mirror of
https://github.com/invoke-ai/InvokeAI
synced 2024-08-30 20:32:17 +00:00
Make the VAE tile size configurable for tiled VAE (#6555)
## Summary - This PR exposes a `tile_size` field on `ImageToLatentsInvocation` and `LatentsToImageInvocation`. - Setting `tile_size = 0` preserves the default behaviour. - This feature is primarily intended to support upscaling workflows that require VAE encoding/decoding high resolution images. In the future, we may want to expose the tile size as a global application config, but that's a separate conversation. - As a general rule, larger tile sizes produce better results at the cost of higher memory usage. ### Example: Original (5472x5472) 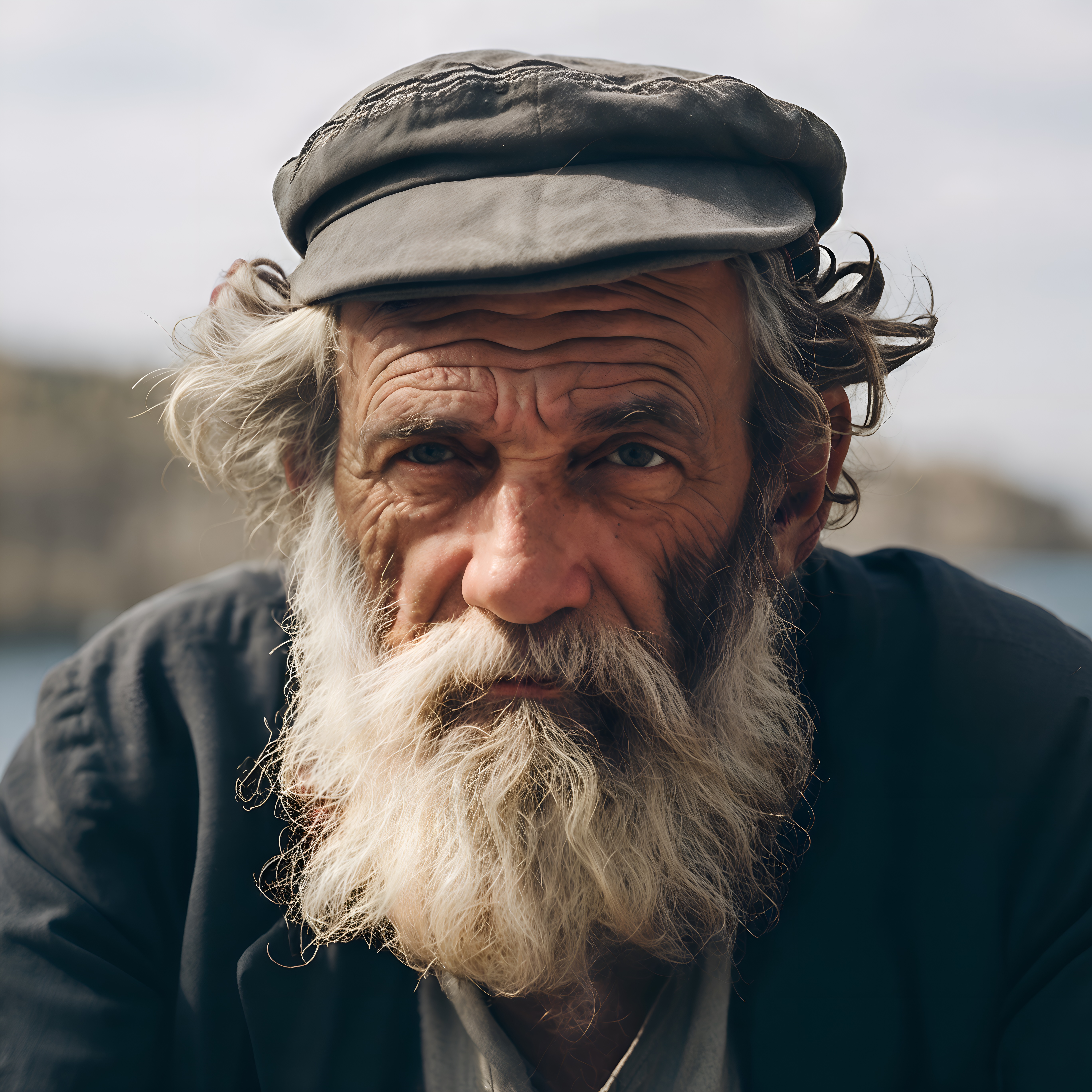 VAE roundtrip with 512x512 tiles (note the discoloration)  VAE roundtrip with 1024x1024 tiles (some discoloration still present, but less severe than at 512x512)  ## Related Issues / Discussions Related: #6144 ## QA Instructions - [x] Test image generation via the Linear tab - [x] Test VAE roundtrip with tiling disabled - [x] Test VAE roundtrip with tiling and tile_size = 0 - [x] Test VAE roundtrip with tiling and tile_size > 0 ## Merge Plan No special instructions. ## Checklist - [x] _The PR has a short but descriptive title, suitable for a changelog_ - [x] _Tests added / updated (if applicable)_ - [x] _Documentation added / updated (if applicable)_
This commit is contained in:
commit
e9936c27fb
@ -160,6 +160,8 @@ class FieldDescriptions:
|
||||
fp32 = "Whether or not to use full float32 precision"
|
||||
precision = "Precision to use"
|
||||
tiled = "Processing using overlapping tiles (reduce memory consumption)"
|
||||
vae_tile_size = "The tile size for VAE tiling in pixels (image space). If set to 0, the default tile size for the "
|
||||
"model will be used. Larger tile sizes generally produce better results at the cost of higher memory usage."
