* new OffloadingDevice loads one model at a time, on demand
* fixup! new OffloadingDevice loads one model at a time, on demand
* fix(prompt_to_embeddings): call the text encoder directly instead of its forward method
allowing any associated hooks to run with it.
* more attempts to get things on the right device from the offloader
* more attempts to get things on the right device from the offloader
* make offloading methods an explicit part of the pipeline interface
* inlining some calls where device is only used once
* ensure model group is ready after pipeline.to is called
* fixup! Strategize slicing based on free [V]RAM (#2572)
* doc(offloading): docstrings for offloading.ModelGroup
* doc(offloading): docstrings for offloading-related pipeline methods
* refactor(offloading): s/SimpleModelGroup/FullyLoadedModelGroup
* refactor(offloading): s/HotSeatModelGroup/LazilyLoadedModelGroup
to frame it is the same terms as "FullyLoadedModelGroup"
---------
Co-authored-by: Damian Stewart <null@damianstewart.com>
- filter paths for `build-container.yml` and `test-invoke-pip.yml`
- add workflow to pass required checks on PRs with `paths-ignore`
- this triggers if `test-invoke-pip.yml` does not
- fix "CI checks on main link" in `/README.md`
- filter paths for `build-container.yml` and `test-invoke-pip.yml`
- add workflow to pass required checks on PRs with `paths-ignore`
- this triggers if `test-invoke-pip.yml` does not
- fix "CI checks on main link" in `/README.md`
Assuming that mixing `"literal strings"` and `{'JSX expressions'}`
throughout the code is not for a explicit reason but just a result IDE
autocompletion, I changed all props to be consistent with the
conventional style of using simple string literals where it is
sufficient.
This is a somewhat trivial change, but it makes the code a little more
readable and uniform
### WebUI Model Conversion
**Model Search Updates**
- Model Search now has a radio group that allows users to pick the type
of model they are importing. If they know their model has a custom
config file, they can assign it right here. Based on their pick, the
model config data is automatically populated. And this same information
is used when converting the model to `diffusers`.
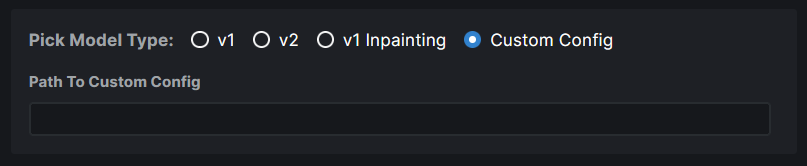
- Files named `model.safetensors` and
`diffusion_pytorch_model.safetensors` are excluded from the search
because these are naming conventions used by diffusers models and they
will end up showing on the list because our conversion saves safetensors
and not bin files.
**Model Conversion UI**
- The **Convert To Diffusers** button can be found on the Edit page of
any **Checkpoint Model**.
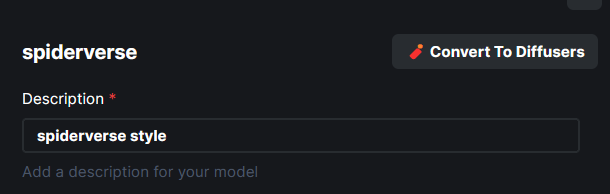
- When converting the model, the entire process is handled
automatically. The corresponding config while at the time of the Ckpt
addition is used in the process.
- Users are presented with the choice on where to save the diffusers
converted model - same location as the ckpt, InvokeAI models root folder
or a completely custom location.
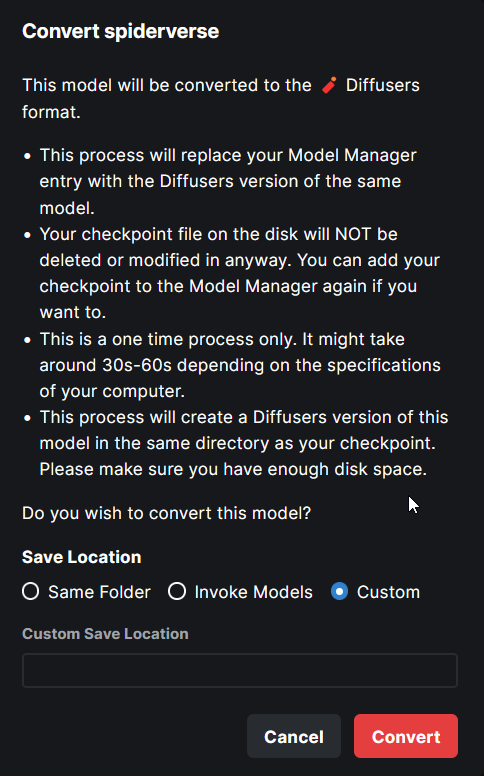
- When the model is converted, the checkpoint entry is replaced with the
diffusers model entry. A user can readd the ckpt if they wish to.
---
More or less done. Might make some minor UX improvements as I refine
things.
Tensors with diffusers no longer have to be multiples of 8. This broke Perlin noise generation. We now generate noise for the next largest multiple of 8 and return a cropped result. Fixes#2674.
`generator` now asks `InvokeAIDiffuserComponent` to do postprocessing work on latents after every step. Thresholding - now implemented as replacing latents outside of the threshold with random noise - is called at this point. This postprocessing step is also where we can hook up symmetry and other image latent manipulations in the future.
Note: code at this layer doesn't need to worry about MPS as relevant torch functions are wrapped and made MPS-safe by `generator.py`.
1. Now works with sites that produce lots of redirects, such as CIVITAI
2. Derive name of destination model file from HTTP Content-Disposition header,
if present.
3. Swap \\ for / in file paths provided by users, to hopefully fix issues with
Windows.
This PR adds a new attributer to ldm.generate, `embedding_trigger_strings`:
```
gen = Generate(...)
strings = gen.embedding_trigger_strings
strings = gen.embedding_trigger_strings()
```
The trigger strings will change when the model is updated to show only
those strings which are compatible with the current
model. Dynamically-downloaded triggers from the HF Concepts Library
will only show up after they are used for the first time. However, the
full list of concepts available for download can be retrieved
programatically like this:
```
from ldm.invoke.concepts_lib import HuggingFAceConceptsLibrary
concepts = HuggingFaceConceptsLibrary()
trigger_strings = concepts.list_concepts()
```
I have added the arabic locale files. There need to be some
modifications to the code in order to detect the language direction and
add it to the current document body properties.
For example we can use this:
import { appWithTranslation, useTranslation } from "next-i18next";
import React, { useEffect } from "react";
const { t, i18n } = useTranslation();
const direction = i18n.dir();
useEffect(() => {
document.body.dir = direction;
}, [direction]);
This should be added to the app file. It uses next-i18next to
automatically get the current language and sets the body text direction
(ltr or rtl) depending on the selected language.