x0=False,
|
||||
custom_schedule=None,
|
||||
decode_interval=1000,
|
||||
resize_enabled=False,
|
||||
custom_shape=None,
|
||||
temperature=1.0,
|
||||
noise_dropout=0.0,
|
||||
corrector=None,
|
||||
corrector_kwargs=None,
|
||||
x_T=None,
|
||||
save_intermediate_vid=False,
|
||||
make_progrow=True,
|
||||
ddim_use_x0_pred=False,
|
||||
):
|
||||
log = dict()
|
||||
|
||||
z, c, x, xrec, xc = model.get_input(
|
||||
batch,
|
||||
model.first_stage_key,
|
||||
return_first_stage_outputs=True,
|
||||
force_c_encode=not (hasattr(model, "split_input_params") and model.cond_stage_key == "coordinates_bbox"),
|
||||
return_original_cond=True,
|
||||
)
|
||||
|
||||
log_every_t = 1 if save_intermediate_vid else None
|
||||
|
||||
if custom_shape is not None:
|
||||
z = torch.randn(custom_shape)
|
||||
print(f"Generating {custom_shape[0]} samples of shape {custom_shape[1:]}")
|
||||
|
||||
z0 = None
|
||||
|
||||
log["input"] = x
|
||||
log["reconstruction"] = xrec
|
||||
|
||||
if ismap(xc):
|
||||
log["original_conditioning"] = model.to_rgb(xc)
|
||||
if hasattr(model, "cond_stage_key"):
|
||||
log[model.cond_stage_key] = model.to_rgb(xc)
|
||||
|
||||
else:
|
||||
log["original_conditioning"] = xc if xc is not None else torch.zeros_like(x)
|
||||
if model.cond_stage_model:
|
||||
log[model.cond_stage_key] = xc if xc is not None else torch.zeros_like(x)
|
||||
if model.cond_stage_key == "class_label":
|
||||
log[model.cond_stage_key] = xc[model.cond_stage_key]
|
||||
|
||||
with model.ema_scope("Plotting"):
|
||||
t0 = time.time()
|
||||
img_cb = None
|
||||
|
||||
sample, intermediates = convsample_ddim(
|
||||
model,
|
||||
c,
|
||||
steps=custom_steps,
|
||||
shape=z.shape,
|
||||
eta=eta,
|
||||
quantize_x0=quantize_x0,
|
||||
img_callback=img_cb,
|
||||
mask=None,
|
||||
x0=z0,
|
||||
temperature=temperature,
|
||||
noise_dropout=noise_dropout,
|
||||
score_corrector=corrector,
|
||||
corrector_kwargs=corrector_kwargs,
|
||||
x_T=x_T,
|
||||
log_every_t=log_every_t,
|
||||
)
|
||||
t1 = time.time()
|
||||
|
||||
if ddim_use_x0_pred:
|
||||
sample = intermediates["pred_x0"][-1]
|
||||
|
||||
x_sample = model.decode_first_stage(sample)
|
||||
|
||||
try:
|
||||
x_sample_noquant = model.decode_first_stage(sample, force_not_quantize=True)
|
||||
log["sample_noquant"] = x_sample_noquant
|
||||
log["sample_diff"] = torch.abs(x_sample_noquant - x_sample)
|
||||
except Exception:
|
||||
pass
|
||||
|
||||
log["sample"] = x_sample
|
||||
log["time"] = t1 - t0
|
||||
|
||||
return log
|
@ -74,6 +74,7 @@ dependencies = [
|
||||
"rich~=13.3",
|
||||
"safetensors==0.3.1",
|
||||
"scikit-image~=0.21.0",
|
||||
"semver~=3.0.1",
|
||||
"send2trash",
|
||||
"test-tube~=0.7.5",
|
||||
"torch~=2.0.1",
|
||||
|
Some files were not shown because too many files have changed in this diff Show More
Loading…
x
Reference in New Issue
Block a user