|
||||
detect_res = "Pixel resolution for detection"
|
||||
image_res = "Pixel resolution for output image"
|
||||
safe_mode = "Whether or not to use safe mode"
|
||||
|
@ -1,3 +1,4 @@
|
||||
from contextlib import nullcontext
|
||||
from functools import singledispatchmethod
|
||||
|
||||
import einops
|
||||
@ -12,7 +13,7 @@ from diffusers.models.autoencoders.autoencoder_kl import AutoencoderKL
|
||||
from diffusers.models.autoencoders.autoencoder_tiny import AutoencoderTiny
|
||||
|
||||
from invokeai.app.invocations.baseinvocation import BaseInvocation, invocation
|
||||
from invokeai.app.invocations.constants import DEFAULT_PRECISION
|
||||
from invokeai.app.invocations.constants import DEFAULT_PRECISION, LATENT_SCALE_FACTOR
|
||||
from invokeai.app.invocations.fields import (
|
||||
FieldDescriptions,
|
||||
ImageField,
|
||||
@ -24,6 +25,7 @@ from invokeai.app.invocations.primitives import LatentsOutput
|
||||
from invokeai.app.services.shared.invocation_context import InvocationContext
|
||||
from invokeai.backend.model_manager import LoadedModel
|
||||
from invokeai.backend.stable_diffusion.diffusers_pipeline import image_resized_to_grid_as_tensor
|
||||
from invokeai.backend.stable_diffusion.vae_tiling import patch_vae_tiling_params
|
||||
|
||||
|
||||
@invocation(
|
||||
@ -31,7 +33,7 @@ from invokeai.backend.stable_diffusion.diffusers_pipeline import image_resized_t
|
||||
title="Image to Latents",
|
||||
tags=["latents", "image", "vae", "i2l"],
|
||||
category="latents",
|
||||
version="1.0.2",
|
||||
version="1.1.0",
|
||||
)
|
||||
class ImageToLatentsInvocation(BaseInvocation):
|
||||
"""Encodes an image into latents."""
|
||||
@ -44,12 +46,17 @@ class ImageToLatentsInvocation(BaseInvocation):
|
||||
input=Input.Connection,
|
||||
)
|
||||
tiled: bool = InputField(default=False, description=FieldDescriptions.tiled)
|
||||
# NOTE: tile_size = 0 is a special value. We use this rather than `int | None`, because the workflow UI does not
|
||||
# offer a way to directly set None values.
|
||||
tile_size: int = InputField(default=0, multiple_of=8, description=FieldDescriptions.vae_tile_size)
|
||||
fp32: bool = InputField(default=DEFAULT_PRECISION == torch.float32, description=FieldDescriptions.fp32)
|
||||
|
||||
@staticmethod
|
||||
def vae_encode(vae_info: LoadedModel, upcast: bool, tiled: bool, image_tensor: torch.Tensor) -> torch.Tensor:
|
||||
def vae_encode(
|
||||
vae_info: LoadedModel, upcast: bool, tiled: bool, image_tensor: torch.Tensor, tile_size: int = 0
|
||||
) -> torch.Tensor:
|
||||
with vae_info as vae:
|
||||
assert isinstance(vae, torch.nn.Module)
|
||||
assert isinstance(vae, (AutoencoderKL, AutoencoderTiny))
|
||||
orig_dtype = vae.dtype
|
||||
if upcast:
|
||||
vae.to(dtype=torch.float32)
|
||||
@ -81,9 +88,18 @@ class ImageToLatentsInvocation(BaseInvocation):
|
||||
else:
|
||||
vae.disable_tiling()
|
||||
|
||||
tiling_context = nullcontext()
|
||||
if tile_size > 0:
|
||||
tiling_context = patch_vae_tiling_params(
|
||||
vae,
|
||||
tile_sample_min_size=tile_size,
|
||||
tile_latent_min_size=tile_size // LATENT_SCALE_FACTOR,
|
||||
tile_overlap_factor=0.25,
|
||||
)
|
||||
|
||||
# non_noised_latents_from_image
|
||||
image_tensor = image_tensor.to(device=vae.device, dtype=vae.dtype)
|
||||
with torch.inference_mode():
|
||||
with torch.inference_mode(), tiling_context:
|
||||
latents = ImageToLatentsInvocation._encode_to_tensor(vae, image_tensor)
|
||||
|
||||
latents = vae.config.scaling_factor * latents
|
||||
@ -101,7 +117,9 @@ class ImageToLatentsInvocation(BaseInvocation):
|
||||
if image_tensor.dim() == 3:
|
||||
image_tensor = einops.rearrange(image_tensor, "c h w -> 1 c h w")
|
||||
|
||||
latents = self.vae_encode(vae_info, self.fp32, self.tiled, image_tensor)
|
||||
latents = self.vae_encode(
|
||||
vae_info=vae_info, upcast=self.fp32, tiled=self.tiled, image_tensor=image_tensor, tile_size=self.tile_size
|
||||
)
|
||||
|
||||
latents = latents.to("cpu")
|
||||
name = context.tensors.save(tensor=latents)
|
||||
|
@ -1,3 +1,5 @@
|
||||
from contextlib import nullcontext
|
||||
|
||||
import torch
|
||||
from diffusers.image_processor import VaeImageProcessor
|
||||
from diffusers.models.attention_processor import (
|
||||
@ -8,10 +10,9 @@ from diffusers.models.attention_processor import (
|
||||
)
|
||||
from diffusers.models.autoencoders.autoencoder_kl import AutoencoderKL
|
||||
from diffusers.models.autoencoders.autoencoder_tiny import AutoencoderTiny
|
||||
from diffusers.models.unets.unet_2d_condition import UNet2DConditionModel
|
||||
|
||||
from invokeai.app.invocations.baseinvocation import BaseInvocation, invocation
|
||||
from invokeai.app.invocations.constants import DEFAULT_PRECISION
|
||||
from invokeai.app.invocations.constants import DEFAULT_PRECISION, LATENT_SCALE_FACTOR
|
||||
from invokeai.app.invocations.fields import (
|
||||
FieldDescriptions,
|
||||
Input,
|
||||
@ -24,6 +25,7 @@ from invokeai.app.invocations.model import VAEField
|
||||
from invokeai.app.invocations.primitives import ImageOutput
|
||||
from invokeai.app.services.shared.invocation_context import InvocationContext
|
||||
from invokeai.backend.stable_diffusion import set_seamless
|
||||
from invokeai.backend.stable_diffusion.vae_tiling import patch_vae_tiling_params
|
||||
from invokeai.backend.util.devices import TorchDevice
|
||||
|
||||
|
||||
@ -32,7 +34,7 @@ from invokeai.backend.util.devices import TorchDevice
|
||||
title="Latents to Image",
|
||||
tags=["latents", "image", "vae", "l2i"],
|
||||
category="latents",
|
||||
version="1.2.2",
|
||||
version="1.3.0",
|
||||
)
|
||||
class LatentsToImageInvocation(BaseInvocation, WithMetadata, WithBoard):
|
||||
"""Generates an image from latents."""
|
||||
@ -46,6 +48,9 @@ class LatentsToImageInvocation(BaseInvocation, WithMetadata, WithBoard):
|
||||
input=Input.Connection,
|
||||
)
|
||||
tiled: bool = InputField(default=False, description=FieldDescriptions.tiled)
|
||||
# NOTE: tile_size = 0 is a special value. We use this rather than `int | None`, because the workflow UI does not
|
||||
# offer a way to directly set None values.
|
||||
tile_size: int = InputField(default=0, multiple_of=8, description=FieldDescriptions.vae_tile_size)
|
||||
fp32: bool = InputField(default=DEFAULT_PRECISION == torch.float32, description=FieldDescriptions.fp32)
|
||||
|
||||
@torch.no_grad()
|
||||
@ -53,9 +58,9 @@ class LatentsToImageInvocation(BaseInvocation, WithMetadata, WithBoard):
|
||||
latents = context.tensors.load(self.latents.latents_name)
|
||||
|
||||
vae_info = context.models.load(self.vae.vae)
|
||||
assert isinstance(vae_info.model, (UNet2DConditionModel, AutoencoderKL, AutoencoderTiny))
|
||||
assert isinstance(vae_info.model, (AutoencoderKL, AutoencoderTiny))
|
||||
with set_seamless(vae_info.model, self.vae.seamless_axes), vae_info as vae:
|
||||
assert isinstance(vae, torch.nn.Module)
|
||||
assert isinstance(vae, (AutoencoderKL, AutoencoderTiny))
|
||||
latents = latents.to(vae.device)
|
||||
if self.fp32:
|
||||
vae.to(dtype=torch.float32)
|
||||
@ -87,10 +92,19 @@ class LatentsToImageInvocation(BaseInvocation, WithMetadata, WithBoard):
|
||||
else:
|
||||
vae.disable_tiling()
|
||||
|
||||
tiling_context = nullcontext()
|
||||
if self.tile_size > 0:
|
||||
tiling_context = patch_vae_tiling_params(
|
||||
vae,
|
||||
tile_sample_min_size=self.tile_size,
|
||||
tile_latent_min_size=self.tile_size // LATENT_SCALE_FACTOR,
|
||||
tile_overlap_factor=0.25,
|
||||
)
|
||||
|
||||
# clear memory as vae decode can request a lot
|
||||
TorchDevice.empty_cache()
|
||||
|
||||
with torch.inference_mode():
|
||||
with torch.inference_mode(), tiling_context:
|
||||
# copied from diffusers pipeline
|
||||
latents = latents / vae.config.scaling_factor
|
||||
image = vae.decode(latents, return_dict=False)[0]
|
||||
|
35
invokeai/backend/stable_diffusion/vae_tiling.py
Normal file
35
invokeai/backend/stable_diffusion/vae_tiling.py
Normal file
@ -0,0 +1,35 @@
|
||||
from contextlib import contextmanager
|
||||
|
||||
from diffusers.models.autoencoders.autoencoder_kl import AutoencoderKL
|
||||
from diffusers.models.autoencoders.autoencoder_tiny import AutoencoderTiny
|
||||
|
||||
|
||||
@contextmanager
|
||||
def patch_vae_tiling_params(
|
||||
vae: AutoencoderKL | AutoencoderTiny,
|
||||
tile_sample_min_size: int,
|
||||
tile_latent_min_size: int,
|
||||
tile_overlap_factor: float,
|
||||
):
|
||||
"""Patch the parameters that control the VAE tiling tile size and overlap.
|
||||
|
||||
These parameters are not explicitly exposed in the VAE's API, but they have a significant impact on the quality of
|
||||
the outputs. As a general rule, bigger tiles produce better results, but this comes at the cost of higher memory
|
||||
usage.
|
||||
"""
|
||||
# Record initial config.
|
||||
orig_tile_sample_min_size = vae.tile_sample_min_size
|
||||
orig_tile_latent_min_size = vae.tile_latent_min_size
|
||||
orig_tile_overlap_factor = vae.tile_overlap_factor
|
||||
|
||||
try:
|
||||
# Apply target config.
|
||||
vae.tile_sample_min_size = tile_sample_min_size
|
||||
vae.tile_latent_min_size = tile_latent_min_size
|
||||
vae.tile_overlap_factor = tile_overlap_factor
|
||||
yield
|
||||
finally:
|
||||
# Restore initial config.
|
||||
vae.tile_sample_min_size = orig_tile_sample_min_size
|
||||
vae.tile_latent_min_size = orig_tile_latent_min_size
|
||||
vae.tile_overlap_factor = orig_tile_overlap_factor
|
13
tests/backend/stable_diffusion/test_vae_tiling.py
Normal file
13
tests/backend/stable_diffusion/test_vae_tiling.py
Normal file
@ -0,0 +1,13 @@
|
||||
from diffusers.models.autoencoders.autoencoder_kl import AutoencoderKL
|
||||
|
||||
from invokeai.backend.stable_diffusion.vae_tiling import patch_vae_tiling_params
|
||||
|
||||
|
||||
def test_patch_vae_tiling_params():
|
||||
"""Smoke test the patch_vae_tiling_params(...) context manager. The main purpose of this unit test is to detect if
|
||||
diffusers ever changes the attributes of the AutoencoderKL class that we expect to exist.
|
||||
"""
|
||||
vae = AutoencoderKL()
|
||||
|
||||
with patch_vae_tiling_params(vae, 1, 2, 3):
|
||||
pass
|
Loading…
Reference in New Issue
Block a user